117,99 €
117,99 €
inkl. MwSt.
Sofort per Download lieferbar
0 °P sammeln
117,99 €
Als Download kaufen
117,99 €
inkl. MwSt.
Sofort per Download lieferbar
0 °P sammeln
Jetzt verschenken
Alle Infos zum eBook verschenken
117,99 €
inkl. MwSt.
Sofort per Download lieferbar
Alle Infos zum eBook verschenken
0 °P sammeln
- Format: PDF
- Merkliste
- Auf die Merkliste
- Bewerten Bewerten
- Teilen
- Produkt teilen
- Produkterinnerung
- Produkterinnerung
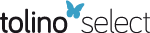
Bitte loggen Sie sich zunächst in Ihr Kundenkonto ein oder registrieren Sie sich bei
bücher.de, um das eBook-Abo tolino select nutzen zu können.
Hier können Sie sich einloggen
Hier können Sie sich einloggen
Sie sind bereits eingeloggt. Klicken Sie auf 2. tolino select Abo, um fortzufahren.
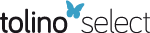
Bitte loggen Sie sich zunächst in Ihr Kundenkonto ein oder registrieren Sie sich bei bücher.de, um das eBook-Abo tolino select nutzen zu können.
* This book deals with the fundamentals of genetic algorithms and their applications in a variety of different areas of engineering and science * Most significant update to the second edition is the MATLAB codes that accompany the text * Provides a thorough discussion of hybrid genetic algorithms * Features more examples than first edition
- Geräte: PC
- mit Kopierschutz
- eBook Hilfe
- Größe: 9.42MB
Andere Kunden interessierten sich auch für
- Andrie De VriesR For Dummies (eBook, PDF)22,99 €
- Curtis PoeBeginning Perl (eBook, PDF)27,99 €
- Renaud MarletProgram Specialization (eBook, PDF)185,99 €
- Laura CassellPython Projects (eBook, PDF)30,99 €
- Richard BlumProfessional Assembly Language (eBook, PDF)35,99 €
- Dennis E. ShashaPuzzles for Programmers and Pros (eBook, PDF)17,99 €
- Pouria AmirianBeginning ArcGIS for Desktop Development using .NET (eBook, PDF)22,99 €
-
-
-
* This book deals with the fundamentals of genetic algorithms and their applications in a variety of different areas of engineering and science * Most significant update to the second edition is the MATLAB codes that accompany the text * Provides a thorough discussion of hybrid genetic algorithms * Features more examples than first edition
Dieser Download kann aus rechtlichen Gründen nur mit Rechnungsadresse in A, B, BG, CY, CZ, D, DK, EW, E, FIN, F, GR, HR, H, IRL, I, LT, L, LR, M, NL, PL, P, R, S, SLO, SK ausgeliefert werden.
Produktdetails
- Produktdetails
- Verlag: John Wiley & Sons
- Seitenzahl: 288
- Erscheinungstermin: 16. Juli 2004
- Englisch
- ISBN-13: 9780471671756
- Artikelnr.: 37302076
- Verlag: John Wiley & Sons
- Seitenzahl: 288
- Erscheinungstermin: 16. Juli 2004
- Englisch
- ISBN-13: 9780471671756
- Artikelnr.: 37302076
- Herstellerkennzeichnung Die Herstellerinformationen sind derzeit nicht verfügbar.
RANDY L. HAUPT, PhD, is Department Head and Senior Scientist at The Pennsylvania State University Applied Research Laboratory, State College, Pennsylvania. SUE ELLEN HAUPT, PhD, is a Senior Research Associate in the Computational Mechanics Division of The Pennsylvania State University Applied Research Laboratory, State College, Pennsylvania. Both Randy and Sue Ellen Haupt are renowned experts in the field of genetic algorithms in engineering and science applications.
Preface. Preface to First Edition. List of Symbols. 1. Introduction to Optimization. 1.1 Finding the Best Solution. 1.2 Minimum-Seeking Algorithms. 1.3 Natural Optimization Methods. 1.4 Biological Optimization: Natural Selection. 1.5 The Genetic Algorithm. 2. The Binary Genetic Algorithm. 2.1 Genetic Algorithms: Natural Selection on a Computer. 2.2 Components of a Binary Genetic Algorithm. 2.3 A Parting Look. 3. The Continuous Genetic Algorithm. 3.1 Components of a Continuous Genetic Algorithm. 3.2 A Parting Look. 4. Basic Applications. 4.1 "Mary Had a Little Lamb". 4.2 Algorithmic Creativity-Genetic Art. 4.3 Word Guess. 4.4 Locating an Emergency Response Unit. 4.5 Antenna Array Design. 4.6 The Evolution of Horses. 4.7 Summary. 5. An Added Level of Sophistication. 5.1 Handling Expensive Cost Functions. 5.2 Multiple Objective Optimization. 5.3 Hybrid GA. 5.4 Gray Codes. 5.5 Gene Size. 5.6 Convergence. 5.7 Alternative Crossovers for Binary GAs. 5.8 Population. 5.9 Mutation. 5.10 Permutation Problems. 5.11 Selling GA Parameters. 5.12 Continuous versus Binary GA. 5.13 Messy Genetic Algorithms. 5.14 Parallel Genetic Algorithms. 6. Advanced Applications. 6.1 Traveling Salespersons Problem. 6.2 Locating an Emergency Response Unit Revisited. 6.3 Decoding a Secret Message. 6.4 Robot Trajectory Planning. 6.5 Stealth Design. 6.6 Building Dynamical Inverse Models-The Linear Case. 6.7 Building Dynamical Inverse Models-The Nonlinear Case. 6.8 Combining GAs with Simulations-Air Pollution Receptor Modeling. 6.9 Combining Methods Neural Nets with GAs. 6.10 Solving High-Order Nonlinear Partial Differential Equations. 7. More Natural Optimization Algorithms. 7.1 Simulated Annealing. 7.2 Particle Swarm Optimization (PSO). 7.3 Ant Colony Optimization (ACO). 7.4 Genetic Programming (GP). 7.5 Cultural Algorithms. 7.6 Evolutionary Strategies. 7.7 The Future of Genetic Algorithms. Appendix I: Test Functions. Appendix II: MATLAB Code. Appendix III. High-Performance Fortran Code. Glossary. Index.
Preface. Preface to First Edition. List of Symbols. 1. Introduction to Optimization. 1.1 Finding the Best Solution. 1.2 Minimum-Seeking Algorithms. 1.3 Natural Optimization Methods. 1.4 Biological Optimization: Natural Selection. 1.5 The Genetic Algorithm. 2. The Binary Genetic Algorithm. 2.1 Genetic Algorithms: Natural Selection on a Computer. 2.2 Components of a Binary Genetic Algorithm. 2.3 A Parting Look. 3. The Continuous Genetic Algorithm. 3.1 Components of a Continuous Genetic Algorithm. 3.2 A Parting Look. 4. Basic Applications. 4.1 "Mary Had a Little Lamb". 4.2 Algorithmic Creativity-Genetic Art. 4.3 Word Guess. 4.4 Locating an Emergency Response Unit. 4.5 Antenna Array Design. 4.6 The Evolution of Horses. 4.7 Summary. 5. An Added Level of Sophistication. 5.1 Handling Expensive Cost Functions. 5.2 Multiple Objective Optimization. 5.3 Hybrid GA. 5.4 Gray Codes. 5.5 Gene Size. 5.6 Convergence. 5.7 Alternative Crossovers for Binary GAs. 5.8 Population. 5.9 Mutation. 5.10 Permutation Problems. 5.11 Selling GA Parameters. 5.12 Continuous versus Binary GA. 5.13 Messy Genetic Algorithms. 5.14 Parallel Genetic Algorithms. 6. Advanced Applications. 6.1 Traveling Salespersons Problem. 6.2 Locating an Emergency Response Unit Revisited. 6.3 Decoding a Secret Message. 6.4 Robot Trajectory Planning. 6.5 Stealth Design. 6.6 Building Dynamical Inverse Models-The Linear Case. 6.7 Building Dynamical Inverse Models-The Nonlinear Case. 6.8 Combining GAs with Simulations-Air Pollution Receptor Modeling. 6.9 Combining Methods Neural Nets with GAs. 6.10 Solving High-Order Nonlinear Partial Differential Equations. 7. More Natural Optimization Algorithms. 7.1 Simulated Annealing. 7.2 Particle Swarm Optimization (PSO). 7.3 Ant Colony Optimization (ACO). 7.4 Genetic Programming (GP). 7.5 Cultural Algorithms. 7.6 Evolutionary Strategies. 7.7 The Future of Genetic Algorithms. Appendix I: Test Functions. Appendix II: MATLAB Code. Appendix III. High-Performance Fortran Code. Glossary. Index.