Shibiao Wan, Man-Wai Mak
Machine Learning for Protein Subcellular Localization Prediction (eBook, PDF)
63,95 €
63,95 €
inkl. MwSt.
Sofort per Download lieferbar
32 °P sammeln
63,95 €
Als Download kaufen
63,95 €
inkl. MwSt.
Sofort per Download lieferbar
32 °P sammeln
Jetzt verschenken
Alle Infos zum eBook verschenken
63,95 €
inkl. MwSt.
Sofort per Download lieferbar
Alle Infos zum eBook verschenken
32 °P sammeln
Shibiao Wan, Man-Wai Mak
Machine Learning for Protein Subcellular Localization Prediction (eBook, PDF)
- Format: PDF
- Merkliste
- Auf die Merkliste
- Bewerten Bewerten
- Teilen
- Produkt teilen
- Produkterinnerung
- Produkterinnerung
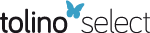
Bitte loggen Sie sich zunächst in Ihr Kundenkonto ein oder registrieren Sie sich bei
bücher.de, um das eBook-Abo tolino select nutzen zu können.
Hier können Sie sich einloggen
Hier können Sie sich einloggen
Sie sind bereits eingeloggt. Klicken Sie auf 2. tolino select Abo, um fortzufahren.
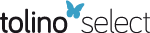
Bitte loggen Sie sich zunächst in Ihr Kundenkonto ein oder registrieren Sie sich bei bücher.de, um das eBook-Abo tolino select nutzen zu können.
Comprehensively covers protein subcellular localization from single-label prediction to multi-label prediction, and includes prediction strategies for virus, plant, and eukaryote species. Three machine learning tools are introduced to improve classification refinement, feature extraction, and dimensionality reduction.
- Geräte: PC
- mit Kopierschutz
- eBook Hilfe
Andere Kunden interessierten sich auch für
- Shibiao WanMachine Learning for Protein Subcellular Localization Prediction (eBook, ePUB)63,95 €
- Qingtang SuColor Image Watermarking (eBook, PDF)91,95 €
- Hans-Gerd LipinskiEinführung in die medizintechnische Informatik (eBook, PDF)109,95 €
- M S BeckProcess Tomography (eBook, PDF)40,95 €
- Kristen KehrerMachine Learning Upgrade: A Data Scientist's Guide to MLOps, LLMs, and ML Infrastructure (eBook, PDF)25,99 €
- Systems Performance Modeling (eBook, PDF)105,95 €
- Eric TopolDeep Medicine (eBook, PDF)25,99 €
-
-
-
Comprehensively covers protein subcellular localization from single-label prediction to multi-label prediction, and includes prediction strategies for virus, plant, and eukaryote species. Three machine learning tools are introduced to improve classification refinement, feature extraction, and dimensionality reduction.
Dieser Download kann aus rechtlichen Gründen nur mit Rechnungsadresse in A, B, BG, CY, CZ, D, DK, EW, E, FIN, F, GR, HR, H, IRL, I, LT, L, LR, M, NL, PL, P, R, S, SLO, SK ausgeliefert werden.
Produktdetails
- Produktdetails
- Verlag: De Gruyter
- Seitenzahl: 209
- Erscheinungstermin: 19. Mai 2015
- Englisch
- ISBN-13: 9781501501500
- Artikelnr.: 43450736
- Verlag: De Gruyter
- Seitenzahl: 209
- Erscheinungstermin: 19. Mai 2015
- Englisch
- ISBN-13: 9781501501500
- Artikelnr.: 43450736
- Herstellerkennzeichnung Die Herstellerinformationen sind derzeit nicht verfügbar.
Shibiao Wan, Man-Wai Mak, Hong Kong Polytechnic University, Hong Kong.
1 Introduction
1.1 Proteins and Their Subcellular Locations
1.2 Why Computationally Predicting Protein Subcellular Localization?
1.3 Organization of The Thesis
2 Literature Review
2.1 Sequence-Based Methods
2.2 Knowledge-Based Methods
2.3 Limitations of Existing Methods
3 Legitimacy of Using Gene Ontology Information
3.1 Direct Table Lookup?
3.2 Only Using Cellular Component GO Terms?
3.3 Equivalent to Homologous Transfer?
3.4 More Reasons for Using GO Information
4 Single-Location Protein Subcellular Localization
4.1 GOASVM: Extracting GO from Gene Ontology Annotation Database
4.2 FusionSVM: Fusion of Gene Ontology and Homology-Based Features
4.3 Summary
5 From Single-Location to Multi-Location
5.1 Significance of Multi-Location Proteins
5.2 Multi-Label Classification
5.3 mGOASVM: A Predictor for Both Single- and Multi-Location Proteins
5.4 AD-SVM: An Adaptive-decision Multi-Label Predictor
5.5 mPLR-Loc: A Multi-Label Predictor Based on Penalized Logistic- Regression
5.6 Summary
6 Mining Deeper on GO for Protein Subcellular Localization
6.1 Related Work
6.2 SS-Loc: Using Semantic Similarity Over GO
6.3 HybridGO-Loc: Hybridizing GO Frequency and Semantic Similarity
Features
6.4 Summary
7 Ensemble Random Projection for Large-Scale Predictions
7.1 Related Work
7.2 RP-SVM: A Multi-Label Classifier with Ensemble Random Projection
7.3 R3P-Loc: A Predictor Based on Ridge Regression and Random
Projection
7.4 Summary
8 Experimental Setup
8.1 Prediction of Single-Label Proteins
8.2 Prediction of Multi-Label Proteins
8.3 Statistical Evaluation Methods
8.4 Summary
9 Results and Analysis
9.1 Performance of GOASVM
9.2 Performance of FusionSVM
9.3 Performance of mGOASVM
9.4 Performance of AD-SVM
9.5 Performance of mPLR-Loc
9.6 Performance of SS-Loc
9.7 Performance of HybridGO-Loc
9.8 Performance of Performance of RP-SVM
9.9 Performance of R3P-Loc
9.10 Comprehensive Comparison of Proposed Predictors
9.11 Summary
10 Discussions
10.1 Analysis of Single-label Predictors
10.2 Advantages of mGOASVM
10.3 Analysis for HybridGO-Loc
10.4 Analysis for RP-SVM
10.5 Comparing the Proposed Multi-Label Predictors
10.6 Summary
11 Conclusions
A Web-Servers for Protein Subcellular Localization
B Proof of No Bias in LOOCV
Bibliography
1.1 Proteins and Their Subcellular Locations
1.2 Why Computationally Predicting Protein Subcellular Localization?
1.3 Organization of The Thesis
2 Literature Review
2.1 Sequence-Based Methods
2.2 Knowledge-Based Methods
2.3 Limitations of Existing Methods
3 Legitimacy of Using Gene Ontology Information
3.1 Direct Table Lookup?
3.2 Only Using Cellular Component GO Terms?
3.3 Equivalent to Homologous Transfer?
3.4 More Reasons for Using GO Information
4 Single-Location Protein Subcellular Localization
4.1 GOASVM: Extracting GO from Gene Ontology Annotation Database
4.2 FusionSVM: Fusion of Gene Ontology and Homology-Based Features
4.3 Summary
5 From Single-Location to Multi-Location
5.1 Significance of Multi-Location Proteins
5.2 Multi-Label Classification
5.3 mGOASVM: A Predictor for Both Single- and Multi-Location Proteins
5.4 AD-SVM: An Adaptive-decision Multi-Label Predictor
5.5 mPLR-Loc: A Multi-Label Predictor Based on Penalized Logistic- Regression
5.6 Summary
6 Mining Deeper on GO for Protein Subcellular Localization
6.1 Related Work
6.2 SS-Loc: Using Semantic Similarity Over GO
6.3 HybridGO-Loc: Hybridizing GO Frequency and Semantic Similarity
Features
6.4 Summary
7 Ensemble Random Projection for Large-Scale Predictions
7.1 Related Work
7.2 RP-SVM: A Multi-Label Classifier with Ensemble Random Projection
7.3 R3P-Loc: A Predictor Based on Ridge Regression and Random
Projection
7.4 Summary
8 Experimental Setup
8.1 Prediction of Single-Label Proteins
8.2 Prediction of Multi-Label Proteins
8.3 Statistical Evaluation Methods
8.4 Summary
9 Results and Analysis
9.1 Performance of GOASVM
9.2 Performance of FusionSVM
9.3 Performance of mGOASVM
9.4 Performance of AD-SVM
9.5 Performance of mPLR-Loc
9.6 Performance of SS-Loc
9.7 Performance of HybridGO-Loc
9.8 Performance of Performance of RP-SVM
9.9 Performance of R3P-Loc
9.10 Comprehensive Comparison of Proposed Predictors
9.11 Summary
10 Discussions
10.1 Analysis of Single-label Predictors
10.2 Advantages of mGOASVM
10.3 Analysis for HybridGO-Loc
10.4 Analysis for RP-SVM
10.5 Comparing the Proposed Multi-Label Predictors
10.6 Summary
11 Conclusions
A Web-Servers for Protein Subcellular Localization
B Proof of No Bias in LOOCV
Bibliography
1 Introduction
1.1 Proteins and Their Subcellular Locations
1.2 Why Computationally Predicting Protein Subcellular Localization?
1.3 Organization of The Thesis
2 Literature Review
2.1 Sequence-Based Methods
2.2 Knowledge-Based Methods
2.3 Limitations of Existing Methods
3 Legitimacy of Using Gene Ontology Information
3.1 Direct Table Lookup?
3.2 Only Using Cellular Component GO Terms?
3.3 Equivalent to Homologous Transfer?
3.4 More Reasons for Using GO Information
4 Single-Location Protein Subcellular Localization
4.1 GOASVM: Extracting GO from Gene Ontology Annotation Database
4.2 FusionSVM: Fusion of Gene Ontology and Homology-Based Features
4.3 Summary
5 From Single-Location to Multi-Location
5.1 Significance of Multi-Location Proteins
5.2 Multi-Label Classification
5.3 mGOASVM: A Predictor for Both Single- and Multi-Location Proteins
5.4 AD-SVM: An Adaptive-decision Multi-Label Predictor
5.5 mPLR-Loc: A Multi-Label Predictor Based on Penalized Logistic- Regression
5.6 Summary
6 Mining Deeper on GO for Protein Subcellular Localization
6.1 Related Work
6.2 SS-Loc: Using Semantic Similarity Over GO
6.3 HybridGO-Loc: Hybridizing GO Frequency and Semantic Similarity
Features
6.4 Summary
7 Ensemble Random Projection for Large-Scale Predictions
7.1 Related Work
7.2 RP-SVM: A Multi-Label Classifier with Ensemble Random Projection
7.3 R3P-Loc: A Predictor Based on Ridge Regression and Random
Projection
7.4 Summary
8 Experimental Setup
8.1 Prediction of Single-Label Proteins
8.2 Prediction of Multi-Label Proteins
8.3 Statistical Evaluation Methods
8.4 Summary
9 Results and Analysis
9.1 Performance of GOASVM
9.2 Performance of FusionSVM
9.3 Performance of mGOASVM
9.4 Performance of AD-SVM
9.5 Performance of mPLR-Loc
9.6 Performance of SS-Loc
9.7 Performance of HybridGO-Loc
9.8 Performance of Performance of RP-SVM
9.9 Performance of R3P-Loc
9.10 Comprehensive Comparison of Proposed Predictors
9.11 Summary
10 Discussions
10.1 Analysis of Single-label Predictors
10.2 Advantages of mGOASVM
10.3 Analysis for HybridGO-Loc
10.4 Analysis for RP-SVM
10.5 Comparing the Proposed Multi-Label Predictors
10.6 Summary
11 Conclusions
A Web-Servers for Protein Subcellular Localization
B Proof of No Bias in LOOCV
Bibliography
1.1 Proteins and Their Subcellular Locations
1.2 Why Computationally Predicting Protein Subcellular Localization?
1.3 Organization of The Thesis
2 Literature Review
2.1 Sequence-Based Methods
2.2 Knowledge-Based Methods
2.3 Limitations of Existing Methods
3 Legitimacy of Using Gene Ontology Information
3.1 Direct Table Lookup?
3.2 Only Using Cellular Component GO Terms?
3.3 Equivalent to Homologous Transfer?
3.4 More Reasons for Using GO Information
4 Single-Location Protein Subcellular Localization
4.1 GOASVM: Extracting GO from Gene Ontology Annotation Database
4.2 FusionSVM: Fusion of Gene Ontology and Homology-Based Features
4.3 Summary
5 From Single-Location to Multi-Location
5.1 Significance of Multi-Location Proteins
5.2 Multi-Label Classification
5.3 mGOASVM: A Predictor for Both Single- and Multi-Location Proteins
5.4 AD-SVM: An Adaptive-decision Multi-Label Predictor
5.5 mPLR-Loc: A Multi-Label Predictor Based on Penalized Logistic- Regression
5.6 Summary
6 Mining Deeper on GO for Protein Subcellular Localization
6.1 Related Work
6.2 SS-Loc: Using Semantic Similarity Over GO
6.3 HybridGO-Loc: Hybridizing GO Frequency and Semantic Similarity
Features
6.4 Summary
7 Ensemble Random Projection for Large-Scale Predictions
7.1 Related Work
7.2 RP-SVM: A Multi-Label Classifier with Ensemble Random Projection
7.3 R3P-Loc: A Predictor Based on Ridge Regression and Random
Projection
7.4 Summary
8 Experimental Setup
8.1 Prediction of Single-Label Proteins
8.2 Prediction of Multi-Label Proteins
8.3 Statistical Evaluation Methods
8.4 Summary
9 Results and Analysis
9.1 Performance of GOASVM
9.2 Performance of FusionSVM
9.3 Performance of mGOASVM
9.4 Performance of AD-SVM
9.5 Performance of mPLR-Loc
9.6 Performance of SS-Loc
9.7 Performance of HybridGO-Loc
9.8 Performance of Performance of RP-SVM
9.9 Performance of R3P-Loc
9.10 Comprehensive Comparison of Proposed Predictors
9.11 Summary
10 Discussions
10.1 Analysis of Single-label Predictors
10.2 Advantages of mGOASVM
10.3 Analysis for HybridGO-Loc
10.4 Analysis for RP-SVM
10.5 Comparing the Proposed Multi-Label Predictors
10.6 Summary
11 Conclusions
A Web-Servers for Protein Subcellular Localization
B Proof of No Bias in LOOCV
Bibliography