Multimodal Intelligent Sensing in Modern Applications (eBook, PDF)
Redaktion: Ur Rehman, Masood; Ramzan, Naeem; Jamshed, Muhammad Ali; Zoha, Ahmed
126,99 €
126,99 €
inkl. MwSt.
Sofort per Download lieferbar
0 °P sammeln
126,99 €
Als Download kaufen
126,99 €
inkl. MwSt.
Sofort per Download lieferbar
0 °P sammeln
Jetzt verschenken
Alle Infos zum eBook verschenken
126,99 €
inkl. MwSt.
Sofort per Download lieferbar
Alle Infos zum eBook verschenken
0 °P sammeln
Multimodal Intelligent Sensing in Modern Applications (eBook, PDF)
Redaktion: Ur Rehman, Masood; Ramzan, Naeem; Jamshed, Muhammad Ali; Zoha, Ahmed
- Format: PDF
- Merkliste
- Auf die Merkliste
- Bewerten Bewerten
- Teilen
- Produkt teilen
- Produkterinnerung
- Produkterinnerung
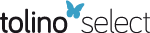
Bitte loggen Sie sich zunächst in Ihr Kundenkonto ein oder registrieren Sie sich bei
bücher.de, um das eBook-Abo tolino select nutzen zu können.
Hier können Sie sich einloggen
Hier können Sie sich einloggen
Sie sind bereits eingeloggt. Klicken Sie auf 2. tolino select Abo, um fortzufahren.
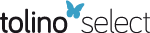
Bitte loggen Sie sich zunächst in Ihr Kundenkonto ein oder registrieren Sie sich bei bücher.de, um das eBook-Abo tolino select nutzen zu können.
Discover the design, implementation, and analytical techniques for multi-modal intelligent sensing in this cutting-edge text
The Internet of Things (IoT) is becoming ever more comprehensively integrated into everyday life. The intelligent systems that power smart technologies rely on increasingly sophisticated sensors in order to monitor inputs and respond dynamically. Multi-modal sensing offers enormous benefits for these technologies, but also comes with greater challenges; it has never been more essential to offer energy-efficient, reliable, interference-free sensing systems for use…mehr
- Geräte: PC
- mit Kopierschutz
- eBook Hilfe
- Größe: 5MB
Andere Kunden interessierten sich auch für
- Internet of Energy Handbook (eBook, PDF)84,95 €
- Mohamed M. AtiaSensor Fusion Approaches for Positioning, Navigation, and Mapping (eBook, PDF)111,99 €
- Micro Electromechanical Systems (MEMS) (eBook, PDF)90,99 €
- Sensing Technologies for Real Time Monitoring of Water Quality (eBook, PDF)103,99 €
- Baljinder KaurBiosensors (eBook, PDF)112,99 €
- Electronically Scanned Arrays MATLAB® Modeling and Simulation (eBook, PDF)88,95 €
- Distributed Control Applications (eBook, PDF)80,95 €
-
-
-
Discover the design, implementation, and analytical techniques for multi-modal intelligent sensing in this cutting-edge text
The Internet of Things (IoT) is becoming ever more comprehensively integrated into everyday life. The intelligent systems that power smart technologies rely on increasingly sophisticated sensors in order to monitor inputs and respond dynamically. Multi-modal sensing offers enormous benefits for these technologies, but also comes with greater challenges; it has never been more essential to offer energy-efficient, reliable, interference-free sensing systems for use with the modern Internet of Things.
Multimodal Intelligent Sensing in Modern Applications provides an introduction to systems which incorporate multiple sensors to produce situational awareness and process inputs. It is divided into three parts-physical design aspects, data acquisition and analysis techniques, and security and energy challenges-which together cover all the major topics in multi-modal sensing. The result is an indispensable volume for engineers and other professionals looking to design the smart devices of the future.
Multimodal Intelligent Sensing in Modern Applications readers will also find:
Multimodal Intelligent Sensing in Modern Applications is ideal for experienced engineers and designers who need to apply their skills to Internet of Things and 5G/6G networks. It can also act as an introductory text for graduate researchers into understanding the background, design, and implementation of various sensor types and data analytics tools.
The Internet of Things (IoT) is becoming ever more comprehensively integrated into everyday life. The intelligent systems that power smart technologies rely on increasingly sophisticated sensors in order to monitor inputs and respond dynamically. Multi-modal sensing offers enormous benefits for these technologies, but also comes with greater challenges; it has never been more essential to offer energy-efficient, reliable, interference-free sensing systems for use with the modern Internet of Things.
Multimodal Intelligent Sensing in Modern Applications provides an introduction to systems which incorporate multiple sensors to produce situational awareness and process inputs. It is divided into three parts-physical design aspects, data acquisition and analysis techniques, and security and energy challenges-which together cover all the major topics in multi-modal sensing. The result is an indispensable volume for engineers and other professionals looking to design the smart devices of the future.
Multimodal Intelligent Sensing in Modern Applications readers will also find:
- Contributions from multidisciplinary contributors in wireless communications, signal processing, and sensor design
- Coverage of both software and hardware solutions to sensing challenges
- Detailed treatment of advanced topics such as efficient deployment, data fusion, machine learning, and more
Multimodal Intelligent Sensing in Modern Applications is ideal for experienced engineers and designers who need to apply their skills to Internet of Things and 5G/6G networks. It can also act as an introductory text for graduate researchers into understanding the background, design, and implementation of various sensor types and data analytics tools.
Dieser Download kann aus rechtlichen Gründen nur mit Rechnungsadresse in D ausgeliefert werden.
Produktdetails
- Produktdetails
- Verlag: Jossey-Bass
- Seitenzahl: 320
- Erscheinungstermin: 27. Dezember 2024
- Englisch
- ISBN-13: 9781394257737
- Artikelnr.: 72710596
- Verlag: Jossey-Bass
- Seitenzahl: 320
- Erscheinungstermin: 27. Dezember 2024
- Englisch
- ISBN-13: 9781394257737
- Artikelnr.: 72710596
- Herstellerkennzeichnung Die Herstellerinformationen sind derzeit nicht verfügbar.
Masood Ur Rehman, PhD, MSc, is a Senior Lecturer at the James Watt School of Engineering, University of Glasgow, UK and leads the Antennas & Radio-wave Propagation group. He received his MSc and PhD in Electronic Engineering from Queen Mary University of London, UK, in 2006 and 2010, respectively. Ahmed Zoha, PhD, MSc, is a Senior Lecturer at the James Watt School of Engineering, University of Glasgow, UK and leads the Distributed Learning and Intelligence group. He received his PhD degree in Electrical and Electronic Engineering from the 5G Innovation Centre at the University of Surrey, UK, and his MSc in Communication Engineering from the Chalmers University of Technology, Sweden. Muhammad Ali Jamshed, PhD, MSc, is with University of Glasgow, UK, since 2021. He is a visiting Research Fellow at the University of Sussex. He is endorsed by the Royal Academy of Engineering under exceptional talent category and was nominated for the Departmental Prize for Excellence in Research in 2019 at the University of Surrey. Naeem Ramzan, PhD, is a Full Professor in Computing Engineering and Chair of Affective and Human Computing for Smart Environment Research Centre and Co-lead of Visual Communication Cluster in AVCN at the University of the West of Scotland, Paisley, UK. He received his PhD in Electronic Engineering from Queen Mary University of London, UK in 2008.
Contents
About the Editors xv
List of Contributors xix
Preface xxiii
1 Advances in Multi-modal Intelligent Sensing 1
Masood Ur Rehman, Muhammad Ali Jamshed, and Tahera Kalsoom
1.1 Multi-modal Intelligent Sensing 1
1.2 Sensors for Multi-modal Intelligent Sensing 3
1.2.1 Sensor Types 3
1.2.2 Integration of Multiple Sensor Types for Enhanced Sensing
Capabilities 5
1.2.2.1 Advantages of Multiple Sensor Integration 5
1.2.2.2 Key Considerations for Multiple Sensor Integration 6
1.2.2.3 Concurrent Data Acquisition Methods 9
1.2.2.4 Data Analysis Tools for Multi-modal Sensing 11
1.2.2.5 Considerations for Data Fusion and Synchronization 13
1.3 Applications of Multi-modal Intelligent Sensing 14
1.3.1 Healthcare and Medical Monitoring 14
1.3.2 Automotive and Transportation Systems 15
1.3.3 Environmental Monitoring and Conservation 16
1.3.4 Smart Cities and Infrastructure Management 17
1.3.5 Industrial Automation 18
1.4 Challenges and Opportunities in Multi-modal Sensing 18
1.4.1 Data Security and Privacy 19
1.4.2 Interoperability and Standardization 19
1.4.3 Energy Efficiency and Power Management 20
1.4.4 Coverage 21
1.4.5 Summary 21
References 22
2 Antennas for Wireless Sensors 29
Abdul Jabbar, Muhammad Ali Jamshed, and Masood Ur Rehman
2.1 Wireless Sensors: Definition and Architecture 29
2.1.1 Wireless Sensor Node Architecture 30
2.1.2 Operating Systems 32
2.1.3 Classification of Wireless Sensors 32
2.2 Multi-modal Wireless Sensing 34
2.3 Antennas: The Sensory Gateway for Wireless Sensors 35
2.4 Fundamental Antenna Parameters 36
2.4.1 Bandwidth and Operating Frequency 36
2.4.2 Gain 37
2.4.3 Radiation Pattern 37
2.4.4 Polarization 38
2.5 Key Operating Frequency Bands for Sensing Antennas 39
2.6 Fabrication Methods for Sensing Antennas 40
2.6.1 Printed Circuit Board (PCB) Antennas 40
2.6.2 On-Chip and Integrated Antenna Fabrication 41
2.6.3 Stitching and Embroidery for Flexible Textile Antennas 41
2.7 Antenna Types for Wireless Sensing Networks 42
2.7.1 Flexible Antennas 43
2.7.2 Omnidirectional Antennas 45
2.7.3 Directional Antennas 46
2.8 Advantages of Electronic Beamsteering Antennas in Sensing Systems 46
2.9 Summary 49
References 49
3 Sensor Design for Multimodal Environmental Monitoring 55
Muhammad Ali Jamshed, Bushra Haq, Syed Ahmed Shah, Kamran Ali, Qammer H.
Abbasi, Mumraiz Khan Kasi, and Masood Ur Rehman
3.1 Environment and Forests 56
3.2 Methods to Combat Deforestation 56
3.2.1 Combating Deforestation Using Wireless Sensor Networks 57
3.2.2 Sensor Types for Combating Deforestation 58
3.3 Design of a WSN to Combat Deforestation 59
3.3.1 Stage 1: System Requirements 59
3.3.1.1 Key Performance Indicators 62
3.3.2 Stage 2: Architecture 63
3.3.3 Stage 3: System Implementation 65
3.3.3.1 Type of Sensors 65
3.3.3.2 Processing Boards 66
3.3.3.3 Communication Modules 67
3.3.3.4 Batteries 67
3.3.3.5 Energy Harvesting Circuit and Equipment 69
3.3.3.6 Weather Protection 70
3.3.3.7 Wireless Communication Link 71
3.3.3.8 Data Processing Algorithms 74
3.4 Summary 76
References 76
4 Wireless Sensors for Multi-modal Health Monitoring 81
Nadeem Ajum, Shagufta Iftikhar, Tahera Kalsoom, and Masood Ur Rehman
4.1 Wearable Sensors 82
4.1.1 Electrocardiography (ECG) Sensors 83
4.1.2 Electroencephalography (EEG) Sensors 83
4.1.3 Electrooculography (EOG) Sensors 84
4.1.4 Electrodermal Activity (EDA) Sensors 86
4.1.5 Respiratory (RESP) Sensors 86
4.1.6 Motion Sensors 86
4.1.7 Temperature (TEMP) Sensors 87
4.1.8 Pressure Sensors 87
4.1.9 Hydration Sensors 88
4.1.10 Lactate Sensors 88
4.1.11 Photoplethysmography (PPG) Sensors 89
4.1.12 Continuous Glucose Monitoring (CGM) Sensors 89
4.2 Flexible Sensors 89
4.3 Multi-modal Healthcare Sensing Devices 90
4.3.1 Wearable Sensing Devices for Healthcare 90
4.3.1.1 Wearable Devices in Detection 90
4.3.1.2 Wearable Devices in Monitoring 92
4.3.1.3 Wearable Devices in Rehabilitation 93
4.3.1.4 Wearable Devices in Personalized Medicine 94
4.3.1.5 Wearable Devices in Skin Patches 94
4.3.1.6 Wearable Devices for Body Fluid Monitoring 95
4.3.1.7 Wearable Devices in Monitoring Body Temperature 96
4.3.1.8 Wearable Devices as Contact Lens 96
4.3.1.9 Wearable Devices in Daily Use Objects 97
4.3.2 Implantable Sensing Devices for Healthcare 97
4.3.2.1 Implantable Cardioverter Defibrillators 98
4.3.2.2 Bioinks and 3D Print Implants 98
4.3.2.3 Deep Brain Stimulation 99
4.3.2.4 Biosensor Tattoos 99
4.4 AI Methods for Multi-modal Healthcare Systems 100
4.4.1 Supervised Learning 100
4.4.2 Unsupervised Learning 101
4.4.3 Semi-supervised Learning 101
4.4.4 Reinforcement Learning 102
4.5 Summary 102
References 103
5 Sensor Design for Industrial Automation 109
Abdul Jabbar, Tahera Kalsoom, and Masood Ur Rehman
5.1 Multimodal Sensing in Industrial Automation 109
5.1.1 IIoT and Multimodal Sensing 111
5.1.2 Advanced Robotics 113
5.1.3 Big Data Analytics 114
5.1.4 Cloud Computing 114
5.1.5 Artificial Intelligence 115
5.1.6 Augmented Reality 116
5.2 Sensors for Realizing Industrial Automation 116
5.2.1 RF Sensors 117
5.2.2 Vision Sensors 118
5.2.3 Localization and Tracking Sensors 119
5.2.4 Infrared Sensors 119
5.2.5 Proximity Sensors 119
5.2.6 IMU Sensors 120
5.2.7 Level Sensors 120
5.2.8 Temperature Sensors 120
5.2.9 Pressure Sensors 121
5.3 Design Considerations for Effective Multimodal Industrial Automation
121
5.3.1 Design of AI-Assisted Multimodal Sensing 122
5.3.2 Design of Radar Sensing Networks 123
5.3.2.1 Transmitter and Receiver Antennas 123
5.3.2.2 Data Collection and Interface 123
5.3.2.3 Signal Processing 124
5.3.2.4 Housing and Enclosure 124
5.4 Challenges and Opportunities of Multimodal Sensing in Industrial
Automation 124
5.5 Summary 126
References 126
6 Hybrid Neuromorphic-Federated Learning for Activity Recognition Using
Multi-modal Wearable Sensors 133
Ahsan Raza Khan, Habib Ullah Manzoor, Fahad Ayaz, Muhammad Ali Imran, and
Ahmed Zoha
6.1 Multi-modal Human Activity Recognition 134
6.2 Machine Learning Methods in Multi-modal Human Activity Recognition 137
6.2.1 Centralized Learning-based HAR Systems 137
6.2.2 Federated Learning-based HAR Systems 138
6.3 System Model 139
6.3.1 Federated Learning Framework 140
6.3.2 Spiking Neural Network 141
6.3.3 Proposed S-LSTM Model 144
6.4 Simulation Setup 146
6.4.1 Dataset Description 146
6.4.1.1 UCI Dataset 147
6.4.1.2 Real-World Dataset 148
6.4.2 Performance Metrics 149
6.5 Results and Discussion 150
6.5.1 UCI Results 151
6.5.2 Real-World Dataset Results 154
6.5.3 Energy Efficiency Comparison 157
6.5.4 Personalized Model Comparison 159
6.6 Summary 159
References 161
7 Multi-modal Beam Prediction for Enhanced Beam Management in Drone
Communication Networks 165
Iftikhar Ahmad, Ahsan Raza Khan, Rao Naveed Bin Rais, Muhammad Ali Imran,
Sajjad Hussain, and Ahmed Zoha
7.1 Drone Communication 166
7.2 Beam Management 167
7.3 System Model 168
7.3.1 Problem Formulation for Beam Prediction 170
7.3.2 Proposed Stacked Vision-Assisted Beam Prediction Model 170
7.4 Simulation and Analysis 171
7.4.1 Description of the Dataset 173
7.4.2 Configuration for Simulation 173
7.4.2.1 YOLO-v5 Training Process 174
7.4.3 Results and Analysis 175
7.5 Summary 178
References 178
8 Multi-modal-Sensing System for Detection and Tracking of Mind Wandering
181
Sara Khosravi, Haobo Li, Ahsan Raza Khan, Ahmed Zoha, and Rami Ghannam
8.1 Mind Wandering 182
8.2 Multi-modal Wearable Systems for Mind-Wandering Detection and
Monitoring 184
8.2.1 Wearable Eye Trackers for Gaze Measurements 185
8.2.2 Wearable GSR and PPG Sensors for Physiological Measurements 186
8.3 Design of Multi-modal Wearable System 187
8.3.1 Selection of Sensor 187
8.3.2 Selection of Participant Groups and Testing Environment 188
8.3.3 Data Collection Process 189
8.3.4 Machine Learning and Multisensory Fusion 190
8.4 Results and Discussion 194
8.5 Summary 197
References 197
9 Adaptive Secure Multi-modal Telehealth Patient-Monitoring System 201
Muhammad Hanif , Ehsan Ullah Munir, Muhammad Maaz Rehan, Saima Gulzar
Ahmad, Tassawar Iqbal, Nasira Kirn, Kashif Ayyub, and Naeem Ramzan
9.1 Healthcare Systems 202
9.1.1 Traditional Healthcare Systems 203
9.1.2 Multi-modal Telehealth Systems 203
9.2 Security in Healthcare Systems 205
9.2.1 Prevailing Techniques for Secure Telehealth 205
9.2.2 Challenges in Ensuring Healthcare Security 208
9.2.3 Strategies to Enhance Healthcare Data Security 209
9.2.4 Key Security Features for Telehealth Systems 210
9.2.4.1 Encryption 210
9.2.4.2 Authentication 211
9.2.4.3 Access Control 211
9.2.4.4 Audit Trails 211
9.2.4.5 Data Integrity Checks 211
9.2.4.6 Secure Communication Protocols 211
9.2.4.7 Security Audits and Penetration Testing 211
9.2.4.8 Cyber Resilience 212
9.2.4.9 Zero-Trust-Based Micro-segmentation 212
9.3 Blockchain-Powered ZTS for Enhanced Security of Telehealth Systems 213
9.3.1 Zero-Trust Security 213
9.3.2 Blockchain 214
9.4 Cyber-resilient Telehealth-Enabled Patient Management System 217
9.4.1 Assessment and Planning 218
9.4.2 Infrastructure Setup 219
9.4.3 Security Controls Implementation 219
9.4.4 Training and Awareness 219
9.4.5 Advantages and Limitations 221
9.5 Summary 222
References 222
10 Advances in Multi-modal Remote Infant Monitoring Systems 227
Najia Saher, Omer Riaz, Muhammad Suleman, Dost Muhammad Khan, Nasira Kirn,
Sana Ullah Jan, Rizwan Shahid, Hassan Rabah, and Naeem Ramzan
10.1 Remote Patient Monitoring 228
10.2 Remote Infant Monitoring (RIM) System 229
10.2.1 Contactless Remote Patient Monitoring (RPM) Systems 230
10.2.2 Contact-Based Remote Patient Monitoring (RPM) Systems 230
10.3 Disease-Specific Remote Infant Monitoring Systems 232
10.3.1 Respiration and Apnea Monitoring System 232
10.3.1.1 Emerging Sensing Techniques for Respiratory Diseases 233
10.3.2 Heart and Blood-Related Diseases Monitoring Systems 238
10.3.2.1 Emerging Sensing Techniques for Heart and Blood Diseases 238
10.3.3 Infant Monitoring Systems for Various Diseases 241
10.4 Challenges in Remote Infant Monitoring Systems 241
10.5 Summary 245
References 246
11 Balancing Innovation with Ethics: Responsible Development of Multi-modal
Intelligent Tutoring Systems 253
Romina Soledad Albornoz-De Luise, Pablo Arnau-González, Ana
Serrano-Mamolar, Sergi Solera-Monforte, and Yuyan Wu
11.1 Intelligent Tutoring Systems and Ethical Considerations 253
11.2 The Promise and Perils of ITS 255
11.2.1 Advantages of ITS in Education 255
11.2.1.1 Personalized Learning 255
11.2.1.2 Increased Accessibility 256
11.2.1.3 Continuous Assessment and Feedback 256
11.2.2 Potential Risks and Challenges 256
11.2.2.1 Bias in Algorithms 257
11.2.2.2 Privacy Concerns 257
11.2.2.3 Socioeconomic Disparities in Access 257
11.2.2.4 Dependency on Technology 258
11.3 Ethical Frameworks for ITS 258
11.3.1 Utilitarian Perspective 258
11.3.2 Deontological Perspective 259
11.3.3 Virtue Ethics 260
11.4 Bias and Fairness in ITS 261
11.5 Privacy and Security Concerns 263
11.5.1 Self-Reported Sources 263
11.5.2 Captured Data Sources 264
11.6 Socioeconomic Disparities in Access 265
11.7 Dependency on Technology 267
11.8 Summary 268
References 269
12 Road Ahead for Multi-modal Intelligent Sensing in the Deep Learning Era
275
Ahmed Zoha, Naeem Ramzan, Muhammad Ali Jamshed, and Masood Ur Rehman
12.1 Future Challenges and Perspectives for Intelligent Multi-modal Sensing
276
12.1.1 Semantic Gaps and Cross-modality Representation 276
12.1.2 Concept Drift and Data Quality 278
12.1.3 Computational Demands and Model Scalability 279
12.1.4 Interpretability 280
12.1.5 Ethical Considerations 281
12.2 Summary 282
References 282
Index 285
About the Editors xv
List of Contributors xix
Preface xxiii
1 Advances in Multi-modal Intelligent Sensing 1
Masood Ur Rehman, Muhammad Ali Jamshed, and Tahera Kalsoom
1.1 Multi-modal Intelligent Sensing 1
1.2 Sensors for Multi-modal Intelligent Sensing 3
1.2.1 Sensor Types 3
1.2.2 Integration of Multiple Sensor Types for Enhanced Sensing
Capabilities 5
1.2.2.1 Advantages of Multiple Sensor Integration 5
1.2.2.2 Key Considerations for Multiple Sensor Integration 6
1.2.2.3 Concurrent Data Acquisition Methods 9
1.2.2.4 Data Analysis Tools for Multi-modal Sensing 11
1.2.2.5 Considerations for Data Fusion and Synchronization 13
1.3 Applications of Multi-modal Intelligent Sensing 14
1.3.1 Healthcare and Medical Monitoring 14
1.3.2 Automotive and Transportation Systems 15
1.3.3 Environmental Monitoring and Conservation 16
1.3.4 Smart Cities and Infrastructure Management 17
1.3.5 Industrial Automation 18
1.4 Challenges and Opportunities in Multi-modal Sensing 18
1.4.1 Data Security and Privacy 19
1.4.2 Interoperability and Standardization 19
1.4.3 Energy Efficiency and Power Management 20
1.4.4 Coverage 21
1.4.5 Summary 21
References 22
2 Antennas for Wireless Sensors 29
Abdul Jabbar, Muhammad Ali Jamshed, and Masood Ur Rehman
2.1 Wireless Sensors: Definition and Architecture 29
2.1.1 Wireless Sensor Node Architecture 30
2.1.2 Operating Systems 32
2.1.3 Classification of Wireless Sensors 32
2.2 Multi-modal Wireless Sensing 34
2.3 Antennas: The Sensory Gateway for Wireless Sensors 35
2.4 Fundamental Antenna Parameters 36
2.4.1 Bandwidth and Operating Frequency 36
2.4.2 Gain 37
2.4.3 Radiation Pattern 37
2.4.4 Polarization 38
2.5 Key Operating Frequency Bands for Sensing Antennas 39
2.6 Fabrication Methods for Sensing Antennas 40
2.6.1 Printed Circuit Board (PCB) Antennas 40
2.6.2 On-Chip and Integrated Antenna Fabrication 41
2.6.3 Stitching and Embroidery for Flexible Textile Antennas 41
2.7 Antenna Types for Wireless Sensing Networks 42
2.7.1 Flexible Antennas 43
2.7.2 Omnidirectional Antennas 45
2.7.3 Directional Antennas 46
2.8 Advantages of Electronic Beamsteering Antennas in Sensing Systems 46
2.9 Summary 49
References 49
3 Sensor Design for Multimodal Environmental Monitoring 55
Muhammad Ali Jamshed, Bushra Haq, Syed Ahmed Shah, Kamran Ali, Qammer H.
Abbasi, Mumraiz Khan Kasi, and Masood Ur Rehman
3.1 Environment and Forests 56
3.2 Methods to Combat Deforestation 56
3.2.1 Combating Deforestation Using Wireless Sensor Networks 57
3.2.2 Sensor Types for Combating Deforestation 58
3.3 Design of a WSN to Combat Deforestation 59
3.3.1 Stage 1: System Requirements 59
3.3.1.1 Key Performance Indicators 62
3.3.2 Stage 2: Architecture 63
3.3.3 Stage 3: System Implementation 65
3.3.3.1 Type of Sensors 65
3.3.3.2 Processing Boards 66
3.3.3.3 Communication Modules 67
3.3.3.4 Batteries 67
3.3.3.5 Energy Harvesting Circuit and Equipment 69
3.3.3.6 Weather Protection 70
3.3.3.7 Wireless Communication Link 71
3.3.3.8 Data Processing Algorithms 74
3.4 Summary 76
References 76
4 Wireless Sensors for Multi-modal Health Monitoring 81
Nadeem Ajum, Shagufta Iftikhar, Tahera Kalsoom, and Masood Ur Rehman
4.1 Wearable Sensors 82
4.1.1 Electrocardiography (ECG) Sensors 83
4.1.2 Electroencephalography (EEG) Sensors 83
4.1.3 Electrooculography (EOG) Sensors 84
4.1.4 Electrodermal Activity (EDA) Sensors 86
4.1.5 Respiratory (RESP) Sensors 86
4.1.6 Motion Sensors 86
4.1.7 Temperature (TEMP) Sensors 87
4.1.8 Pressure Sensors 87
4.1.9 Hydration Sensors 88
4.1.10 Lactate Sensors 88
4.1.11 Photoplethysmography (PPG) Sensors 89
4.1.12 Continuous Glucose Monitoring (CGM) Sensors 89
4.2 Flexible Sensors 89
4.3 Multi-modal Healthcare Sensing Devices 90
4.3.1 Wearable Sensing Devices for Healthcare 90
4.3.1.1 Wearable Devices in Detection 90
4.3.1.2 Wearable Devices in Monitoring 92
4.3.1.3 Wearable Devices in Rehabilitation 93
4.3.1.4 Wearable Devices in Personalized Medicine 94
4.3.1.5 Wearable Devices in Skin Patches 94
4.3.1.6 Wearable Devices for Body Fluid Monitoring 95
4.3.1.7 Wearable Devices in Monitoring Body Temperature 96
4.3.1.8 Wearable Devices as Contact Lens 96
4.3.1.9 Wearable Devices in Daily Use Objects 97
4.3.2 Implantable Sensing Devices for Healthcare 97
4.3.2.1 Implantable Cardioverter Defibrillators 98
4.3.2.2 Bioinks and 3D Print Implants 98
4.3.2.3 Deep Brain Stimulation 99
4.3.2.4 Biosensor Tattoos 99
4.4 AI Methods for Multi-modal Healthcare Systems 100
4.4.1 Supervised Learning 100
4.4.2 Unsupervised Learning 101
4.4.3 Semi-supervised Learning 101
4.4.4 Reinforcement Learning 102
4.5 Summary 102
References 103
5 Sensor Design for Industrial Automation 109
Abdul Jabbar, Tahera Kalsoom, and Masood Ur Rehman
5.1 Multimodal Sensing in Industrial Automation 109
5.1.1 IIoT and Multimodal Sensing 111
5.1.2 Advanced Robotics 113
5.1.3 Big Data Analytics 114
5.1.4 Cloud Computing 114
5.1.5 Artificial Intelligence 115
5.1.6 Augmented Reality 116
5.2 Sensors for Realizing Industrial Automation 116
5.2.1 RF Sensors 117
5.2.2 Vision Sensors 118
5.2.3 Localization and Tracking Sensors 119
5.2.4 Infrared Sensors 119
5.2.5 Proximity Sensors 119
5.2.6 IMU Sensors 120
5.2.7 Level Sensors 120
5.2.8 Temperature Sensors 120
5.2.9 Pressure Sensors 121
5.3 Design Considerations for Effective Multimodal Industrial Automation
121
5.3.1 Design of AI-Assisted Multimodal Sensing 122
5.3.2 Design of Radar Sensing Networks 123
5.3.2.1 Transmitter and Receiver Antennas 123
5.3.2.2 Data Collection and Interface 123
5.3.2.3 Signal Processing 124
5.3.2.4 Housing and Enclosure 124
5.4 Challenges and Opportunities of Multimodal Sensing in Industrial
Automation 124
5.5 Summary 126
References 126
6 Hybrid Neuromorphic-Federated Learning for Activity Recognition Using
Multi-modal Wearable Sensors 133
Ahsan Raza Khan, Habib Ullah Manzoor, Fahad Ayaz, Muhammad Ali Imran, and
Ahmed Zoha
6.1 Multi-modal Human Activity Recognition 134
6.2 Machine Learning Methods in Multi-modal Human Activity Recognition 137
6.2.1 Centralized Learning-based HAR Systems 137
6.2.2 Federated Learning-based HAR Systems 138
6.3 System Model 139
6.3.1 Federated Learning Framework 140
6.3.2 Spiking Neural Network 141
6.3.3 Proposed S-LSTM Model 144
6.4 Simulation Setup 146
6.4.1 Dataset Description 146
6.4.1.1 UCI Dataset 147
6.4.1.2 Real-World Dataset 148
6.4.2 Performance Metrics 149
6.5 Results and Discussion 150
6.5.1 UCI Results 151
6.5.2 Real-World Dataset Results 154
6.5.3 Energy Efficiency Comparison 157
6.5.4 Personalized Model Comparison 159
6.6 Summary 159
References 161
7 Multi-modal Beam Prediction for Enhanced Beam Management in Drone
Communication Networks 165
Iftikhar Ahmad, Ahsan Raza Khan, Rao Naveed Bin Rais, Muhammad Ali Imran,
Sajjad Hussain, and Ahmed Zoha
7.1 Drone Communication 166
7.2 Beam Management 167
7.3 System Model 168
7.3.1 Problem Formulation for Beam Prediction 170
7.3.2 Proposed Stacked Vision-Assisted Beam Prediction Model 170
7.4 Simulation and Analysis 171
7.4.1 Description of the Dataset 173
7.4.2 Configuration for Simulation 173
7.4.2.1 YOLO-v5 Training Process 174
7.4.3 Results and Analysis 175
7.5 Summary 178
References 178
8 Multi-modal-Sensing System for Detection and Tracking of Mind Wandering
181
Sara Khosravi, Haobo Li, Ahsan Raza Khan, Ahmed Zoha, and Rami Ghannam
8.1 Mind Wandering 182
8.2 Multi-modal Wearable Systems for Mind-Wandering Detection and
Monitoring 184
8.2.1 Wearable Eye Trackers for Gaze Measurements 185
8.2.2 Wearable GSR and PPG Sensors for Physiological Measurements 186
8.3 Design of Multi-modal Wearable System 187
8.3.1 Selection of Sensor 187
8.3.2 Selection of Participant Groups and Testing Environment 188
8.3.3 Data Collection Process 189
8.3.4 Machine Learning and Multisensory Fusion 190
8.4 Results and Discussion 194
8.5 Summary 197
References 197
9 Adaptive Secure Multi-modal Telehealth Patient-Monitoring System 201
Muhammad Hanif , Ehsan Ullah Munir, Muhammad Maaz Rehan, Saima Gulzar
Ahmad, Tassawar Iqbal, Nasira Kirn, Kashif Ayyub, and Naeem Ramzan
9.1 Healthcare Systems 202
9.1.1 Traditional Healthcare Systems 203
9.1.2 Multi-modal Telehealth Systems 203
9.2 Security in Healthcare Systems 205
9.2.1 Prevailing Techniques for Secure Telehealth 205
9.2.2 Challenges in Ensuring Healthcare Security 208
9.2.3 Strategies to Enhance Healthcare Data Security 209
9.2.4 Key Security Features for Telehealth Systems 210
9.2.4.1 Encryption 210
9.2.4.2 Authentication 211
9.2.4.3 Access Control 211
9.2.4.4 Audit Trails 211
9.2.4.5 Data Integrity Checks 211
9.2.4.6 Secure Communication Protocols 211
9.2.4.7 Security Audits and Penetration Testing 211
9.2.4.8 Cyber Resilience 212
9.2.4.9 Zero-Trust-Based Micro-segmentation 212
9.3 Blockchain-Powered ZTS for Enhanced Security of Telehealth Systems 213
9.3.1 Zero-Trust Security 213
9.3.2 Blockchain 214
9.4 Cyber-resilient Telehealth-Enabled Patient Management System 217
9.4.1 Assessment and Planning 218
9.4.2 Infrastructure Setup 219
9.4.3 Security Controls Implementation 219
9.4.4 Training and Awareness 219
9.4.5 Advantages and Limitations 221
9.5 Summary 222
References 222
10 Advances in Multi-modal Remote Infant Monitoring Systems 227
Najia Saher, Omer Riaz, Muhammad Suleman, Dost Muhammad Khan, Nasira Kirn,
Sana Ullah Jan, Rizwan Shahid, Hassan Rabah, and Naeem Ramzan
10.1 Remote Patient Monitoring 228
10.2 Remote Infant Monitoring (RIM) System 229
10.2.1 Contactless Remote Patient Monitoring (RPM) Systems 230
10.2.2 Contact-Based Remote Patient Monitoring (RPM) Systems 230
10.3 Disease-Specific Remote Infant Monitoring Systems 232
10.3.1 Respiration and Apnea Monitoring System 232
10.3.1.1 Emerging Sensing Techniques for Respiratory Diseases 233
10.3.2 Heart and Blood-Related Diseases Monitoring Systems 238
10.3.2.1 Emerging Sensing Techniques for Heart and Blood Diseases 238
10.3.3 Infant Monitoring Systems for Various Diseases 241
10.4 Challenges in Remote Infant Monitoring Systems 241
10.5 Summary 245
References 246
11 Balancing Innovation with Ethics: Responsible Development of Multi-modal
Intelligent Tutoring Systems 253
Romina Soledad Albornoz-De Luise, Pablo Arnau-González, Ana
Serrano-Mamolar, Sergi Solera-Monforte, and Yuyan Wu
11.1 Intelligent Tutoring Systems and Ethical Considerations 253
11.2 The Promise and Perils of ITS 255
11.2.1 Advantages of ITS in Education 255
11.2.1.1 Personalized Learning 255
11.2.1.2 Increased Accessibility 256
11.2.1.3 Continuous Assessment and Feedback 256
11.2.2 Potential Risks and Challenges 256
11.2.2.1 Bias in Algorithms 257
11.2.2.2 Privacy Concerns 257
11.2.2.3 Socioeconomic Disparities in Access 257
11.2.2.4 Dependency on Technology 258
11.3 Ethical Frameworks for ITS 258
11.3.1 Utilitarian Perspective 258
11.3.2 Deontological Perspective 259
11.3.3 Virtue Ethics 260
11.4 Bias and Fairness in ITS 261
11.5 Privacy and Security Concerns 263
11.5.1 Self-Reported Sources 263
11.5.2 Captured Data Sources 264
11.6 Socioeconomic Disparities in Access 265
11.7 Dependency on Technology 267
11.8 Summary 268
References 269
12 Road Ahead for Multi-modal Intelligent Sensing in the Deep Learning Era
275
Ahmed Zoha, Naeem Ramzan, Muhammad Ali Jamshed, and Masood Ur Rehman
12.1 Future Challenges and Perspectives for Intelligent Multi-modal Sensing
276
12.1.1 Semantic Gaps and Cross-modality Representation 276
12.1.2 Concept Drift and Data Quality 278
12.1.3 Computational Demands and Model Scalability 279
12.1.4 Interpretability 280
12.1.5 Ethical Considerations 281
12.2 Summary 282
References 282
Index 285
Contents
About the Editors xv
List of Contributors xix
Preface xxiii
1 Advances in Multi-modal Intelligent Sensing 1
Masood Ur Rehman, Muhammad Ali Jamshed, and Tahera Kalsoom
1.1 Multi-modal Intelligent Sensing 1
1.2 Sensors for Multi-modal Intelligent Sensing 3
1.2.1 Sensor Types 3
1.2.2 Integration of Multiple Sensor Types for Enhanced Sensing
Capabilities 5
1.2.2.1 Advantages of Multiple Sensor Integration 5
1.2.2.2 Key Considerations for Multiple Sensor Integration 6
1.2.2.3 Concurrent Data Acquisition Methods 9
1.2.2.4 Data Analysis Tools for Multi-modal Sensing 11
1.2.2.5 Considerations for Data Fusion and Synchronization 13
1.3 Applications of Multi-modal Intelligent Sensing 14
1.3.1 Healthcare and Medical Monitoring 14
1.3.2 Automotive and Transportation Systems 15
1.3.3 Environmental Monitoring and Conservation 16
1.3.4 Smart Cities and Infrastructure Management 17
1.3.5 Industrial Automation 18
1.4 Challenges and Opportunities in Multi-modal Sensing 18
1.4.1 Data Security and Privacy 19
1.4.2 Interoperability and Standardization 19
1.4.3 Energy Efficiency and Power Management 20
1.4.4 Coverage 21
1.4.5 Summary 21
References 22
2 Antennas for Wireless Sensors 29
Abdul Jabbar, Muhammad Ali Jamshed, and Masood Ur Rehman
2.1 Wireless Sensors: Definition and Architecture 29
2.1.1 Wireless Sensor Node Architecture 30
2.1.2 Operating Systems 32
2.1.3 Classification of Wireless Sensors 32
2.2 Multi-modal Wireless Sensing 34
2.3 Antennas: The Sensory Gateway for Wireless Sensors 35
2.4 Fundamental Antenna Parameters 36
2.4.1 Bandwidth and Operating Frequency 36
2.4.2 Gain 37
2.4.3 Radiation Pattern 37
2.4.4 Polarization 38
2.5 Key Operating Frequency Bands for Sensing Antennas 39
2.6 Fabrication Methods for Sensing Antennas 40
2.6.1 Printed Circuit Board (PCB) Antennas 40
2.6.2 On-Chip and Integrated Antenna Fabrication 41
2.6.3 Stitching and Embroidery for Flexible Textile Antennas 41
2.7 Antenna Types for Wireless Sensing Networks 42
2.7.1 Flexible Antennas 43
2.7.2 Omnidirectional Antennas 45
2.7.3 Directional Antennas 46
2.8 Advantages of Electronic Beamsteering Antennas in Sensing Systems 46
2.9 Summary 49
References 49
3 Sensor Design for Multimodal Environmental Monitoring 55
Muhammad Ali Jamshed, Bushra Haq, Syed Ahmed Shah, Kamran Ali, Qammer H.
Abbasi, Mumraiz Khan Kasi, and Masood Ur Rehman
3.1 Environment and Forests 56
3.2 Methods to Combat Deforestation 56
3.2.1 Combating Deforestation Using Wireless Sensor Networks 57
3.2.2 Sensor Types for Combating Deforestation 58
3.3 Design of a WSN to Combat Deforestation 59
3.3.1 Stage 1: System Requirements 59
3.3.1.1 Key Performance Indicators 62
3.3.2 Stage 2: Architecture 63
3.3.3 Stage 3: System Implementation 65
3.3.3.1 Type of Sensors 65
3.3.3.2 Processing Boards 66
3.3.3.3 Communication Modules 67
3.3.3.4 Batteries 67
3.3.3.5 Energy Harvesting Circuit and Equipment 69
3.3.3.6 Weather Protection 70
3.3.3.7 Wireless Communication Link 71
3.3.3.8 Data Processing Algorithms 74
3.4 Summary 76
References 76
4 Wireless Sensors for Multi-modal Health Monitoring 81
Nadeem Ajum, Shagufta Iftikhar, Tahera Kalsoom, and Masood Ur Rehman
4.1 Wearable Sensors 82
4.1.1 Electrocardiography (ECG) Sensors 83
4.1.2 Electroencephalography (EEG) Sensors 83
4.1.3 Electrooculography (EOG) Sensors 84
4.1.4 Electrodermal Activity (EDA) Sensors 86
4.1.5 Respiratory (RESP) Sensors 86
4.1.6 Motion Sensors 86
4.1.7 Temperature (TEMP) Sensors 87
4.1.8 Pressure Sensors 87
4.1.9 Hydration Sensors 88
4.1.10 Lactate Sensors 88
4.1.11 Photoplethysmography (PPG) Sensors 89
4.1.12 Continuous Glucose Monitoring (CGM) Sensors 89
4.2 Flexible Sensors 89
4.3 Multi-modal Healthcare Sensing Devices 90
4.3.1 Wearable Sensing Devices for Healthcare 90
4.3.1.1 Wearable Devices in Detection 90
4.3.1.2 Wearable Devices in Monitoring 92
4.3.1.3 Wearable Devices in Rehabilitation 93
4.3.1.4 Wearable Devices in Personalized Medicine 94
4.3.1.5 Wearable Devices in Skin Patches 94
4.3.1.6 Wearable Devices for Body Fluid Monitoring 95
4.3.1.7 Wearable Devices in Monitoring Body Temperature 96
4.3.1.8 Wearable Devices as Contact Lens 96
4.3.1.9 Wearable Devices in Daily Use Objects 97
4.3.2 Implantable Sensing Devices for Healthcare 97
4.3.2.1 Implantable Cardioverter Defibrillators 98
4.3.2.2 Bioinks and 3D Print Implants 98
4.3.2.3 Deep Brain Stimulation 99
4.3.2.4 Biosensor Tattoos 99
4.4 AI Methods for Multi-modal Healthcare Systems 100
4.4.1 Supervised Learning 100
4.4.2 Unsupervised Learning 101
4.4.3 Semi-supervised Learning 101
4.4.4 Reinforcement Learning 102
4.5 Summary 102
References 103
5 Sensor Design for Industrial Automation 109
Abdul Jabbar, Tahera Kalsoom, and Masood Ur Rehman
5.1 Multimodal Sensing in Industrial Automation 109
5.1.1 IIoT and Multimodal Sensing 111
5.1.2 Advanced Robotics 113
5.1.3 Big Data Analytics 114
5.1.4 Cloud Computing 114
5.1.5 Artificial Intelligence 115
5.1.6 Augmented Reality 116
5.2 Sensors for Realizing Industrial Automation 116
5.2.1 RF Sensors 117
5.2.2 Vision Sensors 118
5.2.3 Localization and Tracking Sensors 119
5.2.4 Infrared Sensors 119
5.2.5 Proximity Sensors 119
5.2.6 IMU Sensors 120
5.2.7 Level Sensors 120
5.2.8 Temperature Sensors 120
5.2.9 Pressure Sensors 121
5.3 Design Considerations for Effective Multimodal Industrial Automation
121
5.3.1 Design of AI-Assisted Multimodal Sensing 122
5.3.2 Design of Radar Sensing Networks 123
5.3.2.1 Transmitter and Receiver Antennas 123
5.3.2.2 Data Collection and Interface 123
5.3.2.3 Signal Processing 124
5.3.2.4 Housing and Enclosure 124
5.4 Challenges and Opportunities of Multimodal Sensing in Industrial
Automation 124
5.5 Summary 126
References 126
6 Hybrid Neuromorphic-Federated Learning for Activity Recognition Using
Multi-modal Wearable Sensors 133
Ahsan Raza Khan, Habib Ullah Manzoor, Fahad Ayaz, Muhammad Ali Imran, and
Ahmed Zoha
6.1 Multi-modal Human Activity Recognition 134
6.2 Machine Learning Methods in Multi-modal Human Activity Recognition 137
6.2.1 Centralized Learning-based HAR Systems 137
6.2.2 Federated Learning-based HAR Systems 138
6.3 System Model 139
6.3.1 Federated Learning Framework 140
6.3.2 Spiking Neural Network 141
6.3.3 Proposed S-LSTM Model 144
6.4 Simulation Setup 146
6.4.1 Dataset Description 146
6.4.1.1 UCI Dataset 147
6.4.1.2 Real-World Dataset 148
6.4.2 Performance Metrics 149
6.5 Results and Discussion 150
6.5.1 UCI Results 151
6.5.2 Real-World Dataset Results 154
6.5.3 Energy Efficiency Comparison 157
6.5.4 Personalized Model Comparison 159
6.6 Summary 159
References 161
7 Multi-modal Beam Prediction for Enhanced Beam Management in Drone
Communication Networks 165
Iftikhar Ahmad, Ahsan Raza Khan, Rao Naveed Bin Rais, Muhammad Ali Imran,
Sajjad Hussain, and Ahmed Zoha
7.1 Drone Communication 166
7.2 Beam Management 167
7.3 System Model 168
7.3.1 Problem Formulation for Beam Prediction 170
7.3.2 Proposed Stacked Vision-Assisted Beam Prediction Model 170
7.4 Simulation and Analysis 171
7.4.1 Description of the Dataset 173
7.4.2 Configuration for Simulation 173
7.4.2.1 YOLO-v5 Training Process 174
7.4.3 Results and Analysis 175
7.5 Summary 178
References 178
8 Multi-modal-Sensing System for Detection and Tracking of Mind Wandering
181
Sara Khosravi, Haobo Li, Ahsan Raza Khan, Ahmed Zoha, and Rami Ghannam
8.1 Mind Wandering 182
8.2 Multi-modal Wearable Systems for Mind-Wandering Detection and
Monitoring 184
8.2.1 Wearable Eye Trackers for Gaze Measurements 185
8.2.2 Wearable GSR and PPG Sensors for Physiological Measurements 186
8.3 Design of Multi-modal Wearable System 187
8.3.1 Selection of Sensor 187
8.3.2 Selection of Participant Groups and Testing Environment 188
8.3.3 Data Collection Process 189
8.3.4 Machine Learning and Multisensory Fusion 190
8.4 Results and Discussion 194
8.5 Summary 197
References 197
9 Adaptive Secure Multi-modal Telehealth Patient-Monitoring System 201
Muhammad Hanif , Ehsan Ullah Munir, Muhammad Maaz Rehan, Saima Gulzar
Ahmad, Tassawar Iqbal, Nasira Kirn, Kashif Ayyub, and Naeem Ramzan
9.1 Healthcare Systems 202
9.1.1 Traditional Healthcare Systems 203
9.1.2 Multi-modal Telehealth Systems 203
9.2 Security in Healthcare Systems 205
9.2.1 Prevailing Techniques for Secure Telehealth 205
9.2.2 Challenges in Ensuring Healthcare Security 208
9.2.3 Strategies to Enhance Healthcare Data Security 209
9.2.4 Key Security Features for Telehealth Systems 210
9.2.4.1 Encryption 210
9.2.4.2 Authentication 211
9.2.4.3 Access Control 211
9.2.4.4 Audit Trails 211
9.2.4.5 Data Integrity Checks 211
9.2.4.6 Secure Communication Protocols 211
9.2.4.7 Security Audits and Penetration Testing 211
9.2.4.8 Cyber Resilience 212
9.2.4.9 Zero-Trust-Based Micro-segmentation 212
9.3 Blockchain-Powered ZTS for Enhanced Security of Telehealth Systems 213
9.3.1 Zero-Trust Security 213
9.3.2 Blockchain 214
9.4 Cyber-resilient Telehealth-Enabled Patient Management System 217
9.4.1 Assessment and Planning 218
9.4.2 Infrastructure Setup 219
9.4.3 Security Controls Implementation 219
9.4.4 Training and Awareness 219
9.4.5 Advantages and Limitations 221
9.5 Summary 222
References 222
10 Advances in Multi-modal Remote Infant Monitoring Systems 227
Najia Saher, Omer Riaz, Muhammad Suleman, Dost Muhammad Khan, Nasira Kirn,
Sana Ullah Jan, Rizwan Shahid, Hassan Rabah, and Naeem Ramzan
10.1 Remote Patient Monitoring 228
10.2 Remote Infant Monitoring (RIM) System 229
10.2.1 Contactless Remote Patient Monitoring (RPM) Systems 230
10.2.2 Contact-Based Remote Patient Monitoring (RPM) Systems 230
10.3 Disease-Specific Remote Infant Monitoring Systems 232
10.3.1 Respiration and Apnea Monitoring System 232
10.3.1.1 Emerging Sensing Techniques for Respiratory Diseases 233
10.3.2 Heart and Blood-Related Diseases Monitoring Systems 238
10.3.2.1 Emerging Sensing Techniques for Heart and Blood Diseases 238
10.3.3 Infant Monitoring Systems for Various Diseases 241
10.4 Challenges in Remote Infant Monitoring Systems 241
10.5 Summary 245
References 246
11 Balancing Innovation with Ethics: Responsible Development of Multi-modal
Intelligent Tutoring Systems 253
Romina Soledad Albornoz-De Luise, Pablo Arnau-González, Ana
Serrano-Mamolar, Sergi Solera-Monforte, and Yuyan Wu
11.1 Intelligent Tutoring Systems and Ethical Considerations 253
11.2 The Promise and Perils of ITS 255
11.2.1 Advantages of ITS in Education 255
11.2.1.1 Personalized Learning 255
11.2.1.2 Increased Accessibility 256
11.2.1.3 Continuous Assessment and Feedback 256
11.2.2 Potential Risks and Challenges 256
11.2.2.1 Bias in Algorithms 257
11.2.2.2 Privacy Concerns 257
11.2.2.3 Socioeconomic Disparities in Access 257
11.2.2.4 Dependency on Technology 258
11.3 Ethical Frameworks for ITS 258
11.3.1 Utilitarian Perspective 258
11.3.2 Deontological Perspective 259
11.3.3 Virtue Ethics 260
11.4 Bias and Fairness in ITS 261
11.5 Privacy and Security Concerns 263
11.5.1 Self-Reported Sources 263
11.5.2 Captured Data Sources 264
11.6 Socioeconomic Disparities in Access 265
11.7 Dependency on Technology 267
11.8 Summary 268
References 269
12 Road Ahead for Multi-modal Intelligent Sensing in the Deep Learning Era
275
Ahmed Zoha, Naeem Ramzan, Muhammad Ali Jamshed, and Masood Ur Rehman
12.1 Future Challenges and Perspectives for Intelligent Multi-modal Sensing
276
12.1.1 Semantic Gaps and Cross-modality Representation 276
12.1.2 Concept Drift and Data Quality 278
12.1.3 Computational Demands and Model Scalability 279
12.1.4 Interpretability 280
12.1.5 Ethical Considerations 281
12.2 Summary 282
References 282
Index 285
About the Editors xv
List of Contributors xix
Preface xxiii
1 Advances in Multi-modal Intelligent Sensing 1
Masood Ur Rehman, Muhammad Ali Jamshed, and Tahera Kalsoom
1.1 Multi-modal Intelligent Sensing 1
1.2 Sensors for Multi-modal Intelligent Sensing 3
1.2.1 Sensor Types 3
1.2.2 Integration of Multiple Sensor Types for Enhanced Sensing
Capabilities 5
1.2.2.1 Advantages of Multiple Sensor Integration 5
1.2.2.2 Key Considerations for Multiple Sensor Integration 6
1.2.2.3 Concurrent Data Acquisition Methods 9
1.2.2.4 Data Analysis Tools for Multi-modal Sensing 11
1.2.2.5 Considerations for Data Fusion and Synchronization 13
1.3 Applications of Multi-modal Intelligent Sensing 14
1.3.1 Healthcare and Medical Monitoring 14
1.3.2 Automotive and Transportation Systems 15
1.3.3 Environmental Monitoring and Conservation 16
1.3.4 Smart Cities and Infrastructure Management 17
1.3.5 Industrial Automation 18
1.4 Challenges and Opportunities in Multi-modal Sensing 18
1.4.1 Data Security and Privacy 19
1.4.2 Interoperability and Standardization 19
1.4.3 Energy Efficiency and Power Management 20
1.4.4 Coverage 21
1.4.5 Summary 21
References 22
2 Antennas for Wireless Sensors 29
Abdul Jabbar, Muhammad Ali Jamshed, and Masood Ur Rehman
2.1 Wireless Sensors: Definition and Architecture 29
2.1.1 Wireless Sensor Node Architecture 30
2.1.2 Operating Systems 32
2.1.3 Classification of Wireless Sensors 32
2.2 Multi-modal Wireless Sensing 34
2.3 Antennas: The Sensory Gateway for Wireless Sensors 35
2.4 Fundamental Antenna Parameters 36
2.4.1 Bandwidth and Operating Frequency 36
2.4.2 Gain 37
2.4.3 Radiation Pattern 37
2.4.4 Polarization 38
2.5 Key Operating Frequency Bands for Sensing Antennas 39
2.6 Fabrication Methods for Sensing Antennas 40
2.6.1 Printed Circuit Board (PCB) Antennas 40
2.6.2 On-Chip and Integrated Antenna Fabrication 41
2.6.3 Stitching and Embroidery for Flexible Textile Antennas 41
2.7 Antenna Types for Wireless Sensing Networks 42
2.7.1 Flexible Antennas 43
2.7.2 Omnidirectional Antennas 45
2.7.3 Directional Antennas 46
2.8 Advantages of Electronic Beamsteering Antennas in Sensing Systems 46
2.9 Summary 49
References 49
3 Sensor Design for Multimodal Environmental Monitoring 55
Muhammad Ali Jamshed, Bushra Haq, Syed Ahmed Shah, Kamran Ali, Qammer H.
Abbasi, Mumraiz Khan Kasi, and Masood Ur Rehman
3.1 Environment and Forests 56
3.2 Methods to Combat Deforestation 56
3.2.1 Combating Deforestation Using Wireless Sensor Networks 57
3.2.2 Sensor Types for Combating Deforestation 58
3.3 Design of a WSN to Combat Deforestation 59
3.3.1 Stage 1: System Requirements 59
3.3.1.1 Key Performance Indicators 62
3.3.2 Stage 2: Architecture 63
3.3.3 Stage 3: System Implementation 65
3.3.3.1 Type of Sensors 65
3.3.3.2 Processing Boards 66
3.3.3.3 Communication Modules 67
3.3.3.4 Batteries 67
3.3.3.5 Energy Harvesting Circuit and Equipment 69
3.3.3.6 Weather Protection 70
3.3.3.7 Wireless Communication Link 71
3.3.3.8 Data Processing Algorithms 74
3.4 Summary 76
References 76
4 Wireless Sensors for Multi-modal Health Monitoring 81
Nadeem Ajum, Shagufta Iftikhar, Tahera Kalsoom, and Masood Ur Rehman
4.1 Wearable Sensors 82
4.1.1 Electrocardiography (ECG) Sensors 83
4.1.2 Electroencephalography (EEG) Sensors 83
4.1.3 Electrooculography (EOG) Sensors 84
4.1.4 Electrodermal Activity (EDA) Sensors 86
4.1.5 Respiratory (RESP) Sensors 86
4.1.6 Motion Sensors 86
4.1.7 Temperature (TEMP) Sensors 87
4.1.8 Pressure Sensors 87
4.1.9 Hydration Sensors 88
4.1.10 Lactate Sensors 88
4.1.11 Photoplethysmography (PPG) Sensors 89
4.1.12 Continuous Glucose Monitoring (CGM) Sensors 89
4.2 Flexible Sensors 89
4.3 Multi-modal Healthcare Sensing Devices 90
4.3.1 Wearable Sensing Devices for Healthcare 90
4.3.1.1 Wearable Devices in Detection 90
4.3.1.2 Wearable Devices in Monitoring 92
4.3.1.3 Wearable Devices in Rehabilitation 93
4.3.1.4 Wearable Devices in Personalized Medicine 94
4.3.1.5 Wearable Devices in Skin Patches 94
4.3.1.6 Wearable Devices for Body Fluid Monitoring 95
4.3.1.7 Wearable Devices in Monitoring Body Temperature 96
4.3.1.8 Wearable Devices as Contact Lens 96
4.3.1.9 Wearable Devices in Daily Use Objects 97
4.3.2 Implantable Sensing Devices for Healthcare 97
4.3.2.1 Implantable Cardioverter Defibrillators 98
4.3.2.2 Bioinks and 3D Print Implants 98
4.3.2.3 Deep Brain Stimulation 99
4.3.2.4 Biosensor Tattoos 99
4.4 AI Methods for Multi-modal Healthcare Systems 100
4.4.1 Supervised Learning 100
4.4.2 Unsupervised Learning 101
4.4.3 Semi-supervised Learning 101
4.4.4 Reinforcement Learning 102
4.5 Summary 102
References 103
5 Sensor Design for Industrial Automation 109
Abdul Jabbar, Tahera Kalsoom, and Masood Ur Rehman
5.1 Multimodal Sensing in Industrial Automation 109
5.1.1 IIoT and Multimodal Sensing 111
5.1.2 Advanced Robotics 113
5.1.3 Big Data Analytics 114
5.1.4 Cloud Computing 114
5.1.5 Artificial Intelligence 115
5.1.6 Augmented Reality 116
5.2 Sensors for Realizing Industrial Automation 116
5.2.1 RF Sensors 117
5.2.2 Vision Sensors 118
5.2.3 Localization and Tracking Sensors 119
5.2.4 Infrared Sensors 119
5.2.5 Proximity Sensors 119
5.2.6 IMU Sensors 120
5.2.7 Level Sensors 120
5.2.8 Temperature Sensors 120
5.2.9 Pressure Sensors 121
5.3 Design Considerations for Effective Multimodal Industrial Automation
121
5.3.1 Design of AI-Assisted Multimodal Sensing 122
5.3.2 Design of Radar Sensing Networks 123
5.3.2.1 Transmitter and Receiver Antennas 123
5.3.2.2 Data Collection and Interface 123
5.3.2.3 Signal Processing 124
5.3.2.4 Housing and Enclosure 124
5.4 Challenges and Opportunities of Multimodal Sensing in Industrial
Automation 124
5.5 Summary 126
References 126
6 Hybrid Neuromorphic-Federated Learning for Activity Recognition Using
Multi-modal Wearable Sensors 133
Ahsan Raza Khan, Habib Ullah Manzoor, Fahad Ayaz, Muhammad Ali Imran, and
Ahmed Zoha
6.1 Multi-modal Human Activity Recognition 134
6.2 Machine Learning Methods in Multi-modal Human Activity Recognition 137
6.2.1 Centralized Learning-based HAR Systems 137
6.2.2 Federated Learning-based HAR Systems 138
6.3 System Model 139
6.3.1 Federated Learning Framework 140
6.3.2 Spiking Neural Network 141
6.3.3 Proposed S-LSTM Model 144
6.4 Simulation Setup 146
6.4.1 Dataset Description 146
6.4.1.1 UCI Dataset 147
6.4.1.2 Real-World Dataset 148
6.4.2 Performance Metrics 149
6.5 Results and Discussion 150
6.5.1 UCI Results 151
6.5.2 Real-World Dataset Results 154
6.5.3 Energy Efficiency Comparison 157
6.5.4 Personalized Model Comparison 159
6.6 Summary 159
References 161
7 Multi-modal Beam Prediction for Enhanced Beam Management in Drone
Communication Networks 165
Iftikhar Ahmad, Ahsan Raza Khan, Rao Naveed Bin Rais, Muhammad Ali Imran,
Sajjad Hussain, and Ahmed Zoha
7.1 Drone Communication 166
7.2 Beam Management 167
7.3 System Model 168
7.3.1 Problem Formulation for Beam Prediction 170
7.3.2 Proposed Stacked Vision-Assisted Beam Prediction Model 170
7.4 Simulation and Analysis 171
7.4.1 Description of the Dataset 173
7.4.2 Configuration for Simulation 173
7.4.2.1 YOLO-v5 Training Process 174
7.4.3 Results and Analysis 175
7.5 Summary 178
References 178
8 Multi-modal-Sensing System for Detection and Tracking of Mind Wandering
181
Sara Khosravi, Haobo Li, Ahsan Raza Khan, Ahmed Zoha, and Rami Ghannam
8.1 Mind Wandering 182
8.2 Multi-modal Wearable Systems for Mind-Wandering Detection and
Monitoring 184
8.2.1 Wearable Eye Trackers for Gaze Measurements 185
8.2.2 Wearable GSR and PPG Sensors for Physiological Measurements 186
8.3 Design of Multi-modal Wearable System 187
8.3.1 Selection of Sensor 187
8.3.2 Selection of Participant Groups and Testing Environment 188
8.3.3 Data Collection Process 189
8.3.4 Machine Learning and Multisensory Fusion 190
8.4 Results and Discussion 194
8.5 Summary 197
References 197
9 Adaptive Secure Multi-modal Telehealth Patient-Monitoring System 201
Muhammad Hanif , Ehsan Ullah Munir, Muhammad Maaz Rehan, Saima Gulzar
Ahmad, Tassawar Iqbal, Nasira Kirn, Kashif Ayyub, and Naeem Ramzan
9.1 Healthcare Systems 202
9.1.1 Traditional Healthcare Systems 203
9.1.2 Multi-modal Telehealth Systems 203
9.2 Security in Healthcare Systems 205
9.2.1 Prevailing Techniques for Secure Telehealth 205
9.2.2 Challenges in Ensuring Healthcare Security 208
9.2.3 Strategies to Enhance Healthcare Data Security 209
9.2.4 Key Security Features for Telehealth Systems 210
9.2.4.1 Encryption 210
9.2.4.2 Authentication 211
9.2.4.3 Access Control 211
9.2.4.4 Audit Trails 211
9.2.4.5 Data Integrity Checks 211
9.2.4.6 Secure Communication Protocols 211
9.2.4.7 Security Audits and Penetration Testing 211
9.2.4.8 Cyber Resilience 212
9.2.4.9 Zero-Trust-Based Micro-segmentation 212
9.3 Blockchain-Powered ZTS for Enhanced Security of Telehealth Systems 213
9.3.1 Zero-Trust Security 213
9.3.2 Blockchain 214
9.4 Cyber-resilient Telehealth-Enabled Patient Management System 217
9.4.1 Assessment and Planning 218
9.4.2 Infrastructure Setup 219
9.4.3 Security Controls Implementation 219
9.4.4 Training and Awareness 219
9.4.5 Advantages and Limitations 221
9.5 Summary 222
References 222
10 Advances in Multi-modal Remote Infant Monitoring Systems 227
Najia Saher, Omer Riaz, Muhammad Suleman, Dost Muhammad Khan, Nasira Kirn,
Sana Ullah Jan, Rizwan Shahid, Hassan Rabah, and Naeem Ramzan
10.1 Remote Patient Monitoring 228
10.2 Remote Infant Monitoring (RIM) System 229
10.2.1 Contactless Remote Patient Monitoring (RPM) Systems 230
10.2.2 Contact-Based Remote Patient Monitoring (RPM) Systems 230
10.3 Disease-Specific Remote Infant Monitoring Systems 232
10.3.1 Respiration and Apnea Monitoring System 232
10.3.1.1 Emerging Sensing Techniques for Respiratory Diseases 233
10.3.2 Heart and Blood-Related Diseases Monitoring Systems 238
10.3.2.1 Emerging Sensing Techniques for Heart and Blood Diseases 238
10.3.3 Infant Monitoring Systems for Various Diseases 241
10.4 Challenges in Remote Infant Monitoring Systems 241
10.5 Summary 245
References 246
11 Balancing Innovation with Ethics: Responsible Development of Multi-modal
Intelligent Tutoring Systems 253
Romina Soledad Albornoz-De Luise, Pablo Arnau-González, Ana
Serrano-Mamolar, Sergi Solera-Monforte, and Yuyan Wu
11.1 Intelligent Tutoring Systems and Ethical Considerations 253
11.2 The Promise and Perils of ITS 255
11.2.1 Advantages of ITS in Education 255
11.2.1.1 Personalized Learning 255
11.2.1.2 Increased Accessibility 256
11.2.1.3 Continuous Assessment and Feedback 256
11.2.2 Potential Risks and Challenges 256
11.2.2.1 Bias in Algorithms 257
11.2.2.2 Privacy Concerns 257
11.2.2.3 Socioeconomic Disparities in Access 257
11.2.2.4 Dependency on Technology 258
11.3 Ethical Frameworks for ITS 258
11.3.1 Utilitarian Perspective 258
11.3.2 Deontological Perspective 259
11.3.3 Virtue Ethics 260
11.4 Bias and Fairness in ITS 261
11.5 Privacy and Security Concerns 263
11.5.1 Self-Reported Sources 263
11.5.2 Captured Data Sources 264
11.6 Socioeconomic Disparities in Access 265
11.7 Dependency on Technology 267
11.8 Summary 268
References 269
12 Road Ahead for Multi-modal Intelligent Sensing in the Deep Learning Era
275
Ahmed Zoha, Naeem Ramzan, Muhammad Ali Jamshed, and Masood Ur Rehman
12.1 Future Challenges and Perspectives for Intelligent Multi-modal Sensing
276
12.1.1 Semantic Gaps and Cross-modality Representation 276
12.1.2 Concept Drift and Data Quality 278
12.1.3 Computational Demands and Model Scalability 279
12.1.4 Interpretability 280
12.1.5 Ethical Considerations 281
12.2 Summary 282
References 282
Index 285