New Frontiers in Mining Complex Patterns (eBook, PDF)
First International Workshop, NFMCP 2012, Held in Conjunction with ECML/PKDD 2012, Bristol, UK, September 24, 2012, Revised Selected Papers
Redaktion: Appice, Annalisa; Ras, Zbigniew; Masciari, Elio; Manco, Giuseppe; Loglisci, Corrado; Ceci, Michelangelo
Alle Infos zum eBook verschenken
New Frontiers in Mining Complex Patterns (eBook, PDF)
First International Workshop, NFMCP 2012, Held in Conjunction with ECML/PKDD 2012, Bristol, UK, September 24, 2012, Revised Selected Papers
Redaktion: Appice, Annalisa; Ras, Zbigniew; Masciari, Elio; Manco, Giuseppe; Loglisci, Corrado; Ceci, Michelangelo
- Format: PDF
- Merkliste
- Auf die Merkliste
- Bewerten Bewerten
- Teilen
- Produkt teilen
- Produkterinnerung
- Produkterinnerung
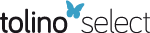
Hier können Sie sich einloggen
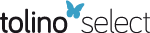
Bitte loggen Sie sich zunächst in Ihr Kundenkonto ein oder registrieren Sie sich bei bücher.de, um das eBook-Abo tolino select nutzen zu können.
This book constitutes the thoroughly refereed conference proceedings of the First International Workshop on New Frontiers in Mining Complex Patterns, NFMCP 2012, held in conjunction with ECML/PKDD 2012, in Bristol, UK, in September 2012. The 15 revised full papers were carefully reviewed and selected from numerous submissions. The papers are organized in topical sections on mining rich (relational) datasets, mining complex patterns from miscellaneous data, mining complex patterns from trajectory and sequence data, and mining complex patterns from graphs and networks.
- Geräte: PC
- ohne Kopierschutz
- eBook Hilfe
- Größe: 15.54MB
- New Frontiers in Mining Complex Patterns (eBook, PDF)38,95 €
- New Frontiers in Mining Complex Patterns (eBook, PDF)38,95 €
- New Frontiers in Mining Complex Patterns (eBook, PDF)40,95 €
- New Frontiers in Mining Complex Patterns (eBook, PDF)40,95 €
- New Frontiers in Mining Complex Patterns (eBook, PDF)40,95 €
- Mining Complex Data (eBook, PDF)40,95 €
- New Frontiers in Mining Complex Patterns (eBook, PDF)40,95 €
-
-
-
Dieser Download kann aus rechtlichen Gründen nur mit Rechnungsadresse in A, B, BG, CY, CZ, D, DK, EW, E, FIN, F, GR, HR, H, IRL, I, LT, L, LR, M, NL, PL, P, R, S, SLO, SK ausgeliefert werden.
- Produktdetails
- Verlag: Springer Berlin Heidelberg
- Seitenzahl: 231
- Erscheinungstermin: 25. März 2013
- Englisch
- ISBN-13: 9783642373824
- Artikelnr.: 44221866
- Verlag: Springer Berlin Heidelberg
- Seitenzahl: 231
- Erscheinungstermin: 25. März 2013
- Englisch
- ISBN-13: 9783642373824
- Artikelnr.: 44221866
- Herstellerkennzeichnung Die Herstellerinformationen sind derzeit nicht verfügbar.
Reducing Examples in Relational Learning with Bounded-Treewidth Hypotheses.- Mining Complex Event Patterns in Computer Networks.- Learning in the Presence of Large Fluctuations: A Study of Aggregation and Correlation.- Machine Learning as an Objective Approach to Understanding Music.- Pair-Based Object-Driven Action Rules.- Effectively Grouping Trajectory Streams.- Healthcare Trajectory Mining by Combining Multidimensional Component and Itemsets.- Graph-Based Approaches to Clustering Network-Constrained Trajectory Data.- Finding the Most Descriptive Substructures in Graphs with Discrete and Numeric Labels.- Learning in Probabilistic Graphs Exploiting Language-ConstrainedPatterns.- Improving Robustness and Flexibility of Concept Taxonomy Learning from Text.- Discovering Evolution Chains in Dynamic Networks.- Supporting Information Spread in a Social Internetworking Scenario.- Context-Aware Predictions on Business Processes: An Ensemble-Based Solution.
Reducing Examples in Relational Learning with Bounded-Treewidth Hypotheses.- Mining Complex Event Patterns in Computer Networks.- Learning in the Presence of Large Fluctuations: A Study of Aggregation and Correlation.- Machine Learning as an Objective Approach to Understanding Music.- Pair-Based Object-Driven Action Rules.- Effectively Grouping Trajectory Streams.- Healthcare Trajectory Mining by Combining Multidimensional Component and Itemsets.- Graph-Based Approaches to Clustering Network-Constrained Trajectory Data.- Finding the Most Descriptive Substructures in Graphs with Discrete and Numeric Labels.- Learning in Probabilistic Graphs Exploiting Language-ConstrainedPatterns.- Improving Robustness and Flexibility of Concept Taxonomy Learning from Text.- Discovering Evolution Chains in Dynamic Networks.- Supporting Information Spread in a Social Internetworking Scenario.- Context-Aware Predictions on Business Processes: An Ensemble-Based Solution.