38,95 €
38,95 €
inkl. MwSt.
Sofort per Download lieferbar
19 °P sammeln
38,95 €
Als Download kaufen
38,95 €
inkl. MwSt.
Sofort per Download lieferbar
19 °P sammeln
Jetzt verschenken
Alle Infos zum eBook verschenken
38,95 €
inkl. MwSt.
Sofort per Download lieferbar
Alle Infos zum eBook verschenken
19 °P sammeln
- Format: PDF
- Merkliste
- Auf die Merkliste
- Bewerten Bewerten
- Teilen
- Produkt teilen
- Produkterinnerung
- Produkterinnerung
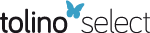
Bitte loggen Sie sich zunächst in Ihr Kundenkonto ein oder registrieren Sie sich bei
bücher.de, um das eBook-Abo tolino select nutzen zu können.
Hier können Sie sich einloggen
Hier können Sie sich einloggen
Sie sind bereits eingeloggt. Klicken Sie auf 2. tolino select Abo, um fortzufahren.
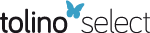
Bitte loggen Sie sich zunächst in Ihr Kundenkonto ein oder registrieren Sie sich bei bücher.de, um das eBook-Abo tolino select nutzen zu können.
A practical approach to using regression and computation to solve real-world problems of estimation, prediction, and causal inference.
- Geräte: PC
- mit Kopierschutz
- eBook Hilfe
- Größe: 42.86MB
Andere Kunden interessierten sich auch für
- Bradley EfronExponential Families in Theory and Practice (eBook, PDF)29,95 €
- Alejandro D. de AcostaLarge Deviations for Markov Chains (eBook, PDF)93,95 €
- Stephanie ParisLeveled Texts for Mathematics (eBook, PDF)36,95 €
- Jeff E. BiddleProgress through Regression (eBook, PDF)97,95 €
- Stacks Project Expository Collection (eBook, PDF)93,95 €
- Andras JuhaszDifferential and Low-Dimensional Topology (eBook, PDF)26,95 €
- Alan FriezeRandom Graphs and Networks: A First Course (eBook, PDF)39,95 €
-
-
-
A practical approach to using regression and computation to solve real-world problems of estimation, prediction, and causal inference.
Dieser Download kann aus rechtlichen Gründen nur mit Rechnungsadresse in A, B, BG, CY, CZ, D, DK, EW, E, FIN, F, GR, HR, H, IRL, I, LT, L, LR, M, NL, PL, P, R, S, SLO, SK ausgeliefert werden.
Produktdetails
- Produktdetails
- Verlag: Cambridge University Press
- Erscheinungstermin: 23. Juli 2020
- Englisch
- ISBN-13: 9781108907354
- Artikelnr.: 70910653
- Verlag: Cambridge University Press
- Erscheinungstermin: 23. Juli 2020
- Englisch
- ISBN-13: 9781108907354
- Artikelnr.: 70910653
The authors are experienced researchers who have published articles in hundreds of different scientific journals in fields including statistics, computer science, policy, public health, political science, economics, sociology, and engineering. They have also published articles in the Washington Post, New York Times, Slate, and other public venues. Their previous books include Bayesian Data Analysis, Teaching Statistics: A Bag of Tricks, and Data Analysis and Regression Using Multilevel/Hierarchical Models. Andrew Gelman is Higgins Professor of Statistics and Professor of Political Science at Columbia University.
Preface
Part I. Fundamentals: 1. Overview
2. Data and measurement
3. Some basic methods in mathematics and probability
4. Statistical inference
5. Simulation
Part II. Linear Regression: 6. Background on regression modeling
7. Linear regression with a single predictor
8. Fitting regression models
9. Prediction and Bayesian inference
10. Linear regression with multiple predictors
11. Assumptions, diagnostics, and model evaluation
12. Transformations and regression
Part III. Generalized Linear Models: 13. Logistic regression
14. Working with logistic regression
15. Other generalized linear models
Part IV. Before and After Fitting a Regression: 16. Design and sample size decisions
17. Poststratification and missing-data imputation
Part V. Causal Inference: 18. Causal inference and randomized experiments
19. Causal inference using regression on the treatment variable
20. Observational studies with all confounders assumed to be measured
21. Additional topics in causal inference
Part VI. What Comes Next?: 22. Advanced regression and multilevel models
Appendices: A. Computing in R
B. 10 quick tips to improve your regression modelling
References
Author index
Subject index.
Part I. Fundamentals: 1. Overview
2. Data and measurement
3. Some basic methods in mathematics and probability
4. Statistical inference
5. Simulation
Part II. Linear Regression: 6. Background on regression modeling
7. Linear regression with a single predictor
8. Fitting regression models
9. Prediction and Bayesian inference
10. Linear regression with multiple predictors
11. Assumptions, diagnostics, and model evaluation
12. Transformations and regression
Part III. Generalized Linear Models: 13. Logistic regression
14. Working with logistic regression
15. Other generalized linear models
Part IV. Before and After Fitting a Regression: 16. Design and sample size decisions
17. Poststratification and missing-data imputation
Part V. Causal Inference: 18. Causal inference and randomized experiments
19. Causal inference using regression on the treatment variable
20. Observational studies with all confounders assumed to be measured
21. Additional topics in causal inference
Part VI. What Comes Next?: 22. Advanced regression and multilevel models
Appendices: A. Computing in R
B. 10 quick tips to improve your regression modelling
References
Author index
Subject index.
Preface
Part I. Fundamentals: 1. Overview
2. Data and measurement
3. Some basic methods in mathematics and probability
4. Statistical inference
5. Simulation
Part II. Linear Regression: 6. Background on regression modeling
7. Linear regression with a single predictor
8. Fitting regression models
9. Prediction and Bayesian inference
10. Linear regression with multiple predictors
11. Assumptions, diagnostics, and model evaluation
12. Transformations and regression
Part III. Generalized Linear Models: 13. Logistic regression
14. Working with logistic regression
15. Other generalized linear models
Part IV. Before and After Fitting a Regression: 16. Design and sample size decisions
17. Poststratification and missing-data imputation
Part V. Causal Inference: 18. Causal inference and randomized experiments
19. Causal inference using regression on the treatment variable
20. Observational studies with all confounders assumed to be measured
21. Additional topics in causal inference
Part VI. What Comes Next?: 22. Advanced regression and multilevel models
Appendices: A. Computing in R
B. 10 quick tips to improve your regression modelling
References
Author index
Subject index.
Part I. Fundamentals: 1. Overview
2. Data and measurement
3. Some basic methods in mathematics and probability
4. Statistical inference
5. Simulation
Part II. Linear Regression: 6. Background on regression modeling
7. Linear regression with a single predictor
8. Fitting regression models
9. Prediction and Bayesian inference
10. Linear regression with multiple predictors
11. Assumptions, diagnostics, and model evaluation
12. Transformations and regression
Part III. Generalized Linear Models: 13. Logistic regression
14. Working with logistic regression
15. Other generalized linear models
Part IV. Before and After Fitting a Regression: 16. Design and sample size decisions
17. Poststratification and missing-data imputation
Part V. Causal Inference: 18. Causal inference and randomized experiments
19. Causal inference using regression on the treatment variable
20. Observational studies with all confounders assumed to be measured
21. Additional topics in causal inference
Part VI. What Comes Next?: 22. Advanced regression and multilevel models
Appendices: A. Computing in R
B. 10 quick tips to improve your regression modelling
References
Author index
Subject index.