126,99 €
126,99 €
inkl. MwSt.
Sofort per Download lieferbar
0 °P sammeln
126,99 €
Als Download kaufen
126,99 €
inkl. MwSt.
Sofort per Download lieferbar
0 °P sammeln
Jetzt verschenken
Alle Infos zum eBook verschenken
126,99 €
inkl. MwSt.
Sofort per Download lieferbar
Alle Infos zum eBook verschenken
0 °P sammeln
- Format: PDF
- Merkliste
- Auf die Merkliste
- Bewerten Bewerten
- Teilen
- Produkt teilen
- Produkterinnerung
- Produkterinnerung
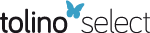
Bitte loggen Sie sich zunächst in Ihr Kundenkonto ein oder registrieren Sie sich bei
bücher.de, um das eBook-Abo tolino select nutzen zu können.
Hier können Sie sich einloggen
Hier können Sie sich einloggen
Sie sind bereits eingeloggt. Klicken Sie auf 2. tolino select Abo, um fortzufahren.
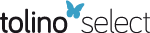
Bitte loggen Sie sich zunächst in Ihr Kundenkonto ein oder registrieren Sie sich bei bücher.de, um das eBook-Abo tolino select nutzen zu können.
Phase type distributions are widely applicable modeling and statistical tools for non-negative random quantities. They are built on Markov chains, which provide a simple, intuitive stochastic interpretation for their use. Phase Type Distribution starts from the Markov chain-based definition of phase type distributions and presents many interesting properties, which follow from the basic definition.
As a general family of non-negative distributions with nice analytical properties, phase type distributions can be used for approximating experimental distributions by fitting or by moments…mehr
- Geräte: PC
- mit Kopierschutz
- eBook Hilfe
- Größe: 9.54MB
Andere Kunden interessierten sich auch für
- Nanny FrömanPhase-Integral Method (eBook, PDF)40,95 €
- Michel FrémondPhase Change in Mechanics (eBook, PDF)40,95 €
- Thomas WickMultiphysics Phase-Field Fracture (eBook, PDF)91,95 €
- George IsacLeray-Schauder Type Alternatives, Complementarity Problems and Variational Inequalities (eBook, PDF)73,95 €
- Rosana Sueli Da Motta JafeliceBiological Models via Interval Type-2 Fuzzy Sets (eBook, PDF)48,95 €
- V. K. Saul'YevIntegration of Equations of Parabolic Type by the Method of Nets (eBook, PDF)40,95 €
- A. V. BitsadzeEquations of the Mixed Type (eBook, PDF)18,95 €
-
-
-
Phase type distributions are widely applicable modeling and statistical tools for non-negative random quantities. They are built on Markov chains, which provide a simple, intuitive stochastic interpretation for their use. Phase Type Distribution starts from the Markov chain-based definition of phase type distributions and presents many interesting properties, which follow from the basic definition.
As a general family of non-negative distributions with nice analytical properties, phase type distributions can be used for approximating experimental distributions by fitting or by moments matching; and, for discrete event simulation of real word systems with stochastic timing, such as production systems, service operations, communication networks, etc. This book summarizes the up-to-date fitting, matching and simulation methods, and presents the limits of flexibility of phase type distributions of a given order.
Additionally, this book lists numerical examples that support the intuitive understanding of the analytical descriptions and software tools that handle phase type distributions.
As a general family of non-negative distributions with nice analytical properties, phase type distributions can be used for approximating experimental distributions by fitting or by moments matching; and, for discrete event simulation of real word systems with stochastic timing, such as production systems, service operations, communication networks, etc. This book summarizes the up-to-date fitting, matching and simulation methods, and presents the limits of flexibility of phase type distributions of a given order.
Additionally, this book lists numerical examples that support the intuitive understanding of the analytical descriptions and software tools that handle phase type distributions.
Dieser Download kann aus rechtlichen Gründen nur mit Rechnungsadresse in D ausgeliefert werden.
Produktdetails
- Produktdetails
- Verlag: Wiley
- Seitenzahl: 280
- Erscheinungstermin: 18. Oktober 2024
- Englisch
- ISBN-13: 9781394329892
- Artikelnr.: 72249794
- Verlag: Wiley
- Seitenzahl: 280
- Erscheinungstermin: 18. Oktober 2024
- Englisch
- ISBN-13: 9781394329892
- Artikelnr.: 72249794
- Herstellerkennzeichnung Die Herstellerinformationen sind derzeit nicht verfügbar.
András Horváth is Associate Professor at the University of Turin, Italy. His research interests include analysis and optimization of stochastic models with applications in production systems, service operations, communication networks and systems biology.
Miklós Telek is a professor in the Department of Networked Systems and Services at Budapest University of Technology and Economics, Hungary, where he currently heads the Information Systems Research Group of the Hungarian Research Network. His research interests include stochastic performance modeling, analysis and optimization of computer and communication systems.
Miklós Telek is a professor in the Department of Networked Systems and Services at Budapest University of Technology and Economics, Hungary, where he currently heads the Information Systems Research Group of the Hungarian Research Network. His research interests include stochastic performance modeling, analysis and optimization of computer and communication systems.
Introduction xi
Chapter 1 Mathematical Background 1
1.1. Basic properties of random variables 1
1.2. Moments of random variables and related quantities 2
1.3. Laplace transformation 3
1.4. z transform 4
1.5. Matrix functions of quadratic matrices 5
1.6. Matrix inverse 5
1.7. Eigenvalues and the characteristic polynomial 5
1.8. Spectral decomposition 6
1.9. Ordinary differential equation of vector functions 10
1.10. Exponential distribution 11
1.11. Erlang distribution 12
1.12. Discrete time Markov chain 12
1.13. Continuous time Markov chain 14
1.14. Kronecker algebra 15
Chapter 2. Continuous Phase Type Distributions 19
2.1. Definition and basic properties 19
2.2 Stochastic meaning of (-A)-1 23
2.3. Rational Laplace transform 24
2.4. Decomposition of matrix exponential functions 26
2.5. Similarity transformation 30
2.5.1. Similarity transformation with identical sizes 30
2.5.2. Similarity transformation with different sizes 31
2.5.3. Full rank representation 32
2.6. Closure properties 32
2.7 Positive density on (0, 1) 34
2.8. Eigenvalue structure 35
2.9. Steepest increase property 39
Chapter 3. Discrete Phase Type Distributions 43
3.1. Definition and basic properties 43
3.2 Stochastic meaning of (I-B)-1 45
3.3. Rational z transform function 46
3.4. Decomposition of the matrix geometric function 46
3.5. Similarity transformations 48
3.6. Closure properties 48
3.7. Eigenvalue structure 49
Chapter 4. Matrix Exponential and Matrix Geometric Distributions 51
4.1. Matrix exponential distributions 51
4.1.1. Non-negative matrix exponential subclasses 53
4.2. Matrix geometric distributions 54
Chapter 5. Classes and Representations of Continuous Phase Type
Distributions 57
5.1. Number of parameters 57
5.2. Representations of continuous phase type distributions 58
5.2.1. Matrix representation 58
5.2.2. Markovian representation 58
5.2.3. Laplace representation 59
5.2.4. Moment representation 59
5.3. Transformations between continuous phase type representations 60
5.3.1. From matrix and Markovian representations to Laplace and moment
representations 60
5.3.2. From Laplace representation to moment representation 60
5.3.3. From moment representation to matrix representation 60
5.3.4. From Laplace representation to matrix representation 61
5.3.5. From matrix representation to Markovian representation 61
5.4. Properties of the matrix representation of PH(n) distributions 62
5.4.1. 2n-1 versus n2 + n-1 parameters 62
5.4.2. Different matrix representations 63
5.5. Subclasses of continuous phase type distributions 65
5.5.1. Subclasses with real eigenvalues 65
5.5.2. Subclasses with complex eigenvalues 73
5.6. Canonical Markovian representation 76
5.7. Analysis of a non-Markovian representation 77
5.7.1. Monocyclic representation 81
5.7.2. Transformation to a Markovian representation 82
5.8. Representation minimization 85
5.8.1. Numerical issues 86
5.9. Markovian representation minimization 86
Chapter 6. Moment Matching 89
6.1. Continuous phase type distributions with minimal squared coefficient
of variation 89
6.2. Moment bounds of continuous phase type distributions based on the
steepest increase property 96
6.3. Matrix exponential distributions with minimal squared coefficient of
variation 99
6.3.1. Matrix exponential distributions with complex eigenvalues 99
6.3.2. Matrix exponential distributions with real eigenvalues 104
6.4. Characteristics of moments of continuous phase type and matrix
exponential distributions 107
6.5. Matching 2n-1 moments with size n matrix representations 114
6.5.1. Padé approximation for continuous distributions 114
6.5.2. Padé approximation for discrete distributions 118
6.6. Matching any three moments with minimal acyclic phase type
distributions 120
6.6.1. Moment bounds 121
6.6.2. Explicit moment matching with minimal number of parameters 126
6.6.3. Proof of theorem 6.28 130
6.6.4. Proof of theorem 6.29 136
6.7. Matching any valid odd number of moments with generalized hyper-Erlang
distributions 137
6.7.1. Moment matching procedure for generalized hyper-Erlang distributions
137
6.7.2. Numerical examples 140
6.8. Matching probability density function at zero 145
6.8.1. Numerical examples 146
6.8.2. Moment matching using the behavior around zero 146
6.8.3. Improving matrix exponential approximation by matching the behavior
around zero 148
6.8.4. Improving matrix exponential approximation by approximating the
behavior around zero 150
6.9. Moment bounds of PH(2) distributions 151
6.10. Moment bounds of PH(3) distributions 155
6.10.1. The second and third normalized moments 156
6.10.2. The fourth and fifth normalized moments 157
Chapter 7. Distribution Fitting 165
7.1. Distance measures 166
7.2. Fitting based on maximum likelihood 168
7.2.1. The expectation maximization algorithm 169
7.2.2. Likelihood optimization for acyclic phase type distributions 176
7.3. Fitting based on families of polynomials 179
7.3.1. Bernstein polynomials and Bernstein expolynomials 179
7.3.2. Application of Bernstein expolynomials to distribution fitting 182
7.4. Fitting heavy-tailed distributions 185
7.4.1. Fitting heavy-tailed distributions with monotone decreasing density
function 186
7.4.2. Fitting heavy-tailed distributions with possibly non-monotone
density function 188
7.5. Continuous versus discrete phase type distributions in practical
fitting problems 190
7.5.1. Limit behavior as the scale factor tends to zero 191
7.5.2. The minimum squared coefficient of variation of scaled discrete
phase type distributions 192
7.5.3. The optimal scale factor in discrete phase type fitting 194
7.5.4. Approximating non-Markovian models 198
7.5.5. PH and scaled DPH approximation of continuous time models 203
Chapter 8. Simulation 205
8.1. Generating random samples based on the probabilistic interpretation
206
8.1.1. Sub-classes of continuous phase type distributions 206
8.1.2. The play algorithm 207
8.2. Representation transformation to improve the Play method 209
8.2.1. Formulating the optimization problem 210
8.2.2. The solution for acyclic phase type distributions 211
8.2.3. A heuristic representation optimization for general continuous phase
type distributions 215
8.3. An acceptance-rejection algorithm 216
8.3.1. Generating random variables from matrix exponential distributions
which have a Markovian generator 217
8.3.2. Generating matrix exponential distributed random variables using
feedback Erlang blocks 218
8.4. Overview of simulation methods 223
8.5. Numerical examples 224
8.5.1. Optimizing acyclic phase type representations 224
8.5.2. Optimizing general continuous phase type representations 225
Chapter 9. Continuous Phase Type Distribution Based Random Variables 229
9.1. Functions of continuous phase type distributions 229
9.1.1. Bijective functions of PH distributions 230
9.1.2. General functions of PH distributions 230
9.1.3. The considered transformation functions 231
9.2. Multivariate phase type distributions 235
9.2.1. Subset-based multivariate phase type distributions 235
9.2.2. Reward-based multivariate phase type distributions 236
9.2.3. Linear projection based multivariate multivariate phase type
distributions 238
Appendices 241
Appendix 1. Description of Related Software Tools 243
Appendix 2. Acronyms 245
References 247
Index 253
Chapter 1 Mathematical Background 1
1.1. Basic properties of random variables 1
1.2. Moments of random variables and related quantities 2
1.3. Laplace transformation 3
1.4. z transform 4
1.5. Matrix functions of quadratic matrices 5
1.6. Matrix inverse 5
1.7. Eigenvalues and the characteristic polynomial 5
1.8. Spectral decomposition 6
1.9. Ordinary differential equation of vector functions 10
1.10. Exponential distribution 11
1.11. Erlang distribution 12
1.12. Discrete time Markov chain 12
1.13. Continuous time Markov chain 14
1.14. Kronecker algebra 15
Chapter 2. Continuous Phase Type Distributions 19
2.1. Definition and basic properties 19
2.2 Stochastic meaning of (-A)-1 23
2.3. Rational Laplace transform 24
2.4. Decomposition of matrix exponential functions 26
2.5. Similarity transformation 30
2.5.1. Similarity transformation with identical sizes 30
2.5.2. Similarity transformation with different sizes 31
2.5.3. Full rank representation 32
2.6. Closure properties 32
2.7 Positive density on (0, 1) 34
2.8. Eigenvalue structure 35
2.9. Steepest increase property 39
Chapter 3. Discrete Phase Type Distributions 43
3.1. Definition and basic properties 43
3.2 Stochastic meaning of (I-B)-1 45
3.3. Rational z transform function 46
3.4. Decomposition of the matrix geometric function 46
3.5. Similarity transformations 48
3.6. Closure properties 48
3.7. Eigenvalue structure 49
Chapter 4. Matrix Exponential and Matrix Geometric Distributions 51
4.1. Matrix exponential distributions 51
4.1.1. Non-negative matrix exponential subclasses 53
4.2. Matrix geometric distributions 54
Chapter 5. Classes and Representations of Continuous Phase Type
Distributions 57
5.1. Number of parameters 57
5.2. Representations of continuous phase type distributions 58
5.2.1. Matrix representation 58
5.2.2. Markovian representation 58
5.2.3. Laplace representation 59
5.2.4. Moment representation 59
5.3. Transformations between continuous phase type representations 60
5.3.1. From matrix and Markovian representations to Laplace and moment
representations 60
5.3.2. From Laplace representation to moment representation 60
5.3.3. From moment representation to matrix representation 60
5.3.4. From Laplace representation to matrix representation 61
5.3.5. From matrix representation to Markovian representation 61
5.4. Properties of the matrix representation of PH(n) distributions 62
5.4.1. 2n-1 versus n2 + n-1 parameters 62
5.4.2. Different matrix representations 63
5.5. Subclasses of continuous phase type distributions 65
5.5.1. Subclasses with real eigenvalues 65
5.5.2. Subclasses with complex eigenvalues 73
5.6. Canonical Markovian representation 76
5.7. Analysis of a non-Markovian representation 77
5.7.1. Monocyclic representation 81
5.7.2. Transformation to a Markovian representation 82
5.8. Representation minimization 85
5.8.1. Numerical issues 86
5.9. Markovian representation minimization 86
Chapter 6. Moment Matching 89
6.1. Continuous phase type distributions with minimal squared coefficient
of variation 89
6.2. Moment bounds of continuous phase type distributions based on the
steepest increase property 96
6.3. Matrix exponential distributions with minimal squared coefficient of
variation 99
6.3.1. Matrix exponential distributions with complex eigenvalues 99
6.3.2. Matrix exponential distributions with real eigenvalues 104
6.4. Characteristics of moments of continuous phase type and matrix
exponential distributions 107
6.5. Matching 2n-1 moments with size n matrix representations 114
6.5.1. Padé approximation for continuous distributions 114
6.5.2. Padé approximation for discrete distributions 118
6.6. Matching any three moments with minimal acyclic phase type
distributions 120
6.6.1. Moment bounds 121
6.6.2. Explicit moment matching with minimal number of parameters 126
6.6.3. Proof of theorem 6.28 130
6.6.4. Proof of theorem 6.29 136
6.7. Matching any valid odd number of moments with generalized hyper-Erlang
distributions 137
6.7.1. Moment matching procedure for generalized hyper-Erlang distributions
137
6.7.2. Numerical examples 140
6.8. Matching probability density function at zero 145
6.8.1. Numerical examples 146
6.8.2. Moment matching using the behavior around zero 146
6.8.3. Improving matrix exponential approximation by matching the behavior
around zero 148
6.8.4. Improving matrix exponential approximation by approximating the
behavior around zero 150
6.9. Moment bounds of PH(2) distributions 151
6.10. Moment bounds of PH(3) distributions 155
6.10.1. The second and third normalized moments 156
6.10.2. The fourth and fifth normalized moments 157
Chapter 7. Distribution Fitting 165
7.1. Distance measures 166
7.2. Fitting based on maximum likelihood 168
7.2.1. The expectation maximization algorithm 169
7.2.2. Likelihood optimization for acyclic phase type distributions 176
7.3. Fitting based on families of polynomials 179
7.3.1. Bernstein polynomials and Bernstein expolynomials 179
7.3.2. Application of Bernstein expolynomials to distribution fitting 182
7.4. Fitting heavy-tailed distributions 185
7.4.1. Fitting heavy-tailed distributions with monotone decreasing density
function 186
7.4.2. Fitting heavy-tailed distributions with possibly non-monotone
density function 188
7.5. Continuous versus discrete phase type distributions in practical
fitting problems 190
7.5.1. Limit behavior as the scale factor tends to zero 191
7.5.2. The minimum squared coefficient of variation of scaled discrete
phase type distributions 192
7.5.3. The optimal scale factor in discrete phase type fitting 194
7.5.4. Approximating non-Markovian models 198
7.5.5. PH and scaled DPH approximation of continuous time models 203
Chapter 8. Simulation 205
8.1. Generating random samples based on the probabilistic interpretation
206
8.1.1. Sub-classes of continuous phase type distributions 206
8.1.2. The play algorithm 207
8.2. Representation transformation to improve the Play method 209
8.2.1. Formulating the optimization problem 210
8.2.2. The solution for acyclic phase type distributions 211
8.2.3. A heuristic representation optimization for general continuous phase
type distributions 215
8.3. An acceptance-rejection algorithm 216
8.3.1. Generating random variables from matrix exponential distributions
which have a Markovian generator 217
8.3.2. Generating matrix exponential distributed random variables using
feedback Erlang blocks 218
8.4. Overview of simulation methods 223
8.5. Numerical examples 224
8.5.1. Optimizing acyclic phase type representations 224
8.5.2. Optimizing general continuous phase type representations 225
Chapter 9. Continuous Phase Type Distribution Based Random Variables 229
9.1. Functions of continuous phase type distributions 229
9.1.1. Bijective functions of PH distributions 230
9.1.2. General functions of PH distributions 230
9.1.3. The considered transformation functions 231
9.2. Multivariate phase type distributions 235
9.2.1. Subset-based multivariate phase type distributions 235
9.2.2. Reward-based multivariate phase type distributions 236
9.2.3. Linear projection based multivariate multivariate phase type
distributions 238
Appendices 241
Appendix 1. Description of Related Software Tools 243
Appendix 2. Acronyms 245
References 247
Index 253
Introduction xi
Chapter 1 Mathematical Background 1
1.1. Basic properties of random variables 1
1.2. Moments of random variables and related quantities 2
1.3. Laplace transformation 3
1.4. z transform 4
1.5. Matrix functions of quadratic matrices 5
1.6. Matrix inverse 5
1.7. Eigenvalues and the characteristic polynomial 5
1.8. Spectral decomposition 6
1.9. Ordinary differential equation of vector functions 10
1.10. Exponential distribution 11
1.11. Erlang distribution 12
1.12. Discrete time Markov chain 12
1.13. Continuous time Markov chain 14
1.14. Kronecker algebra 15
Chapter 2. Continuous Phase Type Distributions 19
2.1. Definition and basic properties 19
2.2 Stochastic meaning of (-A)-1 23
2.3. Rational Laplace transform 24
2.4. Decomposition of matrix exponential functions 26
2.5. Similarity transformation 30
2.5.1. Similarity transformation with identical sizes 30
2.5.2. Similarity transformation with different sizes 31
2.5.3. Full rank representation 32
2.6. Closure properties 32
2.7 Positive density on (0, 1) 34
2.8. Eigenvalue structure 35
2.9. Steepest increase property 39
Chapter 3. Discrete Phase Type Distributions 43
3.1. Definition and basic properties 43
3.2 Stochastic meaning of (I-B)-1 45
3.3. Rational z transform function 46
3.4. Decomposition of the matrix geometric function 46
3.5. Similarity transformations 48
3.6. Closure properties 48
3.7. Eigenvalue structure 49
Chapter 4. Matrix Exponential and Matrix Geometric Distributions 51
4.1. Matrix exponential distributions 51
4.1.1. Non-negative matrix exponential subclasses 53
4.2. Matrix geometric distributions 54
Chapter 5. Classes and Representations of Continuous Phase Type
Distributions 57
5.1. Number of parameters 57
5.2. Representations of continuous phase type distributions 58
5.2.1. Matrix representation 58
5.2.2. Markovian representation 58
5.2.3. Laplace representation 59
5.2.4. Moment representation 59
5.3. Transformations between continuous phase type representations 60
5.3.1. From matrix and Markovian representations to Laplace and moment
representations 60
5.3.2. From Laplace representation to moment representation 60
5.3.3. From moment representation to matrix representation 60
5.3.4. From Laplace representation to matrix representation 61
5.3.5. From matrix representation to Markovian representation 61
5.4. Properties of the matrix representation of PH(n) distributions 62
5.4.1. 2n-1 versus n2 + n-1 parameters 62
5.4.2. Different matrix representations 63
5.5. Subclasses of continuous phase type distributions 65
5.5.1. Subclasses with real eigenvalues 65
5.5.2. Subclasses with complex eigenvalues 73
5.6. Canonical Markovian representation 76
5.7. Analysis of a non-Markovian representation 77
5.7.1. Monocyclic representation 81
5.7.2. Transformation to a Markovian representation 82
5.8. Representation minimization 85
5.8.1. Numerical issues 86
5.9. Markovian representation minimization 86
Chapter 6. Moment Matching 89
6.1. Continuous phase type distributions with minimal squared coefficient
of variation 89
6.2. Moment bounds of continuous phase type distributions based on the
steepest increase property 96
6.3. Matrix exponential distributions with minimal squared coefficient of
variation 99
6.3.1. Matrix exponential distributions with complex eigenvalues 99
6.3.2. Matrix exponential distributions with real eigenvalues 104
6.4. Characteristics of moments of continuous phase type and matrix
exponential distributions 107
6.5. Matching 2n-1 moments with size n matrix representations 114
6.5.1. Padé approximation for continuous distributions 114
6.5.2. Padé approximation for discrete distributions 118
6.6. Matching any three moments with minimal acyclic phase type
distributions 120
6.6.1. Moment bounds 121
6.6.2. Explicit moment matching with minimal number of parameters 126
6.6.3. Proof of theorem 6.28 130
6.6.4. Proof of theorem 6.29 136
6.7. Matching any valid odd number of moments with generalized hyper-Erlang
distributions 137
6.7.1. Moment matching procedure for generalized hyper-Erlang distributions
137
6.7.2. Numerical examples 140
6.8. Matching probability density function at zero 145
6.8.1. Numerical examples 146
6.8.2. Moment matching using the behavior around zero 146
6.8.3. Improving matrix exponential approximation by matching the behavior
around zero 148
6.8.4. Improving matrix exponential approximation by approximating the
behavior around zero 150
6.9. Moment bounds of PH(2) distributions 151
6.10. Moment bounds of PH(3) distributions 155
6.10.1. The second and third normalized moments 156
6.10.2. The fourth and fifth normalized moments 157
Chapter 7. Distribution Fitting 165
7.1. Distance measures 166
7.2. Fitting based on maximum likelihood 168
7.2.1. The expectation maximization algorithm 169
7.2.2. Likelihood optimization for acyclic phase type distributions 176
7.3. Fitting based on families of polynomials 179
7.3.1. Bernstein polynomials and Bernstein expolynomials 179
7.3.2. Application of Bernstein expolynomials to distribution fitting 182
7.4. Fitting heavy-tailed distributions 185
7.4.1. Fitting heavy-tailed distributions with monotone decreasing density
function 186
7.4.2. Fitting heavy-tailed distributions with possibly non-monotone
density function 188
7.5. Continuous versus discrete phase type distributions in practical
fitting problems 190
7.5.1. Limit behavior as the scale factor tends to zero 191
7.5.2. The minimum squared coefficient of variation of scaled discrete
phase type distributions 192
7.5.3. The optimal scale factor in discrete phase type fitting 194
7.5.4. Approximating non-Markovian models 198
7.5.5. PH and scaled DPH approximation of continuous time models 203
Chapter 8. Simulation 205
8.1. Generating random samples based on the probabilistic interpretation
206
8.1.1. Sub-classes of continuous phase type distributions 206
8.1.2. The play algorithm 207
8.2. Representation transformation to improve the Play method 209
8.2.1. Formulating the optimization problem 210
8.2.2. The solution for acyclic phase type distributions 211
8.2.3. A heuristic representation optimization for general continuous phase
type distributions 215
8.3. An acceptance-rejection algorithm 216
8.3.1. Generating random variables from matrix exponential distributions
which have a Markovian generator 217
8.3.2. Generating matrix exponential distributed random variables using
feedback Erlang blocks 218
8.4. Overview of simulation methods 223
8.5. Numerical examples 224
8.5.1. Optimizing acyclic phase type representations 224
8.5.2. Optimizing general continuous phase type representations 225
Chapter 9. Continuous Phase Type Distribution Based Random Variables 229
9.1. Functions of continuous phase type distributions 229
9.1.1. Bijective functions of PH distributions 230
9.1.2. General functions of PH distributions 230
9.1.3. The considered transformation functions 231
9.2. Multivariate phase type distributions 235
9.2.1. Subset-based multivariate phase type distributions 235
9.2.2. Reward-based multivariate phase type distributions 236
9.2.3. Linear projection based multivariate multivariate phase type
distributions 238
Appendices 241
Appendix 1. Description of Related Software Tools 243
Appendix 2. Acronyms 245
References 247
Index 253
Chapter 1 Mathematical Background 1
1.1. Basic properties of random variables 1
1.2. Moments of random variables and related quantities 2
1.3. Laplace transformation 3
1.4. z transform 4
1.5. Matrix functions of quadratic matrices 5
1.6. Matrix inverse 5
1.7. Eigenvalues and the characteristic polynomial 5
1.8. Spectral decomposition 6
1.9. Ordinary differential equation of vector functions 10
1.10. Exponential distribution 11
1.11. Erlang distribution 12
1.12. Discrete time Markov chain 12
1.13. Continuous time Markov chain 14
1.14. Kronecker algebra 15
Chapter 2. Continuous Phase Type Distributions 19
2.1. Definition and basic properties 19
2.2 Stochastic meaning of (-A)-1 23
2.3. Rational Laplace transform 24
2.4. Decomposition of matrix exponential functions 26
2.5. Similarity transformation 30
2.5.1. Similarity transformation with identical sizes 30
2.5.2. Similarity transformation with different sizes 31
2.5.3. Full rank representation 32
2.6. Closure properties 32
2.7 Positive density on (0, 1) 34
2.8. Eigenvalue structure 35
2.9. Steepest increase property 39
Chapter 3. Discrete Phase Type Distributions 43
3.1. Definition and basic properties 43
3.2 Stochastic meaning of (I-B)-1 45
3.3. Rational z transform function 46
3.4. Decomposition of the matrix geometric function 46
3.5. Similarity transformations 48
3.6. Closure properties 48
3.7. Eigenvalue structure 49
Chapter 4. Matrix Exponential and Matrix Geometric Distributions 51
4.1. Matrix exponential distributions 51
4.1.1. Non-negative matrix exponential subclasses 53
4.2. Matrix geometric distributions 54
Chapter 5. Classes and Representations of Continuous Phase Type
Distributions 57
5.1. Number of parameters 57
5.2. Representations of continuous phase type distributions 58
5.2.1. Matrix representation 58
5.2.2. Markovian representation 58
5.2.3. Laplace representation 59
5.2.4. Moment representation 59
5.3. Transformations between continuous phase type representations 60
5.3.1. From matrix and Markovian representations to Laplace and moment
representations 60
5.3.2. From Laplace representation to moment representation 60
5.3.3. From moment representation to matrix representation 60
5.3.4. From Laplace representation to matrix representation 61
5.3.5. From matrix representation to Markovian representation 61
5.4. Properties of the matrix representation of PH(n) distributions 62
5.4.1. 2n-1 versus n2 + n-1 parameters 62
5.4.2. Different matrix representations 63
5.5. Subclasses of continuous phase type distributions 65
5.5.1. Subclasses with real eigenvalues 65
5.5.2. Subclasses with complex eigenvalues 73
5.6. Canonical Markovian representation 76
5.7. Analysis of a non-Markovian representation 77
5.7.1. Monocyclic representation 81
5.7.2. Transformation to a Markovian representation 82
5.8. Representation minimization 85
5.8.1. Numerical issues 86
5.9. Markovian representation minimization 86
Chapter 6. Moment Matching 89
6.1. Continuous phase type distributions with minimal squared coefficient
of variation 89
6.2. Moment bounds of continuous phase type distributions based on the
steepest increase property 96
6.3. Matrix exponential distributions with minimal squared coefficient of
variation 99
6.3.1. Matrix exponential distributions with complex eigenvalues 99
6.3.2. Matrix exponential distributions with real eigenvalues 104
6.4. Characteristics of moments of continuous phase type and matrix
exponential distributions 107
6.5. Matching 2n-1 moments with size n matrix representations 114
6.5.1. Padé approximation for continuous distributions 114
6.5.2. Padé approximation for discrete distributions 118
6.6. Matching any three moments with minimal acyclic phase type
distributions 120
6.6.1. Moment bounds 121
6.6.2. Explicit moment matching with minimal number of parameters 126
6.6.3. Proof of theorem 6.28 130
6.6.4. Proof of theorem 6.29 136
6.7. Matching any valid odd number of moments with generalized hyper-Erlang
distributions 137
6.7.1. Moment matching procedure for generalized hyper-Erlang distributions
137
6.7.2. Numerical examples 140
6.8. Matching probability density function at zero 145
6.8.1. Numerical examples 146
6.8.2. Moment matching using the behavior around zero 146
6.8.3. Improving matrix exponential approximation by matching the behavior
around zero 148
6.8.4. Improving matrix exponential approximation by approximating the
behavior around zero 150
6.9. Moment bounds of PH(2) distributions 151
6.10. Moment bounds of PH(3) distributions 155
6.10.1. The second and third normalized moments 156
6.10.2. The fourth and fifth normalized moments 157
Chapter 7. Distribution Fitting 165
7.1. Distance measures 166
7.2. Fitting based on maximum likelihood 168
7.2.1. The expectation maximization algorithm 169
7.2.2. Likelihood optimization for acyclic phase type distributions 176
7.3. Fitting based on families of polynomials 179
7.3.1. Bernstein polynomials and Bernstein expolynomials 179
7.3.2. Application of Bernstein expolynomials to distribution fitting 182
7.4. Fitting heavy-tailed distributions 185
7.4.1. Fitting heavy-tailed distributions with monotone decreasing density
function 186
7.4.2. Fitting heavy-tailed distributions with possibly non-monotone
density function 188
7.5. Continuous versus discrete phase type distributions in practical
fitting problems 190
7.5.1. Limit behavior as the scale factor tends to zero 191
7.5.2. The minimum squared coefficient of variation of scaled discrete
phase type distributions 192
7.5.3. The optimal scale factor in discrete phase type fitting 194
7.5.4. Approximating non-Markovian models 198
7.5.5. PH and scaled DPH approximation of continuous time models 203
Chapter 8. Simulation 205
8.1. Generating random samples based on the probabilistic interpretation
206
8.1.1. Sub-classes of continuous phase type distributions 206
8.1.2. The play algorithm 207
8.2. Representation transformation to improve the Play method 209
8.2.1. Formulating the optimization problem 210
8.2.2. The solution for acyclic phase type distributions 211
8.2.3. A heuristic representation optimization for general continuous phase
type distributions 215
8.3. An acceptance-rejection algorithm 216
8.3.1. Generating random variables from matrix exponential distributions
which have a Markovian generator 217
8.3.2. Generating matrix exponential distributed random variables using
feedback Erlang blocks 218
8.4. Overview of simulation methods 223
8.5. Numerical examples 224
8.5.1. Optimizing acyclic phase type representations 224
8.5.2. Optimizing general continuous phase type representations 225
Chapter 9. Continuous Phase Type Distribution Based Random Variables 229
9.1. Functions of continuous phase type distributions 229
9.1.1. Bijective functions of PH distributions 230
9.1.2. General functions of PH distributions 230
9.1.3. The considered transformation functions 231
9.2. Multivariate phase type distributions 235
9.2.1. Subset-based multivariate phase type distributions 235
9.2.2. Reward-based multivariate phase type distributions 236
9.2.3. Linear projection based multivariate multivariate phase type
distributions 238
Appendices 241
Appendix 1. Description of Related Software Tools 243
Appendix 2. Acronyms 245
References 247
Index 253