Machine Learning Applied to Composite Materials (eBook, PDF)
Schade – dieser Artikel ist leider ausverkauft. Sobald wir wissen, ob und wann der Artikel wieder verfügbar ist, informieren wir Sie an dieser Stelle.
Machine Learning Applied to Composite Materials (eBook, PDF)
- Format: PDF
- Merkliste
- Auf die Merkliste
- Bewerten Bewerten
- Teilen
- Produkt teilen
- Produkterinnerung
- Produkterinnerung
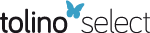
Bitte loggen Sie sich zunächst in Ihr Kundenkonto ein oder registrieren Sie sich bei
bücher.de, um das eBook-Abo tolino select nutzen zu können.
Hier können Sie sich einloggen
Hier können Sie sich einloggen
Sie sind bereits eingeloggt. Klicken Sie auf 2. tolino select Abo, um fortzufahren.
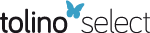
Bitte loggen Sie sich zunächst in Ihr Kundenkonto ein oder registrieren Sie sich bei bücher.de, um das eBook-Abo tolino select nutzen zu können.
This book introduces the approach of Machine Learning (ML) based predictive models in the design of composite materials to achieve the required properties for certain applications. ML can learn from existing experimental data obtained from very limited number of experiments and subsequently can be trained to find solutions of the complex non-linear, multi-dimensional functional relationships without any prior assumptions about their nature. In this case the ML models can learn from existing experimental data obtained from (1) composite design based on various properties of the matrix material…mehr
- Geräte: PC
- ohne Kopierschutz
- eBook Hilfe
- Größe: 8.78MB
- Upload möglich
This book introduces the approach of Machine Learning (ML) based predictive models in the design of composite materials to achieve the required properties for certain applications. ML can learn from existing experimental data obtained from very limited number of experiments and subsequently can be trained to find solutions of the complex non-linear, multi-dimensional functional relationships without any prior assumptions about their nature. In this case the ML models can learn from existing experimental data obtained from (1) composite design based on various properties of the matrix material and fillers/reinforcements (2) material processing during fabrication (3) property relationships. Modelling of these relationships using ML methods significantly reduce the experimental work involved in designing new composites, and therefore offer a new avenue for material design and properties. The book caters to students, academics and researchers who are interested in the field of materialcomposite modelling and design.
Produktdetails
- Produktdetails
- Verlag: Springer Nature Singapore
- Erscheinungstermin: 29. November 2022
- Englisch
- ISBN-13: 9789811962783
- Artikelnr.: 66763730
- Verlag: Springer Nature Singapore
- Erscheinungstermin: 29. November 2022
- Englisch
- ISBN-13: 9789811962783
- Artikelnr.: 66763730
Dr. Vinod Kushvaha earned his Dual Degree (B. Tech. + M. Tech.) from the Indian Institute of Technology Bombay (IIT Bombay) in Civil Engineering. Following that, he earned his second masters and a Ph.D. degree in Mechanical Engineering at Auburn University, USA. His diversified research background led him to publish in various engineering journals and in several international conferences. Dr. Kushvaha also actively reviews for Composite Science and Technology, Composites Part A, Composites Part B: Engineering, Materials Science and Engineering A, and Composite Structures journals, to name a few. Presently, Dr. Kushvaha is an Assistant Professor at the Indian Institute of Technology Jammu (IIT Jammu) in the Civil Engineering department, leading research in Materials and Structural Engineering.
Dr. Sanjay Mavinkere Rangappa, is currently working as a Senior Research Scientist/Associate Professor and also 'Advisor within the office of the President for University Promotion and Development towards International goals' at King Mongkut’s University of Technology North Bangkok, Bangkok, Thailand. He is a Life Member of Indian Society for Technical Education (ISTE) and an Associate Member of Institute of Engineers (India). Also acting as a Board Member of various international journals in the fields of materials science and composites. He is a reviewer for more than 120 international Journals, also a reviewer for book proposals, and international conferences. In addition, he has published more than 200 articles in high-quality international peer-reviewed journals indexed by SCI/Scopus, 11 editorial corners, 60 book chapters, one book, 25 books as an Editor (Published by lead publishers such as Elsevier, Springer, Taylor & Francis, Wiley), and also presented research papers at national/international conferences. He is a lead editor of Several special issues. In addition, 1 Thailand Patent and 2 Indian patents are granted.He has delivered keynote and invited talks at various international conferences and workshops. He has received a ‘Top Peer Reviewer 2019’ award, Global Peer Review Awards, Powered by Publons, Web of Science Group. The KMUTNB selected him for the ‘Outstanding Young Researcher’ Award 2020 and ‘Outstanding Researcher’ Award 2021. He is recognized by Stanford University’s list of the world’s Top 2% of the Most-Cited Scientists in Single Year Citation Impact 2019 and also for the year 2020.
Dr. Priyanka Madhushri is the Internet of Things (IoT) Ideation Research Engineer at Stanley Black and Decker (SBD), Atlanta. Dr. Madhushri earned her Ph.D. in Electrical Engineering from the University of Alabama in Huntsville, USA. She works with the innovation team and brings new ideas to various projects. As a researcher, she provides Proof of Concept (POC) to various SBD teams and assists in developing the company’s software, hardware, and data analytics. Her research interestsinclude predictive analyses using Machine Learning, material modeling, Internet of Things (IoT), mobile computing, etc. She has published in various engineering fields, including materials journals, where her work focused on utilizing machine learning algorithms to predict and explain the mechanical behavior of advanced engineering materials.
Prof. Dr. Suchart Siengchin is President of King Mongkut's University of Technology North Bangkok (KMUTNB), Thailand. He received his Dipl.-Ing. in Mechanical Engineering from University of Applied Sciences Giessen/Friedberg, Hessen, Germany, M.Sc. in Polymer Technology from University of Applied Sciences Aalen, Baden-Wuerttemberg, Germany, M.Sc. in Material Science at the Erlangen-Nürnberg University, Bayern, Germany, Doctor of Philosophy in Engineering (Dr.-Ing.) from Institute for Composite Materials, University of Kaiserslautern, Rheinland-Pfalz, Germany and Postdoctoral Research from School of Materials Engineering, Purdue University, USA. In 2016 he completed the Habilitation (Dr.-Ing. habil.) in Mechanical Engineering from Chemnitz University of Technology, Saxony, Germany and worked as a Lecturer for Mechanical and Process Engineering Department at The Sirindhorn International Thai-German Graduate School of Engineering (TGGS), KMUTNB. He has been full Professor at KMUTNB and became the Vice President for Research and Academic Enhancement in 2012 and elected President of KMUTNB in November 2016. He won the Outstanding Researcher Award in 2010, 2012, 2013 at KMUTNB and National Outstanding Researcher Award for the year 2021 in engineering and industrial research by National Research Council of Thailand (NRCT). His research interests in Polymer Processing and Composite Material. He is Editor-in-Chief: Applied Science and Engineering Progress and International Advisory Board, eXPRESS Polymer Letters and Journal of Production Systems and Manufacturing Science and the author of more than 350 peer reviewed Journal Articles. He also edited more than 30 books and published morethan 80 book chapters. He has participated with presentations in more than 49 International and National Conferences with respect to Materials Science and Engineering topics.
Dr. Sanjay Mavinkere Rangappa, is currently working as a Senior Research Scientist/Associate Professor and also 'Advisor within the office of the President for University Promotion and Development towards International goals' at King Mongkut’s University of Technology North Bangkok, Bangkok, Thailand. He is a Life Member of Indian Society for Technical Education (ISTE) and an Associate Member of Institute of Engineers (India). Also acting as a Board Member of various international journals in the fields of materials science and composites. He is a reviewer for more than 120 international Journals, also a reviewer for book proposals, and international conferences. In addition, he has published more than 200 articles in high-quality international peer-reviewed journals indexed by SCI/Scopus, 11 editorial corners, 60 book chapters, one book, 25 books as an Editor (Published by lead publishers such as Elsevier, Springer, Taylor & Francis, Wiley), and also presented research papers at national/international conferences. He is a lead editor of Several special issues. In addition, 1 Thailand Patent and 2 Indian patents are granted.He has delivered keynote and invited talks at various international conferences and workshops. He has received a ‘Top Peer Reviewer 2019’ award, Global Peer Review Awards, Powered by Publons, Web of Science Group. The KMUTNB selected him for the ‘Outstanding Young Researcher’ Award 2020 and ‘Outstanding Researcher’ Award 2021. He is recognized by Stanford University’s list of the world’s Top 2% of the Most-Cited Scientists in Single Year Citation Impact 2019 and also for the year 2020.
Dr. Priyanka Madhushri is the Internet of Things (IoT) Ideation Research Engineer at Stanley Black and Decker (SBD), Atlanta. Dr. Madhushri earned her Ph.D. in Electrical Engineering from the University of Alabama in Huntsville, USA. She works with the innovation team and brings new ideas to various projects. As a researcher, she provides Proof of Concept (POC) to various SBD teams and assists in developing the company’s software, hardware, and data analytics. Her research interestsinclude predictive analyses using Machine Learning, material modeling, Internet of Things (IoT), mobile computing, etc. She has published in various engineering fields, including materials journals, where her work focused on utilizing machine learning algorithms to predict and explain the mechanical behavior of advanced engineering materials.
Prof. Dr. Suchart Siengchin is President of King Mongkut's University of Technology North Bangkok (KMUTNB), Thailand. He received his Dipl.-Ing. in Mechanical Engineering from University of Applied Sciences Giessen/Friedberg, Hessen, Germany, M.Sc. in Polymer Technology from University of Applied Sciences Aalen, Baden-Wuerttemberg, Germany, M.Sc. in Material Science at the Erlangen-Nürnberg University, Bayern, Germany, Doctor of Philosophy in Engineering (Dr.-Ing.) from Institute for Composite Materials, University of Kaiserslautern, Rheinland-Pfalz, Germany and Postdoctoral Research from School of Materials Engineering, Purdue University, USA. In 2016 he completed the Habilitation (Dr.-Ing. habil.) in Mechanical Engineering from Chemnitz University of Technology, Saxony, Germany and worked as a Lecturer for Mechanical and Process Engineering Department at The Sirindhorn International Thai-German Graduate School of Engineering (TGGS), KMUTNB. He has been full Professor at KMUTNB and became the Vice President for Research and Academic Enhancement in 2012 and elected President of KMUTNB in November 2016. He won the Outstanding Researcher Award in 2010, 2012, 2013 at KMUTNB and National Outstanding Researcher Award for the year 2021 in engineering and industrial research by National Research Council of Thailand (NRCT). His research interests in Polymer Processing and Composite Material. He is Editor-in-Chief: Applied Science and Engineering Progress and International Advisory Board, eXPRESS Polymer Letters and Journal of Production Systems and Manufacturing Science and the author of more than 350 peer reviewed Journal Articles. He also edited more than 30 books and published morethan 80 book chapters. He has participated with presentations in more than 49 International and National Conferences with respect to Materials Science and Engineering topics.
Importance of machine learning in material science.- Machine Learning: A methodology to explain and predict material behavior.- Effect of aspect ratio on dynamic fracture toughness of particulate polymer composite using artificial neural network.- Methodology of K-Nearest Neighbor for predicting the fracture toughness of polymer composites.- Forward machine learning technique to predict dynamic fracture behavior of particulate composite.- Predictive modelling of fracture behavior in silica-filled polymer composite subjected to impact with varying loading rates.- Machine learning approach to determine the elastic modulus of Carbon fiber-reinforced laminates.- Effect of weight ratio on mechanical behaviour of natural fiber based biocomposite using machine learning.- Effect of natural fiber’s mechanical properties and fiber matrix adhesion strength to design biocomposite.- Comparison of various machine learning algorithms to predict material behavior in GFRP.
Importance of machine learning in material science.- Machine Learning: A methodology to explain and predict material behavior.- Effect of aspect ratio on dynamic fracture toughness of particulate polymer composite using artificial neural network.- Methodology of K-Nearest Neighbor for predicting the fracture toughness of polymer composites.- Forward machine learning technique to predict dynamic fracture behavior of particulate composite.- Predictive modelling of fracture behavior in silica-filled polymer composite subjected to impact with varying loading rates.- Machine learning approach to determine the elastic modulus of Carbon fiber-reinforced laminates.- Effect of weight ratio on mechanical behaviour of natural fiber based biocomposite using machine learning.- Effect of natural fiber's mechanical properties and fiber matrix adhesion strength to design biocomposite.- Comparison of various machine learning algorithms to predict material behavior in GFRP.
Importance of machine learning in material science.- Machine Learning: A methodology to explain and predict material behavior.- Effect of aspect ratio on dynamic fracture toughness of particulate polymer composite using artificial neural network.- Methodology of K-Nearest Neighbor for predicting the fracture toughness of polymer composites.- Forward machine learning technique to predict dynamic fracture behavior of particulate composite.- Predictive modelling of fracture behavior in silica-filled polymer composite subjected to impact with varying loading rates.- Machine learning approach to determine the elastic modulus of Carbon fiber-reinforced laminates.- Effect of weight ratio on mechanical behaviour of natural fiber based biocomposite using machine learning.- Effect of natural fiber’s mechanical properties and fiber matrix adhesion strength to design biocomposite.- Comparison of various machine learning algorithms to predict material behavior in GFRP.
Importance of machine learning in material science.- Machine Learning: A methodology to explain and predict material behavior.- Effect of aspect ratio on dynamic fracture toughness of particulate polymer composite using artificial neural network.- Methodology of K-Nearest Neighbor for predicting the fracture toughness of polymer composites.- Forward machine learning technique to predict dynamic fracture behavior of particulate composite.- Predictive modelling of fracture behavior in silica-filled polymer composite subjected to impact with varying loading rates.- Machine learning approach to determine the elastic modulus of Carbon fiber-reinforced laminates.- Effect of weight ratio on mechanical behaviour of natural fiber based biocomposite using machine learning.- Effect of natural fiber's mechanical properties and fiber matrix adhesion strength to design biocomposite.- Comparison of various machine learning algorithms to predict material behavior in GFRP.