Effective Groundwater Model Calibration (eBook, PDF)
With Analysis of Data, Sensitivities, Predictions, and Uncertainty
Alle Infos zum eBook verschenken
Effective Groundwater Model Calibration (eBook, PDF)
With Analysis of Data, Sensitivities, Predictions, and Uncertainty
- Format: PDF
- Merkliste
- Auf die Merkliste
- Bewerten Bewerten
- Teilen
- Produkt teilen
- Produkterinnerung
- Produkterinnerung
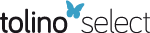
Hier können Sie sich einloggen
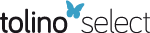
Bitte loggen Sie sich zunächst in Ihr Kundenkonto ein oder registrieren Sie sich bei bücher.de, um das eBook-Abo tolino select nutzen zu können.
Methods and guidelines for developing and using mathematical models Turn to Effective Groundwater Model Calibration for a set of methods and guidelines that can help produce more accurate and transparent mathematical models. The models can represent groundwater flow and transport and other natural and engineered systems. Use this book and its extensive exercises to learn methods to fully exploit the data on hand, maximize the model's potential, and troubleshoot any problems that arise. Use the methods to perform: * Sensitivity analysis to evaluate the information content of data * Data…mehr
- Geräte: PC
- mit Kopierschutz
- eBook Hilfe
- Größe: 8.88MB
- George F. PinderEssentials of Multiphase Flow and Transport in Porous Media (eBook, PDF)131,99 €
- David A. ChinWater-Quality Engineering in Natural Systems (eBook, PDF)123,99 €
- Jane KuceraDesalination (eBook, PDF)214,99 €
- The Water Framework Directive (eBook, PDF)232,99 €
- Analytical Methods for Drinking Water (eBook, PDF)166,99 €
- Michael H. GerardiThe Microbiology of Anaerobic Digesters (eBook, PDF)70,99 €
- Werner StummAquatic Chemistry (eBook, PDF)105,99 €
-
-
-
Dieser Download kann aus rechtlichen Gründen nur mit Rechnungsadresse in A, B, BG, CY, CZ, D, DK, EW, E, FIN, F, GR, HR, H, IRL, I, LT, L, LR, M, NL, PL, P, R, S, SLO, SK ausgeliefert werden.
- Produktdetails
- Verlag: Wiley-Blackwell
- Seitenzahl: 480
- Erscheinungstermin: 19. Juli 2007
- Englisch
- ISBN-13: 9780470041079
- Artikelnr.: 37290057
- Verlag: Wiley-Blackwell
- Seitenzahl: 480
- Erscheinungstermin: 19. Juli 2007
- Englisch
- ISBN-13: 9780470041079
- Artikelnr.: 37290057
- Herstellerkennzeichnung Die Herstellerinformationen sind derzeit nicht verfügbar.
1 Introduction.
1.1 Book and Associated Contributions: Methods, Guidelines, Exercises,
Answers, Software, and PowerPoint Files.
1.2 Model Calibration with Inverse Modeling.
1.2.1 Parameterization.
1.2.2 Objective Function.
1.2.3 Utility of Inverse Modeling and Associated Methods.
1.2.4 Using the Model to Quantitatively Connect Parameters, Observations,
and Predictions.
1.3 Relation of this Book to Other Ideas and Previous Works.
1.3.1 Predictive Versus Calibrated Models.
1.3.2 Previous Work.
1.4 A Few Definitions.
1.4.1 Linear and Nonlinear.
1.4.2 Precision, Accuracy, Reliability, and Uncertainty.
1.5 Advantageous Expertise and Suggested Readings.
1.6 Overview of Chapters 2 Through 15.
2 Computer Software and Groundwater Management Problem Used in the
Exercises.
2.1 Computer Programs MODFLOW-2000, UCODE_2005, and PEST.
2.2 Groundwater Management Problem Used for the Exercises.
2.2.1 Purpose and Strategy.
2.2.2 Flow System Characteristics.
2.3 Exercises.
Exercise 2.1: Simulate Steady-State Heads and Perform Preparatory Steps.
3 Comparing Observed and Simulated Values Using Objective Functions.
3.1 Weighted Least-Squares Objective Function.
3.1.1 With a Diagonal Weight Matrix.
3.1.2 With a Full Weight Matrix.
3.2 Alternative Objective Functions.
3.2.1 Maximum-Likelihood Objective Function.
3.2.2 L1 Norm Objective Function.
3.2.3 Multiobjective Function.
3.3 Requirements for Accurate Simulated Results.
3.3.1 Accurate Model.
3.3.2 Unbiased Observations and Prior Information.
3.3.3 Weighting Reflects Errors.
3.4 Additional Issues.
3.4.1 Prior Information.
3.4.2 Weighting.
3.4.3 Residuals and Weighted Residuals.
3.5 Least-Squares Objective-Function Surfaces.
3.6 Exercises.
Exercise 3.1: Steady-State Parameter Definition.
Exercise 3.2: Observations for the Steady-State Problem.
Exercise 3.3: Evaluate Model Fit Using Starting Parameter Values.
4 Determining the Information that Observations Provide on Parameter Values
using Fit-Independent Statistics.
4.1 Using Observations.
4.1.1 Model Construction and Parameter Definition.
4.1.2 Parameter Values.
4.2 When to Determine the Information that Observations Provide About
Parameter Values.
4.3 Fit-Independent Statistics for Sensitivity Analysis.
4.3.1 Sensitivities.
4.3.2 Scaling.
4.3.3 Dimensionless Scaled Sensitivities (dss).
4.3.4 Composite Scaled Sensitivities (css).
4.3.5 Parameter Correlation Coefficients (pcc).
4.3.6 Leverage Statistics.
4.3.7 One-Percent Scaled Sensitivities.
4.4 Advantages and Limitations of Fit-Independent Statistics for
Sensitivity Analysis.
4.4.1 Scaled Sensitivities.
4.4.2 Parameter Correlation Coefficients.
4.4.3 Leverage Statistics.
4.5 Exercises.
Exercise 4.1: Sensitivity Analysis for the Steady-State Model with Starting
Parameter Values.
5 Estimating Parameter Values.
5.1 The Modified Gauss-Newton Gradient Method.
5.1.1 Normal Equations.
5.1.2 An Example.
5.1.3 Convergence Criteria.
5.2 Alternative Optimization Methods.
5.3 Multiobjective Optimization.
5.4 Log-Transformed Parameters.
5.5 Use of Limits on Estimated Parameter Values.
5.6 Exercises.
Exercise 5.1: Modified Gauss-Newton Method and Application to a
Two-Parameter Problem.
Exercise 5.2: Estimate the Parameters of the Steady-State Model.
6 Evaluating Model Fit.
6.1 Magnitude of Residuals and Weighted Residuals.
6.2 Identify Systematic Misfit.
6.3 Measures of Overall Model Fit.
6.3.1 Objective-Function Value.
6.3.2 Calculated Error Variance and Standard Error.
6.3.3 AIC, AICc, and BIC Statistics.
6.4 Analyzing Model Fit Graphically and Related Statistics.
6.4.1 Using Graphical Analysis of Weighted Residuals to Detect Model Error.
6.4.2 Weighted Residuals Versus Weighted or Unweighted Simulated Values and
Minimum, Maximum, and Average Weighted Residuals.
6.4.3 Weighted or Unweighted Observations Versus Simulated Values and
Correlation Coefficient R.
6.4.4 Graphs and Maps Using Independent Variables and the Runs Statistic.
6.4.5 Normal Probability Graphs and Correlation Coefficient RN2.
6.4.6 Acceptable Deviations from Random, Normally Distributed Weighted
Residuals.
6.5 Exercises.
Exercise 6.1: Statistical Measures of Overall Fit.
Exercise 6.2: Evaluate Graph Model fit and Related Statistics.
7 Evaluating Estimated Parameter Values and Parameter Uncertainty.
7.1 Reevaluating Composite Scaled Sensitivities.
7.2 Using Statistics from the Parameter Variance-Covariance Matrix.
7.2.1 Five Versions of the Variance-Covariance Matrix.
7.2.2 Parameter Variances, Covariances, Standard Deviations, Coefficients
of Variation, and Correlation Coefficients.
7.2.3 Relation Between Sample and Regression Statistics.
7.2.4 Statistics for Log-Transformed Parameters.
7.2.5 When to Use the Five Versions of the Parameter Variance-Covariance
Matrix.
7.2.6 Some Alternate Methods: Eigenvectors, Eigenvalues, and Singular Value
Decomposition.
7.3 Identifying Observations Important to Estimated Parameter Values.
7.3.1 Leverage Statistics.
7.3.2 Influence Statistics.
7.4 Uniqueness and Optimality of the Estimated Parameter Values.
7.5 Quantifying Parameter Value Uncertainty.
7.5.1 Inferential Statistics.
7.5.2 Monte Carlo Methods.
7.6 Checking Parameter Estimates Against Reasonable Values.
7.7 Testing Linearity.
7.8 Exercises.
Exercise 7.1: Parameter Statistics.
Exercise 7.2: Consider All the Different Correlation Coefficients
Presented.
Exercise 7.3: Test for Linearity.
8 Evaluating Model Predictions, Data Needs, and Prediction Uncertainty.
8.1 Simulating Predictions and Prediction Sensitivities and Standard
Deviations.
8.2 Using Predictions to Guide Collection of Data that Directly
Characterize System Properties.
8.2.1 Prediction Scaled Sensitivities (pss).
8.2.2 Prediction Scaled Sensitivities Used in Conjunction with Composite
Scaled Sensitivities.
8.2.3 Parameter Correlation Coefficients without and with Predictions.
8.2.4 Composite and Prediction Scaled Sensitivities Used with Parameter
Correlation Coefficients.
8.2.5 Parameter-Prediction ( ppr) Statistic.
8.3 Using Predictions to Guide Collection of Observation Data.
8.3.1 Use of Prediction, Composite, and Dimensionless Scaled Sensitivities
and Parameter Correlation Coefficients.
8.3.2 Observation-Prediction (opr) Statistic.
8.3.3 Insights About the opr Statistic from Other Fit-Independent
Statistics.
8.3.4 Implications for Monitoring Network Design.
8.4 Quantifying Prediction Uncertainty Using Inferential Statistics.
8.4.1 Definitions.
8.4.2 Linear Confidence and Prediction Intervals on Predictions.
8.4.3 Nonlinear Confidence and Prediction Intervals.
8.4.4 Using the Theis Example to Understand Linear and Nonlinear Confidence
Intervals.
8.4.5 Differences and Their Standard Deviations, Confidence Intervals, and
Prediction Intervals.
8.4.6 Using Confidence Intervals to Serve the Purposes of Traditional
Sensitivity Analysis.
8.5 Quantifying Prediction Uncertainty Using Monte Carlo Analysis.
8.5.1 Elements of a Monte Carlo Analysis.
8.5.2 Relation Between Monte Carlo Analysis and Linear and Nonlinear
Confidence Intervals.
8.5.3 Using the Theis Example to Understand Monte Carlo Methods.
8.6 Quantifying Prediction Uncertainty Using Alternative Models.
8.7 Testing Model Nonlinearity with Respect to the Predictions.
8.8 Exercises.
Exercise 8.1: Predict Advective Transport and Perform Sensitivity Analysis.
Exercise 8.2: Prediction Uncertainty Measured Using Inferential Statistics.
9 Calibrating Transient and Transport Models and Recalibrating Existing
Models.
9.1 Strategies for Calibrating Transient Models.
9.1.1 Initial Conditions.
9.1.2 Transient Observations.
9.1.3 Additional Model Inputs.
9.2 Strategies for Calibrating Transport Models.
9.2.1 Selecting Processes to Include.
9.2.2 Defining Source Geometry and Concentrations.
9.2.3 Scale Issues.
9.2.4 Numerical Issues: Model Accuracy and Execution Time.
9.2.5 Transport Observations.
9.2.6 Additional Model Inputs.
9.2.7 Examples of Obtaining a Tractable, Useful Model.
9.3 Strategies for Recalibrating Existing Models.
9.4 Exercises (optional).
Exercises 9.1 and 9.2: Simulate Transient Hydraulic Heads and Perform
Preparatory Steps.
Exercise 9.3: Transient Parameter Definition.
Exercise 9.4: Observations for the Transient Problem.
Exercise 9.5: Evaluate Transient Model Fit Using Starting Parameter Values.
Exercise 9.6: Sensitivity Analysis for the Initial Model.
Exercise 9.7: Estimate Parameters for the Transient System by Nonlinear
Regression.
Exercise 9.8: Evaluate Measures of Model Fit.
Exercise 9.9: Perform Graphical Analyses of Model Fit and Evaluate Related
Statistics.
Exercise 9.10: Evaluate Estimated Parameters.
Exercise 9.11: Test for Linearity.
Exercise 9.12: Predictions.
10 Guidelines for Effective Modeling.
10.1 Purpose of the Guidelines.
10.2 Relation to Previous Work.
10.3 Suggestions for Effective Implementation.
11 Guidelines 1 Through 8-Model Development.
Guideline 1: Apply the Principle of Parsimony.
G1.1 Problem.
G1.2 Constructive Approaches.
Guideline 2: Use a Broad Range of System Information to Constrain the
Problem.
G2.1 Data Assimilation.
G2.2 Using System Information.
G2.3 Data Management.
G2.4 Application: Characterizing a Fractured Dolomite Aquifer.
Guideline 3: Maintain a Well-Posed, Comprehensive Regression Problem.
G3.1 Examples.
G3.2 Effects of Nonlinearity on the css and pcc.
Guideline 4: Include Many Kinds of Data as Observations in the Regression.
G4.1 Interpolated "Observations".
G4.2 Clustered Observations.
G4.3 Observations that Are Inconsistent with Model Construction.
G4.4 Applications: Using Different Types of Observations to Calibrate
Groundwater Flow and Transport Models.
Guideline 5: Use Prior Information Carefully.
G5.1 Use of Prior Information Compared with Observations.
G5.2 Highly Parameterized Models.
G5.3 Applications: Geophysical Data.
Guideline 6: Assign Weights that Reflect Errors.
G6.1 Determine Weights.
G6.2 Issues of Weighting in Nonlinear Regression.
Guideline 7: Encourage Convergence by Making the Model More Accurate and
Evaluating the Observations.
Guideline 8: Consider Alternative Models.
G8.1 Develop Alternative Models.
G8.2 Discriminate Between Models.
G8.3 Simulate Predictions with Alternative Models.
G8.4 Application.
12 Guidelines 9 and 10-Model Testing.
Guideline 9: Evaluate Model Fit.
G9.1 Determine Model Fit.
G9.2 Examine Fit for Existing Observations Important to the Purpose of the
Model.
G9.3 Diagnose the Cause of Poor Model Fit.
Guideline 10: Evaluate Optimized Parameter Values.
G10.1 Quantify Parameter-Value Uncertainty.
G10.2 Use Parameter Estimates to Detect Model Error.
G10.3 Diagnose the Cause of Unreasonable Optimal Parameter Estimates.
G10.4 Identify Observations Important to the Parameter Estimates.
G10.5 Reduce or Increase the Number of Parameters.
13 Guidelines 11 and 12-Potential New Data.
Guideline 11: Identify New Data to Improve Simulated Processes, Features,
and Properties.
Guideline 12: Identify New Data to Improve Predictions.
G12.1 Potential New Data to Improve Features and Properties Governing
System Dynamics.
G12.2 Potential New Data to Support Observations.
14 Guidelines 13 and 14-Prediction Uncertainty.
Guideline 13: Evaluate Prediction Uncertainty and Accuracy Using
Deterministic Methods.
G13.1 Use Regression to Determine Whether Predicted Values Are Contradicted
by the Calibrated Model.
G13.2 Use Omitted Data and Postaudits.
Guideline 14: Quantify Prediction Uncertainty Using Statistical Methods.
G14.1 Inferential Statistics.
G14.2 Monte Carlo Methods.
15 Using and Testing the Methods and Guidelines.
15.1 Execution Time Issues.
15.2 Field Applications and Synthetic Test Cases.
15.2.1 The Death Valley Regional Flow System, California and Nevada, USA.
15.2.2 Grindsted Landfill, Denmark.
Appendix A: Objective Function Issues.
A.1 Derivation of the Maximum-Likelihood Objective Function.
A.2 Relation of the Maximum-Likelihood and Least-Squares Objective
Functions.
A.3 Assumptions Required for Diagonal Weighting to be Correct.
A.4 References.
Appendix B: Calculation Details of the Modified Gauss-Newton Method.
B.1 Vectors and Matrices for Nonlinear Regression.
B.2 Quasi-Newton Updating of the Normal Equations.
B.3 Calculating the Damping Parameter.
B.4 Solving the Normal Equations.
B.5 References.
Appendix C: Two Important Properties of Linear Regression and the Effects
of Nonlinearity.
C.1 Identities Needed for the Proofs.
C.1.1 True Linear Model.
C.1.2 True Nonlinear Model.
C.1.3 Linearized True Nonlinear Model.
C.1.4 Approximate Linear Model.
C.1.5 Approximate Nonlinear Model.
C.1.6 Linearized Approximate Nonlinear Model.
C.1.7 The Importance of X and X.
C.1.8 Considering Many Observations.
C.1.9 Normal Equations.
C.1.10 Random Variables.
C.1.11 Expected Value.
C.1.12 Variance-Covariance Matrix of a Vector.
C.2 Proof of Property 1: Parameters Estimated by Linear Regression are
Unbiased.
C.3 Proof of Property 2: The Weight Matrix Needs to be Defined in a
Particular Way for Eq. (7.1) to Apply and for the Parameter Estimates to
have the Smallest Variance.
C.4 References.
Appendix D: Selected Statistical Tables.
D.1 References.
References.
Index.
1 Introduction.
1.1 Book and Associated Contributions: Methods, Guidelines, Exercises,
Answers, Software, and PowerPoint Files.
1.2 Model Calibration with Inverse Modeling.
1.2.1 Parameterization.
1.2.2 Objective Function.
1.2.3 Utility of Inverse Modeling and Associated Methods.
1.2.4 Using the Model to Quantitatively Connect Parameters, Observations,
and Predictions.
1.3 Relation of this Book to Other Ideas and Previous Works.
1.3.1 Predictive Versus Calibrated Models.
1.3.2 Previous Work.
1.4 A Few Definitions.
1.4.1 Linear and Nonlinear.
1.4.2 Precision, Accuracy, Reliability, and Uncertainty.
1.5 Advantageous Expertise and Suggested Readings.
1.6 Overview of Chapters 2 Through 15.
2 Computer Software and Groundwater Management Problem Used in the
Exercises.
2.1 Computer Programs MODFLOW-2000, UCODE_2005, and PEST.
2.2 Groundwater Management Problem Used for the Exercises.
2.2.1 Purpose and Strategy.
2.2.2 Flow System Characteristics.
2.3 Exercises.
Exercise 2.1: Simulate Steady-State Heads and Perform Preparatory Steps.
3 Comparing Observed and Simulated Values Using Objective Functions.
3.1 Weighted Least-Squares Objective Function.
3.1.1 With a Diagonal Weight Matrix.
3.1.2 With a Full Weight Matrix.
3.2 Alternative Objective Functions.
3.2.1 Maximum-Likelihood Objective Function.
3.2.2 L1 Norm Objective Function.
3.2.3 Multiobjective Function.
3.3 Requirements for Accurate Simulated Results.
3.3.1 Accurate Model.
3.3.2 Unbiased Observations and Prior Information.
3.3.3 Weighting Reflects Errors.
3.4 Additional Issues.
3.4.1 Prior Information.
3.4.2 Weighting.
3.4.3 Residuals and Weighted Residuals.
3.5 Least-Squares Objective-Function Surfaces.
3.6 Exercises.
Exercise 3.1: Steady-State Parameter Definition.
Exercise 3.2: Observations for the Steady-State Problem.
Exercise 3.3: Evaluate Model Fit Using Starting Parameter Values.
4 Determining the Information that Observations Provide on Parameter Values
using Fit-Independent Statistics.
4.1 Using Observations.
4.1.1 Model Construction and Parameter Definition.
4.1.2 Parameter Values.
4.2 When to Determine the Information that Observations Provide About
Parameter Values.
4.3 Fit-Independent Statistics for Sensitivity Analysis.
4.3.1 Sensitivities.
4.3.2 Scaling.
4.3.3 Dimensionless Scaled Sensitivities (dss).
4.3.4 Composite Scaled Sensitivities (css).
4.3.5 Parameter Correlation Coefficients (pcc).
4.3.6 Leverage Statistics.
4.3.7 One-Percent Scaled Sensitivities.
4.4 Advantages and Limitations of Fit-Independent Statistics for
Sensitivity Analysis.
4.4.1 Scaled Sensitivities.
4.4.2 Parameter Correlation Coefficients.
4.4.3 Leverage Statistics.
4.5 Exercises.
Exercise 4.1: Sensitivity Analysis for the Steady-State Model with Starting
Parameter Values.
5 Estimating Parameter Values.
5.1 The Modified Gauss-Newton Gradient Method.
5.1.1 Normal Equations.
5.1.2 An Example.
5.1.3 Convergence Criteria.
5.2 Alternative Optimization Methods.
5.3 Multiobjective Optimization.
5.4 Log-Transformed Parameters.
5.5 Use of Limits on Estimated Parameter Values.
5.6 Exercises.
Exercise 5.1: Modified Gauss-Newton Method and Application to a
Two-Parameter Problem.
Exercise 5.2: Estimate the Parameters of the Steady-State Model.
6 Evaluating Model Fit.
6.1 Magnitude of Residuals and Weighted Residuals.
6.2 Identify Systematic Misfit.
6.3 Measures of Overall Model Fit.
6.3.1 Objective-Function Value.
6.3.2 Calculated Error Variance and Standard Error.
6.3.3 AIC, AICc, and BIC Statistics.
6.4 Analyzing Model Fit Graphically and Related Statistics.
6.4.1 Using Graphical Analysis of Weighted Residuals to Detect Model Error.
6.4.2 Weighted Residuals Versus Weighted or Unweighted Simulated Values and
Minimum, Maximum, and Average Weighted Residuals.
6.4.3 Weighted or Unweighted Observations Versus Simulated Values and
Correlation Coefficient R.
6.4.4 Graphs and Maps Using Independent Variables and the Runs Statistic.
6.4.5 Normal Probability Graphs and Correlation Coefficient RN2.
6.4.6 Acceptable Deviations from Random, Normally Distributed Weighted
Residuals.
6.5 Exercises.
Exercise 6.1: Statistical Measures of Overall Fit.
Exercise 6.2: Evaluate Graph Model fit and Related Statistics.
7 Evaluating Estimated Parameter Values and Parameter Uncertainty.
7.1 Reevaluating Composite Scaled Sensitivities.
7.2 Using Statistics from the Parameter Variance-Covariance Matrix.
7.2.1 Five Versions of the Variance-Covariance Matrix.
7.2.2 Parameter Variances, Covariances, Standard Deviations, Coefficients
of Variation, and Correlation Coefficients.
7.2.3 Relation Between Sample and Regression Statistics.
7.2.4 Statistics for Log-Transformed Parameters.
7.2.5 When to Use the Five Versions of the Parameter Variance-Covariance
Matrix.
7.2.6 Some Alternate Methods: Eigenvectors, Eigenvalues, and Singular Value
Decomposition.
7.3 Identifying Observations Important to Estimated Parameter Values.
7.3.1 Leverage Statistics.
7.3.2 Influence Statistics.
7.4 Uniqueness and Optimality of the Estimated Parameter Values.
7.5 Quantifying Parameter Value Uncertainty.
7.5.1 Inferential Statistics.
7.5.2 Monte Carlo Methods.
7.6 Checking Parameter Estimates Against Reasonable Values.
7.7 Testing Linearity.
7.8 Exercises.
Exercise 7.1: Parameter Statistics.
Exercise 7.2: Consider All the Different Correlation Coefficients
Presented.
Exercise 7.3: Test for Linearity.
8 Evaluating Model Predictions, Data Needs, and Prediction Uncertainty.
8.1 Simulating Predictions and Prediction Sensitivities and Standard
Deviations.
8.2 Using Predictions to Guide Collection of Data that Directly
Characterize System Properties.
8.2.1 Prediction Scaled Sensitivities (pss).
8.2.2 Prediction Scaled Sensitivities Used in Conjunction with Composite
Scaled Sensitivities.
8.2.3 Parameter Correlation Coefficients without and with Predictions.
8.2.4 Composite and Prediction Scaled Sensitivities Used with Parameter
Correlation Coefficients.
8.2.5 Parameter-Prediction ( ppr) Statistic.
8.3 Using Predictions to Guide Collection of Observation Data.
8.3.1 Use of Prediction, Composite, and Dimensionless Scaled Sensitivities
and Parameter Correlation Coefficients.
8.3.2 Observation-Prediction (opr) Statistic.
8.3.3 Insights About the opr Statistic from Other Fit-Independent
Statistics.
8.3.4 Implications for Monitoring Network Design.
8.4 Quantifying Prediction Uncertainty Using Inferential Statistics.
8.4.1 Definitions.
8.4.2 Linear Confidence and Prediction Intervals on Predictions.
8.4.3 Nonlinear Confidence and Prediction Intervals.
8.4.4 Using the Theis Example to Understand Linear and Nonlinear Confidence
Intervals.
8.4.5 Differences and Their Standard Deviations, Confidence Intervals, and
Prediction Intervals.
8.4.6 Using Confidence Intervals to Serve the Purposes of Traditional
Sensitivity Analysis.
8.5 Quantifying Prediction Uncertainty Using Monte Carlo Analysis.
8.5.1 Elements of a Monte Carlo Analysis.
8.5.2 Relation Between Monte Carlo Analysis and Linear and Nonlinear
Confidence Intervals.
8.5.3 Using the Theis Example to Understand Monte Carlo Methods.
8.6 Quantifying Prediction Uncertainty Using Alternative Models.
8.7 Testing Model Nonlinearity with Respect to the Predictions.
8.8 Exercises.
Exercise 8.1: Predict Advective Transport and Perform Sensitivity Analysis.
Exercise 8.2: Prediction Uncertainty Measured Using Inferential Statistics.
9 Calibrating Transient and Transport Models and Recalibrating Existing
Models.
9.1 Strategies for Calibrating Transient Models.
9.1.1 Initial Conditions.
9.1.2 Transient Observations.
9.1.3 Additional Model Inputs.
9.2 Strategies for Calibrating Transport Models.
9.2.1 Selecting Processes to Include.
9.2.2 Defining Source Geometry and Concentrations.
9.2.3 Scale Issues.
9.2.4 Numerical Issues: Model Accuracy and Execution Time.
9.2.5 Transport Observations.
9.2.6 Additional Model Inputs.
9.2.7 Examples of Obtaining a Tractable, Useful Model.
9.3 Strategies for Recalibrating Existing Models.
9.4 Exercises (optional).
Exercises 9.1 and 9.2: Simulate Transient Hydraulic Heads and Perform
Preparatory Steps.
Exercise 9.3: Transient Parameter Definition.
Exercise 9.4: Observations for the Transient Problem.
Exercise 9.5: Evaluate Transient Model Fit Using Starting Parameter Values.
Exercise 9.6: Sensitivity Analysis for the Initial Model.
Exercise 9.7: Estimate Parameters for the Transient System by Nonlinear
Regression.
Exercise 9.8: Evaluate Measures of Model Fit.
Exercise 9.9: Perform Graphical Analyses of Model Fit and Evaluate Related
Statistics.
Exercise 9.10: Evaluate Estimated Parameters.
Exercise 9.11: Test for Linearity.
Exercise 9.12: Predictions.
10 Guidelines for Effective Modeling.
10.1 Purpose of the Guidelines.
10.2 Relation to Previous Work.
10.3 Suggestions for Effective Implementation.
11 Guidelines 1 Through 8-Model Development.
Guideline 1: Apply the Principle of Parsimony.
G1.1 Problem.
G1.2 Constructive Approaches.
Guideline 2: Use a Broad Range of System Information to Constrain the
Problem.
G2.1 Data Assimilation.
G2.2 Using System Information.
G2.3 Data Management.
G2.4 Application: Characterizing a Fractured Dolomite Aquifer.
Guideline 3: Maintain a Well-Posed, Comprehensive Regression Problem.
G3.1 Examples.
G3.2 Effects of Nonlinearity on the css and pcc.
Guideline 4: Include Many Kinds of Data as Observations in the Regression.
G4.1 Interpolated "Observations".
G4.2 Clustered Observations.
G4.3 Observations that Are Inconsistent with Model Construction.
G4.4 Applications: Using Different Types of Observations to Calibrate
Groundwater Flow and Transport Models.
Guideline 5: Use Prior Information Carefully.
G5.1 Use of Prior Information Compared with Observations.
G5.2 Highly Parameterized Models.
G5.3 Applications: Geophysical Data.
Guideline 6: Assign Weights that Reflect Errors.
G6.1 Determine Weights.
G6.2 Issues of Weighting in Nonlinear Regression.
Guideline 7: Encourage Convergence by Making the Model More Accurate and
Evaluating the Observations.
Guideline 8: Consider Alternative Models.
G8.1 Develop Alternative Models.
G8.2 Discriminate Between Models.
G8.3 Simulate Predictions with Alternative Models.
G8.4 Application.
12 Guidelines 9 and 10-Model Testing.
Guideline 9: Evaluate Model Fit.
G9.1 Determine Model Fit.
G9.2 Examine Fit for Existing Observations Important to the Purpose of the
Model.
G9.3 Diagnose the Cause of Poor Model Fit.
Guideline 10: Evaluate Optimized Parameter Values.
G10.1 Quantify Parameter-Value Uncertainty.
G10.2 Use Parameter Estimates to Detect Model Error.
G10.3 Diagnose the Cause of Unreasonable Optimal Parameter Estimates.
G10.4 Identify Observations Important to the Parameter Estimates.
G10.5 Reduce or Increase the Number of Parameters.
13 Guidelines 11 and 12-Potential New Data.
Guideline 11: Identify New Data to Improve Simulated Processes, Features,
and Properties.
Guideline 12: Identify New Data to Improve Predictions.
G12.1 Potential New Data to Improve Features and Properties Governing
System Dynamics.
G12.2 Potential New Data to Support Observations.
14 Guidelines 13 and 14-Prediction Uncertainty.
Guideline 13: Evaluate Prediction Uncertainty and Accuracy Using
Deterministic Methods.
G13.1 Use Regression to Determine Whether Predicted Values Are Contradicted
by the Calibrated Model.
G13.2 Use Omitted Data and Postaudits.
Guideline 14: Quantify Prediction Uncertainty Using Statistical Methods.
G14.1 Inferential Statistics.
G14.2 Monte Carlo Methods.
15 Using and Testing the Methods and Guidelines.
15.1 Execution Time Issues.
15.2 Field Applications and Synthetic Test Cases.
15.2.1 The Death Valley Regional Flow System, California and Nevada, USA.
15.2.2 Grindsted Landfill, Denmark.
Appendix A: Objective Function Issues.
A.1 Derivation of the Maximum-Likelihood Objective Function.
A.2 Relation of the Maximum-Likelihood and Least-Squares Objective
Functions.
A.3 Assumptions Required for Diagonal Weighting to be Correct.
A.4 References.
Appendix B: Calculation Details of the Modified Gauss-Newton Method.
B.1 Vectors and Matrices for Nonlinear Regression.
B.2 Quasi-Newton Updating of the Normal Equations.
B.3 Calculating the Damping Parameter.
B.4 Solving the Normal Equations.
B.5 References.
Appendix C: Two Important Properties of Linear Regression and the Effects
of Nonlinearity.
C.1 Identities Needed for the Proofs.
C.1.1 True Linear Model.
C.1.2 True Nonlinear Model.
C.1.3 Linearized True Nonlinear Model.
C.1.4 Approximate Linear Model.
C.1.5 Approximate Nonlinear Model.
C.1.6 Linearized Approximate Nonlinear Model.
C.1.7 The Importance of X and X.
C.1.8 Considering Many Observations.
C.1.9 Normal Equations.
C.1.10 Random Variables.
C.1.11 Expected Value.
C.1.12 Variance-Covariance Matrix of a Vector.
C.2 Proof of Property 1: Parameters Estimated by Linear Regression are
Unbiased.
C.3 Proof of Property 2: The Weight Matrix Needs to be Defined in a
Particular Way for Eq. (7.1) to Apply and for the Parameter Estimates to
have the Smallest Variance.
C.4 References.
Appendix D: Selected Statistical Tables.
D.1 References.
References.
Index.
"The book represents a very good combination of long-time expert knowledge and being up to date." ( Clean , January 2008)
"...a welcome addition to my collection of hydrogeologic books...a valuable reference for ground water scientists who use models." ( Ground Water , January-February 2008)