Machine Learning for Asset Management (eBook, PDF)
New Developments and Financial Applications
Redaktion: Jurczenko, Emmanuel
Alle Infos zum eBook verschenken
Machine Learning for Asset Management (eBook, PDF)
New Developments and Financial Applications
Redaktion: Jurczenko, Emmanuel
- Format: PDF
- Merkliste
- Auf die Merkliste
- Bewerten Bewerten
- Teilen
- Produkt teilen
- Produkterinnerung
- Produkterinnerung
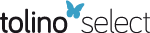
Hier können Sie sich einloggen
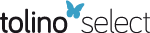
Bitte loggen Sie sich zunächst in Ihr Kundenkonto ein oder registrieren Sie sich bei bücher.de, um das eBook-Abo tolino select nutzen zu können.
This new edited volume consists of a collection of original articles written by leading financial economists and industry experts in the area of machine learning for asset management. The chapters introduce the reader to some of the latest research developments in the area of equity, multi-asset and factor investing. Each chapter deals with new methods for return and risk forecasting, stock selection, portfolio construction, performance attribution and transaction costs modeling. This volume will be of great help to portfolio managers, asset owners and consultants, as well as academics and…mehr
- Geräte: PC
- mit Kopierschutz
- eBook Hilfe
- Größe: 19.95MB
- Innovation Processes in Agro-Ecological Transitions in Developing Countries (eBook, PDF)139,99 €
- Joël-Thomas RavixInnovation and Industrial Policies (eBook, PDF)139,99 €
- Innovation Economics, Engineering and Management Handbook 2 (eBook, PDF)139,99 €
- Pierre MassotteSmart Decisions in Complex Systems (eBook, PDF)139,99 €
- Curt SteinhorstCan I Have Your Attention? (eBook, PDF)17,99 €
- Daniele ChauvelThe Innovative Company (eBook, PDF)139,99 €
- Michael G. LuchsDesign Thinking (eBook, PDF)50,99 €
-
-
-
Dieser Download kann aus rechtlichen Gründen nur mit Rechnungsadresse in A, B, BG, CY, CZ, D, DK, EW, E, FIN, F, GR, HR, H, IRL, I, LT, L, LR, M, NL, PL, P, R, S, SLO, SK ausgeliefert werden.
- Produktdetails
- Verlag: Jossey-Bass
- Seitenzahl: 460
- Erscheinungstermin: 16. Juli 2020
- Englisch
- ISBN-13: 9781119751168
- Artikelnr.: 59906896
- Verlag: Jossey-Bass
- Seitenzahl: 460
- Erscheinungstermin: 16. Juli 2020
- Englisch
- ISBN-13: 9781119751168
- Artikelnr.: 59906896
- Herstellerkennzeichnung Die Herstellerinformationen sind derzeit nicht verfügbar.
Acknowledgments xv
Chapter 1. Time-series and Cross-sectional Stock Return Forecasting: New
Machine Learning Methods 1
David E. RAPACH and Guofu ZHOU
1.1. Introduction 1
1.2. Time-series return forecasts 3
1.2.1. Predictive regression 3
1.2.2. Forecast combination 5
1.2.3. Elastic net 6
1.2.4. Combination elastic net 8
1.3. Empirical application 10
1.3.1. Data 10
1.3.2. Forecasts 12
1.3.3. Statistical gains 17
1.3.4. Economic gains 23
1.4. Cross-sectional return forecasts 26
1.5. Conclusion 29
1.6. Acknowledgements 30
1.7. References 30
Chapter 2. In Search of Return Predictability: Application of Machine
Learning Algorithms in Tactical Allocation 35
Kris BOUDT, Muzafer CELA and Majeed SIMAAN
2.1. Introduction 35
2.2. Empirical investigation 38
2.2.1. The data 38
2.2.2. Tactical asset allocation strategy 40
2.2.3. Implementation 41
2.2.4. Benchmarks 42
2.3. A review of machine learning algorithms for prediction of market
direction 42
2.3.1. K-nearest neighbors 43
2.3.2. Generalized linear model 44
2.3.3. Elastic net regression 44
2.3.4. Linear discriminant analysis 45
2.3.5. Support vector machines with radial kernel 45
2.3.6. C5.0 47
2.3.7. Random forests 48
2.3.8. Multilayer perceptron 48
2.3.9. Model averaging 49
2.3.10. Repeated k-fold cross validation 50
2.4. Evaluation criteria 51
2.4.1. Statistical performance 51
2.4.2. Financial performance 53
2.4.3. Significant features 54
2.5. Results and findings 54
2.5.1. Descriptive statistics of the data 55
2.5.2. Statistical performance 56
2.5.3. Financial performance 58
2.5.4. The best performer, benchmark and model average 67
2.5.5. LIME 68
2.6. Conclusion 70
2.7. Acknowledgments 70
2.8. References 70
Chapter 3. Sparse Predictive Regressions: Statistical Performance and
Economic Significance 75
Daniele BIANCHI and Andrea TAMONI
3.1. Introduction 75
3.2. Related literature 78
3.3. Data: portfolios and predictors 80
3.4. Econometric framework 84
3.4.1. Shrinkage priors 86
3.4.2. Forecast evaluations 92
3.5. Predicting asset returns: empirical results 93
3.5.1. Statistical performance 93
3.5.2. Economic significance 96
3.6. Discussion on the dynamics of sparsity 100
3.7. Conclusion 102
3.8. Appendix 103
3.9. Posterior simulation 103
3.9.1. Ridge regression 103
3.9.2. Lasso and group-lasso 103
3.9.3. Elastic net 105
3.9.4. Horseshoe and the group horseshoe 105
3.10. References 106
Chapter 4. The Artificial Intelligence Approach to Picking Stocks 115
Riccardo BORGHI and Giuliano DE ROSSI
4.1. Introduction 115
4.2. Literature review 120
4.3. Data 123
4.3.1. Equity factors 123
4.3.2. Data cleaning 125
4.3.3. Features used for training and prediction 125
4.4. Model specification and calibration 126
4.4.1. Models 126
4.4.2. Model calibration 133
4.5. Predicting US stock returns 135
4.5.1. Information coefficients 136
4.5.2. Long-short strategy 138
4.5.3. Returns correlation with Alpha model 140
4.5.4. Active returns by basket 141
4.5.5. Calibrated hyperparameters and model complexity 142
4.5.6. Variable importance 144
4.6. Predicting European stock returns 146
4.6.1. Information coefficients 146
4.6.2. Long-short strategy 147
4.6.3. Returns correlation with Alpha model 150
4.6.4. Active returns by basket 150
4.6.5. Calibrated hyperparameters and model complexity 151
4.6.6. Variable importance 152
4.7. The impact of transaction costs 154
4.7.1. Optimized strategies for European stocks 154
4.7.2. Optimized strategies for US stocks 158
4.8. Conclusion 161
4.9. References 163
Chapter 5. Enhancing Alpha Signals from Trade Ideas Data Using Supervised
Learning 167
Georgios V. PAPAIOANNOU and Daniel GIAMOURIDIS
5.1. Introduction 167
5.2. Data 169
5.3. Model and empirical design 174
5.4. Estimation and robustness 179
5.5. Economic significance 186
5.6. Conclusion 188
5.7. References 189
Chapter 6. Natural Language Process and Machine Learning in Global Stock
Selection 191
Yin LUO
6.1. Introduction 191
6.1.1. The performance of traditional stock selection factors continues to
shrink 191
6.1.2. Textual data, natural language processing and machine learning 195
6.2. Natural language analysis of company management presentations 197
6.2.1. Coverage 198
6.2.2. Readability index and language complexity 201
6.2.3. Quantifying executive personalities 206
6.2.4. Syntactic parser and part-of-speech (POS) tagging 207
6.3. Extracting long-term signal from news sentiment data 211
6.3.1. Introducing RavenPack data 211
6.3.2. The challenges of using news sentiment signals in stock selection
215
6.3.3. How do investors react to news? 216
6.3.4. The interaction of news, corporate events and investor behavior 217
6.3.5. A machine learning approach to extract event-based sentiment 221
6.3.6. Welcome to NICE (News with Insightful Categorical Events) 225
6.4. References 228
Chapter 7. Forecasting Beta Using Machine Learning and Equity Sentiment
Variables 231
Alexei JOUROVSKI, Vladyslav DUBIKOVSKYY, Pere ADELL, Ravi RAMAKRISHNAN and
Robert KOSOWSKI
7.1. Introduction 231
7.2. Data 234
7.2.1. Data construction process 234
7.3. Methodology 240
7.3.1. Historical beta 241
7.3.2. Bloomberg's adjusted beta 241
7.3.3. OLS regression 241
7.3.4. Post-LASSO OLS regression 241
7.3.5. Random forest model 242
7.3.6. XGBoost model 242
7.4. Empirical results 242
7.4.1. Variable selection 242
7.4.2. Forecasting models 244
7.4.3. Variable importance 246
7.4.4. SHAP values. 247
7.4.5. Overall level of feature importance 248
7.4.6. Cross-sectional analysis of feature importance 250
7.4.7. Time-series analysis of feature importance 253
7.5. Constructing market neutral long-short portfolios 257
7.6. Concluding remarks 258
7.7. References 259
Chapter 8. Machine Learning Optimization Algorithms & Portfolio Allocation
261
Sarah PERRIN and Thierry RONCALLI
8.1. Introduction 262
8.2. The quadratic programming world of portfolio optimization 264
8.2.1. Quadratic programming 264
8.2.2. Mean-variance optimized portfolios 265
8.2.3. Issues with QP optimization 270
8.3. Machine learning optimization algorithms 271
8.3.1. Coordinate descent 274
8.3.2. Alternating direction method of multipliers 279
8.3.3. Proximal operators 283
8.3.4. Dykstra's algorithm 289
8.4. Applications to portfolio optimization 293
8.4.1. Minimum variance optimization 295
8.4.2. Smart beta portfolios 301
8.4.3. Robo-advisory optimization307
8.4.4. Tips and tricks 312
8.5. Conclusion 315
8.6. Acknowledgements 317
8.7. Appendix 317
8.7.1. Mathematical results 317
8.7.2. Data 323
8.8. References 324
Chapter 9. Hierarchical Risk Parity: Accounting for Tail Dependencies in
Multi-asset Multi-factor Allocations 329
Harald LOHRE, Carsten ROTHER and Kilian Axel SCHÄFER
9.1. Hierarchical risk parity strategies 332
9.1.1. The multi-asset multi-factor universe 333
9.1.2. The hierarchical multi-asset multi-factor structure 334
9.1.3. Hierarchical clustering 338
9.1.4. Portfolio allocation based on hierarchical clustering 342
9.2. Tail dependency and hierarchical clustering 343
9.2.1. Tail dependence coefficients 344
9.2.2. Estimating tail dependence coefficients 345
9.3. Risk-based allocation strategies 347
9.3.1. Classic risk-based allocation techniques 347
9.3.2. Diversified risk parity 348
9.4. Hierarchical risk parity for multi-asset multi-factor allocations 352
9.4.1. Strategy universe 352
9.4.2. A statistical horse race of risk-based allocation strategies 354
9.5. Conclusion 360
9.6. Acknowledgements 362
9.7. Appendix 1: Definition of style factors 362
9.7.1. Foreign exchange (FX) style factors 362
9.7.2. Commodity style factors 363
9.7.3. Rates style factors 364
9.7.4. Equity style factors 364
9.8. Appendix 2: CSR estimator 365
9.9. References 367
Chapter 10. Portfolio Performance Attribution: A Machine Learning-Based
Approach 369
Ryan BROWN, Harindra DE SILVA and Patrick D. NEAL
10.1. Introduction 369
10.2. Methodology 371
10.2.1. Matrix algebra representation of selection and allocation effects
372
10.2.2. Creating categorical variables from continuous variables 374
10.2.3. Optimizing continuous variable breakpoints to maximize systematic
attribution 375
10.3. Data description 377
10.4. Results 378
10.5. Conclusion 385
10.6. References 386
Chapter 11. Modeling Transaction Costs When Trades May Be Crowded: A
Bayesian Network Using Partially Observable Orders Imbalance 387
Marie BRIÈRE, Charles-Albert LEHALLE, Tamara NEFEDOVA and Amine RABOUN
11.1. Introduction 388
11.2. Related literature 391
11.2.1. Transaction costs and market impact 391
11.2.2. Bayesian networks 392
11.3. ANcerno database 394
11.4. Transaction cost modeling 396
11.4.1. Order size 396
11.4.2. Order flow imbalance 398
11.4.3. Joint effect of order size and order flow imbalance 400
11.5. Bayesian network modeling with net order flow imbalance as latent
variable 403
11.5.1. Bayesian inference 404
11.5.2. Bayesian network modeling 406
11.5.3. Net order flow imbalance dependencies 409
11.5.4. Implementation shortfall dependencies 413
11.6. Forecasting implementation shortfall 415
11.6.1. Inference of investors' order flow imbalance given post-trade cost
and market conditions 420
11.7. Conclusion 421
11.8. Appendix A: Garman-Klass volatility definition 423
11.9. Appendix B: bid-ask spread and volatility distribution dependencies
423
11.10. Appendix C: beta distribution properties 424
11.11. Appendix D: net order flow imbalance properties 425
11.12. Appendix E: implementation shortfall distribution 425
11.13. Appendix F: Hastings-Metropolis algorithm 426
11.14. References 427
List of Authors 431
Index 435
Commendations 434
Acknowledgments xv
Chapter 1. Time-series and Cross-sectional Stock Return Forecasting: New
Machine Learning Methods 1
David E. RAPACH and Guofu ZHOU
1.1. Introduction 1
1.2. Time-series return forecasts 3
1.2.1. Predictive regression 3
1.2.2. Forecast combination 5
1.2.3. Elastic net 6
1.2.4. Combination elastic net 8
1.3. Empirical application 10
1.3.1. Data 10
1.3.2. Forecasts 12
1.3.3. Statistical gains 17
1.3.4. Economic gains 23
1.4. Cross-sectional return forecasts 26
1.5. Conclusion 29
1.6. Acknowledgements 30
1.7. References 30
Chapter 2. In Search of Return Predictability: Application of Machine
Learning Algorithms in Tactical Allocation 35
Kris BOUDT, Muzafer CELA and Majeed SIMAAN
2.1. Introduction 35
2.2. Empirical investigation 38
2.2.1. The data 38
2.2.2. Tactical asset allocation strategy 40
2.2.3. Implementation 41
2.2.4. Benchmarks 42
2.3. A review of machine learning algorithms for prediction of market
direction 42
2.3.1. K-nearest neighbors 43
2.3.2. Generalized linear model 44
2.3.3. Elastic net regression 44
2.3.4. Linear discriminant analysis 45
2.3.5. Support vector machines with radial kernel 45
2.3.6. C5.0 47
2.3.7. Random forests 48
2.3.8. Multilayer perceptron 48
2.3.9. Model averaging 49
2.3.10. Repeated k-fold cross validation 50
2.4. Evaluation criteria 51
2.4.1. Statistical performance 51
2.4.2. Financial performance 53
2.4.3. Significant features 54
2.5. Results and findings 54
2.5.1. Descriptive statistics of the data 55
2.5.2. Statistical performance 56
2.5.3. Financial performance 58
2.5.4. The best performer, benchmark and model average 67
2.5.5. LIME 68
2.6. Conclusion 70
2.7. Acknowledgments 70
2.8. References 70
Chapter 3. Sparse Predictive Regressions: Statistical Performance and
Economic Significance 75
Daniele BIANCHI and Andrea TAMONI
3.1. Introduction 75
3.2. Related literature 78
3.3. Data: portfolios and predictors 80
3.4. Econometric framework 84
3.4.1. Shrinkage priors 86
3.4.2. Forecast evaluations 92
3.5. Predicting asset returns: empirical results 93
3.5.1. Statistical performance 93
3.5.2. Economic significance 96
3.6. Discussion on the dynamics of sparsity 100
3.7. Conclusion 102
3.8. Appendix 103
3.9. Posterior simulation 103
3.9.1. Ridge regression 103
3.9.2. Lasso and group-lasso 103
3.9.3. Elastic net 105
3.9.4. Horseshoe and the group horseshoe 105
3.10. References 106
Chapter 4. The Artificial Intelligence Approach to Picking Stocks 115
Riccardo BORGHI and Giuliano DE ROSSI
4.1. Introduction 115
4.2. Literature review 120
4.3. Data 123
4.3.1. Equity factors 123
4.3.2. Data cleaning 125
4.3.3. Features used for training and prediction 125
4.4. Model specification and calibration 126
4.4.1. Models 126
4.4.2. Model calibration 133
4.5. Predicting US stock returns 135
4.5.1. Information coefficients 136
4.5.2. Long-short strategy 138
4.5.3. Returns correlation with Alpha model 140
4.5.4. Active returns by basket 141
4.5.5. Calibrated hyperparameters and model complexity 142
4.5.6. Variable importance 144
4.6. Predicting European stock returns 146
4.6.1. Information coefficients 146
4.6.2. Long-short strategy 147
4.6.3. Returns correlation with Alpha model 150
4.6.4. Active returns by basket 150
4.6.5. Calibrated hyperparameters and model complexity 151
4.6.6. Variable importance 152
4.7. The impact of transaction costs 154
4.7.1. Optimized strategies for European stocks 154
4.7.2. Optimized strategies for US stocks 158
4.8. Conclusion 161
4.9. References 163
Chapter 5. Enhancing Alpha Signals from Trade Ideas Data Using Supervised
Learning 167
Georgios V. PAPAIOANNOU and Daniel GIAMOURIDIS
5.1. Introduction 167
5.2. Data 169
5.3. Model and empirical design 174
5.4. Estimation and robustness 179
5.5. Economic significance 186
5.6. Conclusion 188
5.7. References 189
Chapter 6. Natural Language Process and Machine Learning in Global Stock
Selection 191
Yin LUO
6.1. Introduction 191
6.1.1. The performance of traditional stock selection factors continues to
shrink 191
6.1.2. Textual data, natural language processing and machine learning 195
6.2. Natural language analysis of company management presentations 197
6.2.1. Coverage 198
6.2.2. Readability index and language complexity 201
6.2.3. Quantifying executive personalities 206
6.2.4. Syntactic parser and part-of-speech (POS) tagging 207
6.3. Extracting long-term signal from news sentiment data 211
6.3.1. Introducing RavenPack data 211
6.3.2. The challenges of using news sentiment signals in stock selection
215
6.3.3. How do investors react to news? 216
6.3.4. The interaction of news, corporate events and investor behavior 217
6.3.5. A machine learning approach to extract event-based sentiment 221
6.3.6. Welcome to NICE (News with Insightful Categorical Events) 225
6.4. References 228
Chapter 7. Forecasting Beta Using Machine Learning and Equity Sentiment
Variables 231
Alexei JOUROVSKI, Vladyslav DUBIKOVSKYY, Pere ADELL, Ravi RAMAKRISHNAN and
Robert KOSOWSKI
7.1. Introduction 231
7.2. Data 234
7.2.1. Data construction process 234
7.3. Methodology 240
7.3.1. Historical beta 241
7.3.2. Bloomberg's adjusted beta 241
7.3.3. OLS regression 241
7.3.4. Post-LASSO OLS regression 241
7.3.5. Random forest model 242
7.3.6. XGBoost model 242
7.4. Empirical results 242
7.4.1. Variable selection 242
7.4.2. Forecasting models 244
7.4.3. Variable importance 246
7.4.4. SHAP values. 247
7.4.5. Overall level of feature importance 248
7.4.6. Cross-sectional analysis of feature importance 250
7.4.7. Time-series analysis of feature importance 253
7.5. Constructing market neutral long-short portfolios 257
7.6. Concluding remarks 258
7.7. References 259
Chapter 8. Machine Learning Optimization Algorithms & Portfolio Allocation
261
Sarah PERRIN and Thierry RONCALLI
8.1. Introduction 262
8.2. The quadratic programming world of portfolio optimization 264
8.2.1. Quadratic programming 264
8.2.2. Mean-variance optimized portfolios 265
8.2.3. Issues with QP optimization 270
8.3. Machine learning optimization algorithms 271
8.3.1. Coordinate descent 274
8.3.2. Alternating direction method of multipliers 279
8.3.3. Proximal operators 283
8.3.4. Dykstra's algorithm 289
8.4. Applications to portfolio optimization 293
8.4.1. Minimum variance optimization 295
8.4.2. Smart beta portfolios 301
8.4.3. Robo-advisory optimization307
8.4.4. Tips and tricks 312
8.5. Conclusion 315
8.6. Acknowledgements 317
8.7. Appendix 317
8.7.1. Mathematical results 317
8.7.2. Data 323
8.8. References 324
Chapter 9. Hierarchical Risk Parity: Accounting for Tail Dependencies in
Multi-asset Multi-factor Allocations 329
Harald LOHRE, Carsten ROTHER and Kilian Axel SCHÄFER
9.1. Hierarchical risk parity strategies 332
9.1.1. The multi-asset multi-factor universe 333
9.1.2. The hierarchical multi-asset multi-factor structure 334
9.1.3. Hierarchical clustering 338
9.1.4. Portfolio allocation based on hierarchical clustering 342
9.2. Tail dependency and hierarchical clustering 343
9.2.1. Tail dependence coefficients 344
9.2.2. Estimating tail dependence coefficients 345
9.3. Risk-based allocation strategies 347
9.3.1. Classic risk-based allocation techniques 347
9.3.2. Diversified risk parity 348
9.4. Hierarchical risk parity for multi-asset multi-factor allocations 352
9.4.1. Strategy universe 352
9.4.2. A statistical horse race of risk-based allocation strategies 354
9.5. Conclusion 360
9.6. Acknowledgements 362
9.7. Appendix 1: Definition of style factors 362
9.7.1. Foreign exchange (FX) style factors 362
9.7.2. Commodity style factors 363
9.7.3. Rates style factors 364
9.7.4. Equity style factors 364
9.8. Appendix 2: CSR estimator 365
9.9. References 367
Chapter 10. Portfolio Performance Attribution: A Machine Learning-Based
Approach 369
Ryan BROWN, Harindra DE SILVA and Patrick D. NEAL
10.1. Introduction 369
10.2. Methodology 371
10.2.1. Matrix algebra representation of selection and allocation effects
372
10.2.2. Creating categorical variables from continuous variables 374
10.2.3. Optimizing continuous variable breakpoints to maximize systematic
attribution 375
10.3. Data description 377
10.4. Results 378
10.5. Conclusion 385
10.6. References 386
Chapter 11. Modeling Transaction Costs When Trades May Be Crowded: A
Bayesian Network Using Partially Observable Orders Imbalance 387
Marie BRIÈRE, Charles-Albert LEHALLE, Tamara NEFEDOVA and Amine RABOUN
11.1. Introduction 388
11.2. Related literature 391
11.2.1. Transaction costs and market impact 391
11.2.2. Bayesian networks 392
11.3. ANcerno database 394
11.4. Transaction cost modeling 396
11.4.1. Order size 396
11.4.2. Order flow imbalance 398
11.4.3. Joint effect of order size and order flow imbalance 400
11.5. Bayesian network modeling with net order flow imbalance as latent
variable 403
11.5.1. Bayesian inference 404
11.5.2. Bayesian network modeling 406
11.5.3. Net order flow imbalance dependencies 409
11.5.4. Implementation shortfall dependencies 413
11.6. Forecasting implementation shortfall 415
11.6.1. Inference of investors' order flow imbalance given post-trade cost
and market conditions 420
11.7. Conclusion 421
11.8. Appendix A: Garman-Klass volatility definition 423
11.9. Appendix B: bid-ask spread and volatility distribution dependencies
423
11.10. Appendix C: beta distribution properties 424
11.11. Appendix D: net order flow imbalance properties 425
11.12. Appendix E: implementation shortfall distribution 425
11.13. Appendix F: Hastings-Metropolis algorithm 426
11.14. References 427
List of Authors 431
Index 435
Commendations 434