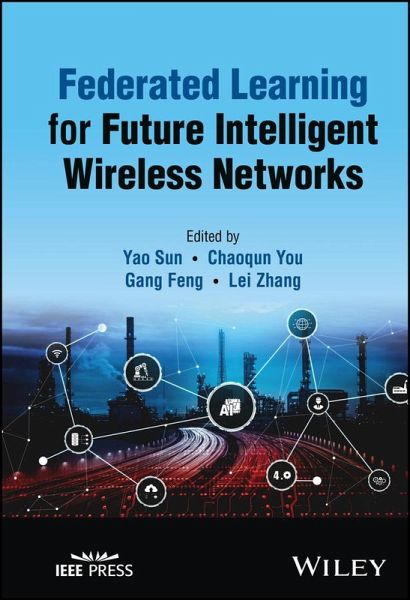
Federated Learning for Future Intelligent Wireless Networks (eBook, PDF)
Versandkostenfrei!
Sofort per Download lieferbar
111,99 €
inkl. MwSt.
Weitere Ausgaben:
PAYBACK Punkte
0 °P sammeln!
Federated Learning for Future Intelligent Wireless Networks Explore the concepts, algorithms, and applications underlying federated learning In Federated Learning for Future Intelligent Wireless Networks, a team of distinguished researchers deliver a robust and insightful collection of resources covering the foundational concepts and algorithms powering federated learning, as well as explanations of how they can be used in wireless communication systems. The editors have included works that examine how communication resource provision affects federated learning performance, accuracy, convergen...
Federated Learning for Future Intelligent Wireless Networks Explore the concepts, algorithms, and applications underlying federated learning In Federated Learning for Future Intelligent Wireless Networks, a team of distinguished researchers deliver a robust and insightful collection of resources covering the foundational concepts and algorithms powering federated learning, as well as explanations of how they can be used in wireless communication systems. The editors have included works that examine how communication resource provision affects federated learning performance, accuracy, convergence, scalability, and security and privacy. Readers will explore a wide range of topics that show how federated learning algorithms, concepts, and design and optimization issues apply to wireless communications. Readers will also find: * A thorough introduction to the fundamental concepts and algorithms of federated learning, including horizontal, vertical, and hybrid FL * Comprehensive explorations of wireless communication network design and optimization for federated learning * Practical discussions of novel federated learning algorithms and frameworks for future wireless networks * Expansive case studies in edge intelligence, autonomous driving, IoT, MEC, blockchain, and content caching and distribution Perfect for electrical and computer science engineers, researchers, professors, and postgraduate students with an interest in machine learning, Federated Learning for Future Intelligent Wireless Networks will also benefit regulators and institutional actors responsible for overseeing and making policy in the area of artificial intelligence.
Dieser Download kann aus rechtlichen Gründen nur mit Rechnungsadresse in A, B, BG, CY, CZ, D, DK, EW, E, FIN, F, GR, HR, H, IRL, I, LT, L, LR, M, NL, PL, P, R, S, SLO, SK ausgeliefert werden.