Interpolation and Extrapolation Optimal Designs 2 (eBook, PDF)
Finite Dimensional General Models
Alle Infos zum eBook verschenken
Interpolation and Extrapolation Optimal Designs 2 (eBook, PDF)
Finite Dimensional General Models
- Format: PDF
- Merkliste
- Auf die Merkliste
- Bewerten Bewerten
- Teilen
- Produkt teilen
- Produkterinnerung
- Produkterinnerung
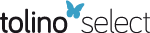
Hier können Sie sich einloggen
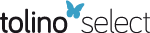
Bitte loggen Sie sich zunächst in Ihr Kundenkonto ein oder registrieren Sie sich bei bücher.de, um das eBook-Abo tolino select nutzen zu können.
This book considers various extensions of the topics treated in the first volume of this series, in relation to the class of models and the type of criterion for optimality. The regressors are supposed to belong to a generic finite dimensional Haar linear space, which substitutes for the classical polynomial case. The estimation pertains to a general linear form of the coefficients of the model, extending the interpolation and extrapolation framework; the errors in the model may be correlated, and the model may be heteroscedastic. Non-linear models, as well as multivariate ones, are briefly…mehr
- Geräte: PC
- mit Kopierschutz
- eBook Hilfe
- Größe: 3.24MB
- Giorgio CelantInterpolation and Extrapolation Optimal Designs V1 (eBook, PDF)139,99 €
- Kirti R. ShahTheory of Optimal Designs (eBook, PDF)40,95 €
- Marine Corlosquet-HabartAsset and Liability Management for Banks and Insurance Companies (eBook, PDF)136,99 €
- Zahari ZlatevRichardson Extrapolation (eBook, PDF)115,95 €
- J. A. JohnCyclic Designs (eBook, PDF)40,95 €
- Eric TysonTaxes For Dummies (eBook, PDF)16,99 €
- Mary Jane SterlingTrigonometry For Dummies (eBook, PDF)16,99 €
-
-
-
Dieser Download kann aus rechtlichen Gründen nur mit Rechnungsadresse in A, B, BG, CY, CZ, D, DK, EW, E, FIN, F, GR, HR, H, IRL, I, LT, L, LR, M, NL, PL, P, R, S, SLO, SK ausgeliefert werden.
Hinweis: Dieser Artikel kann nur an eine deutsche Lieferadresse ausgeliefert werden.
- Produktdetails
- Verlag: Wiley-Blackwell
- Seitenzahl: 320
- Erscheinungstermin: 11. April 2017
- Englisch
- ISBN-13: 9781119422365
- Artikelnr.: 52557699
- Verlag: Wiley-Blackwell
- Seitenzahl: 320
- Erscheinungstermin: 11. April 2017
- Englisch
- ISBN-13: 9781119422365
- Artikelnr.: 52557699
- Herstellerkennzeichnung Die Herstellerinformationen sind derzeit nicht verfügbar.
Introduction xi
Chapter 1 Approximation of Continuous Functions in Normed Spaces 1
1.1. Introduction 1
1.2. Some remarks on the meaning of the word "simple". Choosing the
approximation 2
1.2.1. Splines 6
1.3. The choice of the norm in order to specify the error 8
1.4. Optimality with respect to a norm 12
1.4.1. Existence of an optimal solution 12
1.4.2. Uniqueness of the optimal solution 13
1.4.3. Examples 15
1.5. Characterizing the optimal solution 18
1.5.1. The Hilbertian case 18
1.5.2. The non-Hilbertian case 21
1.5.3. Optimization, Lp norms and robustness 24
Chapter 2 Chebyshev Systems 27
2.1. Introduction 27
2.2. From the classical polynomials to the generalized ones 28
2.2.1. Examples of Chebyshev systems 32
2.3. Properties of a Chebyshev system 34
2.3.1. Vector-type properties 34
2.3.2. Chebyshev systems and interpolation 40
2.3.3. Roots of the generalized polynomials 44
Chapter 3 Uniform Approximations in a Normed Space 45
3.1. Introduction 45
3.2. Characterization of the best uniform approximation in a normed space
46
3.2.1. The Haar-Kolmogorov theorem 46
3.2.2. The generalized Borel - Chebyshev theorem 58
3.2.3. Oscillation properties of the best uniform approximation 64
Chapter 4 Calculation of the Best Uniform Approximation in a Chebyshev
System 69
4.1. Some preliminary results 69
4.2. Functional continuity of the approximation scheme 71
4.3 Property of the uniform approximation on a finite collection of points
in [a, b] 74
4.4. Algorithm of de la Vallée Poussin 80
4.5. Algorithm of Remez 80
Chapter 5 Optimal Extrapolation Design for the Chebyshev Regression 85
5.1. Introduction 85
5.2. The model and Gauss-Markov estimator 87
5.2.1. Description of the dataset 88
5.3. An expression of the extrapolated value through an orthogonalization
procedure 91
5.4. The Gauss-Markov estimator of the extrapolated value 93
5.5. The Optimal extrapolation design for the Chebyshev regression 97
5.5.1. The support of the optimal design 97
5.5.2. The frequencies of the optimal design 102
5.5.3. Identification of the optimal design 104
Chapter 6 Optimal Design for Linear Forms of the Parameters in a Chebyshev
Regression 107
6.1. Outlook and notations 107
6.2. Matrix of moments 113
6.3. Estimable forms 118
6.4. Matrix of moments and Gauss-Markov estimators of a linear form 119
6.4.1. Matrices of moments and estimable linear forms 119
6.4.2. An alternative form of the lower bound of the variance of the
estimator of the c form 129
6.5. Geometric interpretation of estimability: Elfving set 133
6.5.1. Estimable forms and a convex subset of the regression range; the
Elfving set 133
6.5.2. Geometry of the Elfving set 137
6.5.3. The relation between cylinders and the variance of the estimator of
the c-form 142
6.5.4. Lower bound for the variance 143
6.5.5. The lower bound can be achieved 145
6.6. Elfving theorem 148
6.7. An intuitive approach to Elfving theorem 154
6.8. Extension of Hoel-Levine result: optimal design for a linear c-form
160
Chapter 7 Special Topics and Extensions 169
7.1. Introduction 169
7.2. The Gauss-Markov theorem in various contexts 170
7.2.1. The Gauss-Markov theorem for linear transformations of the parameter
under i.i.d. errors 170
7.2.2. Gauss-Markov theorem for heteroscedastic models with correlation 174
7.2.3. The Gauss-Markov theorem and the Loewner order on quadratic forms
176
7.3. Criterions for optimal designs 178
7.3.1. Introduction 178
7.3.2. Some specific criterions 181
7.4. G-optimal interpolation and extrapolation designs for the Chebyshev
regression 188
7.4.1. Criteria for optimality 188
7.4.2. Design with minimal uniform variance for a Chebyshev regression 203
7.5. Some questions pertaining to the model 209
7.5.1. Linear heteroscedastic models 209
7.5.2. Nonlinear models, estimators and optimal designs 216
7.6. Hypotheses pertaining to the regressor 225
7.6.1. Regressor in a linear space with unknown finite dimension 225
7.6.2. An extension to the case of analytic regressors 227
7.6.3. On the choice of the degree of the polynomial model 229
7.7. A few questions pertaining to the support of the optimal design for
extrapolation 229
7.7.1. Preliminary results and notation 230
7.7.2. Optimal designs whose support is the Chebyshev set of points 235
7.8. The proofs of some technical results 239
7.8.1. Proof of proposition 7.1 239
7.8.2. Proof of theorem 7.17 241
Chapter 8 Multivariate Models and Algorithms 249
8.1. Introduction 249
8.2. Multivariate models 250
8.2.1. Notation 250
8.2.2. Predictors and their variance 254
8.2.3. Some construction of multivariate models 255
8.3. Optimality criterions and some optimal designs 257
8.3.1. Criterions for optimality and characterization of the optimal design
257
8.3.2. D¿optimality, direct sum and tensor product 262
8.4. Algorithms 266
8.4.1. General aspects 266
8.4.2. Specific algorithms 271
Bibliography 289
Index 295
Introduction xi
Chapter 1 Approximation of Continuous Functions in Normed Spaces 1
1.1. Introduction 1
1.2. Some remarks on the meaning of the word "simple". Choosing the
approximation 2
1.2.1. Splines 6
1.3. The choice of the norm in order to specify the error 8
1.4. Optimality with respect to a norm 12
1.4.1. Existence of an optimal solution 12
1.4.2. Uniqueness of the optimal solution 13
1.4.3. Examples 15
1.5. Characterizing the optimal solution 18
1.5.1. The Hilbertian case 18
1.5.2. The non-Hilbertian case 21
1.5.3. Optimization, Lp norms and robustness 24
Chapter 2 Chebyshev Systems 27
2.1. Introduction 27
2.2. From the classical polynomials to the generalized ones 28
2.2.1. Examples of Chebyshev systems 32
2.3. Properties of a Chebyshev system 34
2.3.1. Vector-type properties 34
2.3.2. Chebyshev systems and interpolation 40
2.3.3. Roots of the generalized polynomials 44
Chapter 3 Uniform Approximations in a Normed Space 45
3.1. Introduction 45
3.2. Characterization of the best uniform approximation in a normed space
46
3.2.1. The Haar-Kolmogorov theorem 46
3.2.2. The generalized Borel - Chebyshev theorem 58
3.2.3. Oscillation properties of the best uniform approximation 64
Chapter 4 Calculation of the Best Uniform Approximation in a Chebyshev
System 69
4.1. Some preliminary results 69
4.2. Functional continuity of the approximation scheme 71
4.3 Property of the uniform approximation on a finite collection of points
in [a, b] 74
4.4. Algorithm of de la Vallée Poussin 80
4.5. Algorithm of Remez 80
Chapter 5 Optimal Extrapolation Design for the Chebyshev Regression 85
5.1. Introduction 85
5.2. The model and Gauss-Markov estimator 87
5.2.1. Description of the dataset 88
5.3. An expression of the extrapolated value through an orthogonalization
procedure 91
5.4. The Gauss-Markov estimator of the extrapolated value 93
5.5. The Optimal extrapolation design for the Chebyshev regression 97
5.5.1. The support of the optimal design 97
5.5.2. The frequencies of the optimal design 102
5.5.3. Identification of the optimal design 104
Chapter 6 Optimal Design for Linear Forms of the Parameters in a Chebyshev
Regression 107
6.1. Outlook and notations 107
6.2. Matrix of moments 113
6.3. Estimable forms 118
6.4. Matrix of moments and Gauss-Markov estimators of a linear form 119
6.4.1. Matrices of moments and estimable linear forms 119
6.4.2. An alternative form of the lower bound of the variance of the
estimator of the c form 129
6.5. Geometric interpretation of estimability: Elfving set 133
6.5.1. Estimable forms and a convex subset of the regression range; the
Elfving set 133
6.5.2. Geometry of the Elfving set 137
6.5.3. The relation between cylinders and the variance of the estimator of
the c-form 142
6.5.4. Lower bound for the variance 143
6.5.5. The lower bound can be achieved 145
6.6. Elfving theorem 148
6.7. An intuitive approach to Elfving theorem 154
6.8. Extension of Hoel-Levine result: optimal design for a linear c-form
160
Chapter 7 Special Topics and Extensions 169
7.1. Introduction 169
7.2. The Gauss-Markov theorem in various contexts 170
7.2.1. The Gauss-Markov theorem for linear transformations of the parameter
under i.i.d. errors 170
7.2.2. Gauss-Markov theorem for heteroscedastic models with correlation 174
7.2.3. The Gauss-Markov theorem and the Loewner order on quadratic forms
176
7.3. Criterions for optimal designs 178
7.3.1. Introduction 178
7.3.2. Some specific criterions 181
7.4. G-optimal interpolation and extrapolation designs for the Chebyshev
regression 188
7.4.1. Criteria for optimality 188
7.4.2. Design with minimal uniform variance for a Chebyshev regression 203
7.5. Some questions pertaining to the model 209
7.5.1. Linear heteroscedastic models 209
7.5.2. Nonlinear models, estimators and optimal designs 216
7.6. Hypotheses pertaining to the regressor 225
7.6.1. Regressor in a linear space with unknown finite dimension 225
7.6.2. An extension to the case of analytic regressors 227
7.6.3. On the choice of the degree of the polynomial model 229
7.7. A few questions pertaining to the support of the optimal design for
extrapolation 229
7.7.1. Preliminary results and notation 230
7.7.2. Optimal designs whose support is the Chebyshev set of points 235
7.8. The proofs of some technical results 239
7.8.1. Proof of proposition 7.1 239
7.8.2. Proof of theorem 7.17 241
Chapter 8 Multivariate Models and Algorithms 249
8.1. Introduction 249
8.2. Multivariate models 250
8.2.1. Notation 250
8.2.2. Predictors and their variance 254
8.2.3. Some construction of multivariate models 255
8.3. Optimality criterions and some optimal designs 257
8.3.1. Criterions for optimality and characterization of the optimal design
257
8.3.2. D¿optimality, direct sum and tensor product 262
8.4. Algorithms 266
8.4.1. General aspects 266
8.4.2. Specific algorithms 271
Bibliography 289
Index 295