19,95 €
19,95 €
inkl. MwSt.
Sofort per Download lieferbar
10 °P sammeln
19,95 €
Als Download kaufen
19,95 €
inkl. MwSt.
Sofort per Download lieferbar
10 °P sammeln
Jetzt verschenken
Alle Infos zum eBook verschenken
19,95 €
inkl. MwSt.
Sofort per Download lieferbar
Alle Infos zum eBook verschenken
10 °P sammeln
- Format: PDF
- Merkliste
- Auf die Merkliste
- Bewerten Bewerten
- Teilen
- Produkt teilen
- Produkterinnerung
- Produkterinnerung
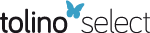
Bitte loggen Sie sich zunächst in Ihr Kundenkonto ein oder registrieren Sie sich bei
bücher.de, um das eBook-Abo tolino select nutzen zu können.
Hier können Sie sich einloggen
Hier können Sie sich einloggen
Sie sind bereits eingeloggt. Klicken Sie auf 2. tolino select Abo, um fortzufahren.
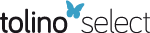
Bitte loggen Sie sich zunächst in Ihr Kundenkonto ein oder registrieren Sie sich bei bücher.de, um das eBook-Abo tolino select nutzen zu können.
Phishing Detection using Content Based Image Classification explores Phishing detection using computer vision through CNN, transfer learning and representation learning, utilizing ML & DL classifiers. This book is primarily aimed at researchers, professionals and students in field of Computer Vision and Cyber Security domain.
- Geräte: PC
- mit Kopierschutz
- eBook Hilfe
Andere Kunden interessierten sich auch für
- Shekhar KhandelwalPhishing Detection Using Content-Based Image Classification (eBook, ePUB)19,95 €
- Cyber Security Threats and Challenges Facing Human Life (eBook, PDF)46,95 €
- Cloud-based Intelligent Informative Engineering for Society 5.0 (eBook, PDF)46,95 €
- Multimedia Security Handbook (eBook, PDF)60,95 €
- Cyber Security Applications for Industry 4.0 (eBook, PDF)56,95 €
- Unleashing the Art of Digital Forensics (eBook, PDF)56,95 €
- Bishwajeet PandeyIntroduction to the Cyber Ranges (eBook, PDF)45,95 €
-
-
-
Phishing Detection using Content Based Image Classification explores Phishing detection using computer vision through CNN, transfer learning and representation learning, utilizing ML & DL classifiers. This book is primarily aimed at researchers, professionals and students in field of Computer Vision and Cyber Security domain.
Hinweis: Dieser Artikel kann nur an eine deutsche Lieferadresse ausgeliefert werden.
Dieser Download kann aus rechtlichen Gründen nur mit Rechnungsadresse in A, B, BG, CY, CZ, D, DK, EW, E, FIN, F, GR, HR, H, IRL, I, LT, L, LR, M, NL, PL, P, R, S, SLO, SK ausgeliefert werden.
Hinweis: Dieser Artikel kann nur an eine deutsche Lieferadresse ausgeliefert werden.
Produktdetails
- Produktdetails
- Verlag: Taylor & Francis eBooks
- Seitenzahl: 130
- Erscheinungstermin: 1. Juni 2022
- Englisch
- ISBN-13: 9781000597684
- Artikelnr.: 63907735
- Verlag: Taylor & Francis eBooks
- Seitenzahl: 130
- Erscheinungstermin: 1. Juni 2022
- Englisch
- ISBN-13: 9781000597684
- Artikelnr.: 63907735
- Herstellerkennzeichnung Die Herstellerinformationen sind derzeit nicht verfügbar.
Shekhar Khandelwal is a Data Scientist and works for Ernst & Young (EY) for Data & Analytics team. He has an extensive experience of around 15 years in the industry, and has worked across every sphere of Software Development Lifecycle. He has worked as a product developer, industry solutions developer, data engineer, data scientist and also as a Cloud developer. Previously, he worked for IBM Software labs where he also got a chance to work for industrial IoT based IBM cognitive product development and client deployment using various Watson tools and technologies. He is an industry leader solving challenging Computer Vision, NLP and Predictive Analytics based problems using Machine Learning and Deep Learning.
Dr. Rik Das is currently a Lead Software Engineer in Computer Vision Research at Siemens Advanta, India. Previously he was with Xavier Institute of Social Service, Ranchi, as an Assistant Professor for the Post Graduate Program in Information Technology. Dr.Das has over 17 years of experience in industrial and academic research. He was professionally associated with many leading universities and institutes in India, including Narsee Monjee Institute of Management Studies (NMIMS) (deemed-to-be-university), Globsyn Business School and Maulana Abul Kalam Azad University of Technology. Dr. Das has a Ph.D. (Tech.) in Information Technology from the University of Calcutta. He has also received his M.Tech. (Information Technology) from the University of Calcutta after his B.E. (Information Technology) from the University of Burdwan, West Bengal, India.
Dr. Rik Das is currently a Lead Software Engineer in Computer Vision Research at Siemens Advanta, India. Previously he was with Xavier Institute of Social Service, Ranchi, as an Assistant Professor for the Post Graduate Program in Information Technology. Dr.Das has over 17 years of experience in industrial and academic research. He was professionally associated with many leading universities and institutes in India, including Narsee Monjee Institute of Management Studies (NMIMS) (deemed-to-be-university), Globsyn Business School and Maulana Abul Kalam Azad University of Technology. Dr. Das has a Ph.D. (Tech.) in Information Technology from the University of Calcutta. He has also received his M.Tech. (Information Technology) from the University of Calcutta after his B.E. (Information Technology) from the University of Burdwan, West Bengal, India.
Chapter 1. Phishing and Cybersecurity. Basics of Phishing in Cybersecurity. Phishing Detection Techniques. List (whitelist/blacklist) based. Heuristics (pre-defined rules) based. Visual similarity based. Race between Phishers and Anti-Phishers. Chapter 2. Image Processing based Phishing Detection Techniques. Image processing based phishing detection techniques. Challenges in Phishing Detection using website images. Comparison of Techniques. Summary of Phishing detection using image processing techniques. Chapter 3. Implementing CNN for classifying phishing websites. Data Selection and Pre-Processing. Classification using CNN. CNN implementation. Performance metrics. Building a Convolutional Neural Network Model. Chapter 4. Transfer Learning Approach in Phishing Detection. Classification using Transfer Learning. Transfer Learning python implementation. Performance assessment of CNN models. Chapter 5. Feature Extraction and Representation Learning. Classification using Representation Learning. Data Preparation.. Feature Extraction using CNN off-the-shelf architectures. Handling class imbalance using SMOTE. SMOTE python implementation. Machine learning Classifier. Performance assessment of various experimentations. Chapter 6. Dimensionality Reduction Techniques. Basics of dimensionality reduction. PCA implementation using python. Performance assessment of various experimentations. Chapter 7. Feature Fusion Techniques. Basics of feature fusion technique. Different combinations of image representations. Different feature fusion approaches. Performance assessment of various experimentations. Chapter 8. Comparison of Phishing detection approaches. Classification Approaches. Evaluation of Classification Experiments. Comparison of the best performing model with the State-of-the-art. Chapter 9. Basics of Digital Image Processing. Basics of digital image processing. Basics of extracting features using OpenCV.
Chapter 1. Phishing and Cybersecurity. Basics of Phishing in Cybersecurity.
Phishing Detection Techniques. List (whitelist/blacklist) based. Heuristics
(pre-defined rules) based. Visual similarity based. Race between Phishers
and Anti-Phishers. Chapter 2. Image Processing based Phishing Detection
Techniques. Image processing based phishing detection techniques.
Challenges in Phishing Detection using website images. Comparison of
Techniques. Summary of Phishing detection using image processing
techniques. Chapter 3. Implementing CNN for classifying phishing websites.
Data Selection and Pre-Processing. Classification using CNN. CNN
implementation. Performance metrics. Building a Convolutional Neural
Network Model. Chapter 4. Transfer Learning Approach in Phishing Detection
. Classification using Transfer Learning. Transfer Learning python
implementation. Performance assessment of CNN models. Chapter 5. Feature
Extraction and Representation Learning. Classification using Representation
Learning. Data Preparation.. Feature Extraction using CNN off-the-shelf
architectures. Handling class imbalance using SMOTE. SMOTE python
implementation. Machine learning Classifier. Performance assessment of
various experimentations. Chapter 6. Dimensionality Reduction Techniques.
Basics of dimensionality reduction. PCA implementation using python.
Performance assessment of various experimentations. Chapter 7. Feature
Fusion Techniques. Basics of feature fusion technique. Different
combinations of image representations. Different feature fusion approaches.
Performance assessment of various experimentations. Chapter 8. Comparison
of Phishing detection approaches. Classification Approaches. Evaluation of
Classification Experiments. Comparison of the best performing model with
the State-of-the-art. Chapter 9. Basics of Digital Image Processing. Basics
of digital image processing. Basics of extracting features using OpenCV.
Phishing Detection Techniques. List (whitelist/blacklist) based. Heuristics
(pre-defined rules) based. Visual similarity based. Race between Phishers
and Anti-Phishers. Chapter 2. Image Processing based Phishing Detection
Techniques. Image processing based phishing detection techniques.
Challenges in Phishing Detection using website images. Comparison of
Techniques. Summary of Phishing detection using image processing
techniques. Chapter 3. Implementing CNN for classifying phishing websites.
Data Selection and Pre-Processing. Classification using CNN. CNN
implementation. Performance metrics. Building a Convolutional Neural
Network Model. Chapter 4. Transfer Learning Approach in Phishing Detection
. Classification using Transfer Learning. Transfer Learning python
implementation. Performance assessment of CNN models. Chapter 5. Feature
Extraction and Representation Learning. Classification using Representation
Learning. Data Preparation.. Feature Extraction using CNN off-the-shelf
architectures. Handling class imbalance using SMOTE. SMOTE python
implementation. Machine learning Classifier. Performance assessment of
various experimentations. Chapter 6. Dimensionality Reduction Techniques.
Basics of dimensionality reduction. PCA implementation using python.
Performance assessment of various experimentations. Chapter 7. Feature
Fusion Techniques. Basics of feature fusion technique. Different
combinations of image representations. Different feature fusion approaches.
Performance assessment of various experimentations. Chapter 8. Comparison
of Phishing detection approaches. Classification Approaches. Evaluation of
Classification Experiments. Comparison of the best performing model with
the State-of-the-art. Chapter 9. Basics of Digital Image Processing. Basics
of digital image processing. Basics of extracting features using OpenCV.
Chapter 1. Phishing and Cybersecurity. Basics of Phishing in Cybersecurity. Phishing Detection Techniques. List (whitelist/blacklist) based. Heuristics (pre-defined rules) based. Visual similarity based. Race between Phishers and Anti-Phishers. Chapter 2. Image Processing based Phishing Detection Techniques. Image processing based phishing detection techniques. Challenges in Phishing Detection using website images. Comparison of Techniques. Summary of Phishing detection using image processing techniques. Chapter 3. Implementing CNN for classifying phishing websites. Data Selection and Pre-Processing. Classification using CNN. CNN implementation. Performance metrics. Building a Convolutional Neural Network Model. Chapter 4. Transfer Learning Approach in Phishing Detection. Classification using Transfer Learning. Transfer Learning python implementation. Performance assessment of CNN models. Chapter 5. Feature Extraction and Representation Learning. Classification using Representation Learning. Data Preparation.. Feature Extraction using CNN off-the-shelf architectures. Handling class imbalance using SMOTE. SMOTE python implementation. Machine learning Classifier. Performance assessment of various experimentations. Chapter 6. Dimensionality Reduction Techniques. Basics of dimensionality reduction. PCA implementation using python. Performance assessment of various experimentations. Chapter 7. Feature Fusion Techniques. Basics of feature fusion technique. Different combinations of image representations. Different feature fusion approaches. Performance assessment of various experimentations. Chapter 8. Comparison of Phishing detection approaches. Classification Approaches. Evaluation of Classification Experiments. Comparison of the best performing model with the State-of-the-art. Chapter 9. Basics of Digital Image Processing. Basics of digital image processing. Basics of extracting features using OpenCV.
Chapter 1. Phishing and Cybersecurity. Basics of Phishing in Cybersecurity.
Phishing Detection Techniques. List (whitelist/blacklist) based. Heuristics
(pre-defined rules) based. Visual similarity based. Race between Phishers
and Anti-Phishers. Chapter 2. Image Processing based Phishing Detection
Techniques. Image processing based phishing detection techniques.
Challenges in Phishing Detection using website images. Comparison of
Techniques. Summary of Phishing detection using image processing
techniques. Chapter 3. Implementing CNN for classifying phishing websites.
Data Selection and Pre-Processing. Classification using CNN. CNN
implementation. Performance metrics. Building a Convolutional Neural
Network Model. Chapter 4. Transfer Learning Approach in Phishing Detection
. Classification using Transfer Learning. Transfer Learning python
implementation. Performance assessment of CNN models. Chapter 5. Feature
Extraction and Representation Learning. Classification using Representation
Learning. Data Preparation.. Feature Extraction using CNN off-the-shelf
architectures. Handling class imbalance using SMOTE. SMOTE python
implementation. Machine learning Classifier. Performance assessment of
various experimentations. Chapter 6. Dimensionality Reduction Techniques.
Basics of dimensionality reduction. PCA implementation using python.
Performance assessment of various experimentations. Chapter 7. Feature
Fusion Techniques. Basics of feature fusion technique. Different
combinations of image representations. Different feature fusion approaches.
Performance assessment of various experimentations. Chapter 8. Comparison
of Phishing detection approaches. Classification Approaches. Evaluation of
Classification Experiments. Comparison of the best performing model with
the State-of-the-art. Chapter 9. Basics of Digital Image Processing. Basics
of digital image processing. Basics of extracting features using OpenCV.
Phishing Detection Techniques. List (whitelist/blacklist) based. Heuristics
(pre-defined rules) based. Visual similarity based. Race between Phishers
and Anti-Phishers. Chapter 2. Image Processing based Phishing Detection
Techniques. Image processing based phishing detection techniques.
Challenges in Phishing Detection using website images. Comparison of
Techniques. Summary of Phishing detection using image processing
techniques. Chapter 3. Implementing CNN for classifying phishing websites.
Data Selection and Pre-Processing. Classification using CNN. CNN
implementation. Performance metrics. Building a Convolutional Neural
Network Model. Chapter 4. Transfer Learning Approach in Phishing Detection
. Classification using Transfer Learning. Transfer Learning python
implementation. Performance assessment of CNN models. Chapter 5. Feature
Extraction and Representation Learning. Classification using Representation
Learning. Data Preparation.. Feature Extraction using CNN off-the-shelf
architectures. Handling class imbalance using SMOTE. SMOTE python
implementation. Machine learning Classifier. Performance assessment of
various experimentations. Chapter 6. Dimensionality Reduction Techniques.
Basics of dimensionality reduction. PCA implementation using python.
Performance assessment of various experimentations. Chapter 7. Feature
Fusion Techniques. Basics of feature fusion technique. Different
combinations of image representations. Different feature fusion approaches.
Performance assessment of various experimentations. Chapter 8. Comparison
of Phishing detection approaches. Classification Approaches. Evaluation of
Classification Experiments. Comparison of the best performing model with
the State-of-the-art. Chapter 9. Basics of Digital Image Processing. Basics
of digital image processing. Basics of extracting features using OpenCV.