Matthew Betti
Mathematics and Statistics for the Quantitative Sciences (eBook, ePUB)
52,95 €
52,95 €
inkl. MwSt.
Sofort per Download lieferbar
26 °P sammeln
52,95 €
Als Download kaufen
52,95 €
inkl. MwSt.
Sofort per Download lieferbar
26 °P sammeln
Jetzt verschenken
Alle Infos zum eBook verschenken
52,95 €
inkl. MwSt.
Sofort per Download lieferbar
Alle Infos zum eBook verschenken
26 °P sammeln
Matthew Betti
Mathematics and Statistics for the Quantitative Sciences (eBook, ePUB)
- Format: ePub
- Merkliste
- Auf die Merkliste
- Bewerten Bewerten
- Teilen
- Produkt teilen
- Produkterinnerung
- Produkterinnerung
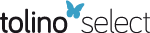
Bitte loggen Sie sich zunächst in Ihr Kundenkonto ein oder registrieren Sie sich bei
bücher.de, um das eBook-Abo tolino select nutzen zu können.
Hier können Sie sich einloggen
Hier können Sie sich einloggen
Sie sind bereits eingeloggt. Klicken Sie auf 2. tolino select Abo, um fortzufahren.
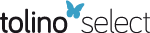
Bitte loggen Sie sich zunächst in Ihr Kundenkonto ein oder registrieren Sie sich bei bücher.de, um das eBook-Abo tolino select nutzen zu können.
This book serves as a foundational text in everyday mathematics in a way that is both engaging and practically useful. The book seeks to teach the mathematics needed to start to answer fundamental questions like 'why' or 'how'.
- Geräte: eReader
- ohne Kopierschutz
- eBook Hilfe
- Größe: 10.62MB
Andere Kunden interessierten sich auch für
- Thomas PfaffR For College Mathematics and Statistics (eBook, ePUB)46,95 €
- Cinzia BisiMathematical Methods for Life Sciences (eBook, ePUB)54,95 €
- Elisa AlosMalliavin Calculus in Finance (eBook, ePUB)48,95 €
- Gary ChartrandGraphs & Digraphs (eBook, ePUB)57,95 €
- Alexandr DraganovTaking the "Oof!" Out of Proofs (eBook, ePUB)48,95 €
- Robert JarrowModeling Fixed Income Securities and Interest Rate Options (eBook, ePUB)46,95 €
- Samuel DittmerPuzzle and Proof (eBook, ePUB)31,95 €
-
-
-
This book serves as a foundational text in everyday mathematics in a way that is both engaging and practically useful. The book seeks to teach the mathematics needed to start to answer fundamental questions like 'why' or 'how'.
Dieser Download kann aus rechtlichen Gründen nur mit Rechnungsadresse in A, B, BG, CY, CZ, D, DK, EW, E, FIN, F, GR, HR, H, IRL, I, LT, L, LR, M, NL, PL, P, R, S, SLO, SK ausgeliefert werden.
Produktdetails
- Produktdetails
- Verlag: Taylor & Francis
- Seitenzahl: 472
- Erscheinungstermin: 12. Dezember 2022
- Englisch
- ISBN-13: 9781000806069
- Artikelnr.: 66224870
- Verlag: Taylor & Francis
- Seitenzahl: 472
- Erscheinungstermin: 12. Dezember 2022
- Englisch
- ISBN-13: 9781000806069
- Artikelnr.: 66224870
- Herstellerkennzeichnung Die Herstellerinformationen sind derzeit nicht verfügbar.
Matthew Betti is an applied mathematician focusing on mathematical modeling of ecological and evolutionary problems, and disease spread. He is currently situated in Sackville, NB at Mount Allison University where he has developed and taught most first year courses in mathematics and computer science. Betti focuses on blending the rigourous with the intuitive to renew interesting in mathematics and statistics in students who see the subjects as a hurdle. Betti also incorporates discussions of ethics and social problems and the place of the mathematical sciences in this context.
Betti's research on disease spread and the ecology of honey bees has been published in numerous international journals. His approachable synthesis of complex material has been recognized by a number of presentation awards, and consultation work with governments at all levels.
Betti's research on disease spread and the ecology of honey bees has been published in numerous international journals. His approachable synthesis of complex material has been recognized by a number of presentation awards, and consultation work with governments at all levels.
Section I. Applied Mathematics. The Plot (so you don't lose it). 1.
Functions. 1.1. Anatomy of a Function. 1.2. Modeling With Mathematics. 1.3.
Constants and Linear Functions. 1.4. Polynomials. 1.5. Exponentials And
Logarithms. 1.6. Functions in Higher Dimensions. 1.7. Contour Diagrams.
1.8. Models In Two Dimensions. 1.9. Variables vs Parameters. 2.
Derivatives. 2.1. The Tangent Line. 2.2. Approximating Derivatives of
Functions. 2.3. Limits. 2.4. Limits And Derivates. 2.5. Derivative
Formulas. 2.6. The Product Rule. 2.7. The Chain Rule. 2.8. Mixing Rules.
2.9. Critical Values. 2.10. Constrained Optimization. 2.11. Elasticity.
2.12. Partial Derivatives. 3. Linear Algebra. 3.1. Vectors. 3.2. Matrices.
3.3. Multiplication: Numbers and Matrices. 3.4. Multiplication: Matrix and
Vectors. 3.5. Multiplication: Matrix and Matrix. 3.6. Leslie Matrices. 3.7.
The Determinant. 3.8. Eigenvalues & Eigenvectors. 4. Derivatives in
Multiple Dimensions. 4.1. Applications. 4.2. Distribution Fitting,
Probability and Likelihood. 5. Differential Equations. 5.1. Solving Basic
Differential Equations; With an Example. 5.2 Equilibria and Stability. 5.3
Equilibria and Linear Stability in Higher Dimensions. 5.4. The Jacobian.
6. Integration. 6.1. Accumulated Change. 6.2. The Fundamental Theorem of
Calculus. 6.3. The Anti-Derivative. 6.4. Fundamental Theorem of Calculus
Revisited. 6.5. Properties Of Integrals. 6.6. Integration by Parts. 6.7.
Substitution. Section II. Applied Stats & Data Science. Some Context to
Anchor Us. Math vs. the World. 7. Data and Summary Statistics. 7.1. What Is
Data? 7.2. Data In Python. 7.3. Summary Statistics. 7.4. Ethical and Moral
Considerations: Part 1. 7.5. Mean vs. Median vs. Mode. 7.6. Variance &
Standard Deviation. 7.7. Ethical And Moral Considerations: Episode 2. 7.8.
An Example. 7.9. The Empirical Rule. 8. Visualizing Data. 8.1. Plotting In
Python. 8.2. Scatter Plots. 8.3. Outliers. 8.4. Correlation. 8.6. The
Anatomy of a Technical Document. 8.7. Bad Plots and Why They're Bad. 9.
Probability. 9.1. Ethical and Moral Considerations: A Very Special Episode.
9.2. Counting. 9.3. Permutations. 9.4. Combinations. 9.5. Combinations With
Replacement. 9.6. Probability. 9.7. Properties of Probabilities. 9.8. More
Notation. 9.9. Conditional Probability. 9.10. Bayes' Theorem. 9.11. The
Prosecutor's Fallacy. 9.12. The Law of Total Probability. 10. Probability
Distributions. 10.1. Discrete Probability Distributions. 10.2. The Binomial
Distribution. 10.3. Trinomial Distribution. 10.4. Cumulative Probability
Distributions. 10.5. Continuous Probability. 10.6. Continuous vs Discrete
Probability Distributions. 10.7. Probability Density Functions. 10.8. The
Normal Distribution. 10.9. Other Useful Distributions. 10.10. Mean, Median,
Mode, Variance. 10.11. Summing To Infinity. 10.12. Probability and Python.
10.13. Practice Problems. 11. Fitting Data. 11.1. Defining Relationships.
11.2. Data and Lines. 11.3. Distribution Fitting & Likelihood. 11.4. Dummy
Variables. 11.5. Logistic Regression. 11.6. Logistic Regression in Python.
11.7. Iterated Logistic Regression. 11.8. Random Forest Classification.
11.9. Bootstrapping & Confidence Intervals. 11.10. T-Statistics. 11.11. The
Dichotomous Nature of P-Values. A. A Crash Course in Python.
Functions. 1.1. Anatomy of a Function. 1.2. Modeling With Mathematics. 1.3.
Constants and Linear Functions. 1.4. Polynomials. 1.5. Exponentials And
Logarithms. 1.6. Functions in Higher Dimensions. 1.7. Contour Diagrams.
1.8. Models In Two Dimensions. 1.9. Variables vs Parameters. 2.
Derivatives. 2.1. The Tangent Line. 2.2. Approximating Derivatives of
Functions. 2.3. Limits. 2.4. Limits And Derivates. 2.5. Derivative
Formulas. 2.6. The Product Rule. 2.7. The Chain Rule. 2.8. Mixing Rules.
2.9. Critical Values. 2.10. Constrained Optimization. 2.11. Elasticity.
2.12. Partial Derivatives. 3. Linear Algebra. 3.1. Vectors. 3.2. Matrices.
3.3. Multiplication: Numbers and Matrices. 3.4. Multiplication: Matrix and
Vectors. 3.5. Multiplication: Matrix and Matrix. 3.6. Leslie Matrices. 3.7.
The Determinant. 3.8. Eigenvalues & Eigenvectors. 4. Derivatives in
Multiple Dimensions. 4.1. Applications. 4.2. Distribution Fitting,
Probability and Likelihood. 5. Differential Equations. 5.1. Solving Basic
Differential Equations; With an Example. 5.2 Equilibria and Stability. 5.3
Equilibria and Linear Stability in Higher Dimensions. 5.4. The Jacobian.
6. Integration. 6.1. Accumulated Change. 6.2. The Fundamental Theorem of
Calculus. 6.3. The Anti-Derivative. 6.4. Fundamental Theorem of Calculus
Revisited. 6.5. Properties Of Integrals. 6.6. Integration by Parts. 6.7.
Substitution. Section II. Applied Stats & Data Science. Some Context to
Anchor Us. Math vs. the World. 7. Data and Summary Statistics. 7.1. What Is
Data? 7.2. Data In Python. 7.3. Summary Statistics. 7.4. Ethical and Moral
Considerations: Part 1. 7.5. Mean vs. Median vs. Mode. 7.6. Variance &
Standard Deviation. 7.7. Ethical And Moral Considerations: Episode 2. 7.8.
An Example. 7.9. The Empirical Rule. 8. Visualizing Data. 8.1. Plotting In
Python. 8.2. Scatter Plots. 8.3. Outliers. 8.4. Correlation. 8.6. The
Anatomy of a Technical Document. 8.7. Bad Plots and Why They're Bad. 9.
Probability. 9.1. Ethical and Moral Considerations: A Very Special Episode.
9.2. Counting. 9.3. Permutations. 9.4. Combinations. 9.5. Combinations With
Replacement. 9.6. Probability. 9.7. Properties of Probabilities. 9.8. More
Notation. 9.9. Conditional Probability. 9.10. Bayes' Theorem. 9.11. The
Prosecutor's Fallacy. 9.12. The Law of Total Probability. 10. Probability
Distributions. 10.1. Discrete Probability Distributions. 10.2. The Binomial
Distribution. 10.3. Trinomial Distribution. 10.4. Cumulative Probability
Distributions. 10.5. Continuous Probability. 10.6. Continuous vs Discrete
Probability Distributions. 10.7. Probability Density Functions. 10.8. The
Normal Distribution. 10.9. Other Useful Distributions. 10.10. Mean, Median,
Mode, Variance. 10.11. Summing To Infinity. 10.12. Probability and Python.
10.13. Practice Problems. 11. Fitting Data. 11.1. Defining Relationships.
11.2. Data and Lines. 11.3. Distribution Fitting & Likelihood. 11.4. Dummy
Variables. 11.5. Logistic Regression. 11.6. Logistic Regression in Python.
11.7. Iterated Logistic Regression. 11.8. Random Forest Classification.
11.9. Bootstrapping & Confidence Intervals. 11.10. T-Statistics. 11.11. The
Dichotomous Nature of P-Values. A. A Crash Course in Python.
Section I. Applied Mathematics. The Plot (so you don't lose it). 1.
Functions. 1.1. Anatomy of a Function. 1.2. Modeling With Mathematics. 1.3.
Constants and Linear Functions. 1.4. Polynomials. 1.5. Exponentials And
Logarithms. 1.6. Functions in Higher Dimensions. 1.7. Contour Diagrams.
1.8. Models In Two Dimensions. 1.9. Variables vs Parameters. 2.
Derivatives. 2.1. The Tangent Line. 2.2. Approximating Derivatives of
Functions. 2.3. Limits. 2.4. Limits And Derivates. 2.5. Derivative
Formulas. 2.6. The Product Rule. 2.7. The Chain Rule. 2.8. Mixing Rules.
2.9. Critical Values. 2.10. Constrained Optimization. 2.11. Elasticity.
2.12. Partial Derivatives. 3. Linear Algebra. 3.1. Vectors. 3.2. Matrices.
3.3. Multiplication: Numbers and Matrices. 3.4. Multiplication: Matrix and
Vectors. 3.5. Multiplication: Matrix and Matrix. 3.6. Leslie Matrices. 3.7.
The Determinant. 3.8. Eigenvalues & Eigenvectors. 4. Derivatives in
Multiple Dimensions. 4.1. Applications. 4.2. Distribution Fitting,
Probability and Likelihood. 5. Differential Equations. 5.1. Solving Basic
Differential Equations; With an Example. 5.2 Equilibria and Stability. 5.3
Equilibria and Linear Stability in Higher Dimensions. 5.4. The Jacobian.
6. Integration. 6.1. Accumulated Change. 6.2. The Fundamental Theorem of
Calculus. 6.3. The Anti-Derivative. 6.4. Fundamental Theorem of Calculus
Revisited. 6.5. Properties Of Integrals. 6.6. Integration by Parts. 6.7.
Substitution. Section II. Applied Stats & Data Science. Some Context to
Anchor Us. Math vs. the World. 7. Data and Summary Statistics. 7.1. What Is
Data? 7.2. Data In Python. 7.3. Summary Statistics. 7.4. Ethical and Moral
Considerations: Part 1. 7.5. Mean vs. Median vs. Mode. 7.6. Variance &
Standard Deviation. 7.7. Ethical And Moral Considerations: Episode 2. 7.8.
An Example. 7.9. The Empirical Rule. 8. Visualizing Data. 8.1. Plotting In
Python. 8.2. Scatter Plots. 8.3. Outliers. 8.4. Correlation. 8.6. The
Anatomy of a Technical Document. 8.7. Bad Plots and Why They're Bad. 9.
Probability. 9.1. Ethical and Moral Considerations: A Very Special Episode.
9.2. Counting. 9.3. Permutations. 9.4. Combinations. 9.5. Combinations With
Replacement. 9.6. Probability. 9.7. Properties of Probabilities. 9.8. More
Notation. 9.9. Conditional Probability. 9.10. Bayes' Theorem. 9.11. The
Prosecutor's Fallacy. 9.12. The Law of Total Probability. 10. Probability
Distributions. 10.1. Discrete Probability Distributions. 10.2. The Binomial
Distribution. 10.3. Trinomial Distribution. 10.4. Cumulative Probability
Distributions. 10.5. Continuous Probability. 10.6. Continuous vs Discrete
Probability Distributions. 10.7. Probability Density Functions. 10.8. The
Normal Distribution. 10.9. Other Useful Distributions. 10.10. Mean, Median,
Mode, Variance. 10.11. Summing To Infinity. 10.12. Probability and Python.
10.13. Practice Problems. 11. Fitting Data. 11.1. Defining Relationships.
11.2. Data and Lines. 11.3. Distribution Fitting & Likelihood. 11.4. Dummy
Variables. 11.5. Logistic Regression. 11.6. Logistic Regression in Python.
11.7. Iterated Logistic Regression. 11.8. Random Forest Classification.
11.9. Bootstrapping & Confidence Intervals. 11.10. T-Statistics. 11.11. The
Dichotomous Nature of P-Values. A. A Crash Course in Python.
Functions. 1.1. Anatomy of a Function. 1.2. Modeling With Mathematics. 1.3.
Constants and Linear Functions. 1.4. Polynomials. 1.5. Exponentials And
Logarithms. 1.6. Functions in Higher Dimensions. 1.7. Contour Diagrams.
1.8. Models In Two Dimensions. 1.9. Variables vs Parameters. 2.
Derivatives. 2.1. The Tangent Line. 2.2. Approximating Derivatives of
Functions. 2.3. Limits. 2.4. Limits And Derivates. 2.5. Derivative
Formulas. 2.6. The Product Rule. 2.7. The Chain Rule. 2.8. Mixing Rules.
2.9. Critical Values. 2.10. Constrained Optimization. 2.11. Elasticity.
2.12. Partial Derivatives. 3. Linear Algebra. 3.1. Vectors. 3.2. Matrices.
3.3. Multiplication: Numbers and Matrices. 3.4. Multiplication: Matrix and
Vectors. 3.5. Multiplication: Matrix and Matrix. 3.6. Leslie Matrices. 3.7.
The Determinant. 3.8. Eigenvalues & Eigenvectors. 4. Derivatives in
Multiple Dimensions. 4.1. Applications. 4.2. Distribution Fitting,
Probability and Likelihood. 5. Differential Equations. 5.1. Solving Basic
Differential Equations; With an Example. 5.2 Equilibria and Stability. 5.3
Equilibria and Linear Stability in Higher Dimensions. 5.4. The Jacobian.
6. Integration. 6.1. Accumulated Change. 6.2. The Fundamental Theorem of
Calculus. 6.3. The Anti-Derivative. 6.4. Fundamental Theorem of Calculus
Revisited. 6.5. Properties Of Integrals. 6.6. Integration by Parts. 6.7.
Substitution. Section II. Applied Stats & Data Science. Some Context to
Anchor Us. Math vs. the World. 7. Data and Summary Statistics. 7.1. What Is
Data? 7.2. Data In Python. 7.3. Summary Statistics. 7.4. Ethical and Moral
Considerations: Part 1. 7.5. Mean vs. Median vs. Mode. 7.6. Variance &
Standard Deviation. 7.7. Ethical And Moral Considerations: Episode 2. 7.8.
An Example. 7.9. The Empirical Rule. 8. Visualizing Data. 8.1. Plotting In
Python. 8.2. Scatter Plots. 8.3. Outliers. 8.4. Correlation. 8.6. The
Anatomy of a Technical Document. 8.7. Bad Plots and Why They're Bad. 9.
Probability. 9.1. Ethical and Moral Considerations: A Very Special Episode.
9.2. Counting. 9.3. Permutations. 9.4. Combinations. 9.5. Combinations With
Replacement. 9.6. Probability. 9.7. Properties of Probabilities. 9.8. More
Notation. 9.9. Conditional Probability. 9.10. Bayes' Theorem. 9.11. The
Prosecutor's Fallacy. 9.12. The Law of Total Probability. 10. Probability
Distributions. 10.1. Discrete Probability Distributions. 10.2. The Binomial
Distribution. 10.3. Trinomial Distribution. 10.4. Cumulative Probability
Distributions. 10.5. Continuous Probability. 10.6. Continuous vs Discrete
Probability Distributions. 10.7. Probability Density Functions. 10.8. The
Normal Distribution. 10.9. Other Useful Distributions. 10.10. Mean, Median,
Mode, Variance. 10.11. Summing To Infinity. 10.12. Probability and Python.
10.13. Practice Problems. 11. Fitting Data. 11.1. Defining Relationships.
11.2. Data and Lines. 11.3. Distribution Fitting & Likelihood. 11.4. Dummy
Variables. 11.5. Logistic Regression. 11.6. Logistic Regression in Python.
11.7. Iterated Logistic Regression. 11.8. Random Forest Classification.
11.9. Bootstrapping & Confidence Intervals. 11.10. T-Statistics. 11.11. The
Dichotomous Nature of P-Values. A. A Crash Course in Python.