Handbook of Bayesian Variable Selection (eBook, PDF)
Redaktion: Tadesse, Mahlet G.; Vannucci, Marina
62,95 €
62,95 €
inkl. MwSt.
Sofort per Download lieferbar
31 °P sammeln
62,95 €
Als Download kaufen
62,95 €
inkl. MwSt.
Sofort per Download lieferbar
31 °P sammeln
Jetzt verschenken
Alle Infos zum eBook verschenken
62,95 €
inkl. MwSt.
Sofort per Download lieferbar
Alle Infos zum eBook verschenken
31 °P sammeln
Handbook of Bayesian Variable Selection (eBook, PDF)
Redaktion: Tadesse, Mahlet G.; Vannucci, Marina
- Format: PDF
- Merkliste
- Auf die Merkliste
- Bewerten Bewerten
- Teilen
- Produkt teilen
- Produkterinnerung
- Produkterinnerung
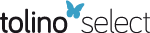
Bitte loggen Sie sich zunächst in Ihr Kundenkonto ein oder registrieren Sie sich bei
bücher.de, um das eBook-Abo tolino select nutzen zu können.
Hier können Sie sich einloggen
Hier können Sie sich einloggen
Sie sind bereits eingeloggt. Klicken Sie auf 2. tolino select Abo, um fortzufahren.
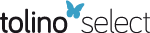
Bitte loggen Sie sich zunächst in Ihr Kundenkonto ein oder registrieren Sie sich bei bücher.de, um das eBook-Abo tolino select nutzen zu können.
The Handbook of Bayesian Variable Selection provides a comprehensive review of theoretical, methodological and computational aspects of Bayesian methods for variable selection. It also provides a valuable reference for all interested in applying existing methods and/or pursuing methodological extensions.
- Geräte: PC
- mit Kopierschutz
- eBook Hilfe
Andere Kunden interessierten sich auch für
- Handbook of Bayesian Variable Selection (eBook, ePUB)62,95 €
- Michael J. DanielsBayesian Nonparametrics for Causal Inference and Missing Data (eBook, PDF)51,95 €
- David LunnThe BUGS Book (eBook, PDF)45,95 €
- Felix AbramovichStatistical Theory (eBook, PDF)79,95 €
- Dani GamermanMarkov Chain Monte Carlo (eBook, PDF)100,95 €
- Holmes FinchMultilevel Modeling Using Mplus (eBook, PDF)55,95 €
- Joseph K. BlitzsteinIntroduction to Probability, Second Edition (eBook, PDF)71,95 €
-
-
-
The Handbook of Bayesian Variable Selection provides a comprehensive review of theoretical, methodological and computational aspects of Bayesian methods for variable selection. It also provides a valuable reference for all interested in applying existing methods and/or pursuing methodological extensions.
Dieser Download kann aus rechtlichen Gründen nur mit Rechnungsadresse in A, B, BG, CY, CZ, D, DK, EW, E, FIN, F, GR, HR, H, IRL, I, LT, L, LR, M, NL, PL, P, R, S, SLO, SK ausgeliefert werden.
Produktdetails
- Produktdetails
- Verlag: Taylor & Francis
- Seitenzahl: 490
- Erscheinungstermin: 24. Dezember 2021
- Englisch
- ISBN-13: 9781000510201
- Artikelnr.: 63096037
- Verlag: Taylor & Francis
- Seitenzahl: 490
- Erscheinungstermin: 24. Dezember 2021
- Englisch
- ISBN-13: 9781000510201
- Artikelnr.: 63096037
- Herstellerkennzeichnung Die Herstellerinformationen sind derzeit nicht verfügbar.
Mahlet Tadesse is Professor and Chair in the Department of Mathematics and Statistics at Georgetown University, USA. Her research over the past two decades has focused on Bayesian modeling for high-dimensional data with an emphasis on variable selection methods and mixture models. She also works on various interdisciplinary projects in genomics and public health. She is a recipient of the Myrto Lefkopoulou Distinguished Lectureship award, an elected member of the International Statistical Institute and an elected fellow of the American Statistical Association. Marina Vannucci is Noah Harding Professor of Statistics at Rice University, USA. Her research over the past 25 years has focused on the development of methodologies for Bayesian variable selection in linear settings, mixture models and graphical models, and on related computational algorithms. She also has a solid history of scientific collaborations and is particularly interested in applications of Bayesian inference to genomics and neuroscience. She has received an NSF CAREER award and the Mitchell prize by ISBA for her research, and the Zellner Medal by ISBA for exceptional service over an extended period of time with long-lasting impact. She is an elected Member of ISI and RSS and an elected fellow of ASA, IMS, AAAS and ISBA.
1. Discrete Spike-and-Slab Priors: Models and Computational Aspects
2. Recent Theoretical Advances with the Discrete Spike-and-Slab Priors
3. Theoretical and Computational Aspects of Continuous Spike-and-Slab Priors
4. Spike-and-Slab Meets LASSO: A Review of the Spike-and-Slab LASSO
5. Adaptive Computational Methods for Bayesian Variable Selection
6. Theoretical guarantees for the horseshoe and other global-local shrinkage priors
7. MCMC for Global-Local Shrinkage Priors in High-Dimensional Settings
8. Variable Selection with Shrinkage Priors via Sparse Posterior Summaries
9. Bayesian Model Averaging in Causal Inference
10. Variable Selection for Hierarchically-Related Outcomes: Models and Algorithms
11. Bayesian variable selection in spatial regression models
12. Effect Selection and Regularization in Structured Additive Distributional Regression
13. Sparse Bayesian State-Space and Time-Varying Parameter Models
14. Bayesian estimation of single and multiple graphs
15. Bayes Factors Based on g-Priors for Variable Selection
16. Balancing Sparsity and Power: Likelihoods, Priors, and Misspecification
17. Variable Selection and Interaction Detection with Bayesian Additive Regression Trees
18. Variable Selection for Bayesian Decision Tree Ensembles
19. Stochastic Partitioning for Variable Selection in Multivariate Mixture of Regression Models
2. Recent Theoretical Advances with the Discrete Spike-and-Slab Priors
3. Theoretical and Computational Aspects of Continuous Spike-and-Slab Priors
4. Spike-and-Slab Meets LASSO: A Review of the Spike-and-Slab LASSO
5. Adaptive Computational Methods for Bayesian Variable Selection
6. Theoretical guarantees for the horseshoe and other global-local shrinkage priors
7. MCMC for Global-Local Shrinkage Priors in High-Dimensional Settings
8. Variable Selection with Shrinkage Priors via Sparse Posterior Summaries
9. Bayesian Model Averaging in Causal Inference
10. Variable Selection for Hierarchically-Related Outcomes: Models and Algorithms
11. Bayesian variable selection in spatial regression models
12. Effect Selection and Regularization in Structured Additive Distributional Regression
13. Sparse Bayesian State-Space and Time-Varying Parameter Models
14. Bayesian estimation of single and multiple graphs
15. Bayes Factors Based on g-Priors for Variable Selection
16. Balancing Sparsity and Power: Likelihoods, Priors, and Misspecification
17. Variable Selection and Interaction Detection with Bayesian Additive Regression Trees
18. Variable Selection for Bayesian Decision Tree Ensembles
19. Stochastic Partitioning for Variable Selection in Multivariate Mixture of Regression Models
1. Discrete Spike-and-Slab Priors: Models and Computational Aspects
2. Recent Theoretical Advances with the Discrete Spike-and-Slab Priors
3. Theoretical and Computational Aspects of Continuous Spike-and-Slab Priors
4. Spike-and-Slab Meets LASSO: A Review of the Spike-and-Slab LASSO
5. Adaptive Computational Methods for Bayesian Variable Selection
6. Theoretical guarantees for the horseshoe and other global-local shrinkage priors
7. MCMC for Global-Local Shrinkage Priors in High-Dimensional Settings
8. Variable Selection with Shrinkage Priors via Sparse Posterior Summaries
9. Bayesian Model Averaging in Causal Inference
10. Variable Selection for Hierarchically-Related Outcomes: Models and Algorithms
11. Bayesian variable selection in spatial regression models
12. Effect Selection and Regularization in Structured Additive Distributional Regression
13. Sparse Bayesian State-Space and Time-Varying Parameter Models
14. Bayesian estimation of single and multiple graphs
15. Bayes Factors Based on g-Priors for Variable Selection
16. Balancing Sparsity and Power: Likelihoods, Priors, and Misspecification
17. Variable Selection and Interaction Detection with Bayesian Additive Regression Trees
18. Variable Selection for Bayesian Decision Tree Ensembles
19. Stochastic Partitioning for Variable Selection in Multivariate Mixture of Regression Models
2. Recent Theoretical Advances with the Discrete Spike-and-Slab Priors
3. Theoretical and Computational Aspects of Continuous Spike-and-Slab Priors
4. Spike-and-Slab Meets LASSO: A Review of the Spike-and-Slab LASSO
5. Adaptive Computational Methods for Bayesian Variable Selection
6. Theoretical guarantees for the horseshoe and other global-local shrinkage priors
7. MCMC for Global-Local Shrinkage Priors in High-Dimensional Settings
8. Variable Selection with Shrinkage Priors via Sparse Posterior Summaries
9. Bayesian Model Averaging in Causal Inference
10. Variable Selection for Hierarchically-Related Outcomes: Models and Algorithms
11. Bayesian variable selection in spatial regression models
12. Effect Selection and Regularization in Structured Additive Distributional Regression
13. Sparse Bayesian State-Space and Time-Varying Parameter Models
14. Bayesian estimation of single and multiple graphs
15. Bayes Factors Based on g-Priors for Variable Selection
16. Balancing Sparsity and Power: Likelihoods, Priors, and Misspecification
17. Variable Selection and Interaction Detection with Bayesian Additive Regression Trees
18. Variable Selection for Bayesian Decision Tree Ensembles
19. Stochastic Partitioning for Variable Selection in Multivariate Mixture of Regression Models