Machine Learning Applications in Subsurface Energy Resource Management (eBook, ePUB)
State of the Art and Future Prognosis
Redaktion: Mishra, Srikanta
119,95 €
119,95 €
inkl. MwSt.
Sofort per Download lieferbar
60 °P sammeln
119,95 €
Als Download kaufen
119,95 €
inkl. MwSt.
Sofort per Download lieferbar
60 °P sammeln
Jetzt verschenken
Alle Infos zum eBook verschenken
119,95 €
inkl. MwSt.
Sofort per Download lieferbar
Alle Infos zum eBook verschenken
60 °P sammeln
Machine Learning Applications in Subsurface Energy Resource Management (eBook, ePUB)
State of the Art and Future Prognosis
Redaktion: Mishra, Srikanta
- Format: ePub
- Merkliste
- Auf die Merkliste
- Bewerten Bewerten
- Teilen
- Produkt teilen
- Produkterinnerung
- Produkterinnerung
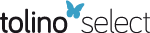
Bitte loggen Sie sich zunächst in Ihr Kundenkonto ein oder registrieren Sie sich bei
bücher.de, um das eBook-Abo tolino select nutzen zu können.
Hier können Sie sich einloggen
Hier können Sie sich einloggen
Sie sind bereits eingeloggt. Klicken Sie auf 2. tolino select Abo, um fortzufahren.
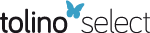
Bitte loggen Sie sich zunächst in Ihr Kundenkonto ein oder registrieren Sie sich bei bücher.de, um das eBook-Abo tolino select nutzen zu können.
Machine Learning Applications in Subsurface Energy Resource Management presents a current snapshot of the state of the art and future outlook for machine learning applications in subsurface energy resource management (e.g., oil and gas, geologic carbon sequestration, geothermal energy).
- Geräte: eReader
- ohne Kopierschutz
- eBook Hilfe
- Größe: 10.83MB
Andere Kunden interessierten sich auch für
- Machine Learning Applications in Subsurface Energy Resource Management (eBook, PDF)119,95 €
- Shahab D. MohagheghArtificial Intelligence for Science and Engineering Applications (eBook, ePUB)80,95 €
- Distributed Artificial Intelligence (eBook, ePUB)59,95 €
- Hybrid Intelligence for Smart Grid Systems (eBook, ePUB)47,95 €
- AI in Manufacturing and Green Technology (eBook, ePUB)47,95 €
- Integrating Deep Learning Algorithms to Overcome Challenges in Big Data Analytics (eBook, ePUB)50,95 €
- Maged MarghanyAutomatic Detection Algorithms of Oil Spill in Radar Images (eBook, ePUB)47,95 €
-
-
-
Machine Learning Applications in Subsurface Energy Resource Management presents a current snapshot of the state of the art and future outlook for machine learning applications in subsurface energy resource management (e.g., oil and gas, geologic carbon sequestration, geothermal energy).
Dieser Download kann aus rechtlichen Gründen nur mit Rechnungsadresse in A, B, BG, CY, CZ, D, DK, EW, E, FIN, F, GR, HR, H, IRL, I, LT, L, LR, M, NL, PL, P, R, S, SLO, SK ausgeliefert werden.
Produktdetails
- Produktdetails
- Verlag: Taylor & Francis eBooks
- Seitenzahl: 378
- Erscheinungstermin: 27. Dezember 2022
- Englisch
- ISBN-13: 9781000823899
- Artikelnr.: 66653301
- Verlag: Taylor & Francis eBooks
- Seitenzahl: 378
- Erscheinungstermin: 27. Dezember 2022
- Englisch
- ISBN-13: 9781000823899
- Artikelnr.: 66653301
- Herstellerkennzeichnung Die Herstellerinformationen sind derzeit nicht verfügbar.
Dr. Srikanta Mishra is Senior Research Leader and Technical Director for Geo-energy Resource Modeling and Analytics at Battelle Memorial Institute, the world's largest independent contract R&D organization. He is nationally and internationally recognized for his expertise in developing and communicating physics-based and data-driven predictive models for subsurface resource management. Dr. Mishra currently serves as the Technical Lead of the SMART (Science Informed Machine Learning for Accelerating Real-time Decisions for Subsurface applications) initiative, organized by the US Department of Energy and involving multiple national laboratories and universities. He was a recipient of the Society of Petroleum Engineers (SPE) Distinguished Member Award in 2021, and also served as a Global Distinguished Lecturer on Big Data Analytics for SPE during 2018-19 and received the 2022 SPE Data Science and Engineering Analytics Award.
Section I: Introduction
1. Machine Learning Applications in Subsurface Energy Resource Management: State of the Art
2. Solving Problems with Data Science
Section II: Reservoir Characterization Applications
3. Machine Learning-Aided Characterization Using Geophysical Data Modalities
4. Machine Learning to Discover
Characterize
and Produce Geothermal Energy
Section III: Drilling Operations Applications
5. Real-Time Drilling and Completion Analytics: From Cloud Computing to Edge Computing and Their Machine Learning Applications
6. Using Machine Learning to Improve Drilling of Unconventional Resources
Section IV: Production Data Analysis Applications
7. Machine Learning Assisted Production Data Filtering and Decline Curve Analysis in Unconventional Plays
8. Hybrid Data-Driven and Physics-Informed Reservoir Modeling for Unconventional Reservoirs
9. Role of Analytics in Extracting Data-Driven Models from Reservoir Surveillance
10. Machine Learning Assisted Forecasting of Reservoir Performance
Section V: Reservoir Modeling Applications
11. An Efficient Deep Learning Based Workflow Incorporating a Reduced Physics Model for Drainage Volume Visualization in Unconventional Reservoirs
12. Reservoir Modeling Using Fast Predictive Machine Learning Algorithms for Geological Carbon Storage
13. Physics-Embedded Machine Learning for Modeling and Optimization of Mature Fields
14. Deep Neural Network Surrogate Flow Models for History Matching and Uncertainty Quantification
15. Generalizable Field Development Optimization Using Deep Reinforcement Learning with Field Examples
Section VI: Predictive Maintenance Applications
16. Case Studies Involving Machine Learning for Predictive Maintenance in Oil and Gas Production Operations
17. Machine Learning for Multiphase Flow Metering
Section VII: Summary and Future Outlook
18. Machine Learning Applications in Subsurface Energy Resource Management: Future Prognosis
1. Machine Learning Applications in Subsurface Energy Resource Management: State of the Art
2. Solving Problems with Data Science
Section II: Reservoir Characterization Applications
3. Machine Learning-Aided Characterization Using Geophysical Data Modalities
4. Machine Learning to Discover
Characterize
and Produce Geothermal Energy
Section III: Drilling Operations Applications
5. Real-Time Drilling and Completion Analytics: From Cloud Computing to Edge Computing and Their Machine Learning Applications
6. Using Machine Learning to Improve Drilling of Unconventional Resources
Section IV: Production Data Analysis Applications
7. Machine Learning Assisted Production Data Filtering and Decline Curve Analysis in Unconventional Plays
8. Hybrid Data-Driven and Physics-Informed Reservoir Modeling for Unconventional Reservoirs
9. Role of Analytics in Extracting Data-Driven Models from Reservoir Surveillance
10. Machine Learning Assisted Forecasting of Reservoir Performance
Section V: Reservoir Modeling Applications
11. An Efficient Deep Learning Based Workflow Incorporating a Reduced Physics Model for Drainage Volume Visualization in Unconventional Reservoirs
12. Reservoir Modeling Using Fast Predictive Machine Learning Algorithms for Geological Carbon Storage
13. Physics-Embedded Machine Learning for Modeling and Optimization of Mature Fields
14. Deep Neural Network Surrogate Flow Models for History Matching and Uncertainty Quantification
15. Generalizable Field Development Optimization Using Deep Reinforcement Learning with Field Examples
Section VI: Predictive Maintenance Applications
16. Case Studies Involving Machine Learning for Predictive Maintenance in Oil and Gas Production Operations
17. Machine Learning for Multiphase Flow Metering
Section VII: Summary and Future Outlook
18. Machine Learning Applications in Subsurface Energy Resource Management: Future Prognosis
Section I: Introduction
1. Machine Learning Applications in Subsurface Energy Resource Management: State of the Art
2. Solving Problems with Data Science
Section II: Reservoir Characterization Applications
3. Machine Learning-Aided Characterization Using Geophysical Data Modalities
4. Machine Learning to Discover
Characterize
and Produce Geothermal Energy
Section III: Drilling Operations Applications
5. Real-Time Drilling and Completion Analytics: From Cloud Computing to Edge Computing and Their Machine Learning Applications
6. Using Machine Learning to Improve Drilling of Unconventional Resources
Section IV: Production Data Analysis Applications
7. Machine Learning Assisted Production Data Filtering and Decline Curve Analysis in Unconventional Plays
8. Hybrid Data-Driven and Physics-Informed Reservoir Modeling for Unconventional Reservoirs
9. Role of Analytics in Extracting Data-Driven Models from Reservoir Surveillance
10. Machine Learning Assisted Forecasting of Reservoir Performance
Section V: Reservoir Modeling Applications
11. An Efficient Deep Learning Based Workflow Incorporating a Reduced Physics Model for Drainage Volume Visualization in Unconventional Reservoirs
12. Reservoir Modeling Using Fast Predictive Machine Learning Algorithms for Geological Carbon Storage
13. Physics-Embedded Machine Learning for Modeling and Optimization of Mature Fields
14. Deep Neural Network Surrogate Flow Models for History Matching and Uncertainty Quantification
15. Generalizable Field Development Optimization Using Deep Reinforcement Learning with Field Examples
Section VI: Predictive Maintenance Applications
16. Case Studies Involving Machine Learning for Predictive Maintenance in Oil and Gas Production Operations
17. Machine Learning for Multiphase Flow Metering
Section VII: Summary and Future Outlook
18. Machine Learning Applications in Subsurface Energy Resource Management: Future Prognosis
1. Machine Learning Applications in Subsurface Energy Resource Management: State of the Art
2. Solving Problems with Data Science
Section II: Reservoir Characterization Applications
3. Machine Learning-Aided Characterization Using Geophysical Data Modalities
4. Machine Learning to Discover
Characterize
and Produce Geothermal Energy
Section III: Drilling Operations Applications
5. Real-Time Drilling and Completion Analytics: From Cloud Computing to Edge Computing and Their Machine Learning Applications
6. Using Machine Learning to Improve Drilling of Unconventional Resources
Section IV: Production Data Analysis Applications
7. Machine Learning Assisted Production Data Filtering and Decline Curve Analysis in Unconventional Plays
8. Hybrid Data-Driven and Physics-Informed Reservoir Modeling for Unconventional Reservoirs
9. Role of Analytics in Extracting Data-Driven Models from Reservoir Surveillance
10. Machine Learning Assisted Forecasting of Reservoir Performance
Section V: Reservoir Modeling Applications
11. An Efficient Deep Learning Based Workflow Incorporating a Reduced Physics Model for Drainage Volume Visualization in Unconventional Reservoirs
12. Reservoir Modeling Using Fast Predictive Machine Learning Algorithms for Geological Carbon Storage
13. Physics-Embedded Machine Learning for Modeling and Optimization of Mature Fields
14. Deep Neural Network Surrogate Flow Models for History Matching and Uncertainty Quantification
15. Generalizable Field Development Optimization Using Deep Reinforcement Learning with Field Examples
Section VI: Predictive Maintenance Applications
16. Case Studies Involving Machine Learning for Predictive Maintenance in Oil and Gas Production Operations
17. Machine Learning for Multiphase Flow Metering
Section VII: Summary and Future Outlook
18. Machine Learning Applications in Subsurface Energy Resource Management: Future Prognosis