Linear Model Theory (eBook, PDF)
Univariate, Multivariate, and Mixed Models
Alle Infos zum eBook verschenken
Linear Model Theory (eBook, PDF)
Univariate, Multivariate, and Mixed Models
- Format: PDF
- Merkliste
- Auf die Merkliste
- Bewerten Bewerten
- Teilen
- Produkt teilen
- Produkterinnerung
- Produkterinnerung
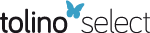
Hier können Sie sich einloggen
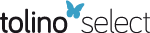
Bitte loggen Sie sich zunächst in Ihr Kundenkonto ein oder registrieren Sie sich bei bücher.de, um das eBook-Abo tolino select nutzen zu können.
A precise and accessible presentation of linear model theory, illustrated with data examples Statisticians often use linear models for data analysis and for developing new statistical methods. Most books on the subject have historically discussed univariate, multivariate, and mixed linear models separately, whereas Linear Model Theory: Univariate, Multivariate, and Mixed Models presents a unified treatment in order to make clear the distinctions among the three classes of models. Linear Model Theory: Univariate, Multivariate, and Mixed Models begins with six chapters devoted to providing brief…mehr
- Geräte: PC
- mit Kopierschutz
- eBook Hilfe
- Größe: 24.47MB
- Eric J. BehCorrespondence Analysis (eBook, PDF)81,99 €
- P. M. KroonenbergApplied Multiway Data Analysis (eBook, PDF)148,99 €
- A. K. Md. Ehsanes SalehStatistical Inference for Models with Multivariate t-Distributed Errors (eBook, PDF)99,99 €
- Daniel ZeltermanDiscrete Distributions (eBook, PDF)103,99 €
- Raymond H. MyersGeneralized Linear Models (eBook, PDF)135,99 €
- Linda M. CollinsLatent Class and Latent Transition Analysis (eBook, PDF)118,99 €
- Alvin C. RencherLinear Models in Statistics (eBook, PDF)162,99 €
-
-
-
Dieser Download kann aus rechtlichen Gründen nur mit Rechnungsadresse in A, B, BG, CY, CZ, D, DK, EW, E, FIN, F, GR, HR, H, IRL, I, LT, L, LR, M, NL, PL, P, R, S, SLO, SK ausgeliefert werden.
- Produktdetails
- Verlag: John Wiley & Sons
- Seitenzahl: 424
- Erscheinungstermin: 31. August 2006
- Englisch
- ISBN-13: 9780470052136
- Artikelnr.: 37290099
- Verlag: John Wiley & Sons
- Seitenzahl: 424
- Erscheinungstermin: 31. August 2006
- Englisch
- ISBN-13: 9780470052136
- Artikelnr.: 37290099
- Herstellerkennzeichnung Die Herstellerinformationen sind derzeit nicht verfügbar.
. 2.11 Sufficient Statistics for the Univariate Model. Exercises. 3. The General Linear Multivariate Model. 3.1 Motivation. 3.2 Definition of the Multivariate Model. 3.3 The Multivariate General Linear Hypothesis. 3.4 Tests About Covariance Matrices. 3.5 Population Correlation. 3.6 Statistical Estimates. 3.7 Overview of Testing Multivariate Hypotheses. 3.8 Computing MULTIREP Tests. 3.9 Computing UNIREP tests. 3.10 Confidence Regions for
. 3.11 Sufficient Statistics for the Multivariate Model. 3.12 Allowing Missing Data in the Multivariate Model. Exercises. 4. Generalizations of the Multivariate Linear Model. 4.1 Motivation. 4.2 The Generalized General Linear Univariate Model: Exact and Approximate Weighted Least Squares. 4.3 Doubly Multivariate Models. 4.4 Seemingly Unrelated Regression. 4.5 Growth Curve Models (GMANOVA). 4.6 The Relationship of the GCM to the Multivariate Model. 4.7 Mixed, Hierarchical, and Related Models. 5. The Linear Mixed Model. 5.1 Motivation. 5.2 Definition of the Mixed Model. 5.3 Distribution-Free and Noniterative Estimates. 5.4 Gaussian Likelihood and Iterative Estimates. 5.5 Tests about ß (Means, Fixed Effects). 5.6 Tests of Covariance Parameters,
(random Effects). Exercises. 6. Choosing the Form of a Linear Model for Analysis. 6.1 The Importance of Understanding Dependence. 6.2 How Many Variables per Independent Sampling Unit? 6.3 What Types of Variables Play a Role? 6.4 What Repeated Sampling Scheme Was Used? 6.5 Analysis Strategies for Multivariate Data. 6.6 cautions and Recommendations. 6.7 Review of Linear Model Notation. PART II: MULTIVARIATE DISTRIBUTION THEORY. 7. General Theory of Multivariate Distributions. 7.1 Motivation. 7.2 Notation and Concepts. 7.3 Families of Distributions. 7.4 Cumulative Distribution Function. 7.5 Probability Density Function. 7.6 Formulas for Probabilities and Moments. 7.7 Characteristic Function. 7.8 Moment Generating Function. 7.9 Cumulant generating Function. 7.10 Transforming Random Variables. 7.11 Marginal Distributions. 7.12 Independence of Random Vectors. 7.13 Conditional Distributions. 7.14 (Joint) Moments of Multivariate Distributions. 7.15 Conditional Moments of Distributions. 7.16 Special Considerations for Random Matrices. 8. Scalar, vector, and Matrix Gaussian Distributions. 8.1 Motivation. 8.2 The Scalar Gaussian Distribution. 8.3 The Vector ("Multivariate") Gaussian Distribution. 8.4 Marginal Distributions. 8.5 Independence. 8.6 Conditional Distributions. 8.7 Asymptotic Properties. 8.8 The matrix Gaussian Distribution. 8.9 Assessing, Multivariate Gaussian Distribution. 8.10 Tests for Gaussian Distribution. Exercises. 9. Univariate Quadratic Forms. 9.1 Motivation. 9.2 Chi-Square Distributions. 9.3 General Properties of Quadratic Forms. 9.4 Properties of Quadratic Forms in Gaussian Vectors. 9.5 Independence among Linear and Quadratic Forms. 9.6 The ANOVA Theorem. 9.7 Ratios Involving Quadratic Forms. Exercises. 10. Multivariate Quadratic Forms. 10.1 The Wishart Distribution. 10.2 The Characteristic Function of the Wishart. 10.3 Properties of the Wishart. 10.4 The Inverse Wishart. 10.5 Related Distributions. Exercises. PART III: ESTIMATION IN LINEAR MODELS. 11. Estimation for Univariate and Weighted Linear Models. 11.1 Motivation. 11.2 Statement of the Problem. 11.3 (Unrestricted) Linear Equivalent Linear Models. 11.4 Estimability and Criteria for Checking It. 11.5 Coding Schemes and the Essence matrix. 11.6 Unrestricted Maximum Likelihood Estimation of ß. 11.7 Unrestricted BLUE Estimation of ß. 11.8 Unrestricted Least Squares Estimation of ß. 11.9 Unrestricted Maximum Likelihood Estimation of
. 11.10 Unrestricted BLUE of
. 11.11 Related Distributions. 11.12 Formulations of Explicit Restrictions of ß and
. 11.13 Restricted Estimation Via Equivalent Models. 11.14 Fitting Piecewise Polynomial Models Via Splines. 11.15 Estimation for the GGLM: Weighted Least Squares. Exercises. 12. Estimation for Multivariate Linear Models. 12.1 Alternate Formulations of the Model. 12.2 Estimability in the Multivariate GLM. 12.3 Unrestricted Likelihood Estimation. 12.4 Estimation of Secondary Parameters. 12.5 Estimation with Multivariate Restrictions. 12.6 Unrestricted Estimation With Compound Symmetry: the "Univariate" Approach to Repeated Measures. Exercises. 13. Estimation for Generalizations of Multivariate Models. 13.1 Motivation. 13.2 Criteria and Algorithms. 13.3 Weighted Estimation of ß and
. 13.4 Transformations among Growth Curve Designs. 13.5 Within-Individual Design matrices. 13.6 Estimation Methods. 13.7 Relationships to the Univariate and Mixed Models. Exercises. 14. Estimation for Linear Mixed Models. 14.1 Motivation. 14.2 Statement of the General Linear Mixed Models. 14.3 Estimation and Estimability. 14.4 Some Special Types of Models. 14.5 ML Estimation. 14.6 REML Estimation. 14.7 Small-Sample Properties of Estimators. 14.8 Large-Sample Properties of Variance Estimators. 14.9 Conditional Estimation of di and BLUP Prediction. Exercises. PART IV: TESTS IN GAUSSIAN LINEAR MODELS. 15. Tests for Univariate Linear Models. 15.1 Motivation. 15.2 Testability of Univariate Hypotheses. 15.3 Tests of a Priori Hypotheses. 15.4 Related Distributions. 15.5 Transformations and Invariance Properties. 15.6 Confidence Regions for
. Exercises. 16. Tests for Multivariate Linear Models. 16.1 Motivation. 16.2 Testability of Multivariate Hypotheses. 16.3 Tests of a Priori Hypotheses. 16.4 Linear Invariance. 16.5 Four Multivariate Test Statistics. 16.6 Which Multivariate Test Is Best? 16.7 Univariate Approach to Repeated Measures: UNIREP. 16.8 More on Invariance Properties. 16.9 Tests of Hypotheses about
. 16.10 Confidence Regions for
. Exercises. 17. Tests for Generalizations of Multivariate Linear Models. 17.1 Motivation. 17.2 Doubly Multivariate Models. 17.3 Missing Responses in Multivariate Linear Models. 17.4 Exact and Approximate Weighted Least Squares. 17.5 Seemingly Unrelated Regressions. 17.6 Growth Curve Models (GMANOVA). 17.7 Testing Hypotheses in the GCM. 17.8 Confidence Bands for Growth Curves. 18. Tests for Linear Mixed Models. 18.1 Overview. 18.2 Estimability of
= Cß. 18.3 Likelihood Ratio Tests of Cß. 18.4 Likelihood Ratio Tests Involving
. 18.5 Test Size of Wald-Type Tests of ß Using REML. 18.6 Using Wald-Type Tests of ß with REML. 18.7 Using Wald-Type Tests of {ß,
} with REML. 19. A Review of Multivariate and Univariate Linear Models. 19.1 Matrix Gaussian and Wishart Properties. 19.2 Design Matrix Properties. 19.3 Model Components. 19.4 Primary Parameter and Related Estimators. 19.5 Secondary Parameter Estimators. 19.6 Added-Last and Added-in-Order Tests. PART V: CHOOSING A SIMPLE SIZE IN GAUSSIAN LINEAR MODELS. 20. Sample Size for Univariate Linear Model. 20.1 Sample Size Consulting: Before You begin. 20.2 The Machinery of a Power Analysis. 20.3 Independent t Example. 20.4 Paired t Example. 20.5 The Impact of
2 or ß in Power analysis. 20.6 Random Predictors. 20.7 Internal Pilot Design. 20.8 Other Criteria for Choosing a Sample Size. Exercises. 21. Sample Size for Multivariate Linear Model. 21.1 The Machinery of a Power Analysis. 21.2 Paired t Example. 21.3 Time by Treatment Example. 21.4 Comparing between and within Designs. 21.5 Some Invariance Properties. 21.6 Random Predictors. 21.7 Internal Pilot Designs. Exercises. 22. Sample Size for Generalizations of Multivariate Models. 22.1 Motivation. 22.2 Sample Size Methods for Growth Curve Models. 23. Sample Size for Linear Mixed Models. 23.1 Motivation. 23.2 Methods. 23.3 Internal Pilot Designs. Appendix: Computing Resources. References. Index.
. 2.11 Sufficient Statistics for the Univariate Model. Exercises. 3. The General Linear Multivariate Model. 3.1 Motivation. 3.2 Definition of the Multivariate Model. 3.3 The Multivariate General Linear Hypothesis. 3.4 Tests About Covariance Matrices. 3.5 Population Correlation. 3.6 Statistical Estimates. 3.7 Overview of Testing Multivariate Hypotheses. 3.8 Computing MULTIREP Tests. 3.9 Computing UNIREP tests. 3.10 Confidence Regions for
. 3.11 Sufficient Statistics for the Multivariate Model. 3.12 Allowing Missing Data in the Multivariate Model. Exercises. 4. Generalizations of the Multivariate Linear Model. 4.1 Motivation. 4.2 The Generalized General Linear Univariate Model: Exact and Approximate Weighted Least Squares. 4.3 Doubly Multivariate Models. 4.4 Seemingly Unrelated Regression. 4.5 Growth Curve Models (GMANOVA). 4.6 The Relationship of the GCM to the Multivariate Model. 4.7 Mixed, Hierarchical, and Related Models. 5. The Linear Mixed Model. 5.1 Motivation. 5.2 Definition of the Mixed Model. 5.3 Distribution-Free and Noniterative Estimates. 5.4 Gaussian Likelihood and Iterative Estimates. 5.5 Tests about ß (Means, Fixed Effects). 5.6 Tests of Covariance Parameters,
(random Effects). Exercises. 6. Choosing the Form of a Linear Model for Analysis. 6.1 The Importance of Understanding Dependence. 6.2 How Many Variables per Independent Sampling Unit? 6.3 What Types of Variables Play a Role? 6.4 What Repeated Sampling Scheme Was Used? 6.5 Analysis Strategies for Multivariate Data. 6.6 cautions and Recommendations. 6.7 Review of Linear Model Notation. PART II: MULTIVARIATE DISTRIBUTION THEORY. 7. General Theory of Multivariate Distributions. 7.1 Motivation. 7.2 Notation and Concepts. 7.3 Families of Distributions. 7.4 Cumulative Distribution Function. 7.5 Probability Density Function. 7.6 Formulas for Probabilities and Moments. 7.7 Characteristic Function. 7.8 Moment Generating Function. 7.9 Cumulant generating Function. 7.10 Transforming Random Variables. 7.11 Marginal Distributions. 7.12 Independence of Random Vectors. 7.13 Conditional Distributions. 7.14 (Joint) Moments of Multivariate Distributions. 7.15 Conditional Moments of Distributions. 7.16 Special Considerations for Random Matrices. 8. Scalar, vector, and Matrix Gaussian Distributions. 8.1 Motivation. 8.2 The Scalar Gaussian Distribution. 8.3 The Vector ("Multivariate") Gaussian Distribution. 8.4 Marginal Distributions. 8.5 Independence. 8.6 Conditional Distributions. 8.7 Asymptotic Properties. 8.8 The matrix Gaussian Distribution. 8.9 Assessing, Multivariate Gaussian Distribution. 8.10 Tests for Gaussian Distribution. Exercises. 9. Univariate Quadratic Forms. 9.1 Motivation. 9.2 Chi-Square Distributions. 9.3 General Properties of Quadratic Forms. 9.4 Properties of Quadratic Forms in Gaussian Vectors. 9.5 Independence among Linear and Quadratic Forms. 9.6 The ANOVA Theorem. 9.7 Ratios Involving Quadratic Forms. Exercises. 10. Multivariate Quadratic Forms. 10.1 The Wishart Distribution. 10.2 The Characteristic Function of the Wishart. 10.3 Properties of the Wishart. 10.4 The Inverse Wishart. 10.5 Related Distributions. Exercises. PART III: ESTIMATION IN LINEAR MODELS. 11. Estimation for Univariate and Weighted Linear Models. 11.1 Motivation. 11.2 Statement of the Problem. 11.3 (Unrestricted) Linear Equivalent Linear Models. 11.4 Estimability and Criteria for Checking It. 11.5 Coding Schemes and the Essence matrix. 11.6 Unrestricted Maximum Likelihood Estimation of ß. 11.7 Unrestricted BLUE Estimation of ß. 11.8 Unrestricted Least Squares Estimation of ß. 11.9 Unrestricted Maximum Likelihood Estimation of
. 11.10 Unrestricted BLUE of
. 11.11 Related Distributions. 11.12 Formulations of Explicit Restrictions of ß and
. 11.13 Restricted Estimation Via Equivalent Models. 11.14 Fitting Piecewise Polynomial Models Via Splines. 11.15 Estimation for the GGLM: Weighted Least Squares. Exercises. 12. Estimation for Multivariate Linear Models. 12.1 Alternate Formulations of the Model. 12.2 Estimability in the Multivariate GLM. 12.3 Unrestricted Likelihood Estimation. 12.4 Estimation of Secondary Parameters. 12.5 Estimation with Multivariate Restrictions. 12.6 Unrestricted Estimation With Compound Symmetry: the "Univariate" Approach to Repeated Measures. Exercises. 13. Estimation for Generalizations of Multivariate Models. 13.1 Motivation. 13.2 Criteria and Algorithms. 13.3 Weighted Estimation of ß and
. 13.4 Transformations among Growth Curve Designs. 13.5 Within-Individual Design matrices. 13.6 Estimation Methods. 13.7 Relationships to the Univariate and Mixed Models. Exercises. 14. Estimation for Linear Mixed Models. 14.1 Motivation. 14.2 Statement of the General Linear Mixed Models. 14.3 Estimation and Estimability. 14.4 Some Special Types of Models. 14.5 ML Estimation. 14.6 REML Estimation. 14.7 Small-Sample Properties of Estimators. 14.8 Large-Sample Properties of Variance Estimators. 14.9 Conditional Estimation of di and BLUP Prediction. Exercises. PART IV: TESTS IN GAUSSIAN LINEAR MODELS. 15. Tests for Univariate Linear Models. 15.1 Motivation. 15.2 Testability of Univariate Hypotheses. 15.3 Tests of a Priori Hypotheses. 15.4 Related Distributions. 15.5 Transformations and Invariance Properties. 15.6 Confidence Regions for
. Exercises. 16. Tests for Multivariate Linear Models. 16.1 Motivation. 16.2 Testability of Multivariate Hypotheses. 16.3 Tests of a Priori Hypotheses. 16.4 Linear Invariance. 16.5 Four Multivariate Test Statistics. 16.6 Which Multivariate Test Is Best? 16.7 Univariate Approach to Repeated Measures: UNIREP. 16.8 More on Invariance Properties. 16.9 Tests of Hypotheses about
. 16.10 Confidence Regions for
. Exercises. 17. Tests for Generalizations of Multivariate Linear Models. 17.1 Motivation. 17.2 Doubly Multivariate Models. 17.3 Missing Responses in Multivariate Linear Models. 17.4 Exact and Approximate Weighted Least Squares. 17.5 Seemingly Unrelated Regressions. 17.6 Growth Curve Models (GMANOVA). 17.7 Testing Hypotheses in the GCM. 17.8 Confidence Bands for Growth Curves. 18. Tests for Linear Mixed Models. 18.1 Overview. 18.2 Estimability of
= Cß. 18.3 Likelihood Ratio Tests of Cß. 18.4 Likelihood Ratio Tests Involving
. 18.5 Test Size of Wald-Type Tests of ß Using REML. 18.6 Using Wald-Type Tests of ß with REML. 18.7 Using Wald-Type Tests of {ß,
} with REML. 19. A Review of Multivariate and Univariate Linear Models. 19.1 Matrix Gaussian and Wishart Properties. 19.2 Design Matrix Properties. 19.3 Model Components. 19.4 Primary Parameter and Related Estimators. 19.5 Secondary Parameter Estimators. 19.6 Added-Last and Added-in-Order Tests. PART V: CHOOSING A SIMPLE SIZE IN GAUSSIAN LINEAR MODELS. 20. Sample Size for Univariate Linear Model. 20.1 Sample Size Consulting: Before You begin. 20.2 The Machinery of a Power Analysis. 20.3 Independent t Example. 20.4 Paired t Example. 20.5 The Impact of
2 or ß in Power analysis. 20.6 Random Predictors. 20.7 Internal Pilot Design. 20.8 Other Criteria for Choosing a Sample Size. Exercises. 21. Sample Size for Multivariate Linear Model. 21.1 The Machinery of a Power Analysis. 21.2 Paired t Example. 21.3 Time by Treatment Example. 21.4 Comparing between and within Designs. 21.5 Some Invariance Properties. 21.6 Random Predictors. 21.7 Internal Pilot Designs. Exercises. 22. Sample Size for Generalizations of Multivariate Models. 22.1 Motivation. 22.2 Sample Size Methods for Growth Curve Models. 23. Sample Size for Linear Mixed Models. 23.1 Motivation. 23.2 Methods. 23.3 Internal Pilot Designs. Appendix: Computing Resources. References. Index.