Gerhard X. Ritter, Gonzalo Urcid
Introduction to Lattice Algebra (eBook, ePUB)
With Applications in AI, Pattern Recognition, Image Analysis, and Biomimetic Neural Networks
49,95 €
49,95 €
inkl. MwSt.
Sofort per Download lieferbar
25 °P sammeln
49,95 €
Als Download kaufen
49,95 €
inkl. MwSt.
Sofort per Download lieferbar
25 °P sammeln
Jetzt verschenken
Alle Infos zum eBook verschenken
49,95 €
inkl. MwSt.
Sofort per Download lieferbar
Alle Infos zum eBook verschenken
25 °P sammeln
Gerhard X. Ritter, Gonzalo Urcid
Introduction to Lattice Algebra (eBook, ePUB)
With Applications in AI, Pattern Recognition, Image Analysis, and Biomimetic Neural Networks
- Format: ePub
- Merkliste
- Auf die Merkliste
- Bewerten Bewerten
- Teilen
- Produkt teilen
- Produkterinnerung
- Produkterinnerung
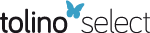
Bitte loggen Sie sich zunächst in Ihr Kundenkonto ein oder registrieren Sie sich bei
bücher.de, um das eBook-Abo tolino select nutzen zu können.
Hier können Sie sich einloggen
Hier können Sie sich einloggen
Sie sind bereits eingeloggt. Klicken Sie auf 2. tolino select Abo, um fortzufahren.
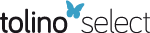
Bitte loggen Sie sich zunächst in Ihr Kundenkonto ein oder registrieren Sie sich bei bücher.de, um das eBook-Abo tolino select nutzen zu können.
This book lays emphasis on two subjects, the first being lattice algebra and the second the practical applications of that algebra. This textbook is intended to be used for a special topics course in arti¿cial intelligence with focus on pattern recognition, multispectral image analysis, and biomimetic arti¿cial neural networks.
- Geräte: eReader
- mit Kopierschutz
- eBook Hilfe
Andere Kunden interessierten sich auch für
- Gerhard X. RitterIntroduction to Lattice Algebra (eBook, PDF)49,95 €
- Steven J. RosenbergAn Invitation to Abstract Algebra (eBook, ePUB)59,95 €
- Jonathan D. H. SmithIntroduction to Abstract Algebra (eBook, ePUB)101,95 €
- Advances in Algebra Analysis and Topology (eBook, ePUB)52,95 €
- Kenier CastilloA First Course on Orthogonal Polynomials (eBook, ePUB)52,95 €
- Benjamin FineIntroduction to Abstract Algebra (eBook, ePUB)55,95 €
- W. Keith NicholsonIntroduction to Abstract Algebra (eBook, ePUB)124,99 €
-
-
-
This book lays emphasis on two subjects, the first being lattice algebra and the second the practical applications of that algebra. This textbook is intended to be used for a special topics course in arti¿cial intelligence with focus on pattern recognition, multispectral image analysis, and biomimetic arti¿cial neural networks.
Dieser Download kann aus rechtlichen Gründen nur mit Rechnungsadresse in A, B, BG, CY, CZ, D, DK, EW, E, FIN, F, GR, HR, H, IRL, I, LT, L, LR, M, NL, PL, P, R, S, SLO, SK ausgeliefert werden.
Produktdetails
- Produktdetails
- Verlag: Taylor & Francis eBooks
- Seitenzahl: 432
- Erscheinungstermin: 23. August 2021
- Englisch
- ISBN-13: 9781000412604
- Artikelnr.: 62380043
- Verlag: Taylor & Francis eBooks
- Seitenzahl: 432
- Erscheinungstermin: 23. August 2021
- Englisch
- ISBN-13: 9781000412604
- Artikelnr.: 62380043
- Herstellerkennzeichnung Die Herstellerinformationen sind derzeit nicht verfügbar.
Gerhard X. Ritter received both his B.A. degree with honors in 1966 and his Ph.D. degree in Mathematics in 1971 from the University of Wisconsin-Madison. He is a Florida Blue Key Distinguished Professor Emeritus in both the Department of Mathematics and the Department of Computer and Information Science and Engineering (CISE) of the University of Florida. He was the Chair of the CISE department from 1994 to 2001, and Acting Chair from 2011 to 2012.
Professor Ritter has written more than 140 research papers in subjects ranging from pure and applied mathematics to pattern recognition, computer vision, and artificial neural networks. He is the founding editor of the Journal of Mathematical Imaging and Vision, and founding member and first chair of the Society for Industrial and Applied Mathematics (SIAM) Activity Group on Imaging Science (SIAG-IS). He was a member of the Deputy Undersecretary of Defense for Research and Advanced Technology's advanced technology research on emerging technologies panel (1988) and a member of the advanced sensors committee on key technologies for the 1990s, formed by the same undersecretary (1989). Among other U.S. government-requested briefings attended by Professor Ritter were the annual Automatic Target Recognition Working Group (ATRWG) meetings held across the U.S. (1984-1996 and 2003). For his contribution, he was awarded the General Ronald W. Yates Award for Excellence in Technology Transfer by the U.S. Air Force Research Laboratory (1998). Among honors outside the realm of the Department of Defense are the Silver Core Award of the International Federation for Information Processing (1989); the Best Session Award at the American Society for Engineering Education (ASEE) Conference for Industry and Education Collaboration in San Jose, CA (1996); and the Best Paper Presentation Award at the International Joint Conference on Neural Networks (IJCNN) sponsored by the Institute of Electrical and Electronics Engineers Neural Networks Council (IEEE/NNC) and the International Neural Network Society (INNS) in Washington, DC (1999).
Gonzalo Urcid received his Bachelor degree in Communications and Electronic Engineering (1982) and his Master degree in Computational and Information Systems (1985) both from the University of the Americas in Puebla (UDLAP), Mexico. He has a Ph.D. degree (1999) in Optical Sciences from the National Institute of Astrophysics, Optics, and Electronics (INAOE) in Tonantzintla, Mexico and made a postdoctoral residence, between 2001 and 2002, as invited faculty at the CISE Department, University of Florida. Also, from 2001 to 2020 was awarded the distinction of National Researcher from the Mexican National Council of Science and Technology (SNI-CONACYT). Currently is an Associate Professor in the Optics Department at INAOE. His research interests include digital image processing and analysis, artificial neural networks based on lattice algebra, and lattice computing applied to artificial intelligence and pattern recognition.
Professor Ritter has written more than 140 research papers in subjects ranging from pure and applied mathematics to pattern recognition, computer vision, and artificial neural networks. He is the founding editor of the Journal of Mathematical Imaging and Vision, and founding member and first chair of the Society for Industrial and Applied Mathematics (SIAM) Activity Group on Imaging Science (SIAG-IS). He was a member of the Deputy Undersecretary of Defense for Research and Advanced Technology's advanced technology research on emerging technologies panel (1988) and a member of the advanced sensors committee on key technologies for the 1990s, formed by the same undersecretary (1989). Among other U.S. government-requested briefings attended by Professor Ritter were the annual Automatic Target Recognition Working Group (ATRWG) meetings held across the U.S. (1984-1996 and 2003). For his contribution, he was awarded the General Ronald W. Yates Award for Excellence in Technology Transfer by the U.S. Air Force Research Laboratory (1998). Among honors outside the realm of the Department of Defense are the Silver Core Award of the International Federation for Information Processing (1989); the Best Session Award at the American Society for Engineering Education (ASEE) Conference for Industry and Education Collaboration in San Jose, CA (1996); and the Best Paper Presentation Award at the International Joint Conference on Neural Networks (IJCNN) sponsored by the Institute of Electrical and Electronics Engineers Neural Networks Council (IEEE/NNC) and the International Neural Network Society (INNS) in Washington, DC (1999).
Gonzalo Urcid received his Bachelor degree in Communications and Electronic Engineering (1982) and his Master degree in Computational and Information Systems (1985) both from the University of the Americas in Puebla (UDLAP), Mexico. He has a Ph.D. degree (1999) in Optical Sciences from the National Institute of Astrophysics, Optics, and Electronics (INAOE) in Tonantzintla, Mexico and made a postdoctoral residence, between 2001 and 2002, as invited faculty at the CISE Department, University of Florida. Also, from 2001 to 2020 was awarded the distinction of National Researcher from the Mexican National Council of Science and Technology (SNI-CONACYT). Currently is an Associate Professor in the Optics Department at INAOE. His research interests include digital image processing and analysis, artificial neural networks based on lattice algebra, and lattice computing applied to artificial intelligence and pattern recognition.
1. Elements of Algebra. 1.1. Sets, Functions, and Notations. 1.2. Algebraic
Structures. 2. Pertinent Properties of R. 2.2. Elementary Properties of
Euclidean Spaces. 3. Lattice Theory. 3.1. Historical Background. 3.2.
Partial Orders and Lattices. 3.3. Relations with other branches of
Mathematics. 4. Lattice Algebra. 4.1. Lattice Semigroups and Lattice
Groups. 4.2. Minimax Algebra. 4.3. Minimax Matrix Theory. 4.4. The Geometry
of S(X).5. Matrix-Based Lattice Associative Memories. 5.1. Historical
Background. 5.2. Associative Memories. 6. Extreme Points of Data Sets.
6.1. Relevant Concepts of Convex Set Theory. 6.2. Affine Subsets of EXT(
ß(X)).7. Image Unmixing and Segmentation. 7,1, Spectral Endmembers and
Linear Unmixing. 7.2. Aviris Hyperspectral Image Examples. 7.3. Endmembers
and Clustering Validation Indexes. 7.4. Color Image Segmentation. 8.
Lattice-Based Biomimetic Neural Networks. 8.1. Biomimetics Artificial
Neural Networks. 8.2. Lattice Biomimetic Neural Networks. 9. Learning in
Biomimetic Neural Networks. 9.1 Learning in Single-Layer LBNNS. 9.2.
Multi-Layer Lattice Biomimetic Neural Network. Epilogues. Bibliography.
Structures. 2. Pertinent Properties of R. 2.2. Elementary Properties of
Euclidean Spaces. 3. Lattice Theory. 3.1. Historical Background. 3.2.
Partial Orders and Lattices. 3.3. Relations with other branches of
Mathematics. 4. Lattice Algebra. 4.1. Lattice Semigroups and Lattice
Groups. 4.2. Minimax Algebra. 4.3. Minimax Matrix Theory. 4.4. The Geometry
of S(X).5. Matrix-Based Lattice Associative Memories. 5.1. Historical
Background. 5.2. Associative Memories. 6. Extreme Points of Data Sets.
6.1. Relevant Concepts of Convex Set Theory. 6.2. Affine Subsets of EXT(
ß(X)).7. Image Unmixing and Segmentation. 7,1, Spectral Endmembers and
Linear Unmixing. 7.2. Aviris Hyperspectral Image Examples. 7.3. Endmembers
and Clustering Validation Indexes. 7.4. Color Image Segmentation. 8.
Lattice-Based Biomimetic Neural Networks. 8.1. Biomimetics Artificial
Neural Networks. 8.2. Lattice Biomimetic Neural Networks. 9. Learning in
Biomimetic Neural Networks. 9.1 Learning in Single-Layer LBNNS. 9.2.
Multi-Layer Lattice Biomimetic Neural Network. Epilogues. Bibliography.
1. Elements of Algebra. 1.1. Sets, Functions, and Notations. 1.2. Algebraic
Structures. 2. Pertinent Properties of R. 2.2. Elementary Properties of
Euclidean Spaces. 3. Lattice Theory. 3.1. Historical Background. 3.2.
Partial Orders and Lattices. 3.3. Relations with other branches of
Mathematics. 4. Lattice Algebra. 4.1. Lattice Semigroups and Lattice
Groups. 4.2. Minimax Algebra. 4.3. Minimax Matrix Theory. 4.4. The Geometry
of S(X).5. Matrix-Based Lattice Associative Memories. 5.1. Historical
Background. 5.2. Associative Memories. 6. Extreme Points of Data Sets.
6.1. Relevant Concepts of Convex Set Theory. 6.2. Affine Subsets of EXT(
ß(X)).7. Image Unmixing and Segmentation. 7,1, Spectral Endmembers and
Linear Unmixing. 7.2. Aviris Hyperspectral Image Examples. 7.3. Endmembers
and Clustering Validation Indexes. 7.4. Color Image Segmentation. 8.
Lattice-Based Biomimetic Neural Networks. 8.1. Biomimetics Artificial
Neural Networks. 8.2. Lattice Biomimetic Neural Networks. 9. Learning in
Biomimetic Neural Networks. 9.1 Learning in Single-Layer LBNNS. 9.2.
Multi-Layer Lattice Biomimetic Neural Network. Epilogues. Bibliography.
Structures. 2. Pertinent Properties of R. 2.2. Elementary Properties of
Euclidean Spaces. 3. Lattice Theory. 3.1. Historical Background. 3.2.
Partial Orders and Lattices. 3.3. Relations with other branches of
Mathematics. 4. Lattice Algebra. 4.1. Lattice Semigroups and Lattice
Groups. 4.2. Minimax Algebra. 4.3. Minimax Matrix Theory. 4.4. The Geometry
of S(X).5. Matrix-Based Lattice Associative Memories. 5.1. Historical
Background. 5.2. Associative Memories. 6. Extreme Points of Data Sets.
6.1. Relevant Concepts of Convex Set Theory. 6.2. Affine Subsets of EXT(
ß(X)).7. Image Unmixing and Segmentation. 7,1, Spectral Endmembers and
Linear Unmixing. 7.2. Aviris Hyperspectral Image Examples. 7.3. Endmembers
and Clustering Validation Indexes. 7.4. Color Image Segmentation. 8.
Lattice-Based Biomimetic Neural Networks. 8.1. Biomimetics Artificial
Neural Networks. 8.2. Lattice Biomimetic Neural Networks. 9. Learning in
Biomimetic Neural Networks. 9.1 Learning in Single-Layer LBNNS. 9.2.
Multi-Layer Lattice Biomimetic Neural Network. Epilogues. Bibliography.