Alle Infos zum eBook verschenken
- Format: PDF
- Merkliste
- Auf die Merkliste
- Bewerten Bewerten
- Teilen
- Produkt teilen
- Produkterinnerung
- Produkterinnerung
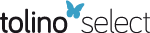
Hier können Sie sich einloggen
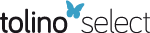
Bitte loggen Sie sich zunächst in Ihr Kundenkonto ein oder registrieren Sie sich bei bücher.de, um das eBook-Abo tolino select nutzen zu können.
Rainfall-Runoff Modelling: The Primer, Second Edition is the follow-up of this popular and authoritative text, first published in 2001. The book provides both a primer for the novice and detailed descriptions of techniques for more advanced practitioners, covering rainfall-runoff models and their practical applications. This new edition extends these aims to include additional chapters dealing with prediction in ungauged basins, predicting residence time distributions, predicting the impacts of change and the next generation of hydrological models. Giving a comprehensive summary of available…mehr
- Geräte: PC
- mit Kopierschutz
- eBook Hilfe
- Größe: 7.39MB
Dieser Download kann aus rechtlichen Gründen nur mit Rechnungsadresse in A, B, BG, CY, CZ, D, DK, EW, E, FIN, F, GR, HR, H, IRL, I, LT, L, LR, M, NL, PL, P, R, S, SLO, SK ausgeliefert werden.
- Produktdetails
- Verlag: John Wiley & Sons
- Seitenzahl: 488
- Erscheinungstermin: 29. November 2011
- Englisch
- ISBN-13: 9781119951018
- Artikelnr.: 37357931
- Verlag: John Wiley & Sons
- Seitenzahl: 488
- Erscheinungstermin: 29. November 2011
- Englisch
- ISBN-13: 9781119951018
- Artikelnr.: 37357931
- Herstellerkennzeichnung Die Herstellerinformationen sind derzeit nicht verfügbar.
About the Author xvii
List of Figures xix
1 Down to Basics: Runoff Processes and the Modelling Process 1
1.1 Why Model? 1
1.2 How to Use This Book 3
1.3 The Modelling Process 3
1.4 Perceptual Models of Catchment Hydrology 6
1.5 Flow Processes and Geochemical Characteristics 13
1.6 Runoff Generation and Runoff Routing 15
1.7 The Problem of Choosing a Conceptual Model 16
1.8 Model Calibration and Validation Issues 18
1.9 Key Points from Chapter 1 21
Box 1.1 The Legacy of Robert Elmer Horton (1875-1945) 22
2 Evolution of Rainfall-Runoff Models: Survival of the Fittest? 25
2.1 The Starting Point: The Rational Method 25
2.2 Practical Prediction: Runoff Coefficients and Time Transformations 26
2.3 Variations on the Unit Hydrograph 33
2.4 Early Digital Computer Models: The Stanford Watershed Model and Its
Descendants 36
2.5 Distributed Process Description Based Models 40
2.6 Simplified Distributed Models Based on Distribution Functions 42
2.7 Recent Developments: What is the Current State of the Art? 43
2.8 Where to Find More on the History and Variety of Rainfall-Runoff Models
43
2.9 Key Points from Chapter 2 44
Box 2.1 Linearity, Nonlinearity and Nonstationarity 45
Box 2.2 The Xinanjiang, ARNO or VIC Model 46
Box 2.3 Control Volumes and Differential Equations 49
3 Data for Rainfall-Runoff Modelling 51
3.1 Rainfall Data 51
3.2 Discharge Data 55
3.3 Meteorological Data and the Estimation of Interception and
Evapotranspiration 56
3.4 Meteorological Data and The Estimation of Snowmelt 60
3.5 Distributing Meteorological Data within a Catchment 61
3.6 Other Hydrological Variables 61
3.7 Digital Elevation Data 61
3.8 Geographical Information and Data Management Systems 66
3.9 Remote-sensing Data 67
3.10 Tracer Data for Understanding Catchment Responses 69
3.11 Linking Model Components and Data Series 70
3.12 Key Points from Chapter 3 71
Box 3.1 The Penman-Monteith Combination Equation for Estimating
Evapotranspiration Rates 72
Box 3.2 Estimating Interception Losses 76
Box 3.3 Estimating Snowmelt by the Degree-Day Method 79
4 Predicting Hydrographs Using Models Based on Data 83
4.1 Data Availability and Empirical Modelling 83
4.2 Doing Hydrology Backwards 84
4.3 Transfer Function Models 87
4.4 Case Study: DBM Modelling of the CI6 Catchment at Llyn Briane, Wales 93
4.5 Physical Derivation of Transfer Functions 95
4.6 Other Methods of Developing Inductive Rainfall-Runoff Models from
Observations 99
4.7 Key Points from Chapter 4 106
Box 4.1 Linear Transfer Function Models 107
Box 4.2 Use of Transfer Functions to Infer Effective Rainfalls 112
Box 4.3 Time Variable Estimation of Transfer Function Parameters and
Derivation of Catchment Nonlinearity 113
5 Predicting Hydrographs Using Distributed Models Based on Process
Descriptions 119
5.1 The Physical Basis of Distributed Models 119
5.2 Physically Based Rainfall-Runoff Models at the Catchment Scale 128
5.3 Case Study: Modelling Flow Processes at Reynolds Creek, Idaho 135
5.4 Case Study: Blind Validation Test of the SHE Model on the Slapton Wood
Catchment 138
5.5 Simplified Distributed Models 140
5.6 Case Study: Distributed Modelling of Runoff Generation at Walnut Gulch,
Arizona 148
5.7 Case Study: Modelling the R-5 Catchment at Chickasha, Oklahoma 151
5.8 Good Practice in the Application of Distributed Models 154
5.9 Discussion of Distributed Models Based on Continuum Differential
Equations 155
5.10 Key Points from Chapter 5 157
Box 5.1 Descriptive Equations for Subsurface Flows 158
Box 5.2 Estimating Infiltration Rates at the Soil Surface 160
Box 5.3 Solution of Partial Differential Equations: Some Basic Concepts 166
Box 5.4 Soil Moisture Characteristic Functions for Use in the Richards
Equation 171
Box 5.5 Pedotransfer Functions 175
Box 5.6 Descriptive Equations for Surface Flows 177
Box 5.7 Derivation of the Kinematic Wave Equation 181
6 Hydrological Similarity, Distribution Functions and Semi-Distributed
Rainfall-Runoff Models 185
6.1 Hydrological Similarity and Hydrological Response Units 185
6.2 The Probability Distributed Moisture (PDM) and Grid to Grid (G2G)
Models 187
6.3 TOPMODEL 190
6.4 Case Study: Application of TOPMODEL to the Saeternbekken Catchment,
Norway 198
6.5 TOPKAPI 203
6.6 Semi-Distributed Hydrological Response Unit (HRU) Models 204
6.7 Some Comments on the HRU Approach 207
6.8 Key Points from Chapter 6 208
Box 6.1 The Theory Underlying TOPMODEL 210
Box 6.2 The Soil and Water Assessment Tool (SWAT) Model 219
Box 6.3 The SCS Curve Number Model Revisited 224
7 Parameter Estimation and Predictive Uncertainty 231
7.1 Model Calibration or Conditioning 231
7.2 Parameter Response Surfaces and Sensitivity Analysis 233
7.3 Performance Measures and Likelihood Measures 239
7.4 Automatic Optimisation Techniques 241
7.5 Recognising Uncertainty in Models and Data: Forward Uncertainty
Estimation 243
7.6 Types of Uncertainty Interval 244
7.7 Model Calibration Using Bayesian Statistical Methods 245
7.8 Dealing with Input Uncertainty in a Bayesian Framework 247
7.9 Model Calibration Using Set Theoretic Methods 249
7.10 Recognising Equifinality: The GLUE Method 252
7.11 Case Study: An Application of the GLUE Methodology in Modelling the
Saeternbekken MINIFELT Catchment, Norway 258
7.12 Case Study: Application of GLUE Limits of Acceptability Approach to
Evaluation in Modelling the Brue Catchment, Somerset, England 261
7.13 Other Applications of GLUE in Rainfall-Runoff Modelling 265
7.14 Comparison of GLUE and Bayesian Approaches to Uncertainty Estimation
266
7.15 Predictive Uncertainty, Risk and Decisions 267
7.16 Dynamic Parameters and Model Structural Error 268
7.17 Quality Control and Disinformation in Rainfall-Runoff Modelling 269
7.18 The Value of Data in Model Conditioning 274
7.19 Key Points from Chapter 7 274
Box 7.1 Likelihood Measures for use in Evaluating Models 276
Box 7.2 Combining Likelihood Measures 283
Box 7.3 Defining the Shape of a Response or Likelihood Surface 284
8 Beyond the Primer: Models for Changing Risk 289
8.1 The Role of Rainfall-Runoff Models in Managing Future Risk 289
8.2 Short-Term Future Risk: Flood Forecasting 290
8.3 Data Requirements for Flood Forecasting 291
8.4 Rainfall-Runoff Modelling for Flood Forecasting 293
8.5 Case Study: Flood Forecasting in the River Eden Catchment, Cumbria,
England 297
8.6 Rainfall-Runoff Modelling for Flood Frequency Estimation 299
8.7 Case Study: Modelling the Flood Frequency Characteristics on the Skalka
Catchment, Czech Republic 302
8.8 Changing Risk: Catchment Change 305
8.9 Changing Risk: Climate Change 307
8.10 Key Points from Chapter 8 309
Box 8.1 Adaptive Gain Parameter Estimation for Real-Time Forecasting 311
9 Beyond the Primer: Next Generation Hydrological Models 313
9.1 Why are New Modelling Techniques Needed? 313
9.2 Representative Elementary Watershed Concepts 315
9.3 How are the REW Concepts Different from Other Hydrological Models? 318
9.4 Implementation of the REW Concepts 318
9.5 Inferring Scale-Dependent Hysteresis from Simplified Hydrological
Theory 320
9.6 Representing Water Fluxes by Particle Tracking 321
9.7 Catchments as Complex Adaptive Systems 324
9.8 Optimality Constraints on Hydrological Responses 325
9.9 Key Points from Chapter 9 327
10 Beyond the Primer: Predictions in Ungauged Basins 329
10.1 The Ungauged Catchment Challenge 329
10.2 The PUB Initiative 330
10.3 The MOPEX Initiative 331
10.4 Ways of Making Predictions in Ungauged Basins 331
10.5 PUB as a Learning Process 332
10.6 Regression of Model Parameters Against Catchment Characteristics 333
10.7 Donor Catchment and Pooling Group Methods 335
10.8 Direct Estimation of Hydrograph Characteristics for Constraining Model
Parameters 336
10.9 Comparing Regionalisation Methods for Model Parameters 338
10.10 HRUs and LSPs as Models of Ungauged Basins 339
10.11 Gauging the Ungauged Basin 339
10.12 Key Points from Chapter 10 341
11 Beyond the Primer:Water Sources and Residence Times in Catchments 343
11.1 Natural and Artificial Tracers 343
11.2 Advection and Dispersion in the Catchment System 345
11.3 Simple Mixing Models 346
11.4 Assessing Spatial Patterns of Incremental Discharge 347
11.5 End Member Mixing Analysis (EMMA) 347
11.6 On the Implications of Tracer Information for Hydrological Processes
348
11.7 Case Study: End Member Mixing with Routing 349
11.8 Residence Time Distribution Models 353
11.9 Case Study: Predicting Tracer Transport at the Gåardsjön Catchment,
Sweden 357
11.10 Implications for Water Quality Models 359
11.11 Key Points from Chapter 11 360
Box 11.1 Representing Advection and Dispersion 361
Box 11.2 Analysing Residence Times in Catchment Systems 365
12 Beyond the Primer: Hypotheses, Measurements and Models of Everywhere
369
12.1 Model Choice in Rainfall-Runoff Modelling as Hypothesis Testing 369
12.2 The Value of Prior Information 371
12.3 Models as Hypotheses 372
12.4 Models of Everywhere 374
12.5 Guidelines for Good Practice 375
12.6 Models of Everywhere and Stakeholder Involvement 376
12.7 Models of Everywhere and Information 377
12.8 Some Final Questions 378
Appendix A Web Resources for Software and Data 381
Appendix B Glossary of Terms 387
References 397
Index 449
About the Author xvii
List of Figures xix
1 Down to Basics: Runoff Processes and the Modelling Process 1
1.1 Why Model? 1
1.2 How to Use This Book 3
1.3 The Modelling Process 3
1.4 Perceptual Models of Catchment Hydrology 6
1.5 Flow Processes and Geochemical Characteristics 13
1.6 Runoff Generation and Runoff Routing 15
1.7 The Problem of Choosing a Conceptual Model 16
1.8 Model Calibration and Validation Issues 18
1.9 Key Points from Chapter 1 21
Box 1.1 The Legacy of Robert Elmer Horton (1875-1945) 22
2 Evolution of Rainfall-Runoff Models: Survival of the Fittest? 25
2.1 The Starting Point: The Rational Method 25
2.2 Practical Prediction: Runoff Coefficients and Time Transformations 26
2.3 Variations on the Unit Hydrograph 33
2.4 Early Digital Computer Models: The Stanford Watershed Model and Its
Descendants 36
2.5 Distributed Process Description Based Models 40
2.6 Simplified Distributed Models Based on Distribution Functions 42
2.7 Recent Developments: What is the Current State of the Art? 43
2.8 Where to Find More on the History and Variety of Rainfall-Runoff Models
43
2.9 Key Points from Chapter 2 44
Box 2.1 Linearity, Nonlinearity and Nonstationarity 45
Box 2.2 The Xinanjiang, ARNO or VIC Model 46
Box 2.3 Control Volumes and Differential Equations 49
3 Data for Rainfall-Runoff Modelling 51
3.1 Rainfall Data 51
3.2 Discharge Data 55
3.3 Meteorological Data and the Estimation of Interception and
Evapotranspiration 56
3.4 Meteorological Data and The Estimation of Snowmelt 60
3.5 Distributing Meteorological Data within a Catchment 61
3.6 Other Hydrological Variables 61
3.7 Digital Elevation Data 61
3.8 Geographical Information and Data Management Systems 66
3.9 Remote-sensing Data 67
3.10 Tracer Data for Understanding Catchment Responses 69
3.11 Linking Model Components and Data Series 70
3.12 Key Points from Chapter 3 71
Box 3.1 The Penman-Monteith Combination Equation for Estimating
Evapotranspiration Rates 72
Box 3.2 Estimating Interception Losses 76
Box 3.3 Estimating Snowmelt by the Degree-Day Method 79
4 Predicting Hydrographs Using Models Based on Data 83
4.1 Data Availability and Empirical Modelling 83
4.2 Doing Hydrology Backwards 84
4.3 Transfer Function Models 87
4.4 Case Study: DBM Modelling of the CI6 Catchment at Llyn Briane, Wales 93
4.5 Physical Derivation of Transfer Functions 95
4.6 Other Methods of Developing Inductive Rainfall-Runoff Models from
Observations 99
4.7 Key Points from Chapter 4 106
Box 4.1 Linear Transfer Function Models 107
Box 4.2 Use of Transfer Functions to Infer Effective Rainfalls 112
Box 4.3 Time Variable Estimation of Transfer Function Parameters and
Derivation of Catchment Nonlinearity 113
5 Predicting Hydrographs Using Distributed Models Based on Process
Descriptions 119
5.1 The Physical Basis of Distributed Models 119
5.2 Physically Based Rainfall-Runoff Models at the Catchment Scale 128
5.3 Case Study: Modelling Flow Processes at Reynolds Creek, Idaho 135
5.4 Case Study: Blind Validation Test of the SHE Model on the Slapton Wood
Catchment 138
5.5 Simplified Distributed Models 140
5.6 Case Study: Distributed Modelling of Runoff Generation at Walnut Gulch,
Arizona 148
5.7 Case Study: Modelling the R-5 Catchment at Chickasha, Oklahoma 151
5.8 Good Practice in the Application of Distributed Models 154
5.9 Discussion of Distributed Models Based on Continuum Differential
Equations 155
5.10 Key Points from Chapter 5 157
Box 5.1 Descriptive Equations for Subsurface Flows 158
Box 5.2 Estimating Infiltration Rates at the Soil Surface 160
Box 5.3 Solution of Partial Differential Equations: Some Basic Concepts 166
Box 5.4 Soil Moisture Characteristic Functions for Use in the Richards
Equation 171
Box 5.5 Pedotransfer Functions 175
Box 5.6 Descriptive Equations for Surface Flows 177
Box 5.7 Derivation of the Kinematic Wave Equation 181
6 Hydrological Similarity, Distribution Functions and Semi-Distributed
Rainfall-Runoff Models 185
6.1 Hydrological Similarity and Hydrological Response Units 185
6.2 The Probability Distributed Moisture (PDM) and Grid to Grid (G2G)
Models 187
6.3 TOPMODEL 190
6.4 Case Study: Application of TOPMODEL to the Saeternbekken Catchment,
Norway 198
6.5 TOPKAPI 203
6.6 Semi-Distributed Hydrological Response Unit (HRU) Models 204
6.7 Some Comments on the HRU Approach 207
6.8 Key Points from Chapter 6 208
Box 6.1 The Theory Underlying TOPMODEL 210
Box 6.2 The Soil and Water Assessment Tool (SWAT) Model 219
Box 6.3 The SCS Curve Number Model Revisited 224
7 Parameter Estimation and Predictive Uncertainty 231
7.1 Model Calibration or Conditioning 231
7.2 Parameter Response Surfaces and Sensitivity Analysis 233
7.3 Performance Measures and Likelihood Measures 239
7.4 Automatic Optimisation Techniques 241
7.5 Recognising Uncertainty in Models and Data: Forward Uncertainty
Estimation 243
7.6 Types of Uncertainty Interval 244
7.7 Model Calibration Using Bayesian Statistical Methods 245
7.8 Dealing with Input Uncertainty in a Bayesian Framework 247
7.9 Model Calibration Using Set Theoretic Methods 249
7.10 Recognising Equifinality: The GLUE Method 252
7.11 Case Study: An Application of the GLUE Methodology in Modelling the
Saeternbekken MINIFELT Catchment, Norway 258
7.12 Case Study: Application of GLUE Limits of Acceptability Approach to
Evaluation in Modelling the Brue Catchment, Somerset, England 261
7.13 Other Applications of GLUE in Rainfall-Runoff Modelling 265
7.14 Comparison of GLUE and Bayesian Approaches to Uncertainty Estimation
266
7.15 Predictive Uncertainty, Risk and Decisions 267
7.16 Dynamic Parameters and Model Structural Error 268
7.17 Quality Control and Disinformation in Rainfall-Runoff Modelling 269
7.18 The Value of Data in Model Conditioning 274
7.19 Key Points from Chapter 7 274
Box 7.1 Likelihood Measures for use in Evaluating Models 276
Box 7.2 Combining Likelihood Measures 283
Box 7.3 Defining the Shape of a Response or Likelihood Surface 284
8 Beyond the Primer: Models for Changing Risk 289
8.1 The Role of Rainfall-Runoff Models in Managing Future Risk 289
8.2 Short-Term Future Risk: Flood Forecasting 290
8.3 Data Requirements for Flood Forecasting 291
8.4 Rainfall-Runoff Modelling for Flood Forecasting 293
8.5 Case Study: Flood Forecasting in the River Eden Catchment, Cumbria,
England 297
8.6 Rainfall-Runoff Modelling for Flood Frequency Estimation 299
8.7 Case Study: Modelling the Flood Frequency Characteristics on the Skalka
Catchment, Czech Republic 302
8.8 Changing Risk: Catchment Change 305
8.9 Changing Risk: Climate Change 307
8.10 Key Points from Chapter 8 309
Box 8.1 Adaptive Gain Parameter Estimation for Real-Time Forecasting 311
9 Beyond the Primer: Next Generation Hydrological Models 313
9.1 Why are New Modelling Techniques Needed? 313
9.2 Representative Elementary Watershed Concepts 315
9.3 How are the REW Concepts Different from Other Hydrological Models? 318
9.4 Implementation of the REW Concepts 318
9.5 Inferring Scale-Dependent Hysteresis from Simplified Hydrological
Theory 320
9.6 Representing Water Fluxes by Particle Tracking 321
9.7 Catchments as Complex Adaptive Systems 324
9.8 Optimality Constraints on Hydrological Responses 325
9.9 Key Points from Chapter 9 327
10 Beyond the Primer: Predictions in Ungauged Basins 329
10.1 The Ungauged Catchment Challenge 329
10.2 The PUB Initiative 330
10.3 The MOPEX Initiative 331
10.4 Ways of Making Predictions in Ungauged Basins 331
10.5 PUB as a Learning Process 332
10.6 Regression of Model Parameters Against Catchment Characteristics 333
10.7 Donor Catchment and Pooling Group Methods 335
10.8 Direct Estimation of Hydrograph Characteristics for Constraining Model
Parameters 336
10.9 Comparing Regionalisation Methods for Model Parameters 338
10.10 HRUs and LSPs as Models of Ungauged Basins 339
10.11 Gauging the Ungauged Basin 339
10.12 Key Points from Chapter 10 341
11 Beyond the Primer:Water Sources and Residence Times in Catchments 343
11.1 Natural and Artificial Tracers 343
11.2 Advection and Dispersion in the Catchment System 345
11.3 Simple Mixing Models 346
11.4 Assessing Spatial Patterns of Incremental Discharge 347
11.5 End Member Mixing Analysis (EMMA) 347
11.6 On the Implications of Tracer Information for Hydrological Processes
348
11.7 Case Study: End Member Mixing with Routing 349
11.8 Residence Time Distribution Models 353
11.9 Case Study: Predicting Tracer Transport at the Gåardsjön Catchment,
Sweden 357
11.10 Implications for Water Quality Models 359
11.11 Key Points from Chapter 11 360
Box 11.1 Representing Advection and Dispersion 361
Box 11.2 Analysing Residence Times in Catchment Systems 365
12 Beyond the Primer: Hypotheses, Measurements and Models of Everywhere
369
12.1 Model Choice in Rainfall-Runoff Modelling as Hypothesis Testing 369
12.2 The Value of Prior Information 371
12.3 Models as Hypotheses 372
12.4 Models of Everywhere 374
12.5 Guidelines for Good Practice 375
12.6 Models of Everywhere and Stakeholder Involvement 376
12.7 Models of Everywhere and Information 377
12.8 Some Final Questions 378
Appendix A Web Resources for Software and Data 381
Appendix B Glossary of Terms 387
References 397
Index 449