Intelligent Data-Driven Modelling and Optimization in Power and Energy Applications (eBook, PDF)
Redaktion: Prusty, B Rajanarayan; Sehgal, Rakesh; Bingi, Kishore; Gupta, Neeraj
68,95 €
68,95 €
inkl. MwSt.
Sofort per Download lieferbar
34 °P sammeln
68,95 €
Als Download kaufen
68,95 €
inkl. MwSt.
Sofort per Download lieferbar
34 °P sammeln
Jetzt verschenken
Alle Infos zum eBook verschenken
68,95 €
inkl. MwSt.
Sofort per Download lieferbar
Alle Infos zum eBook verschenken
34 °P sammeln
Intelligent Data-Driven Modelling and Optimization in Power and Energy Applications (eBook, PDF)
Redaktion: Prusty, B Rajanarayan; Sehgal, Rakesh; Bingi, Kishore; Gupta, Neeraj
- Format: PDF
- Merkliste
- Auf die Merkliste
- Bewerten Bewerten
- Teilen
- Produkt teilen
- Produkterinnerung
- Produkterinnerung
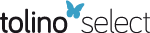
Bitte loggen Sie sich zunächst in Ihr Kundenkonto ein oder registrieren Sie sich bei
bücher.de, um das eBook-Abo tolino select nutzen zu können.
Hier können Sie sich einloggen
Hier können Sie sich einloggen
Sie sind bereits eingeloggt. Klicken Sie auf 2. tolino select Abo, um fortzufahren.
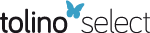
Bitte loggen Sie sich zunächst in Ihr Kundenkonto ein oder registrieren Sie sich bei bücher.de, um das eBook-Abo tolino select nutzen zu können.
This book provides a comprehensive understanding of how intelligent data-driven techniques can be used for modelling, controlling, and optimizing various power and energy applications.
- Geräte: PC
- mit Kopierschutz
- eBook Hilfe
Andere Kunden interessierten sich auch für
- Intelligent Data-Driven Modelling and Optimization in Power and Energy Applications (eBook, ePUB)68,95 €
- Optimization and Computing using Intelligent Data-Driven Approaches for Decision-Making (eBook, PDF)52,95 €
- Microgrid (eBook, PDF)52,95 €
- Data-Driven Systems and Intelligent Applications (eBook, PDF)52,95 €
- Optimization and Computing using Intelligent Data-Driven Approaches for Decision-Making (eBook, PDF)54,95 €
- Applications of Mathematical Modeling, Machine Learning, and Intelligent Computing for Industrial Development (eBook, PDF)59,95 €
- Artificial Intelligence based Solutions for Industrial Applications (eBook, PDF)52,95 €
-
-
-
This book provides a comprehensive understanding of how intelligent data-driven techniques can be used for modelling, controlling, and optimizing various power and energy applications.
Dieser Download kann aus rechtlichen Gründen nur mit Rechnungsadresse in A, B, BG, CY, CZ, D, DK, EW, E, FIN, F, GR, HR, H, IRL, I, LT, L, LR, M, NL, PL, P, R, S, SLO, SK ausgeliefert werden.
Produktdetails
- Produktdetails
- Verlag: Taylor & Francis eBooks
- Seitenzahl: 252
- Erscheinungstermin: 9. Mai 2024
- Englisch
- ISBN-13: 9781040016114
- Artikelnr.: 70184656
- Verlag: Taylor & Francis eBooks
- Seitenzahl: 252
- Erscheinungstermin: 9. Mai 2024
- Englisch
- ISBN-13: 9781040016114
- Artikelnr.: 70184656
- Herstellerkennzeichnung Die Herstellerinformationen sind derzeit nicht verfügbar.
B Rajanarayan Prusty (Senior Member, IEEE) is a Professor and Associate Dean Research in the School of Engineering, Galgotias University, Greater Noida, India. He obtained his Ph.D. from the National Institute of Technology Karnataka, Surathkal. His exceptional research work during his Ph.D. has led him to win the prestigious POSOCO Power System Awards for 2019 by Power System Operation Corporation Limited in partnership with IIT Delhi. In recognition of his publications from 2017 to 2019, he was awarded the University Foundation Day Research Award 2019 from BPUT, Rourkela, Odisha. He has 30 SCI journal publications and 50 international conference publications. He has authored 10 book chapters. He has co-authored a textbook entitled Power System Analysis: Operation and Control in I. K. International Publishing House Pvt. Ltd. He has also edited two books for CRC Press. He has been an active reviewer and has reviewed more than 500 manuscripts. He is the Associate Editor of the Journal of Electrical Engineering & Technology and the International Journal of Power and Energy Systems. He is also the Academic Editor for the journals (i) Mathematical Problems in Engineering, (ii) International Transactions on Electrical Energy Systems, and (iii) Journal of Electrical and Computer Engineering. He has handled more than 200 manuscripts in the capacity of Journal Editor. His research interests include data preprocessing, time series forecasting, high-dimensional dependence modelling, and applying machine learning and probabilistic methods to power system problems. Neeraj Gupta obtained his Ph.D. in power systems from the Indian Institute of Technology Roorkee, Roorkee, India. He is a senior member of IEEE. He was a faculty with Thapar University, from 2008 to 2009, Adani Institute of Infrastructure Engineering, Ahmedabad, India, in 2015 and NIT Hamirpur from 2015 to 2018, and presently, he has been working as Assistant Professor with the Electrical Engineering Department, National Institute of Technology, Srinagar, J&K, India. His work has been published in Q-1 international journals of repute like IEEE, Elsevier, etc. He is presently guiding four Ph.D. scholars in the area of power systems. He has also supervised eight M.Tech. and four B.Tech. dissertations. He has more than 40 SCI journal publications/conference publications/book chapters to his credit. He has edited three books titled Control of Standalone Microgrid (Elsevier 2021), Renewable Energy Integration to the Grid: A Probabilistic Perspective (CRC Press 2022), and Smart Electrical and Mechanical Systems: An Application Publisher (Elsevier 2022). He has been an active reviewer since 2015 and has reviewed 200 manuscripts submitted to repute SCI-indexed journals/conferences. He has delivered 15 invited expert talks in various organizations in India. He is also the scientific advisory/organizing secretary of many reputed conferences in the country. He is a referee of reputed journals of IEEE, Elsevier, Taylor and Francis, IET, and so on. He has been included in the list of top 2% highly cited scientists by Stanford University working in power in 2021. His research interests include the uncertainty quantification of power system; probabilistic power system; solar, wind, and electric vehicle technologies; artificial intelligence; machine learning; prediction; and so on. Kishore Bingi received his B.Tech. degree in Electrical and Electronics Engineering from Acharya Nagarjuna University, Guntur, Andhra Pradesh, India, in 2012. He received his M.Tech. degree in Instrumentation and Control Systems from the National Institute of Technology Calicut, India, in 2014, and a Ph.D. in Electrical and Electronic Engineering from Universiti Teknologi PETRONAS, Malaysia, in 2019. From 2014 to 2015, he worked as Assistant Systems Engineer at TATA Consultancy Services Limited, India. From 2019 to 2020, he worked as Research Scientist and Post-Doctoral Researcher at the Universiti Teknologi PETRONAS, Malaysia. From 2020 to 2022, he served as Assistant Professor at the Process Control Laboratory, School of Electrical Engineering, Vellore Institute of Technology, Vellore, India. Since 2022, he has been working as a faculty member at the Department of Electrical and Electronic Engineering at Universiti Teknologi PETRONAS, Seri Iskandar, Perak, Malaysia. His research area is developing fractional-order neural networks, including fractional-order systems and controllers, chaos prediction and forecasting, and advanced hybrid optimization techniques. He is an IEEE and IET Member and a registered Chartered Engineer (CEng) from the Engineering Council, UK. Rakesh Sehgal is currently working as Professor (HAG) at the National Institute of Technology, Hamirpur (H.P.), after serving as Director of the National Institute of Technology for more than five years. Prof. Sehgal received his B.E. degree in Mechanical Engineering with distinction from the Faculty of Engineering & Technology, Annamalai University (T.N.), M.Tech. in Design of Mechanical Equipment from IIT Delhi with 9.75 CGPA securing the first position in Design stream and Ph.D. in Tribology from R.E.C. Kurukshetra, Kurukshetra University. He pursued Post-Doctorate in the area of thermal behaviour of non-circular hydrodynamic journal bearings under the UGC Fellowship Award between 2009 and 2011 and developed film thickness equations for elliptical and off-set halves hydrodynamic journal bearings. Prof. Sehgal has a distinguished career of 38 years in the field, teaching, research, and administration. Prof. Sehgal has supervised 11 Ph.D. scholars and 1 post-doctoral scholar in the area of tribo-materials, active vibration control, and thermal analysis of non-circular journal bearings for various industrial applications in automobile, aerospace, and metal rolling sectors. He is presently guiding seven Ph.D. scholars in the area of material's tribology. He has published 178 research papers in international/national journals and international/national conference proceedings, 6 reference books, 3 patents, and 22 high-quality book chapters. Prof. Sehgal has completed 6 high-value research projects (5 national and 1 international) and is currently handling 3 (1 national and 2 international) projects. He has attended more than 35 international/national conferences in India and abroad.
1. Preprocessing Approaches for Data-Driven Modeling. 2. Power System Planning Using Data-Driven Models. 3. Data-Driven Analytics for Power System Stability Assessment. 4. Data-Driven Machine Learning Models for Load Power Forecasting in Photovoltaic systems. 5. Forecasting of Renewable Energy Using Fractional-Order Neural Networks. 6. Data-Driven Photovoltaic System Characteristic Determination using Nonlinear System Identification. 7. Fractional Feedforward Neural Network-Based Smart Grid Stability Prediction Model. 8. Data-driven Optimization Framework for Microgrid Energy Management Considering Demand Response and Generation Uncertainties. 9. Optimization of Controllers for Sustained Building. 10. Intelligent Data-Driven Approach for Fractional-Order Wireless Power Transfer System
1. Preprocessing Approaches for Data-Driven Modeling. 2. Power System Planning Using Data-Driven Models. 3. Data-Driven Analytics for Power System Stability Assessment. 4. Data-Driven Machine Learning Models for Load Power Forecasting in Photovoltaic systems. 5. Forecasting of Renewable Energy Using Fractional-Order Neural Networks. 6. Data-Driven Photovoltaic System Characteristic Determination using Nonlinear System Identification. 7. Fractional Feedforward Neural Network-Based Smart Grid Stability Prediction Model. 8. Data-driven Optimization Framework for Microgrid Energy Management Considering Demand Response and Generation Uncertainties. 9. Optimization of Controllers for Sustained Building. 10. Intelligent Data-Driven Approach for Fractional-Order Wireless Power Transfer System