Alle Infos zum eBook verschenken
- Format: ePub
- Merkliste
- Auf die Merkliste
- Bewerten Bewerten
- Teilen
- Produkt teilen
- Produkterinnerung
- Produkterinnerung
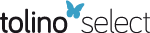
Hier können Sie sich einloggen
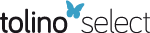
Bitte loggen Sie sich zunächst in Ihr Kundenkonto ein oder registrieren Sie sich bei bücher.de, um das eBook-Abo tolino select nutzen zu können.
Modeling is now one of the most efficient methodologies in life sciences. From practice to theory, this book develops this approach illustrated by many examples; general concepts and the current state of the art are also presented and discussed. An historical and general introduction informs the reader how mathematics and formal tools are used to solve biological problems at all levels of the organization of life. The core of this book explains how this is done, based on practical examples coming, for the most part, from the author's personal experience. In most cases, data are included so…mehr
- Geräte: eReader
- mit Kopierschutz
- eBook Hilfe
- Größe: 6.84MB
- James P. GibbsProblem-Solving in Conservation Biology and Wildlife Management (eBook, ePUB)50,99 €
- Fifty Years of Invasion Ecology (eBook, ePUB)63,99 €
- C. Barry CoxBiogeography (eBook, ePUB)61,99 €
- Douglas E. FaceyThe Diversity of Fishes (eBook, ePUB)55,99 €
- Christian LévêqueBiodiversity Erosion (eBook, ePUB)126,99 €
- Michael J. ConroyQuantitative Conservation of Vertebrates (eBook, ePUB)66,99 €
- James S. DianaBiology and Ecology of Fishes (eBook, ePUB)76,99 €
-
-
-
Dieser Download kann aus rechtlichen Gründen nur mit Rechnungsadresse in A, B, BG, CY, CZ, D, DK, EW, E, FIN, F, GR, HR, H, IRL, I, LT, L, LR, M, NL, PL, P, R, S, SLO, SK ausgeliefert werden.
- Produktdetails
- Verlag: John Wiley & Sons
- Seitenzahl: 620
- Erscheinungstermin: 27. Dezember 2012
- Englisch
- ISBN-13: 9781118569696
- Artikelnr.: 38254115
- Verlag: John Wiley & Sons
- Seitenzahl: 620
- Erscheinungstermin: 27. Dezember 2012
- Englisch
- ISBN-13: 9781118569696
- Artikelnr.: 38254115
- Herstellerkennzeichnung Die Herstellerinformationen sind derzeit nicht verfügbar.
Introduction xv
Chapter 1. Methodology of Modeling in Biology and Ecology 1
1.1. Models and modeling 1
1.1.1. Models 2
1.1.2. Modeling 4
1.2. Mathematical modeling 6
1.2.1. Analysis of the biological situation and problem 7
1.2.2. Characterization and analysis of the system 11
1.2.3. Choice or construction of a model 14
1.2.4. Study of the properties of the model 18
1.2.5. Identification 25
1.2.6. Validation 26
1.2.7. Use 31
1.2.8. Conclusion 32
1.3. Supplements 33
1.3.1. Differences between a mathematical object and a mathematical
model 33
1.3.2. Different types of objects and formalizations used in mathematical
modeling 34
1.3.3. Elements for choosing a mathematical formalism 36
1.3.4. Stochastic and deterministic approaches 37
1.3.5. Discrete and continuous time 39
1.3.6. Biological and physical variables 39
1.3.7. The quantitative - qualitative debate 40
1.4. Models and modeling in life sciences 41
1.4.1. Historical overview 42
1.4.2. Modeling in biological disciplines 46
1.4.3. Modeling in population biology and ecology 47
1.4.4. Actors 48
1.4.5. Modeling and informatics 49
1.4.6. Definition of bioinformatics 49
1.5. A brief history of ecology and the importance of models in this
discipline 51
1.6. Systems: a unifying concept 56
Chapter 2. Functional Representations: Construction and Interpretation of
Mathematical Models 59
2.1. Introduction 60
2.2. Box and arrow diagrams: compartmental models 62
2.3. Representations based on Forrester diagrams 65
2.4. "Chemical-type" representation and multilinear differential models 66
2.4.1. General overview of the translation algorithm 67
2.4.2. Example of the logistic model 71
2.4.3. Saturation phenomena 73
2.5. Functional representations of models in population dynamics 76
2.5.1. Single population model 76
2.5.2. Models with two interacting populations 79
2.6. General points on functional representations and the interpretation of
differential models 84
2.6.1. General hypotheses 84
2.6.2. Interpretation: phenomenological and mechanistic aspects,
superficial knowledge and deep knowledge 85
2.6.3. Towards a classification of differential and integro-differential
models of population dynamics 86
2.7. Conclusion 87
Chapter 3. Growth Models - Population Dynamics and Genetics 89
3.1. The biological processes of growth 90
3.2. Experimental data 93
3.2.1. Organism growth data 93
3.2.2. Data relating to population growth 96
3.3. Models 98
3.3.1. Questions and uses of models 99
3.3.2. Some examples of classic growth models 100
3.4. Growth modeling and functional representations 104
3.4.1. Quantitative aspects 106
3.4.2. Qualitative aspects: choice and construction of models 107
3.4.3. Functional representations and growth models 107
3.4.4. Examples of the construction of new models 110
3.4.5. Typology of growth models 115
3.5. Growth of organisms: some examples 117
3.5.1. Individual growth of the European herring gull, Larus argentatus 117
3.5.2. Individual growth of young muskrats, Ondatra zibethica 118
3.5.3. Growth of a tree in a forest: examples of the application of
individual growth models 124
3.5.4. Human growth 132
3.6. Models of population dynamics 133
3.6.1. Examples of growth models for bacterial populations: the exponential
model, the logistic model, the Monod model and the Contois model 133
3.6.2. Dynamics of biodiversity at a geological level 146
3.7. Discrete time elementary demographic models 153
3.7.1. A discrete time demographic model of microbial populations 153
3.7.2. The Fibonacci model 155
3.7.3. Lindenmayer systems as demographic models 157
3.7.4. Examples of branching processes 164
3.7.5. Evolution of the "Grand Paradis" ibex population 170
3.7.6. Conclusion 172
3.8. Continuous time model of the age structure of a population 173
3.9. Spatialized dynamics: example of fishing populations and the
regulation of sea-fishing 174
3.10. Evolution of the structure of an autogamous diploid population 175
3.10.1. The Mendelian system 176
3.10.2. Genetic evolution of an autogamous population 177
Chapter 4. Models of the Interaction Between Populations 183
4.1. The Volterra-Kostitzin model: an example of use in molecular biology.
Dynamics of RNA populations 184
4.1.1. Experimental data 185
4.1.2. Elements of qualitative analysis using the Kostitzin model 187
4.1.3. Initial data 190
4.1.4. Estimation of parameters and analysis of results 190
4.2. Models of competition between populations 193
4.2.1. The differential system 194
4.2.2. Description of competition using functional representations 198
4.2.3. Application to the study of competition between Fusarium populations
in soil 203
4.2.4. Theoretical study of competition in an open system 207
4.2.5. Competition in a variable environment 210
4.3. Predator-prey systems 217
4.3.1. The basic model (model I) 218
4.3.2. Model in a limited environment (model II) 222
4.3.3. Model with limited capacities of assimilation of prey by the
predator (model III) 227
4.3.4. Model with variable limited capacities for assimilation of prey by
the predator 233
4.3.5. Model with limited capacities for assimilation of prey by the
predator and spatial heterogeneity 234
4.3.6. Population dynamics of Rhizobium japonicum in soil 237
4.3.7. Predation of Rhizobium japonicum by amoeba in soil 239
4.4. Modeling the process of nitrification by microbial populations in
soil: an example of succession 241
4.4.1. Introduction 241
4.4.2. Experimental procedure 243
4.4.3. Construction of the model - identification 244
4.4.4. Results 248
4.4.5. Discussion and conclusion 249
4.5. Conclusion and other details 251
Chapter 5. Compartmental Models¿253
5.1. Diagrammatic representations and associated mathematical models 256
5.1.1. Diagrammatic representations 256
5.1.2. Mathematical models 257
5.2. General autonomous compartmental models 265
5.2.1. Catenary systems 266
5.2.2. Looped systems 267
5.2.3. Mammillary systems 268
5.2.4. Systems representing spatial processes 268
5.2.5. General representation of an autonomous compartmental system 269
5.3. Estimation of model parameters 272
5.3.1. Least squares method (elementary principles) 272
5.3.2. Study of sensitivity functions - optimization of the experimental
procedure 274
5.4. Open systems 274
5.4.1. The single compartment 274
5.4.2. The single compartment with input and output 275
5.5. General open compartmental models 278
5.6. Controllabillity, observability and identifiability of a compartmental
system 280
5.6.1. Controllabillity, observability and identifiability 280
5.6.2. Applications of these notions 281
5.7. Other mathematical models 282
5.8. Examples and additional information 283
5.8.1. Model of a single compartment system: application to the definition
of optimal posology 283
5.8.2. Reversible two-compartment system 287
5.8.3. Estimation of tracer waiting time in cellular structures 293
5.8.4. Example of construction of the diffusion equation 300
Chapter 6. Complexity, Scales, Chaos, Chance and Other Oddities 305
6.1. Complexity 307
6.1.1. Some aspects of word use for complex and complexity 308
6.1.2. Biodiversity and complexity towards a unifying theory of
biodiversity? 325
6.1.3. Random, logical, structural and dynamic complexity 328
6.2. Nonlinearities, temporal and spatial scales, the concept of
equilibrium and its avatars 331
6.2.1. Time and spatial scales 335
6.2.2. About the concept of equilibrium 337
6.2.3. Transitions between attractors: are the bifurcations
predictable? 342
6.3. The modeling of complexity 344
6.3.1. Complex dynamics: the example of deterministic chaos 344
6.3.2. Dynamics of complex systems and their structure 352
6.3.3. Shapes and morphogenesis - spatial structure dynamics: Lindenmayer
systems, fractals and cellular automata 358
6.3.4. Random behavior 369
6.4. Conclusion 371
6.4.1. Chance and complexity 371
6.4.2. The modeling approach 375
6.4.3. Problems linked to predictions 378
APPENDICES 383
Appendix 1. Differential Equations 385
Appendix 2. Recurrence Equations 465
Appendix 3. Fitting a Model to Experimental Results 489
Appendix 4. Introduction to Stochastic Processes 561
Bibliography 597
Index 617
Introduction xv
Chapter 1. Methodology of Modeling in Biology and Ecology 1
1.1. Models and modeling 1
1.1.1. Models 2
1.1.2. Modeling 4
1.2. Mathematical modeling 6
1.2.1. Analysis of the biological situation and problem 7
1.2.2. Characterization and analysis of the system 11
1.2.3. Choice or construction of a model 14
1.2.4. Study of the properties of the model 18
1.2.5. Identification 25
1.2.6. Validation 26
1.2.7. Use 31
1.2.8. Conclusion 32
1.3. Supplements 33
1.3.1. Differences between a mathematical object and a mathematical
model 33
1.3.2. Different types of objects and formalizations used in mathematical
modeling 34
1.3.3. Elements for choosing a mathematical formalism 36
1.3.4. Stochastic and deterministic approaches 37
1.3.5. Discrete and continuous time 39
1.3.6. Biological and physical variables 39
1.3.7. The quantitative - qualitative debate 40
1.4. Models and modeling in life sciences 41
1.4.1. Historical overview 42
1.4.2. Modeling in biological disciplines 46
1.4.3. Modeling in population biology and ecology 47
1.4.4. Actors 48
1.4.5. Modeling and informatics 49
1.4.6. Definition of bioinformatics 49
1.5. A brief history of ecology and the importance of models in this
discipline 51
1.6. Systems: a unifying concept 56
Chapter 2. Functional Representations: Construction and Interpretation of
Mathematical Models 59
2.1. Introduction 60
2.2. Box and arrow diagrams: compartmental models 62
2.3. Representations based on Forrester diagrams 65
2.4. "Chemical-type" representation and multilinear differential models 66
2.4.1. General overview of the translation algorithm 67
2.4.2. Example of the logistic model 71
2.4.3. Saturation phenomena 73
2.5. Functional representations of models in population dynamics 76
2.5.1. Single population model 76
2.5.2. Models with two interacting populations 79
2.6. General points on functional representations and the interpretation of
differential models 84
2.6.1. General hypotheses 84
2.6.2. Interpretation: phenomenological and mechanistic aspects,
superficial knowledge and deep knowledge 85
2.6.3. Towards a classification of differential and integro-differential
models of population dynamics 86
2.7. Conclusion 87
Chapter 3. Growth Models - Population Dynamics and Genetics 89
3.1. The biological processes of growth 90
3.2. Experimental data 93
3.2.1. Organism growth data 93
3.2.2. Data relating to population growth 96
3.3. Models 98
3.3.1. Questions and uses of models 99
3.3.2. Some examples of classic growth models 100
3.4. Growth modeling and functional representations 104
3.4.1. Quantitative aspects 106
3.4.2. Qualitative aspects: choice and construction of models 107
3.4.3. Functional representations and growth models 107
3.4.4. Examples of the construction of new models 110
3.4.5. Typology of growth models 115
3.5. Growth of organisms: some examples 117
3.5.1. Individual growth of the European herring gull, Larus argentatus 117
3.5.2. Individual growth of young muskrats, Ondatra zibethica 118
3.5.3. Growth of a tree in a forest: examples of the application of
individual growth models 124
3.5.4. Human growth 132
3.6. Models of population dynamics 133
3.6.1. Examples of growth models for bacterial populations: the exponential
model, the logistic model, the Monod model and the Contois model 133
3.6.2. Dynamics of biodiversity at a geological level 146
3.7. Discrete time elementary demographic models 153
3.7.1. A discrete time demographic model of microbial populations 153
3.7.2. The Fibonacci model 155
3.7.3. Lindenmayer systems as demographic models 157
3.7.4. Examples of branching processes 164
3.7.5. Evolution of the "Grand Paradis" ibex population 170
3.7.6. Conclusion 172
3.8. Continuous time model of the age structure of a population 173
3.9. Spatialized dynamics: example of fishing populations and the
regulation of sea-fishing 174
3.10. Evolution of the structure of an autogamous diploid population 175
3.10.1. The Mendelian system 176
3.10.2. Genetic evolution of an autogamous population 177
Chapter 4. Models of the Interaction Between Populations 183
4.1. The Volterra-Kostitzin model: an example of use in molecular biology.
Dynamics of RNA populations 184
4.1.1. Experimental data 185
4.1.2. Elements of qualitative analysis using the Kostitzin model 187
4.1.3. Initial data 190
4.1.4. Estimation of parameters and analysis of results 190
4.2. Models of competition between populations 193
4.2.1. The differential system 194
4.2.2. Description of competition using functional representations 198
4.2.3. Application to the study of competition between Fusarium populations
in soil 203
4.2.4. Theoretical study of competition in an open system 207
4.2.5. Competition in a variable environment 210
4.3. Predator-prey systems 217
4.3.1. The basic model (model I) 218
4.3.2. Model in a limited environment (model II) 222
4.3.3. Model with limited capacities of assimilation of prey by the
predator (model III) 227
4.3.4. Model with variable limited capacities for assimilation of prey by
the predator 233
4.3.5. Model with limited capacities for assimilation of prey by the
predator and spatial heterogeneity 234
4.3.6. Population dynamics of Rhizobium japonicum in soil 237
4.3.7. Predation of Rhizobium japonicum by amoeba in soil 239
4.4. Modeling the process of nitrification by microbial populations in
soil: an example of succession 241
4.4.1. Introduction 241
4.4.2. Experimental procedure 243
4.4.3. Construction of the model - identification 244
4.4.4. Results 248
4.4.5. Discussion and conclusion 249
4.5. Conclusion and other details 251
Chapter 5. Compartmental Models¿253
5.1. Diagrammatic representations and associated mathematical models 256
5.1.1. Diagrammatic representations 256
5.1.2. Mathematical models 257
5.2. General autonomous compartmental models 265
5.2.1. Catenary systems 266
5.2.2. Looped systems 267
5.2.3. Mammillary systems 268
5.2.4. Systems representing spatial processes 268
5.2.5. General representation of an autonomous compartmental system 269
5.3. Estimation of model parameters 272
5.3.1. Least squares method (elementary principles) 272
5.3.2. Study of sensitivity functions - optimization of the experimental
procedure 274
5.4. Open systems 274
5.4.1. The single compartment 274
5.4.2. The single compartment with input and output 275
5.5. General open compartmental models 278
5.6. Controllabillity, observability and identifiability of a compartmental
system 280
5.6.1. Controllabillity, observability and identifiability 280
5.6.2. Applications of these notions 281
5.7. Other mathematical models 282
5.8. Examples and additional information 283
5.8.1. Model of a single compartment system: application to the definition
of optimal posology 283
5.8.2. Reversible two-compartment system 287
5.8.3. Estimation of tracer waiting time in cellular structures 293
5.8.4. Example of construction of the diffusion equation 300
Chapter 6. Complexity, Scales, Chaos, Chance and Other Oddities 305
6.1. Complexity 307
6.1.1. Some aspects of word use for complex and complexity 308
6.1.2. Biodiversity and complexity towards a unifying theory of
biodiversity? 325
6.1.3. Random, logical, structural and dynamic complexity 328
6.2. Nonlinearities, temporal and spatial scales, the concept of
equilibrium and its avatars 331
6.2.1. Time and spatial scales 335
6.2.2. About the concept of equilibrium 337
6.2.3. Transitions between attractors: are the bifurcations
predictable? 342
6.3. The modeling of complexity 344
6.3.1. Complex dynamics: the example of deterministic chaos 344
6.3.2. Dynamics of complex systems and their structure 352
6.3.3. Shapes and morphogenesis - spatial structure dynamics: Lindenmayer
systems, fractals and cellular automata 358
6.3.4. Random behavior 369
6.4. Conclusion 371
6.4.1. Chance and complexity 371
6.4.2. The modeling approach 375
6.4.3. Problems linked to predictions 378
APPENDICES 383
Appendix 1. Differential Equations 385
Appendix 2. Recurrence Equations 465
Appendix 3. Fitting a Model to Experimental Results 489
Appendix 4. Introduction to Stochastic Processes 561
Bibliography 597
Index 617