Intelligent Data Analytics for Bioinformatics and Biomedical Systems (eBook, ePUB)
Redaktion: Sharma, Neha; Chatterjee, Prasenjit; Cengiz, Korhan
Alle Infos zum eBook verschenken
Intelligent Data Analytics for Bioinformatics and Biomedical Systems (eBook, ePUB)
Redaktion: Sharma, Neha; Chatterjee, Prasenjit; Cengiz, Korhan
- Format: ePub
- Merkliste
- Auf die Merkliste
- Bewerten Bewerten
- Teilen
- Produkt teilen
- Produkterinnerung
- Produkterinnerung
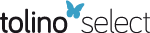
Hier können Sie sich einloggen
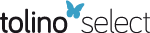
Bitte loggen Sie sich zunächst in Ihr Kundenkonto ein oder registrieren Sie sich bei bücher.de, um das eBook-Abo tolino select nutzen zu können.
The book analyzes the combination of intelligent data analytics with the intricacies of biological data that has become a crucial factor for innovation and growth in the fast-changing field of bioinformatics and biomedical systems.
Intelligent Data Analytics for Bioinformatics and Biomedical Systems delves into the transformative nature of data analytics for bioinformatics and biomedical research. It offers a thorough examination of advanced techniques, methodologies, and applications that utilize intelligence to improve results in the healthcare sector. With the exponential growth of…mehr
- Geräte: eReader
- mit Kopierschutz
- eBook Hilfe
- Größe: 3.86MB
- Christopher A. ShawNeural Dynamics of Neurological Disease (eBook, ePUB)114,99 €
- Richard L. DotyHandbook of Olfaction and Gustation (eBook, ePUB)356,99 €
- Biological Data Integration (eBook, ePUB)142,99 €
- Paola LeccaBig Data Analysis and Artificial Intelligence for Medical Sciences (eBook, ePUB)144,99 €
- Irving RetiBrain Stimulation (eBook, ePUB)132,99 €
- Munsif Ali JatoiBrain Source Localization Using EEG Signal Analysis (eBook, ePUB)49,95 €
- Introduction to Non-Invasive EEG-Based Brain-Computer Interfaces for Assistive Technologies (eBook, ePUB)48,95 €
-
-
-
Intelligent Data Analytics for Bioinformatics and Biomedical Systems delves into the transformative nature of data analytics for bioinformatics and biomedical research. It offers a thorough examination of advanced techniques, methodologies, and applications that utilize intelligence to improve results in the healthcare sector. With the exponential growth of data in these domains, the book explores how computational intelligence and advanced analytic techniques can be harnessed to extract insights, drive informed decisions, and unlock hidden patterns from vast datasets. From genomic analysis to disease diagnostics and personalized medicine, the book aims to showcase intelligent approaches that enable researchers, clinicians, and data scientists to unravel complex biological processes and make significant strides in understanding human health and diseases.
This book is divided into three sections, each focusing on computational intelligence and data sets in biomedical systems. The first section discusses the fundamental concepts of computational intelligence and big data in the context of bioinformatics. This section emphasizes data mining, pattern recognition, and knowledge discovery for bioinformatics applications. The second part talks about computational intelligence and big data in biomedical systems. Based on how these advanced techniques are utilized in the system, this section discusses how personalized medicine and precision healthcare enable treatment based on individual data and genetic profiles. The last section investigates the challenges and future directions of computational intelligence and big data in bioinformatics and biomedical systems. This section concludes with discussions on the potential impact of computational intelligence on addressing global healthcare challenges.
Audience
Intelligent Data Analytics for Bioinformatics and Biomedical Systems is primarily targeted to professionals and researchers in bioinformatics, genetics, molecular biology, biomedical engineering, and healthcare. The book will also suit academicians, students, and professionals working in pharmaceuticals and interpreting biomedical data.
Dieser Download kann aus rechtlichen Gründen nur mit Rechnungsadresse in D ausgeliefert werden.
- Produktdetails
- Verlag: John Wiley & Sons
- Seitenzahl: 600
- Erscheinungstermin: 11. Oktober 2024
- Englisch
- ISBN-13: 9781394270897
- Artikelnr.: 72246867
- Verlag: John Wiley & Sons
- Seitenzahl: 600
- Erscheinungstermin: 11. Oktober 2024
- Englisch
- ISBN-13: 9781394270897
- Artikelnr.: 72246867
- Herstellerkennzeichnung Die Herstellerinformationen sind derzeit nicht verfügbar.
Operative Care, Elderly Care, Etc. 301 12.6 Challenges of Traditional In-Person Care vs. Remote Monitoring 302 12.7 Data Collection and Transmission 303 12.7.1 Sensors and Data Collection Methods in Wearables 303 12.8 Wireless Data Transmission Technologies (Bluetooth, Wi-Fi, Cellular, Etc.) 304 12.8.1 Ensuring Data Security and Privacy 304 12.8.2 Big-Data Analytics in Healthcare 304 12.8.3 Role of Big Data in Healthcare Decision-Making 305 12.8.4 Handling and Processing Large Volumes of Wearable
Generated Data 305 12.8.5 Data Storage, Integration, and Interoperability 305 12.8.6 AI and Machine Learning in Health Monitoring 306 12.9 Introduction to AI and ML Applications in Healthcare 306 12.9.1 Predictive Analytics for Early Disease Detection 307 12.9.2 Real-Time Anomaly Detection and Alerts 307 12.9.3 Clinical Decision Support Systems 307 12.9.4 Integration of AI Insights into Clinical Workflows 308 12.9.5 Enabling Personalized Treatment Plans Based on Wearable Data 308 12.9.6 Enhancing Healthcare Professional Decision-Making 308 12.9.7 Challenges and Ethical Considerations in Using Patient
Generated Data 309 12.10 Future Directions and Trends 309 12.11 Conclusion 310 References 311 13 Disease Biomarker Discovery with Big Data Analysis 313 G. Venu Gopal, Kanakaprabha S., Gangavarapu Moahana Rao, Yallapragada Ravi Raju and G. Ganesh Kumar 13.1 Introduction 314 13.1.1 The Need for Multi-Omics Data Integration in Biomarker Discovery 314 13.1.2 Role of Machine Learning in Multi-Omics Data Analysis 314 13.2 Literature Survey 316 13.3 Challenges in Multi-Omics Data Integration 319 13.3.1 Data Heterogeneity and Integration Challenges 319 13.3.2 Dimensionality Reduction and Feature Selection 319 13.3.3 Feature Representation and Integration Techniques 319 13.3.4 Early Fusion vs. Late Fusion Approaches 320 13.3.5 Network-Based Integration Methods 320 13.4 Deep Learning Architectures for Multi-Omics Data 320 13.4.1 Disease Subtyping and Stratification 321 13.4.2 Identification of Key Regulatory Pathways 322 13.4.3 Predictive Modeling for Treatment Response 322 13.4.4 Cancer Biomarker Discovery Using Multi-Omics Data 322 13.4.5 Neurological Disorder Classification through Integration 322 13.5 Evaluation Metrics and Validation Strategies 323 13.5.1 Cross-Validation Techniques for Multi-Omics Data 324 13.5.2 Assessing Robustness and Generalizability of Biomarker Models 325 13.6 Ethical Considerations in Biomarker Discovery 325 13.6.1 Privacy and Security of Patient Data 325 13.6.2 Bias and Fairness in Machine Learning Models 326 13.6.3 Integration of Single-Cell Omics Data 326 13.6.4 Explainable AI for Biomarker Discovery 327 13.6.5 Personalized Medicine and Biomarker-Based Therapies 327 13.7 Conclusion 328 References 329 14 Real-Time Epilepsy Monitoring and Alerting System Using IoT Devices and Machine Learning Techniques in Blockchain-Based Environment 331 Mohsen Ghorbian and Saeid Ghorbian 14.1 Introduction 332 14.2 Preliminaries 334 14.2.1 Overview of IoT Technology 334 14.2.2 Blockchain Technology 335 14.2.3 Overview of ML Technology 336 14.2.4 Epilepsy Disease 337 14.3 IoT and ML in Healthcare 338 14.3.1 HLF Architectural Framework 338 14.3.2 Epilepsy Detection Procedures 341 14.3.3 Various Approaches to ml 342 14.4 Incorporating ML with IoT in the Blockchain 343 14.5 Intelligent Alert Mechanism in IoT Healthcare 345 14.5.1 Data Gathering, Transmission, and Storage 347 14.5.2 Analyzing Stored Data 348 14.5.3 Sending an Alert Message 349 14.6 Conclusion 351 References 352 15 Integrating Quantum Computing in Bioinformatics and Biomedical Research 357 Prasad Selladurai, Ruby Dahiya, Baskar Kandasamy and Venkateswaran Radhakrishnan 15.1 Introduction 358 15.1.1 Quantum Computing 360 15.1.2 The Role of Quantum Computing in Bioinformatics 361 15.1.3 Application of Quantum Technologies 363 15.1.4 Characteristics of Quantum Computing in Bioinformatics 364 15.1.5 What are the Tools Used in Quantum Computing in Bioinformatics? 366 15.2 Novel Approaches of Quantum Computing in Bioinformatics 367 15.2.1 Quantum Chemistry for Drug Discovery 367 15.2.2 A Quantum Advance in Genetics 369 15.2.3 Hybrid Quantum-Classical Approaches 370 15.2.4 Quantum-Inspired Machine Learning 372 15.2.5 Challenges and Limitations 374 15.3 Conclusion 375 15.4 The Future of Quantum Computing in Bioinformatics and Biomedical Research 376 References 378 16 Future Perspective and Emerging Trends in Computational Intelligence 381 Chander Prabha 16.1 Introduction 382 16.2 Emerging Trends in CI for Bioinformatics 384 16.3 ci Emerging Trends for Biomedical Systems 386 16.4 ci Future Perspective in Bioinformatics 388 16.5 The Future of CI in Biomedical Systems 391 16.6 Conclusion and Future Scope 393 References 394 Index 397
Operative Care, Elderly Care, Etc. 301 12.6 Challenges of Traditional In-Person Care vs. Remote Monitoring 302 12.7 Data Collection and Transmission 303 12.7.1 Sensors and Data Collection Methods in Wearables 303 12.8 Wireless Data Transmission Technologies (Bluetooth, Wi-Fi, Cellular, Etc.) 304 12.8.1 Ensuring Data Security and Privacy 304 12.8.2 Big-Data Analytics in Healthcare 304 12.8.3 Role of Big Data in Healthcare Decision-Making 305 12.8.4 Handling and Processing Large Volumes of Wearable
Generated Data 305 12.8.5 Data Storage, Integration, and Interoperability 305 12.8.6 AI and Machine Learning in Health Monitoring 306 12.9 Introduction to AI and ML Applications in Healthcare 306 12.9.1 Predictive Analytics for Early Disease Detection 307 12.9.2 Real-Time Anomaly Detection and Alerts 307 12.9.3 Clinical Decision Support Systems 307 12.9.4 Integration of AI Insights into Clinical Workflows 308 12.9.5 Enabling Personalized Treatment Plans Based on Wearable Data 308 12.9.6 Enhancing Healthcare Professional Decision-Making 308 12.9.7 Challenges and Ethical Considerations in Using Patient
Generated Data 309 12.10 Future Directions and Trends 309 12.11 Conclusion 310 References 311 13 Disease Biomarker Discovery with Big Data Analysis 313 G. Venu Gopal, Kanakaprabha S., Gangavarapu Moahana Rao, Yallapragada Ravi Raju and G. Ganesh Kumar 13.1 Introduction 314 13.1.1 The Need for Multi-Omics Data Integration in Biomarker Discovery 314 13.1.2 Role of Machine Learning in Multi-Omics Data Analysis 314 13.2 Literature Survey 316 13.3 Challenges in Multi-Omics Data Integration 319 13.3.1 Data Heterogeneity and Integration Challenges 319 13.3.2 Dimensionality Reduction and Feature Selection 319 13.3.3 Feature Representation and Integration Techniques 319 13.3.4 Early Fusion vs. Late Fusion Approaches 320 13.3.5 Network-Based Integration Methods 320 13.4 Deep Learning Architectures for Multi-Omics Data 320 13.4.1 Disease Subtyping and Stratification 321 13.4.2 Identification of Key Regulatory Pathways 322 13.4.3 Predictive Modeling for Treatment Response 322 13.4.4 Cancer Biomarker Discovery Using Multi-Omics Data 322 13.4.5 Neurological Disorder Classification through Integration 322 13.5 Evaluation Metrics and Validation Strategies 323 13.5.1 Cross-Validation Techniques for Multi-Omics Data 324 13.5.2 Assessing Robustness and Generalizability of Biomarker Models 325 13.6 Ethical Considerations in Biomarker Discovery 325 13.6.1 Privacy and Security of Patient Data 325 13.6.2 Bias and Fairness in Machine Learning Models 326 13.6.3 Integration of Single-Cell Omics Data 326 13.6.4 Explainable AI for Biomarker Discovery 327 13.6.5 Personalized Medicine and Biomarker-Based Therapies 327 13.7 Conclusion 328 References 329 14 Real-Time Epilepsy Monitoring and Alerting System Using IoT Devices and Machine Learning Techniques in Blockchain-Based Environment 331 Mohsen Ghorbian and Saeid Ghorbian 14.1 Introduction 332 14.2 Preliminaries 334 14.2.1 Overview of IoT Technology 334 14.2.2 Blockchain Technology 335 14.2.3 Overview of ML Technology 336 14.2.4 Epilepsy Disease 337 14.3 IoT and ML in Healthcare 338 14.3.1 HLF Architectural Framework 338 14.3.2 Epilepsy Detection Procedures 341 14.3.3 Various Approaches to ml 342 14.4 Incorporating ML with IoT in the Blockchain 343 14.5 Intelligent Alert Mechanism in IoT Healthcare 345 14.5.1 Data Gathering, Transmission, and Storage 347 14.5.2 Analyzing Stored Data 348 14.5.3 Sending an Alert Message 349 14.6 Conclusion 351 References 352 15 Integrating Quantum Computing in Bioinformatics and Biomedical Research 357 Prasad Selladurai, Ruby Dahiya, Baskar Kandasamy and Venkateswaran Radhakrishnan 15.1 Introduction 358 15.1.1 Quantum Computing 360 15.1.2 The Role of Quantum Computing in Bioinformatics 361 15.1.3 Application of Quantum Technologies 363 15.1.4 Characteristics of Quantum Computing in Bioinformatics 364 15.1.5 What are the Tools Used in Quantum Computing in Bioinformatics? 366 15.2 Novel Approaches of Quantum Computing in Bioinformatics 367 15.2.1 Quantum Chemistry for Drug Discovery 367 15.2.2 A Quantum Advance in Genetics 369 15.2.3 Hybrid Quantum-Classical Approaches 370 15.2.4 Quantum-Inspired Machine Learning 372 15.2.5 Challenges and Limitations 374 15.3 Conclusion 375 15.4 The Future of Quantum Computing in Bioinformatics and Biomedical Research 376 References 378 16 Future Perspective and Emerging Trends in Computational Intelligence 381 Chander Prabha 16.1 Introduction 382 16.2 Emerging Trends in CI for Bioinformatics 384 16.3 ci Emerging Trends for Biomedical Systems 386 16.4 ci Future Perspective in Bioinformatics 388 16.5 The Future of CI in Biomedical Systems 391 16.6 Conclusion and Future Scope 393 References 394 Index 397