William P. Fox, Robert E. Burks
Modeling Change and Uncertainty (eBook, PDF)
Machine Learning and Other Techniques
59,95 €
59,95 €
inkl. MwSt.
Sofort per Download lieferbar
30 °P sammeln
59,95 €
Als Download kaufen
59,95 €
inkl. MwSt.
Sofort per Download lieferbar
30 °P sammeln
Jetzt verschenken
Alle Infos zum eBook verschenken
59,95 €
inkl. MwSt.
Sofort per Download lieferbar
Alle Infos zum eBook verschenken
30 °P sammeln
William P. Fox, Robert E. Burks
Modeling Change and Uncertainty (eBook, PDF)
Machine Learning and Other Techniques
- Format: PDF
- Merkliste
- Auf die Merkliste
- Bewerten Bewerten
- Teilen
- Produkt teilen
- Produkterinnerung
- Produkterinnerung
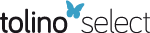
Bitte loggen Sie sich zunächst in Ihr Kundenkonto ein oder registrieren Sie sich bei
bücher.de, um das eBook-Abo tolino select nutzen zu können.
Hier können Sie sich einloggen
Hier können Sie sich einloggen
Sie sind bereits eingeloggt. Klicken Sie auf 2. tolino select Abo, um fortzufahren.
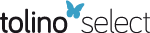
Bitte loggen Sie sich zunächst in Ihr Kundenkonto ein oder registrieren Sie sich bei bücher.de, um das eBook-Abo tolino select nutzen zu können.
This book offers a problem-solving approach. The authors introduce a problem to help motivate the learning of a particular mathematical modeling topic. The problem provides the issue or what is needed to solve using an appropriate modeling technique.
- Geräte: PC
- mit Kopierschutz
- eBook Hilfe
Andere Kunden interessierten sich auch für
- William P. FoxModeling Change and Uncertainty (eBook, ePUB)59,95 €
- Advances on Mathematical Modeling and Optimization with Its Applications (eBook, PDF)51,95 €
- William P. FoxAdvanced Mathematical Modeling with Technology (eBook, PDF)59,95 €
- Neha GuptaOptimization with LINGO-18 (eBook, PDF)47,95 €
- Bogumil KaminskiMining Complex Networks (eBook, PDF)59,95 €
- Metaheuristic Algorithms in Industry 4.0 (eBook, PDF)45,95 €
- William P FoxModeling Operations Research and Business Analytics (eBook, PDF)49,95 €
-
-
-
This book offers a problem-solving approach. The authors introduce a problem to help motivate the learning of a particular mathematical modeling topic. The problem provides the issue or what is needed to solve using an appropriate modeling technique.
Dieser Download kann aus rechtlichen Gründen nur mit Rechnungsadresse in A, B, BG, CY, CZ, D, DK, EW, E, FIN, F, GR, HR, H, IRL, I, LT, L, LR, M, NL, PL, P, R, S, SLO, SK ausgeliefert werden.
Produktdetails
- Produktdetails
- Verlag: Taylor & Francis eBooks
- Seitenzahl: 464
- Erscheinungstermin: 20. Juli 2022
- Englisch
- ISBN-13: 9781000603873
- Artikelnr.: 64069987
- Verlag: Taylor & Francis eBooks
- Seitenzahl: 464
- Erscheinungstermin: 20. Juli 2022
- Englisch
- ISBN-13: 9781000603873
- Artikelnr.: 64069987
- Herstellerkennzeichnung Die Herstellerinformationen sind derzeit nicht verfügbar.
Dr. William P. Fox is currently a visiting professor of Computational Operations Research at the College of William and Mary. He is an emeritus professor in the Department of Defense Analysis at the Naval Postgraduate School and teaches a three-course sequence in mathematical modeling for decision making. He received his Ph.D. in Industrial Engineering from Clemson University. He has taught at the United States Military Academy for twelve years until retiring and at Francis Marion University where he was the chair of mathematics for eight years. He has many publications and scholarly activities including twenty plus books and one hundred and fifty journal articles.
Colonel (R) Robert E. Burks, Jr., Ph.D. is an Associate Professor in the Defense Analysis Department of the Naval Postgraduate School (NPS) and the Director of the NPS' Wargaming Center. He holds a Ph.D. in Operations Research from the Air Force Institute of Technology. He is a retired logistics Army Colonel with more than thirty years of military experience in leadership, advanced analytics, decision modeling, and logistics operations who served as an Army Operations Research analyst at the Naval Postgraduate School, TRADOC Analysis Center, United States Military Academy, and the United States Army Recruiting Command.
Other book by William P. Fox and Robert E. Burks: Advanced Mathematical Modeling with Technology, 2021, CRC Press.
Other books by William P. Fox from CRC Press:
Mathematical Modeling in the Age of the Pandemic, 2021, CRC Press.
Advanced Problem Solving Using Maple: Applied Mathematics, Operations Research, Business Analytics, and Decision Analysis (w/William Bauldry), 2020, CRC Press.
Mathematical Modeling with Excel (w/Brian Albright), 2020, CRC Press.
Nonlinear Optimization: Models and Applications, 2020, CRC Press.
Advanced Problem Solving with Maple: A First Course (w/William Bauldry), 2019. CRC Press.
Mathematical Modeling for Business Analytics, 2018, CRC Press.
Colonel (R) Robert E. Burks, Jr., Ph.D. is an Associate Professor in the Defense Analysis Department of the Naval Postgraduate School (NPS) and the Director of the NPS' Wargaming Center. He holds a Ph.D. in Operations Research from the Air Force Institute of Technology. He is a retired logistics Army Colonel with more than thirty years of military experience in leadership, advanced analytics, decision modeling, and logistics operations who served as an Army Operations Research analyst at the Naval Postgraduate School, TRADOC Analysis Center, United States Military Academy, and the United States Army Recruiting Command.
Other book by William P. Fox and Robert E. Burks: Advanced Mathematical Modeling with Technology, 2021, CRC Press.
Other books by William P. Fox from CRC Press:
Mathematical Modeling in the Age of the Pandemic, 2021, CRC Press.
Advanced Problem Solving Using Maple: Applied Mathematics, Operations Research, Business Analytics, and Decision Analysis (w/William Bauldry), 2020, CRC Press.
Mathematical Modeling with Excel (w/Brian Albright), 2020, CRC Press.
Nonlinear Optimization: Models and Applications, 2020, CRC Press.
Advanced Problem Solving with Maple: A First Course (w/William Bauldry), 2019. CRC Press.
Mathematical Modeling for Business Analytics, 2018, CRC Press.
1. Perfect Partners: Combining Models of Change and Uncertainty with Technology. 1.1. Overview of the Process of Mathematical Modeling. 1.2. The Modeling Process. 1.3. Illustrative Examples. 1.4. Technology. 1.5. Exercises. 1.6. Projects. 1.7. References and Suggested Further Reading. 2. Modeling Change: Discrete Dynamical Systems (DDS) and Modeling Systems of DDS. 2.1. Introduction and Review of Modeling with Discrete Dynamical Systems. 2.2. Equilibrium and Stability Values and Long-Term Behavior. 2.3. Introduction to Systems of Discrete Dynamical Systems. 2.4. Iteration and Graphical Solution. 2.5. Modeling of Predator-prey Model, SIR Model, and Military Models. 2.6. Technology Examples for Discrete Dynamical Systems. 2.7. Exercises. 2.8. Projects. 2.9. References. 3. Statistical and Probabilistic Models. 3.1. Introduction. 3.2. Understanding Univariate & Multivariate Data. 3.3. Displays of Data and Statistics. 3.4. Statistical Measures. 3.5. Exercises. 3.6. References. 4. Modeling with Probability. 4.1. Classical Probability. 4.2. Bayes' Theorem. 4.3. Discrete Distributions in Modeling. 4.4. Continuous Probability Models. 4.5. Confidence Intervals and Hypothesis Testing. 4.6. Exercises. 4.7. References. 5. Differential Equations. 5.1. Introduction. 5.1. Qualitative Assessment of Autonomous Systems of First Order Differential Equations. 5.2. Solving Homogeneous and Non-Homogeneous Systems. 5.3. Technology Examples for Systems of Ordinary Differential Equations. 5.4. Exercises. 5.5. Projects. 5.6. References and Suggested Future Readings. 6. Forecasting with Linear Programming and Machine Learning. 6.1. Introduction to Forecasting. 6.2. Machine Learning. 6.3. Model Fitting. 6.4. Time Series Models. 6.5. Case Studies of Time Series Data. 6.6. Summary and Conclusions. 6.7. References and Suggested Readings. 7. Stochastic Models and Markov Chains. 7.1. Introduction. 7.2. Transition Matrices. 7.3. Markov Chains and Bayes' Theorem. 7.4. Markov Processes. 7.5. Exercises. 7.6. References. 8. Linear Programming. 8.1. Introduction. 8.2. Formulating Linear Programming Problems. 8.3. Technology Examples for Linear Programming. 8.4. Transportation and Assignment Problems. 8.5. Case Studies in Linear Programming. 8.6. Sensitivity Analysis in MAPLE. 8.7. Stochastic Optimization. 8.8. References. 9. Simulation of Queueing Models. 9.1. Introduction. 9.2. Queueing Model Practice Problems: Solutions. 9.3. Exercises. 9.4. References. 10. Modeling of Financial Analysis. 10.1. Introduction. 10.2. Simple and Compound Interest. 10.3. Rates of Interest, Discounting, and Depreciation. 10.4. Present Value. 10.5. Bond, Annuities, and Shrinking Funds. 10.6. Mortgages and Amortization. 10.7. Advanced Financial Models. 10.8. Exercises. 10.9. Projects. 10.10. References. 11. Reliability Models. 11.1. Introduction to Total Conflict (Zero-Sum) Games. 11.2. Modeling Component Reliability. 11.3. Modeling Series and Parallel Components. 11.4. Modeling Active Redundant Systems. 11.5. Modeling Standby Redundant Systems. 11.6. Models of Large-scale Systems. 11.7. Exercises. 11.8. References. 12. Machine Learning and Unconstrained Optimal Process. 12.1. Introduction. 12.2. The Gradient Method. 12.3. Machine Learning Regression: A Note on Complexity. 12.4. Genetic Algorithm as Machine Learning in R. 12.5. Initial Population. 12.6. Simulated Annealing. 12.7. Exercises. 12.8. References.
1. Perfect Partners: Combining Models of Change and Uncertainty with
Technology. 1.1. Overview of the Process of Mathematical Modeling. 1.2. The
Modeling Process. 1.3. Illustrative Examples. 1.4. Technology. 1.5.
Exercises. 1.6. Projects. 1.7. References and Suggested Further Reading.
2. Modeling Change: Discrete Dynamical Systems (DDS) and Modeling Systems
of DDS. 2.1. Introduction and Review of Modeling with Discrete Dynamical
Systems. 2.2. Equilibrium and Stability Values and Long-Term Behavior. 2.3.
Introduction to Systems of Discrete Dynamical Systems. 2.4. Iteration and
Graphical Solution. 2.5. Modeling of Predator-prey Model, SIR Model, and
Military Models. 2.6. Technology Examples for Discrete Dynamical Systems.
2.7. Exercises. 2.8. Projects. 2.9. References. 3. Statistical and
Probabilistic Models. 3.1. Introduction. 3.2. Understanding Univariate &
Multivariate Data. 3.3. Displays of Data and Statistics. 3.4. Statistical
Measures. 3.5. Exercises. 3.6. References. 4. Modeling with Probability.
4.1. Classical Probability. 4.2. Bayes' Theorem. 4.3. Discrete
Distributions in Modeling. 4.4. Continuous Probability Models. 4.5.
Confidence Intervals and Hypothesis Testing. 4.6. Exercises. 4.7.
References. 5. Differential Equations. 5.1. Introduction. 5.1. Qualitative
Assessment of Autonomous Systems of First Order Differential Equations.
5.2. Solving Homogeneous and Non-Homogeneous Systems. 5.3. Technology
Examples for Systems of Ordinary Differential Equations. 5.4. Exercises.
5.5. Projects. 5.6. References and Suggested Future Readings. 6.
Forecasting with Linear Programming and Machine Learning. 6.1. Introduction
to Forecasting. 6.2. Machine Learning. 6.3. Model Fitting. 6.4. Time Series
Models. 6.5. Case Studies of Time Series Data. 6.6. Summary and
Conclusions. 6.7. References and Suggested Readings. 7. Stochastic Models
and Markov Chains. 7.1. Introduction. 7.2. Transition Matrices. 7.3. Markov
Chains and Bayes' Theorem. 7.4. Markov Processes. 7.5. Exercises. 7.6.
References. 8. Linear Programming. 8.1. Introduction. 8.2. Formulating
Linear Programming Problems. 8.3. Technology Examples for Linear
Programming. 8.4. Transportation and Assignment Problems. 8.5. Case Studies
in Linear Programming. 8.6. Sensitivity Analysis in MAPLE. 8.7. Stochastic
Optimization. 8.8. References. 9. Simulation of Queueing Models. 9.1.
Introduction. 9.2. Queueing Model Practice Problems: Solutions. 9.3.
Exercises. 9.4. References. 10. Modeling of Financial Analysis. 10.1.
Introduction. 10.2. Simple and Compound Interest. 10.3. Rates of Interest,
Discounting, and Depreciation. 10.4. Present Value. 10.5. Bond, Annuities,
and Shrinking Funds. 10.6. Mortgages and Amortization. 10.7. Advanced
Financial Models. 10.8. Exercises. 10.9. Projects. 10.10. References. 11.
Reliability Models. 11.1. Introduction to Total Conflict (Zero-Sum) Games.
11.2. Modeling Component Reliability. 11.3. Modeling Series and Parallel
Components. 11.4. Modeling Active Redundant Systems. 11.5. Modeling Standby
Redundant Systems. 11.6. Models of Large-scale Systems. 11.7. Exercises.
11.8. References. 12. Machine Learning and Unconstrained Optimal Process.
12.1. Introduction. 12.2. The Gradient Method. 12.3. Machine Learning
Regression: A Note on Complexity. 12.4. Genetic Algorithm as Machine
Learning in R. 12.5. Initial Population. 12.6. Simulated Annealing. 12.7.
Exercises. 12.8. References.
Technology. 1.1. Overview of the Process of Mathematical Modeling. 1.2. The
Modeling Process. 1.3. Illustrative Examples. 1.4. Technology. 1.5.
Exercises. 1.6. Projects. 1.7. References and Suggested Further Reading.
2. Modeling Change: Discrete Dynamical Systems (DDS) and Modeling Systems
of DDS. 2.1. Introduction and Review of Modeling with Discrete Dynamical
Systems. 2.2. Equilibrium and Stability Values and Long-Term Behavior. 2.3.
Introduction to Systems of Discrete Dynamical Systems. 2.4. Iteration and
Graphical Solution. 2.5. Modeling of Predator-prey Model, SIR Model, and
Military Models. 2.6. Technology Examples for Discrete Dynamical Systems.
2.7. Exercises. 2.8. Projects. 2.9. References. 3. Statistical and
Probabilistic Models. 3.1. Introduction. 3.2. Understanding Univariate &
Multivariate Data. 3.3. Displays of Data and Statistics. 3.4. Statistical
Measures. 3.5. Exercises. 3.6. References. 4. Modeling with Probability.
4.1. Classical Probability. 4.2. Bayes' Theorem. 4.3. Discrete
Distributions in Modeling. 4.4. Continuous Probability Models. 4.5.
Confidence Intervals and Hypothesis Testing. 4.6. Exercises. 4.7.
References. 5. Differential Equations. 5.1. Introduction. 5.1. Qualitative
Assessment of Autonomous Systems of First Order Differential Equations.
5.2. Solving Homogeneous and Non-Homogeneous Systems. 5.3. Technology
Examples for Systems of Ordinary Differential Equations. 5.4. Exercises.
5.5. Projects. 5.6. References and Suggested Future Readings. 6.
Forecasting with Linear Programming and Machine Learning. 6.1. Introduction
to Forecasting. 6.2. Machine Learning. 6.3. Model Fitting. 6.4. Time Series
Models. 6.5. Case Studies of Time Series Data. 6.6. Summary and
Conclusions. 6.7. References and Suggested Readings. 7. Stochastic Models
and Markov Chains. 7.1. Introduction. 7.2. Transition Matrices. 7.3. Markov
Chains and Bayes' Theorem. 7.4. Markov Processes. 7.5. Exercises. 7.6.
References. 8. Linear Programming. 8.1. Introduction. 8.2. Formulating
Linear Programming Problems. 8.3. Technology Examples for Linear
Programming. 8.4. Transportation and Assignment Problems. 8.5. Case Studies
in Linear Programming. 8.6. Sensitivity Analysis in MAPLE. 8.7. Stochastic
Optimization. 8.8. References. 9. Simulation of Queueing Models. 9.1.
Introduction. 9.2. Queueing Model Practice Problems: Solutions. 9.3.
Exercises. 9.4. References. 10. Modeling of Financial Analysis. 10.1.
Introduction. 10.2. Simple and Compound Interest. 10.3. Rates of Interest,
Discounting, and Depreciation. 10.4. Present Value. 10.5. Bond, Annuities,
and Shrinking Funds. 10.6. Mortgages and Amortization. 10.7. Advanced
Financial Models. 10.8. Exercises. 10.9. Projects. 10.10. References. 11.
Reliability Models. 11.1. Introduction to Total Conflict (Zero-Sum) Games.
11.2. Modeling Component Reliability. 11.3. Modeling Series and Parallel
Components. 11.4. Modeling Active Redundant Systems. 11.5. Modeling Standby
Redundant Systems. 11.6. Models of Large-scale Systems. 11.7. Exercises.
11.8. References. 12. Machine Learning and Unconstrained Optimal Process.
12.1. Introduction. 12.2. The Gradient Method. 12.3. Machine Learning
Regression: A Note on Complexity. 12.4. Genetic Algorithm as Machine
Learning in R. 12.5. Initial Population. 12.6. Simulated Annealing. 12.7.
Exercises. 12.8. References.
1. Perfect Partners: Combining Models of Change and Uncertainty with Technology. 1.1. Overview of the Process of Mathematical Modeling. 1.2. The Modeling Process. 1.3. Illustrative Examples. 1.4. Technology. 1.5. Exercises. 1.6. Projects. 1.7. References and Suggested Further Reading. 2. Modeling Change: Discrete Dynamical Systems (DDS) and Modeling Systems of DDS. 2.1. Introduction and Review of Modeling with Discrete Dynamical Systems. 2.2. Equilibrium and Stability Values and Long-Term Behavior. 2.3. Introduction to Systems of Discrete Dynamical Systems. 2.4. Iteration and Graphical Solution. 2.5. Modeling of Predator-prey Model, SIR Model, and Military Models. 2.6. Technology Examples for Discrete Dynamical Systems. 2.7. Exercises. 2.8. Projects. 2.9. References. 3. Statistical and Probabilistic Models. 3.1. Introduction. 3.2. Understanding Univariate & Multivariate Data. 3.3. Displays of Data and Statistics. 3.4. Statistical Measures. 3.5. Exercises. 3.6. References. 4. Modeling with Probability. 4.1. Classical Probability. 4.2. Bayes' Theorem. 4.3. Discrete Distributions in Modeling. 4.4. Continuous Probability Models. 4.5. Confidence Intervals and Hypothesis Testing. 4.6. Exercises. 4.7. References. 5. Differential Equations. 5.1. Introduction. 5.1. Qualitative Assessment of Autonomous Systems of First Order Differential Equations. 5.2. Solving Homogeneous and Non-Homogeneous Systems. 5.3. Technology Examples for Systems of Ordinary Differential Equations. 5.4. Exercises. 5.5. Projects. 5.6. References and Suggested Future Readings. 6. Forecasting with Linear Programming and Machine Learning. 6.1. Introduction to Forecasting. 6.2. Machine Learning. 6.3. Model Fitting. 6.4. Time Series Models. 6.5. Case Studies of Time Series Data. 6.6. Summary and Conclusions. 6.7. References and Suggested Readings. 7. Stochastic Models and Markov Chains. 7.1. Introduction. 7.2. Transition Matrices. 7.3. Markov Chains and Bayes' Theorem. 7.4. Markov Processes. 7.5. Exercises. 7.6. References. 8. Linear Programming. 8.1. Introduction. 8.2. Formulating Linear Programming Problems. 8.3. Technology Examples for Linear Programming. 8.4. Transportation and Assignment Problems. 8.5. Case Studies in Linear Programming. 8.6. Sensitivity Analysis in MAPLE. 8.7. Stochastic Optimization. 8.8. References. 9. Simulation of Queueing Models. 9.1. Introduction. 9.2. Queueing Model Practice Problems: Solutions. 9.3. Exercises. 9.4. References. 10. Modeling of Financial Analysis. 10.1. Introduction. 10.2. Simple and Compound Interest. 10.3. Rates of Interest, Discounting, and Depreciation. 10.4. Present Value. 10.5. Bond, Annuities, and Shrinking Funds. 10.6. Mortgages and Amortization. 10.7. Advanced Financial Models. 10.8. Exercises. 10.9. Projects. 10.10. References. 11. Reliability Models. 11.1. Introduction to Total Conflict (Zero-Sum) Games. 11.2. Modeling Component Reliability. 11.3. Modeling Series and Parallel Components. 11.4. Modeling Active Redundant Systems. 11.5. Modeling Standby Redundant Systems. 11.6. Models of Large-scale Systems. 11.7. Exercises. 11.8. References. 12. Machine Learning and Unconstrained Optimal Process. 12.1. Introduction. 12.2. The Gradient Method. 12.3. Machine Learning Regression: A Note on Complexity. 12.4. Genetic Algorithm as Machine Learning in R. 12.5. Initial Population. 12.6. Simulated Annealing. 12.7. Exercises. 12.8. References.
1. Perfect Partners: Combining Models of Change and Uncertainty with
Technology. 1.1. Overview of the Process of Mathematical Modeling. 1.2. The
Modeling Process. 1.3. Illustrative Examples. 1.4. Technology. 1.5.
Exercises. 1.6. Projects. 1.7. References and Suggested Further Reading.
2. Modeling Change: Discrete Dynamical Systems (DDS) and Modeling Systems
of DDS. 2.1. Introduction and Review of Modeling with Discrete Dynamical
Systems. 2.2. Equilibrium and Stability Values and Long-Term Behavior. 2.3.
Introduction to Systems of Discrete Dynamical Systems. 2.4. Iteration and
Graphical Solution. 2.5. Modeling of Predator-prey Model, SIR Model, and
Military Models. 2.6. Technology Examples for Discrete Dynamical Systems.
2.7. Exercises. 2.8. Projects. 2.9. References. 3. Statistical and
Probabilistic Models. 3.1. Introduction. 3.2. Understanding Univariate &
Multivariate Data. 3.3. Displays of Data and Statistics. 3.4. Statistical
Measures. 3.5. Exercises. 3.6. References. 4. Modeling with Probability.
4.1. Classical Probability. 4.2. Bayes' Theorem. 4.3. Discrete
Distributions in Modeling. 4.4. Continuous Probability Models. 4.5.
Confidence Intervals and Hypothesis Testing. 4.6. Exercises. 4.7.
References. 5. Differential Equations. 5.1. Introduction. 5.1. Qualitative
Assessment of Autonomous Systems of First Order Differential Equations.
5.2. Solving Homogeneous and Non-Homogeneous Systems. 5.3. Technology
Examples for Systems of Ordinary Differential Equations. 5.4. Exercises.
5.5. Projects. 5.6. References and Suggested Future Readings. 6.
Forecasting with Linear Programming and Machine Learning. 6.1. Introduction
to Forecasting. 6.2. Machine Learning. 6.3. Model Fitting. 6.4. Time Series
Models. 6.5. Case Studies of Time Series Data. 6.6. Summary and
Conclusions. 6.7. References and Suggested Readings. 7. Stochastic Models
and Markov Chains. 7.1. Introduction. 7.2. Transition Matrices. 7.3. Markov
Chains and Bayes' Theorem. 7.4. Markov Processes. 7.5. Exercises. 7.6.
References. 8. Linear Programming. 8.1. Introduction. 8.2. Formulating
Linear Programming Problems. 8.3. Technology Examples for Linear
Programming. 8.4. Transportation and Assignment Problems. 8.5. Case Studies
in Linear Programming. 8.6. Sensitivity Analysis in MAPLE. 8.7. Stochastic
Optimization. 8.8. References. 9. Simulation of Queueing Models. 9.1.
Introduction. 9.2. Queueing Model Practice Problems: Solutions. 9.3.
Exercises. 9.4. References. 10. Modeling of Financial Analysis. 10.1.
Introduction. 10.2. Simple and Compound Interest. 10.3. Rates of Interest,
Discounting, and Depreciation. 10.4. Present Value. 10.5. Bond, Annuities,
and Shrinking Funds. 10.6. Mortgages and Amortization. 10.7. Advanced
Financial Models. 10.8. Exercises. 10.9. Projects. 10.10. References. 11.
Reliability Models. 11.1. Introduction to Total Conflict (Zero-Sum) Games.
11.2. Modeling Component Reliability. 11.3. Modeling Series and Parallel
Components. 11.4. Modeling Active Redundant Systems. 11.5. Modeling Standby
Redundant Systems. 11.6. Models of Large-scale Systems. 11.7. Exercises.
11.8. References. 12. Machine Learning and Unconstrained Optimal Process.
12.1. Introduction. 12.2. The Gradient Method. 12.3. Machine Learning
Regression: A Note on Complexity. 12.4. Genetic Algorithm as Machine
Learning in R. 12.5. Initial Population. 12.6. Simulated Annealing. 12.7.
Exercises. 12.8. References.
Technology. 1.1. Overview of the Process of Mathematical Modeling. 1.2. The
Modeling Process. 1.3. Illustrative Examples. 1.4. Technology. 1.5.
Exercises. 1.6. Projects. 1.7. References and Suggested Further Reading.
2. Modeling Change: Discrete Dynamical Systems (DDS) and Modeling Systems
of DDS. 2.1. Introduction and Review of Modeling with Discrete Dynamical
Systems. 2.2. Equilibrium and Stability Values and Long-Term Behavior. 2.3.
Introduction to Systems of Discrete Dynamical Systems. 2.4. Iteration and
Graphical Solution. 2.5. Modeling of Predator-prey Model, SIR Model, and
Military Models. 2.6. Technology Examples for Discrete Dynamical Systems.
2.7. Exercises. 2.8. Projects. 2.9. References. 3. Statistical and
Probabilistic Models. 3.1. Introduction. 3.2. Understanding Univariate &
Multivariate Data. 3.3. Displays of Data and Statistics. 3.4. Statistical
Measures. 3.5. Exercises. 3.6. References. 4. Modeling with Probability.
4.1. Classical Probability. 4.2. Bayes' Theorem. 4.3. Discrete
Distributions in Modeling. 4.4. Continuous Probability Models. 4.5.
Confidence Intervals and Hypothesis Testing. 4.6. Exercises. 4.7.
References. 5. Differential Equations. 5.1. Introduction. 5.1. Qualitative
Assessment of Autonomous Systems of First Order Differential Equations.
5.2. Solving Homogeneous and Non-Homogeneous Systems. 5.3. Technology
Examples for Systems of Ordinary Differential Equations. 5.4. Exercises.
5.5. Projects. 5.6. References and Suggested Future Readings. 6.
Forecasting with Linear Programming and Machine Learning. 6.1. Introduction
to Forecasting. 6.2. Machine Learning. 6.3. Model Fitting. 6.4. Time Series
Models. 6.5. Case Studies of Time Series Data. 6.6. Summary and
Conclusions. 6.7. References and Suggested Readings. 7. Stochastic Models
and Markov Chains. 7.1. Introduction. 7.2. Transition Matrices. 7.3. Markov
Chains and Bayes' Theorem. 7.4. Markov Processes. 7.5. Exercises. 7.6.
References. 8. Linear Programming. 8.1. Introduction. 8.2. Formulating
Linear Programming Problems. 8.3. Technology Examples for Linear
Programming. 8.4. Transportation and Assignment Problems. 8.5. Case Studies
in Linear Programming. 8.6. Sensitivity Analysis in MAPLE. 8.7. Stochastic
Optimization. 8.8. References. 9. Simulation of Queueing Models. 9.1.
Introduction. 9.2. Queueing Model Practice Problems: Solutions. 9.3.
Exercises. 9.4. References. 10. Modeling of Financial Analysis. 10.1.
Introduction. 10.2. Simple and Compound Interest. 10.3. Rates of Interest,
Discounting, and Depreciation. 10.4. Present Value. 10.5. Bond, Annuities,
and Shrinking Funds. 10.6. Mortgages and Amortization. 10.7. Advanced
Financial Models. 10.8. Exercises. 10.9. Projects. 10.10. References. 11.
Reliability Models. 11.1. Introduction to Total Conflict (Zero-Sum) Games.
11.2. Modeling Component Reliability. 11.3. Modeling Series and Parallel
Components. 11.4. Modeling Active Redundant Systems. 11.5. Modeling Standby
Redundant Systems. 11.6. Models of Large-scale Systems. 11.7. Exercises.
11.8. References. 12. Machine Learning and Unconstrained Optimal Process.
12.1. Introduction. 12.2. The Gradient Method. 12.3. Machine Learning
Regression: A Note on Complexity. 12.4. Genetic Algorithm as Machine
Learning in R. 12.5. Initial Population. 12.6. Simulated Annealing. 12.7.
Exercises. 12.8. References.