Darius M. Dziuda
Multivariate Biomarker Discovery (eBook, ePUB)
Data Science Methods for Efficient Analysis of High-Dimensional Biomedical Data
59,95 €
59,95 €
inkl. MwSt.
Sofort per Download lieferbar
30 °P sammeln
59,95 €
Als Download kaufen
59,95 €
inkl. MwSt.
Sofort per Download lieferbar
30 °P sammeln
Jetzt verschenken
Alle Infos zum eBook verschenken
59,95 €
inkl. MwSt.
Sofort per Download lieferbar
Alle Infos zum eBook verschenken
30 °P sammeln
Darius M. Dziuda
Multivariate Biomarker Discovery (eBook, ePUB)
Data Science Methods for Efficient Analysis of High-Dimensional Biomedical Data
- Format: ePub
- Merkliste
- Auf die Merkliste
- Bewerten Bewerten
- Teilen
- Produkt teilen
- Produkterinnerung
- Produkterinnerung
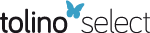
Bitte loggen Sie sich zunächst in Ihr Kundenkonto ein oder registrieren Sie sich bei
bücher.de, um das eBook-Abo tolino select nutzen zu können.
Hier können Sie sich einloggen
Hier können Sie sich einloggen
Sie sind bereits eingeloggt. Klicken Sie auf 2. tolino select Abo, um fortzufahren.
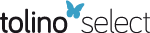
Bitte loggen Sie sich zunächst in Ihr Kundenkonto ein oder registrieren Sie sich bei bücher.de, um das eBook-Abo tolino select nutzen zu können.
- Geräte: eReader
- mit Kopierschutz
- eBook Hilfe
- Größe: 23.38MB
- FamilySharing(5)
Andere Kunden interessierten sich auch für
- Integrative Bioinformatics for Biomedical Big Data (eBook, ePUB)49,95 €
- Steve PresseData Modeling for the Sciences (eBook, ePUB)63,95 €
- Emanuel KulczyckiEvaluation Game (eBook, ePUB)31,95 €
- Integrative Bioinformatics for Biomedical Big Data (eBook, PDF)49,95 €
- Maria Dos Santos LonsdaleInformation Visualisation (eBook, ePUB)44,95 €
- Maria Dos Santos LonsdaleInformation Visualisation (eBook, ePUB)44,95 €
- Robert AndersenPresenting Statistical Results Effectively (eBook, ePUB)40,95 €
-
-
-
Produktdetails
- Verlag: Cambridge University Press
- Erscheinungstermin: 30. April 2024
- Englisch
- ISBN-13: 9781009007702
- Artikelnr.: 72466530
Dieser Download kann aus rechtlichen Gründen nur mit Rechnungsadresse in A, B, BG, CY, CZ, D, DK, EW, E, FIN, F, GR, HR, H, IRL, I, LT, L, LR, M, NL, PL, P, R, S, SLO, SK ausgeliefert werden.
- Herstellerkennzeichnung Die Herstellerinformationen sind derzeit nicht verfügbar.
Darius M. Dziuda, Ph.D., is Professor of Data Science and Bioinformatics at Central Connecticut State University (CCSU), with both academic and biotechnology industry experience. His research focuses on multivariate biomarker discovery for medical diagnosis, prognosis, and personalized medicine. Dr. Dziuda is also designing and teaching courses for two specializations of CCSU's graduate data science program: Bioinformatics and Advanced Data Science Methods.
Preface
Acknowledgments
Part I. Framework for Multivariate Biomarker Discovery: 1. Introduction
2. Multivariate analytics based on high-dimensional data: concepts and misconceptions
3. Predictive modeling for biomarker discovery
4. Evaluation of predictive models
5. Multivariate feature selection
Part II. Regression Methods for Estimation: 6. Basic regression methods
7. Regularized regression methods
8. Regression with random forests
9. Support vector regression
Part III. Classification Methods: 10. Classification with random forests
11. Classification with support vector machines
12. Discriminant analysis
13. Neural networks and deep learning
Part IV. Biomarker Discovery via Multistage Signal Enhancement and Identification of Essential Patterns: 14. Multistage signal enhancement
15. Essential patterns, essential variables, and interpretable biomarkers
Part V. Multivariate Biomarker Discovery Studies: 16. Biomarker discovery study 1: searching for essential gene expression patterns and multivariate biomarkers that are common for multiple types of cancer
17. Biomarker discovery study 2: multivariate biomarkers for liver cancer
References
Index.
Acknowledgments
Part I. Framework for Multivariate Biomarker Discovery: 1. Introduction
2. Multivariate analytics based on high-dimensional data: concepts and misconceptions
3. Predictive modeling for biomarker discovery
4. Evaluation of predictive models
5. Multivariate feature selection
Part II. Regression Methods for Estimation: 6. Basic regression methods
7. Regularized regression methods
8. Regression with random forests
9. Support vector regression
Part III. Classification Methods: 10. Classification with random forests
11. Classification with support vector machines
12. Discriminant analysis
13. Neural networks and deep learning
Part IV. Biomarker Discovery via Multistage Signal Enhancement and Identification of Essential Patterns: 14. Multistage signal enhancement
15. Essential patterns, essential variables, and interpretable biomarkers
Part V. Multivariate Biomarker Discovery Studies: 16. Biomarker discovery study 1: searching for essential gene expression patterns and multivariate biomarkers that are common for multiple types of cancer
17. Biomarker discovery study 2: multivariate biomarkers for liver cancer
References
Index.
Preface
Acknowledgments
Part I. Framework for Multivariate Biomarker Discovery: 1. Introduction
2. Multivariate analytics based on high-dimensional data: concepts and misconceptions
3. Predictive modeling for biomarker discovery
4. Evaluation of predictive models
5. Multivariate feature selection
Part II. Regression Methods for Estimation: 6. Basic regression methods
7. Regularized regression methods
8. Regression with random forests
9. Support vector regression
Part III. Classification Methods: 10. Classification with random forests
11. Classification with support vector machines
12. Discriminant analysis
13. Neural networks and deep learning
Part IV. Biomarker Discovery via Multistage Signal Enhancement and Identification of Essential Patterns: 14. Multistage signal enhancement
15. Essential patterns, essential variables, and interpretable biomarkers
Part V. Multivariate Biomarker Discovery Studies: 16. Biomarker discovery study 1: searching for essential gene expression patterns and multivariate biomarkers that are common for multiple types of cancer
17. Biomarker discovery study 2: multivariate biomarkers for liver cancer
References
Index.
Acknowledgments
Part I. Framework for Multivariate Biomarker Discovery: 1. Introduction
2. Multivariate analytics based on high-dimensional data: concepts and misconceptions
3. Predictive modeling for biomarker discovery
4. Evaluation of predictive models
5. Multivariate feature selection
Part II. Regression Methods for Estimation: 6. Basic regression methods
7. Regularized regression methods
8. Regression with random forests
9. Support vector regression
Part III. Classification Methods: 10. Classification with random forests
11. Classification with support vector machines
12. Discriminant analysis
13. Neural networks and deep learning
Part IV. Biomarker Discovery via Multistage Signal Enhancement and Identification of Essential Patterns: 14. Multistage signal enhancement
15. Essential patterns, essential variables, and interpretable biomarkers
Part V. Multivariate Biomarker Discovery Studies: 16. Biomarker discovery study 1: searching for essential gene expression patterns and multivariate biomarkers that are common for multiple types of cancer
17. Biomarker discovery study 2: multivariate biomarkers for liver cancer
References
Index.