Trevor F. Cox
Medical Statistics for Cancer Studies (eBook, ePUB)
48,95 €
48,95 €
inkl. MwSt.
Sofort per Download lieferbar
24 °P sammeln
48,95 €
Als Download kaufen
48,95 €
inkl. MwSt.
Sofort per Download lieferbar
24 °P sammeln
Jetzt verschenken
Alle Infos zum eBook verschenken
48,95 €
inkl. MwSt.
Sofort per Download lieferbar
Alle Infos zum eBook verschenken
24 °P sammeln
Trevor F. Cox
Medical Statistics for Cancer Studies (eBook, ePUB)
- Format: ePub
- Merkliste
- Auf die Merkliste
- Bewerten Bewerten
- Teilen
- Produkt teilen
- Produkterinnerung
- Produkterinnerung
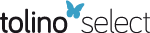
Bitte loggen Sie sich zunächst in Ihr Kundenkonto ein oder registrieren Sie sich bei
bücher.de, um das eBook-Abo tolino select nutzen zu können.
Hier können Sie sich einloggen
Hier können Sie sich einloggen
Sie sind bereits eingeloggt. Klicken Sie auf 2. tolino select Abo, um fortzufahren.
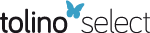
Bitte loggen Sie sich zunächst in Ihr Kundenkonto ein oder registrieren Sie sich bei bücher.de, um das eBook-Abo tolino select nutzen zu können.
This textbook shows how cancer data can be analysed in a variety of ways, covering cancer clinical trial data, epidemiological data, biological data, and genetic data. It provides detailed overviews of survival analysis, clinical trials, regression analysis, epidemiology, meta-analysis, biomarkers, and cancer informatics.
- Geräte: eReader
- mit Kopierschutz
- eBook Hilfe
Andere Kunden interessierten sich auch für
- Trevor F. CoxMedical Statistics for Cancer Studies (eBook, PDF)48,95 €
- Nusrat RabbeeBiomarker Analysis in Clinical Trials with R (eBook, ePUB)43,95 €
- Principles and Practice of Clinical Research (eBook, ePUB)84,95 €
- Andrew P. GrieveHybrid Frequentist/Bayesian Power and Bayesian Power in Planning Clinical Trials (eBook, ePUB)47,95 €
- Andrew B. LawsonUsing R for Bayesian Spatial and Spatio-Temporal Health Modeling (eBook, ePUB)47,95 €
- Value of Information for Healthcare Decision-Making (eBook, ePUB)52,95 €
- Handbook of Statistical Methods for Randomized Controlled Trials (eBook, ePUB)68,95 €
-
-
-
This textbook shows how cancer data can be analysed in a variety of ways, covering cancer clinical trial data, epidemiological data, biological data, and genetic data. It provides detailed overviews of survival analysis, clinical trials, regression analysis, epidemiology, meta-analysis, biomarkers, and cancer informatics.
Dieser Download kann aus rechtlichen Gründen nur mit Rechnungsadresse in A, B, BG, CY, CZ, D, DK, EW, E, FIN, F, GR, HR, H, IRL, I, LT, L, LR, M, NL, PL, P, R, S, SLO, SK ausgeliefert werden.
Produktdetails
- Produktdetails
- Verlag: Taylor & Francis eBooks
- Seitenzahl: 333
- Erscheinungstermin: 23. Juni 2022
- Englisch
- ISBN-13: 9781000601152
- Artikelnr.: 63912237
- Verlag: Taylor & Francis eBooks
- Seitenzahl: 333
- Erscheinungstermin: 23. Juni 2022
- Englisch
- ISBN-13: 9781000601152
- Artikelnr.: 63912237
- Herstellerkennzeichnung Die Herstellerinformationen sind derzeit nicht verfügbar.
Trevor F. Cox is retired from Liverpool Cancer Trials Unit, University of Liverpool, UK
1 Introduction. 1.1. About Cancer. 1.2. Cancer studies. 1.3. R Code. 2. Cancer Biology and Genetics for Non-Biologists. 2.1. Cells. 2.2. DNA, Genes, RNA and Proteins. 2.3. Cancer - DNA Gone Wrong. 2.4. Cancer Treatments. 2.5. Measuring Cancer in the Patient. 3. Survival Analysis. 3.1. The Amazing Survival Equations. 3.2. Non-parametric Estimation of Survival Curves. 3.3. Fitting Parametric Survival Curves to Data. 3.4. Comparing Two Survival Distributions. 3.5. The ESPAC4-Trial. 3.6. Comparing Two Parametric Survival Curves. 4. Designing and Running a Clinical Trial. 4.1. Types of Trials and Studies. 4.2. Clinical Trials. 5. Regression Analysis for Survival Data. 5.1. A Weibull Parametric Regression Model. 5.2. Cox Proportional Hazards Model. 5.3. Accelerated Failure Time (AFT) Models. 5.4. Proportional Odds Models. 5.5. Parametric Survival Distributions for PH and AFT Models. 5.6. Flexible Parametric Models. 6. Clinical Trials: The Statistician's Role. 6.1. Sample Size Calculation. 6.2. Examples of Sample Size Calculations; Phases I to III. 6.3. Group Sequential Designs. 6.4. More Statistical Tasks for Clinical Trials. 7. Cancer Epidemiology. 7.1. Measuring Cancer. 7.2. Cancer Statistics for Countries. 7.3. Cohort Studies. 7.4. Case-control Studies. 7.5. Cross-sectional Studies. 7.6. Spatial Epidemiology. 8. Meta-Analysis. 8.1. How to Carry Out a Systematic Review. 8.2. Fixed Effects Model. 8.3. Random Effects Model. 8.4. Bayesian Meta-analysis. 8.5. Network Meta-analysis. 8.6. Individual Patient Data. 9. Cancer Biomarkers. 9.1. Diagnostic Biomarkers. 9.2. Prognostic Biomarkers. 9.3. Predictive Biomarkers for Pancreatic Cancer. 9.4. Biomarker Trial Design. 10. Cancer Informatics. 10.1. Producing Genetic Data. 10.2. Analysis of Microarray Data. 10.3. Pre-processing NGS Data. 10.4. TCGA-KIRC: Renal Clear Cell Carcinoma.
1 Introduction. 1.1. About Cancer. 1.2. Cancer studies. 1.3. R Code. 2.
Cancer Biology and Genetics for Non-Biologists. 2.1. Cells. 2.2. DNA,
Genes, RNA and Proteins. 2.3. Cancer - DNA Gone Wrong. 2.4. Cancer
Treatments. 2.5. Measuring Cancer in the Patient. 3. Survival Analysis.
3.1. The Amazing Survival Equations. 3.2. Non-parametric Estimation of
Survival Curves. 3.3. Fitting Parametric Survival Curves to Data. 3.4.
Comparing Two Survival Distributions. 3.5. The ESPAC4-Trial. 3.6. Comparing
Two Parametric Survival Curves. 4. Designing and Running a Clinical Trial.
4.1. Types of Trials and Studies. 4.2. Clinical Trials. 5. Regression
Analysis for Survival Data. 5.1. A Weibull Parametric Regression Model.
5.2. Cox Proportional Hazards Model. 5.3. Accelerated Failure Time (AFT)
Models. 5.4. Proportional Odds Models. 5.5. Parametric Survival
Distributions for PH and AFT Models. 5.6. Flexible Parametric Models. 6.
Clinical Trials: The Statistician's Role. 6.1. Sample Size Calculation.
6.2. Examples of Sample Size Calculations; Phases I to III. 6.3. Group
Sequential Designs. 6.4. More Statistical Tasks for Clinical Trials. 7.
Cancer Epidemiology. 7.1. Measuring Cancer. 7.2. Cancer Statistics for
Countries. 7.3. Cohort Studies. 7.4. Case-control Studies. 7.5.
Cross-sectional Studies. 7.6. Spatial Epidemiology. 8. Meta-Analysis. 8.1.
How to Carry Out a Systematic Review. 8.2. Fixed Effects Model. 8.3. Random
Effects Model. 8.4. Bayesian Meta-analysis. 8.5. Network Meta-analysis.
8.6. Individual Patient Data. 9. Cancer Biomarkers. 9.1. Diagnostic
Biomarkers. 9.2. Prognostic Biomarkers. 9.3. Predictive Biomarkers for
Pancreatic Cancer. 9.4. Biomarker Trial Design. 10. Cancer Informatics.
10.1. Producing Genetic Data. 10.2. Analysis of Microarray Data. 10.3.
Pre-processing NGS Data. 10.4. TCGA-KIRC: Renal Clear Cell Carcinoma.
Cancer Biology and Genetics for Non-Biologists. 2.1. Cells. 2.2. DNA,
Genes, RNA and Proteins. 2.3. Cancer - DNA Gone Wrong. 2.4. Cancer
Treatments. 2.5. Measuring Cancer in the Patient. 3. Survival Analysis.
3.1. The Amazing Survival Equations. 3.2. Non-parametric Estimation of
Survival Curves. 3.3. Fitting Parametric Survival Curves to Data. 3.4.
Comparing Two Survival Distributions. 3.5. The ESPAC4-Trial. 3.6. Comparing
Two Parametric Survival Curves. 4. Designing and Running a Clinical Trial.
4.1. Types of Trials and Studies. 4.2. Clinical Trials. 5. Regression
Analysis for Survival Data. 5.1. A Weibull Parametric Regression Model.
5.2. Cox Proportional Hazards Model. 5.3. Accelerated Failure Time (AFT)
Models. 5.4. Proportional Odds Models. 5.5. Parametric Survival
Distributions for PH and AFT Models. 5.6. Flexible Parametric Models. 6.
Clinical Trials: The Statistician's Role. 6.1. Sample Size Calculation.
6.2. Examples of Sample Size Calculations; Phases I to III. 6.3. Group
Sequential Designs. 6.4. More Statistical Tasks for Clinical Trials. 7.
Cancer Epidemiology. 7.1. Measuring Cancer. 7.2. Cancer Statistics for
Countries. 7.3. Cohort Studies. 7.4. Case-control Studies. 7.5.
Cross-sectional Studies. 7.6. Spatial Epidemiology. 8. Meta-Analysis. 8.1.
How to Carry Out a Systematic Review. 8.2. Fixed Effects Model. 8.3. Random
Effects Model. 8.4. Bayesian Meta-analysis. 8.5. Network Meta-analysis.
8.6. Individual Patient Data. 9. Cancer Biomarkers. 9.1. Diagnostic
Biomarkers. 9.2. Prognostic Biomarkers. 9.3. Predictive Biomarkers for
Pancreatic Cancer. 9.4. Biomarker Trial Design. 10. Cancer Informatics.
10.1. Producing Genetic Data. 10.2. Analysis of Microarray Data. 10.3.
Pre-processing NGS Data. 10.4. TCGA-KIRC: Renal Clear Cell Carcinoma.
1 Introduction. 1.1. About Cancer. 1.2. Cancer studies. 1.3. R Code. 2. Cancer Biology and Genetics for Non-Biologists. 2.1. Cells. 2.2. DNA, Genes, RNA and Proteins. 2.3. Cancer - DNA Gone Wrong. 2.4. Cancer Treatments. 2.5. Measuring Cancer in the Patient. 3. Survival Analysis. 3.1. The Amazing Survival Equations. 3.2. Non-parametric Estimation of Survival Curves. 3.3. Fitting Parametric Survival Curves to Data. 3.4. Comparing Two Survival Distributions. 3.5. The ESPAC4-Trial. 3.6. Comparing Two Parametric Survival Curves. 4. Designing and Running a Clinical Trial. 4.1. Types of Trials and Studies. 4.2. Clinical Trials. 5. Regression Analysis for Survival Data. 5.1. A Weibull Parametric Regression Model. 5.2. Cox Proportional Hazards Model. 5.3. Accelerated Failure Time (AFT) Models. 5.4. Proportional Odds Models. 5.5. Parametric Survival Distributions for PH and AFT Models. 5.6. Flexible Parametric Models. 6. Clinical Trials: The Statistician's Role. 6.1. Sample Size Calculation. 6.2. Examples of Sample Size Calculations; Phases I to III. 6.3. Group Sequential Designs. 6.4. More Statistical Tasks for Clinical Trials. 7. Cancer Epidemiology. 7.1. Measuring Cancer. 7.2. Cancer Statistics for Countries. 7.3. Cohort Studies. 7.4. Case-control Studies. 7.5. Cross-sectional Studies. 7.6. Spatial Epidemiology. 8. Meta-Analysis. 8.1. How to Carry Out a Systematic Review. 8.2. Fixed Effects Model. 8.3. Random Effects Model. 8.4. Bayesian Meta-analysis. 8.5. Network Meta-analysis. 8.6. Individual Patient Data. 9. Cancer Biomarkers. 9.1. Diagnostic Biomarkers. 9.2. Prognostic Biomarkers. 9.3. Predictive Biomarkers for Pancreatic Cancer. 9.4. Biomarker Trial Design. 10. Cancer Informatics. 10.1. Producing Genetic Data. 10.2. Analysis of Microarray Data. 10.3. Pre-processing NGS Data. 10.4. TCGA-KIRC: Renal Clear Cell Carcinoma.
1 Introduction. 1.1. About Cancer. 1.2. Cancer studies. 1.3. R Code. 2.
Cancer Biology and Genetics for Non-Biologists. 2.1. Cells. 2.2. DNA,
Genes, RNA and Proteins. 2.3. Cancer - DNA Gone Wrong. 2.4. Cancer
Treatments. 2.5. Measuring Cancer in the Patient. 3. Survival Analysis.
3.1. The Amazing Survival Equations. 3.2. Non-parametric Estimation of
Survival Curves. 3.3. Fitting Parametric Survival Curves to Data. 3.4.
Comparing Two Survival Distributions. 3.5. The ESPAC4-Trial. 3.6. Comparing
Two Parametric Survival Curves. 4. Designing and Running a Clinical Trial.
4.1. Types of Trials and Studies. 4.2. Clinical Trials. 5. Regression
Analysis for Survival Data. 5.1. A Weibull Parametric Regression Model.
5.2. Cox Proportional Hazards Model. 5.3. Accelerated Failure Time (AFT)
Models. 5.4. Proportional Odds Models. 5.5. Parametric Survival
Distributions for PH and AFT Models. 5.6. Flexible Parametric Models. 6.
Clinical Trials: The Statistician's Role. 6.1. Sample Size Calculation.
6.2. Examples of Sample Size Calculations; Phases I to III. 6.3. Group
Sequential Designs. 6.4. More Statistical Tasks for Clinical Trials. 7.
Cancer Epidemiology. 7.1. Measuring Cancer. 7.2. Cancer Statistics for
Countries. 7.3. Cohort Studies. 7.4. Case-control Studies. 7.5.
Cross-sectional Studies. 7.6. Spatial Epidemiology. 8. Meta-Analysis. 8.1.
How to Carry Out a Systematic Review. 8.2. Fixed Effects Model. 8.3. Random
Effects Model. 8.4. Bayesian Meta-analysis. 8.5. Network Meta-analysis.
8.6. Individual Patient Data. 9. Cancer Biomarkers. 9.1. Diagnostic
Biomarkers. 9.2. Prognostic Biomarkers. 9.3. Predictive Biomarkers for
Pancreatic Cancer. 9.4. Biomarker Trial Design. 10. Cancer Informatics.
10.1. Producing Genetic Data. 10.2. Analysis of Microarray Data. 10.3.
Pre-processing NGS Data. 10.4. TCGA-KIRC: Renal Clear Cell Carcinoma.
Cancer Biology and Genetics for Non-Biologists. 2.1. Cells. 2.2. DNA,
Genes, RNA and Proteins. 2.3. Cancer - DNA Gone Wrong. 2.4. Cancer
Treatments. 2.5. Measuring Cancer in the Patient. 3. Survival Analysis.
3.1. The Amazing Survival Equations. 3.2. Non-parametric Estimation of
Survival Curves. 3.3. Fitting Parametric Survival Curves to Data. 3.4.
Comparing Two Survival Distributions. 3.5. The ESPAC4-Trial. 3.6. Comparing
Two Parametric Survival Curves. 4. Designing and Running a Clinical Trial.
4.1. Types of Trials and Studies. 4.2. Clinical Trials. 5. Regression
Analysis for Survival Data. 5.1. A Weibull Parametric Regression Model.
5.2. Cox Proportional Hazards Model. 5.3. Accelerated Failure Time (AFT)
Models. 5.4. Proportional Odds Models. 5.5. Parametric Survival
Distributions for PH and AFT Models. 5.6. Flexible Parametric Models. 6.
Clinical Trials: The Statistician's Role. 6.1. Sample Size Calculation.
6.2. Examples of Sample Size Calculations; Phases I to III. 6.3. Group
Sequential Designs. 6.4. More Statistical Tasks for Clinical Trials. 7.
Cancer Epidemiology. 7.1. Measuring Cancer. 7.2. Cancer Statistics for
Countries. 7.3. Cohort Studies. 7.4. Case-control Studies. 7.5.
Cross-sectional Studies. 7.6. Spatial Epidemiology. 8. Meta-Analysis. 8.1.
How to Carry Out a Systematic Review. 8.2. Fixed Effects Model. 8.3. Random
Effects Model. 8.4. Bayesian Meta-analysis. 8.5. Network Meta-analysis.
8.6. Individual Patient Data. 9. Cancer Biomarkers. 9.1. Diagnostic
Biomarkers. 9.2. Prognostic Biomarkers. 9.3. Predictive Biomarkers for
Pancreatic Cancer. 9.4. Biomarker Trial Design. 10. Cancer Informatics.
10.1. Producing Genetic Data. 10.2. Analysis of Microarray Data. 10.3.
Pre-processing NGS Data. 10.4. TCGA-KIRC: Renal Clear Cell Carcinoma.