Machine Learning in Medical Imaging (eBook, PDF)
15th International Workshop, MLMI 2024, Held in Conjunction with MICCAI 2024, Marrakesh, Morocco, October 6, 2024, Proceedings, Part II
Redaktion: Xu, Xuanang; Sun, Kaicong; Ouyang, Xi; Rekik, Islem; Cui, Zhiming
Alle Infos zum eBook verschenken
Machine Learning in Medical Imaging (eBook, PDF)
15th International Workshop, MLMI 2024, Held in Conjunction with MICCAI 2024, Marrakesh, Morocco, October 6, 2024, Proceedings, Part II
Redaktion: Xu, Xuanang; Sun, Kaicong; Ouyang, Xi; Rekik, Islem; Cui, Zhiming
- Format: PDF
- Merkliste
- Auf die Merkliste
- Bewerten Bewerten
- Teilen
- Produkt teilen
- Produkterinnerung
- Produkterinnerung
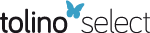
Hier können Sie sich einloggen
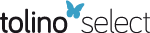
Bitte loggen Sie sich zunächst in Ihr Kundenkonto ein oder registrieren Sie sich bei bücher.de, um das eBook-Abo tolino select nutzen zu können.
This book constitutes the proceedings of the 15th International Workshop on Machine Learning in Medical Imaging, MLMI 2024, held in conjunction with MICCAI 2024, Marrakesh, Morocco, on October 6, 2024.
The 63 full papers presented in this volume were carefully reviewed and selected from 100 submissions. They focus on major trends and challenges in the above-mentioned area, aiming to identify new-cutting-edge techniques and their uses in medical imaging using artificial intelligence (AI) and machine learning (ML).
- Geräte: PC
- ohne Kopierschutz
- eBook Hilfe
- Größe: 42.64MB
- Machine Learning in Medical Imaging (eBook, PDF)60,95 €
- Machine Learning in Medical Imaging (eBook, PDF)65,95 €
- Machine Learning in Medical Imaging (eBook, PDF)59,95 €
- Machine Learning in Medical Imaging (eBook, PDF)59,95 €
- Medical Image Understanding and Analysis (eBook, PDF)40,95 €
- Machine Learning in Medical Imaging (eBook, PDF)73,95 €
- Machine Learning for Medical Image Reconstruction (eBook, PDF)40,95 €
-
-
-
The 63 full papers presented in this volume were carefully reviewed and selected from 100 submissions. They focus on major trends and challenges in the above-mentioned area, aiming to identify new-cutting-edge techniques and their uses in medical imaging using artificial intelligence (AI) and machine learning (ML).
Dieser Download kann aus rechtlichen Gründen nur mit Rechnungsadresse in A, B, BG, CY, CZ, D, DK, EW, E, FIN, F, GR, HR, H, IRL, I, LT, L, LR, M, NL, PL, P, R, S, SLO, SK ausgeliefert werden.
- Produktdetails
- Verlag: Springer International Publishing
- Seitenzahl: 247
- Erscheinungstermin: 22. Oktober 2024
- Englisch
- ISBN-13: 9783031732904
- Artikelnr.: 72242210
- Verlag: Springer International Publishing
- Seitenzahl: 247
- Erscheinungstermin: 22. Oktober 2024
- Englisch
- ISBN-13: 9783031732904
- Artikelnr.: 72242210
- Herstellerkennzeichnung Die Herstellerinformationen sind derzeit nicht verfügbar.