Quantum Machine Learning (eBook, PDF)
A Modern Approach
Redaktion: Karthikeyan, S.; Poongodi, T.; Sumathi, D.; Akila, M.
52,95 €
52,95 €
inkl. MwSt.
Sofort per Download lieferbar
26 °P sammeln
52,95 €
Als Download kaufen
52,95 €
inkl. MwSt.
Sofort per Download lieferbar
26 °P sammeln
Jetzt verschenken
Alle Infos zum eBook verschenken
52,95 €
inkl. MwSt.
Sofort per Download lieferbar
Alle Infos zum eBook verschenken
26 °P sammeln
Quantum Machine Learning (eBook, PDF)
A Modern Approach
Redaktion: Karthikeyan, S.; Poongodi, T.; Sumathi, D.; Akila, M.
- Format: PDF
- Merkliste
- Auf die Merkliste
- Bewerten Bewerten
- Teilen
- Produkt teilen
- Produkterinnerung
- Produkterinnerung
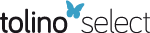
Bitte loggen Sie sich zunächst in Ihr Kundenkonto ein oder registrieren Sie sich bei
bücher.de, um das eBook-Abo tolino select nutzen zu können.
Hier können Sie sich einloggen
Hier können Sie sich einloggen
Sie sind bereits eingeloggt. Klicken Sie auf 2. tolino select Abo, um fortzufahren.
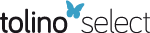
Bitte loggen Sie sich zunächst in Ihr Kundenkonto ein oder registrieren Sie sich bei bücher.de, um das eBook-Abo tolino select nutzen zu können.
This text presents the research into and application of machine learning in quantum computation, known as quantum machine learning (QML). It presents a comparison of quantum machine learning, classical machine learning, and traditional programming.
- Geräte: PC
- mit Kopierschutz
- eBook Hilfe
- Größe: 13.57MB
Andere Kunden interessierten sich auch für
- Quantum Machine Learning (eBook, ePUB)52,95 €
- Sarika JainUnderstanding Semantics-Based Decision Support (eBook, PDF)47,95 €
- Pijush DuttaArtificial Intelligence for Cognitive Modeling (eBook, PDF)52,95 €
- M. S. RamkarthikAn Object-Oriented Python Cookbook in Quantum Information Theory and Quantum Computing (eBook, PDF)48,95 €
- Srinidhi HiriyannaiahCloud-based Multi-Modal Information Analytics (eBook, PDF)52,95 €
- Alexei PetrenkoApplied Quantum Cryptanalysis (eBook, PDF)102,95 €
- Innovation in the University 4.0 System based on Smart Technologies (eBook, PDF)52,95 €
-
-
-
This text presents the research into and application of machine learning in quantum computation, known as quantum machine learning (QML). It presents a comparison of quantum machine learning, classical machine learning, and traditional programming.
Dieser Download kann aus rechtlichen Gründen nur mit Rechnungsadresse in A, B, BG, CY, CZ, D, DK, EW, E, FIN, F, GR, HR, H, IRL, I, LT, L, LR, M, NL, PL, P, R, S, SLO, SK ausgeliefert werden.
Produktdetails
- Produktdetails
- Verlag: Taylor & Francis
- Seitenzahl: 300
- Erscheinungstermin: 28. Oktober 2024
- Englisch
- ISBN-13: 9781040116104
- Artikelnr.: 72302581
- Verlag: Taylor & Francis
- Seitenzahl: 300
- Erscheinungstermin: 28. Oktober 2024
- Englisch
- ISBN-13: 9781040116104
- Artikelnr.: 72302581
- Herstellerkennzeichnung Die Herstellerinformationen sind derzeit nicht verfügbar.
Dr. Karthikeyan Saminathan is currently working as an Associate Professor(Research) in the Department of Cyber Security at Sri Venkateshwara College of Engineering, Bengaluru, Karnataka, India and He is associated with Bluechip CyberTech Services as a Asst. Vice President which is the wing of Bluechip Services International Pvt Ltd, Bangalore. he is Founder CEO for AI Quantalytics Startup which primarily focuses on IT consulting and product development to AI-driven solutions and cloud innovations. His Educational Background includes, he received his B.E-CSE degree from Anna University, Chennai in 2010 and his M.E-Software Engineering from Anna University, Chennai in 2012. He received his PhD in Cloud and Bigdata Analytics from VIT University, AP. His research interests include artificial intelligence and machine learning, high-performance computing, cloud and big data analytics, and data sciences. He has published more than 60+ papers in reputed journals, seven book chapters, and more than 12 patents. He has Delivered 100+ technical talks to various Academic Institutions and Industries. Also he holds the prestigious role of NASSCOM Prime brand Ambassador. He is a life member of international professional bodies such as ISTE, IAENG, ISRD, and IFERP and he is also a senior member of IEEE. Dr. M. Akila is a CEO of Metasage Alliance Consulting Expert Pvt Limited, Coimbatore, India. She is also an insatiable appetite for continuous learning and teaching, a philanthropic leader, a diligent researcher, and an experienced and insightful academician. With 27 years of experience, her mission is to enhance standards of education by providing an excellent, ingenious learning environment that is rational with the core values of Academic Institutions. Dr. Akila's main research interest is machine learning with applications to computer vision and data science, but she is also interested in the efficient implementation of optimization algorithms in engineering problems. An inspiring, motivating, and committed CSE professor, she is actively engaged with professional organizations such as IET and IEEE and is an additional secretary in the Institution of Green Engineers. She has published three patents and delivered 32 invited lectures at various institutes. Dr. Akila received the Kalam 2020 award for service to Green Technology, 2018 and has been awarded the Cambridge International Certificate for Teachers and Trainers and a Certificate of Achievement from IGEN. She is, in addition, a renowned reviewer in neuro-computing, IET biometrics, IET image processing, and IET electronics letters. Dr. D. Sumathi is currently serving as a Professor Grade 1-SCOPE at VIT-AP University, Andhra Pradesh. She earned her B.E in Computer Science and Engineering from Bharathiar University in 1994 and her M.E in Computer Science and Engineering from Sathyabama University in 2006, Chennai. She completed her doctoral degree at Anna University, Chennai. With a total of 23 years of experience, including 6 years in the industry and 17 years in the teaching field, she holds the additional responsibility of serving as an Assistant Director of the Software Development Cell, which automates various campus upkeep functionalities. Her research interests encompass Cloud Computing, Network Security, Data Mining, Natural Language Processing, Machine Learning, Deep Learning, and Theoretical Foundations of Computer Science. She has published numerous papers in reputed international journals and conferences. Furthermore, she has organized several international conferences, acting as a Technical Chair and tutorial presenter. Dr. D. Sumathi is a life member of ISTE and has published book chapters and edited books with reputed publishers. In addition to this, she holds patents related to the health sector. Currently, she is guiding five research scholars under research areas in biomedical applications. Dr. T. Poongodi is presently working as a Professor in the Department of Computer Science and Engineering, School of Engineering, Dayananda Sagar University, Bangalore, India. She received her Ph.D. degree in Information Technology (Information and Communication Engineering) from Anna University, Tamil Nadu, India. Her current research interests include Network Security, Internet of Things (IoT), Data Science and Blockchain Technology for emerging communication networks. She is the author of over 50+ (Scopus Indexed) book chapters including some renowned publishers such as Springer, Elsevier, IET, Wiley, De-Gruyter, CRC Press, IGI global, and 40+ (SCI/Scopus) international journals and conferences. She has published 15+ authored/edited books in Springer, IET, Wiley, CRC Taylor & Francis, and Apple Academic Press. With over 18 years of extensive experience in teaching and multi-disciplinary research, she has been recognized with several prestigious awards, including the Research and Innovation award (2019, 2020, 2021), and Excellence in the area of Research & Innovation/ Academic Excellence / Extension Activities (2018-19, 2019-20) from Galgotias University, Delhi-NCR. She has been invited as a keynote speaker, session chair, program committee and advisory committee member in international conferences. She is a senior member of The Institute of Electrical and Electronics Engineers (IEEE), IEEE Women in Engineering (WIE), and active member in Association for Computing Machinery (ACM). She serves as a Guest Editor for the peer-reviewed international journal BMC Medical Informatics and Decision Making, Springer Nature.
Part I: Introduction to Statistical & Quantum Learning 1: Fundamentals of
Statistics 2: Fundamentals of Quantum Machines Part II: Introduction to
Quantum Machine Learning 3: Machine Learning with Supervised Quantum Models
4: Machine Learning with Unsupervised Quantum Models 5: Artificial Neural
Networks Part III: Quantum Models 6: Quantum Information Science: Bridging
the Gap between the Classical and Quantum Worlds 7: Quantum Machine
Learning Approaches 8: Quantum Classification 9: Boosting in QMLPart IV:
Quantum Evaluation Models 10: Deep Quantum Learning 11: Ensembles and
QBoost 12: Quantum Process Tomography and Regression
Statistics 2: Fundamentals of Quantum Machines Part II: Introduction to
Quantum Machine Learning 3: Machine Learning with Supervised Quantum Models
4: Machine Learning with Unsupervised Quantum Models 5: Artificial Neural
Networks Part III: Quantum Models 6: Quantum Information Science: Bridging
the Gap between the Classical and Quantum Worlds 7: Quantum Machine
Learning Approaches 8: Quantum Classification 9: Boosting in QMLPart IV:
Quantum Evaluation Models 10: Deep Quantum Learning 11: Ensembles and
QBoost 12: Quantum Process Tomography and Regression
Part I: Introduction to Statistical & Quantum Learning 1: Fundamentals of
Statistics 2: Fundamentals of Quantum Machines Part II: Introduction to
Quantum Machine Learning 3: Machine Learning with Supervised Quantum Models
4: Machine Learning with Unsupervised Quantum Models 5: Artificial Neural
Networks Part III: Quantum Models 6: Quantum Information Science: Bridging
the Gap between the Classical and Quantum Worlds 7: Quantum Machine
Learning Approaches 8: Quantum Classification 9: Boosting in QMLPart IV:
Quantum Evaluation Models 10: Deep Quantum Learning 11: Ensembles and
QBoost 12: Quantum Process Tomography and Regression
Statistics 2: Fundamentals of Quantum Machines Part II: Introduction to
Quantum Machine Learning 3: Machine Learning with Supervised Quantum Models
4: Machine Learning with Unsupervised Quantum Models 5: Artificial Neural
Networks Part III: Quantum Models 6: Quantum Information Science: Bridging
the Gap between the Classical and Quantum Worlds 7: Quantum Machine
Learning Approaches 8: Quantum Classification 9: Boosting in QMLPart IV:
Quantum Evaluation Models 10: Deep Quantum Learning 11: Ensembles and
QBoost 12: Quantum Process Tomography and Regression