71,95 €
71,95 €
inkl. MwSt.
Sofort per Download lieferbar
36 °P sammeln
71,95 €
Als Download kaufen
71,95 €
inkl. MwSt.
Sofort per Download lieferbar
36 °P sammeln
Jetzt verschenken
Alle Infos zum eBook verschenken
71,95 €
inkl. MwSt.
Sofort per Download lieferbar
Alle Infos zum eBook verschenken
36 °P sammeln
- Format: PDF
- Merkliste
- Auf die Merkliste
- Bewerten Bewerten
- Teilen
- Produkt teilen
- Produkterinnerung
- Produkterinnerung
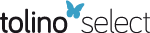
Bitte loggen Sie sich zunächst in Ihr Kundenkonto ein oder registrieren Sie sich bei
bücher.de, um das eBook-Abo tolino select nutzen zu können.
Hier können Sie sich einloggen
Hier können Sie sich einloggen
Sie sind bereits eingeloggt. Klicken Sie auf 2. tolino select Abo, um fortzufahren.
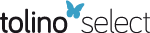
Bitte loggen Sie sich zunächst in Ihr Kundenkonto ein oder registrieren Sie sich bei bücher.de, um das eBook-Abo tolino select nutzen zu können.
This book includes chapters on specifying the correct linear regression model, adjusting for measurement error, understanding the effects of influential observations, and using multilevel data.
- Geräte: PC
- ohne Kopierschutz
- eBook Hilfe
- Größe: 104.53MB
Andere Kunden interessierten sich auch für
- John P. HoffmannLinear Regression Models (eBook, ePUB)71,95 €
- Christer ThraneDoing Statistical Analysis (eBook, PDF)52,95 €
- Jocelyn E. BolinRegression Analysis in R (eBook, PDF)61,95 €
- E. Michael NussbaumCategorical and Nonparametric Data Analysis (eBook, PDF)83,95 €
- Ronald H. HeckMultilevel and Longitudinal Modeling with IBM SPSS (eBook, PDF)49,95 €
- Ronald H HeckMultilevel Modeling of Categorical Outcomes Using IBM SPSS (eBook, PDF)49,95 €
- Debbie L. Hahs-VaughnStatistical Concepts - A Second Course (eBook, PDF)74,95 €
-
-
-
This book includes chapters on specifying the correct linear regression model, adjusting for measurement error, understanding the effects of influential observations, and using multilevel data.
Dieser Download kann aus rechtlichen Gründen nur mit Rechnungsadresse in A, B, BG, CY, CZ, D, DK, EW, E, FIN, F, GR, HR, H, IRL, I, LT, L, LR, M, NL, PL, P, R, S, SLO, SK ausgeliefert werden.
Produktdetails
- Produktdetails
- Verlag: Taylor & Francis eBooks
- Seitenzahl: 436
- Erscheinungstermin: 12. September 2021
- Englisch
- ISBN-13: 9781000437966
- Artikelnr.: 62302363
- Verlag: Taylor & Francis eBooks
- Seitenzahl: 436
- Erscheinungstermin: 12. September 2021
- Englisch
- ISBN-13: 9781000437966
- Artikelnr.: 62302363
- Herstellerkennzeichnung Die Herstellerinformationen sind derzeit nicht verfügbar.
John P. Hoffmann is a professor of sociology at Brigham Young University. He holds a PhD in Criminology from the State University of New York at Albany and a Masters of Public Health (MPH) from Emory University. He has worked at the U.S. Centers for Disease Control and Prevention (CDC) and the National Opinion Research Center (NORC) of the University of Chicago; and taught at Hokkaido University and the University of South Carolina. Hoffmann is the author of more than 100 journal articles and book chapters and 10 books on applied statistics, criminology, and the sociology of religion.
1. Introduction
2. Review of Elementary Statistical Concepts
3. Simple Linear Regression Models
4. Multiple Linear Regression Models
5. The ANOVA Table and Goodness-of-Fit Statistics
6. Comparing Linear Regression Models
7. Indicator Variables in Linear Regression Models
8. Independence
9. Homoscedasticity
10. Collinearity and Multicollinearity
11. Normality, Linearity, and Interaction Effects
12. Model Specification
13. Measurement Errors
14. Influential Observations: Leverage Points and Outliers
15. Multilevel Linear Regression Models
16. A Brief Introduction to Logistic Regression
17. Conclusions
Appendix A: Data Management
Appendix B: Using Simulations to Examine Assumptions of Linear Regression
Models
Appendix C: Formulas
Appendix C: User-Written R Packages Employed in Examples
2. Review of Elementary Statistical Concepts
3. Simple Linear Regression Models
4. Multiple Linear Regression Models
5. The ANOVA Table and Goodness-of-Fit Statistics
6. Comparing Linear Regression Models
7. Indicator Variables in Linear Regression Models
8. Independence
9. Homoscedasticity
10. Collinearity and Multicollinearity
11. Normality, Linearity, and Interaction Effects
12. Model Specification
13. Measurement Errors
14. Influential Observations: Leverage Points and Outliers
15. Multilevel Linear Regression Models
16. A Brief Introduction to Logistic Regression
17. Conclusions
Appendix A: Data Management
Appendix B: Using Simulations to Examine Assumptions of Linear Regression
Models
Appendix C: Formulas
Appendix C: User-Written R Packages Employed in Examples
1. Introduction
2. Review of Elementary Statistical Concepts
3. Simple Linear Regression Models
4. Multiple Linear Regression Models
5. The ANOVA Table and Goodness-of-Fit Statistics
6. Comparing Linear Regression Models
7. Indicator Variables in Linear Regression Models
8. Independence
9. Homoscedasticity
10. Collinearity and Multicollinearity
11. Normality, Linearity, and Interaction Effects
12. Model Specification
13. Measurement Errors
14. Influential Observations: Leverage Points and Outliers
15. Multilevel Linear Regression Models
16. A Brief Introduction to Logistic Regression
17. Conclusions
Appendix A: Data Management
Appendix B: Using Simulations to Examine Assumptions of Linear Regression Models
Appendix C: Formulas
Appendix C: User-Written R Packages Employed in Examples
2. Review of Elementary Statistical Concepts
3. Simple Linear Regression Models
4. Multiple Linear Regression Models
5. The ANOVA Table and Goodness-of-Fit Statistics
6. Comparing Linear Regression Models
7. Indicator Variables in Linear Regression Models
8. Independence
9. Homoscedasticity
10. Collinearity and Multicollinearity
11. Normality, Linearity, and Interaction Effects
12. Model Specification
13. Measurement Errors
14. Influential Observations: Leverage Points and Outliers
15. Multilevel Linear Regression Models
16. A Brief Introduction to Logistic Regression
17. Conclusions
Appendix A: Data Management
Appendix B: Using Simulations to Examine Assumptions of Linear Regression Models
Appendix C: Formulas
Appendix C: User-Written R Packages Employed in Examples
1. Introduction
2. Review of Elementary Statistical Concepts
3. Simple Linear Regression Models
4. Multiple Linear Regression Models
5. The ANOVA Table and Goodness-of-Fit Statistics
6. Comparing Linear Regression Models
7. Indicator Variables in Linear Regression Models
8. Independence
9. Homoscedasticity
10. Collinearity and Multicollinearity
11. Normality, Linearity, and Interaction Effects
12. Model Specification
13. Measurement Errors
14. Influential Observations: Leverage Points and Outliers
15. Multilevel Linear Regression Models
16. A Brief Introduction to Logistic Regression
17. Conclusions
Appendix A: Data Management
Appendix B: Using Simulations to Examine Assumptions of Linear Regression
Models
Appendix C: Formulas
Appendix C: User-Written R Packages Employed in Examples
2. Review of Elementary Statistical Concepts
3. Simple Linear Regression Models
4. Multiple Linear Regression Models
5. The ANOVA Table and Goodness-of-Fit Statistics
6. Comparing Linear Regression Models
7. Indicator Variables in Linear Regression Models
8. Independence
9. Homoscedasticity
10. Collinearity and Multicollinearity
11. Normality, Linearity, and Interaction Effects
12. Model Specification
13. Measurement Errors
14. Influential Observations: Leverage Points and Outliers
15. Multilevel Linear Regression Models
16. A Brief Introduction to Logistic Regression
17. Conclusions
Appendix A: Data Management
Appendix B: Using Simulations to Examine Assumptions of Linear Regression
Models
Appendix C: Formulas
Appendix C: User-Written R Packages Employed in Examples
1. Introduction
2. Review of Elementary Statistical Concepts
3. Simple Linear Regression Models
4. Multiple Linear Regression Models
5. The ANOVA Table and Goodness-of-Fit Statistics
6. Comparing Linear Regression Models
7. Indicator Variables in Linear Regression Models
8. Independence
9. Homoscedasticity
10. Collinearity and Multicollinearity
11. Normality, Linearity, and Interaction Effects
12. Model Specification
13. Measurement Errors
14. Influential Observations: Leverage Points and Outliers
15. Multilevel Linear Regression Models
16. A Brief Introduction to Logistic Regression
17. Conclusions
Appendix A: Data Management
Appendix B: Using Simulations to Examine Assumptions of Linear Regression Models
Appendix C: Formulas
Appendix C: User-Written R Packages Employed in Examples
2. Review of Elementary Statistical Concepts
3. Simple Linear Regression Models
4. Multiple Linear Regression Models
5. The ANOVA Table and Goodness-of-Fit Statistics
6. Comparing Linear Regression Models
7. Indicator Variables in Linear Regression Models
8. Independence
9. Homoscedasticity
10. Collinearity and Multicollinearity
11. Normality, Linearity, and Interaction Effects
12. Model Specification
13. Measurement Errors
14. Influential Observations: Leverage Points and Outliers
15. Multilevel Linear Regression Models
16. A Brief Introduction to Logistic Regression
17. Conclusions
Appendix A: Data Management
Appendix B: Using Simulations to Examine Assumptions of Linear Regression Models
Appendix C: Formulas
Appendix C: User-Written R Packages Employed in Examples