Alle Infos zum eBook verschenken
- Format: PDF
- Merkliste
- Auf die Merkliste
- Bewerten Bewerten
- Teilen
- Produkt teilen
- Produkterinnerung
- Produkterinnerung
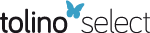
Hier können Sie sich einloggen
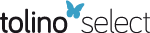
Bitte loggen Sie sich zunächst in Ihr Kundenkonto ein oder registrieren Sie sich bei bücher.de, um das eBook-Abo tolino select nutzen zu können.
We all like to know how reliable and how risky certain situations are, and our increasing reliance on technology has led to the need for more precise assessments than ever before. Such precision has resulted in efforts both to sharpen the notions of risk and reliability, and to quantify them. Quantification is required for normative decision-making, especially decisions pertaining to our safety and wellbeing. Increasingly in recent years Bayesian methods have become key to such quantifications. Reliability and Risk provides a comprehensive overview of the mathematical and statistical aspects…mehr
- Geräte: PC
- mit Kopierschutz
- eBook Hilfe
- Größe: 3.44MB
- Peter CongdonApplied Bayesian Modelling (eBook, PDF)68,99 €
- Timo KoskiBayesian Networks (eBook, PDF)80,99 €
- Peter CongdonBayesian Models for Categorical Data (eBook, PDF)99,99 €
- Bayesian Networks (eBook, PDF)92,99 €
- Dennis V. LindleyUnderstanding Uncertainty, Revised Edition (eBook, PDF)107,99 €
- Michael GoldsteinBayes Linear Statistics (eBook, PDF)134,99 €
- Bayesian Approach to Inverse Problems (eBook, PDF)181,99 €
-
-
-
Dieser Download kann aus rechtlichen Gründen nur mit Rechnungsadresse in A, B, BG, CY, CZ, D, DK, EW, E, FIN, F, GR, HR, H, IRL, I, LT, L, LR, M, NL, PL, P, R, S, SLO, SK ausgeliefert werden.
- Produktdetails
- Verlag: Wiley
- Seitenzahl: 396
- Erscheinungstermin: 14. August 2006
- Englisch
- ISBN-13: 9780470060339
- Artikelnr.: 38190439
- Verlag: Wiley
- Seitenzahl: 396
- Erscheinungstermin: 14. August 2006
- Englisch
- ISBN-13: 9780470060339
- Artikelnr.: 38190439
- Herstellerkennzeichnung Die Herstellerinformationen sind derzeit nicht verfügbar.
Acknowledgements xv
1 Introduction and Overview 1
1.1 Preamble: What do 'Reliability', 'Risk' and 'Robustness' Mean? 1
1.2 Objectives and Prospective Readership 3
1.3 Reliability, Risk and Survival: State-of-the-Art 3
1.4 Risk Management: A Motivation for Risk Analysis 4
1.5 Books on Reliability, Risk and Survival Analysis 6
1.6 Overview of the Book 7
2 The Quantification of Uncertainty 9
2.1 Uncertain Quantities and Uncertain Events: Their Definition and
Codification 9
2.2 Probability: A Satisfactory Way to Quantify Uncertainty 10
2.2.1 The Rules of Probability 11
2.2.2 Justifying the Rules of Probability 12
2.3 Overview of the Different Interpretations of Probability 13
2.3.1 A Brief History of Probability 14
2.3.2 The Different Kinds of Probability 16
2.4 Extending the Rules of Probability: Law of Total Probability and Bayes'
Law 19
2.4.1 Marginalization 20
2.4.2 The Law of Total Probability 20
2.4.3 Bayes' Law: The Incorporation of Evidence and the Likelihood 20
2.5 The Bayesian Paradigm: A Prescription for Reliability, Risk and
Survival Analysis 22
2.6 Probability Models, Parameters, Inference and Prediction 23
2.6.1 The Genesis of Probability Models and Their Parameters 24
2.6.2 Statistical Inference and Probabilistic Prediction 26
2.7 Testing Hypotheses: Posterior Odds and Bayes Factors 27
2.7.1 Bayes Factors: Weight of Evidence and Change in Odds 28
2.7.2 Uses of the Bayes Factor 30
2.7.3 Alternatives to Bayes Factors 31
2.8 Utility as Probability and Maximization of Expected Utility 32
2.8.1 Utility as a Probability 32
2.8.2 Maximization of Expected Utility 33
2.8.3 Attitudes to Risk: The Utility of Money 33
2.9 Decision Trees and Influence Diagrams for Risk Analysis 34
2.9.1 The Decision Tree 34
2.9.2 The Influence Diagram 35
3 Exchangeability and Indifference 45
3.1 Introduction to Exchangeability: de Finetti's Theorem 45
3.1.1 Motivation for the Judgment of Exchangeability 46
3.1.2 Relationship between Independence and Exchangeability 46
3.1.3 de Finetti's Representation Theorem for Zero-one Exchangeable
Sequences 48
3.1.4 Exchangeable Sequences and the Law of Large Numbers 49
3.2 de Finetti-style Theorems for Infinite Sequences of Non-binary Random
Quantities 50
3.2.1 Sufficiency and Indifference in Zero-one Exchangeable Sequences 51
3.2.2 Invariance Conditions Leading to Mixtures of Other Distributions 51
3.3 Error Bounds on de Finetti-style Results for Finite Sequences of Random
Quantities 55
3.3.1 Bounds for Finitely Extendable Zero-one Random Quantities 55
3.3.2 Bounds for Finitely Extendable Non-binary Random Quantities 56
4 Stochastic Models of Failure 59
4.1 Introduction 59
4.2 Preliminaries: Univariate, Multivariate and Multi-indexed Distribution
Functions 59
4.3 The Predictive Failure Rate Function of a Univariate Probability
Distribution 62
4.3.1 The Case of Discontinuity 65
4.4 Interpretation and Uses of the Failure Rate Function - the Model
Failure Rate 66
4.4.1 The True Failure Rate: Does it Exist? 69
4.4.2 Decreasing Failure Rates, Reliability Growth, Burn-in and the Bathtub
Curve 69
4.4.3 The Retrospective (or Reversed) Failure Rate 74
4.5 Multivariate Analogues of the Failure Rate Function 76
4.5.1 The Hazard Gradient 76
4.5.2 The Multivariate Failure Rate Function 77
4.5.3 The Conditional Failure Rate Functions 78
4.6 The Hazard Potential of Items and Individuals 79
4.6.1 Hazard Potentials and Dependent Lifelengths 81
4.6.2 The Hazard Gradient and Conditional Hazard Potentials 83
4.7 Probability Models for Interdependent Lifelengths 85
4.7.1 Preliminaries: Bivariate Distributions 85
4.7.2 The Bivariate Exponential Distributions of Gumbel 89
4.7.3 Freund's Bivariate Exponential Distribution 91
4.7.4 The Bivariate Exponential of Marshall and Olkin 93
4.7.5 The Bivariate Pareto as a Failure Model 107
4.7.6 A Bivariate Exponential Induced by a Shot-noise Process 110
4.7.7 A Bivariate Exponential Induced by a Bivariate Pareto's Copula 115
4.7.8 Other Specialized Bivariate Distributions 115
4.8 Causality and Models for Cascading Failures 117
4.8.1 Probabilistic Causality and Causal Failures 117
4.8.2 Cascading and Models of Cascading Failures 118
4.9 Failure Distributions with Multiple Scales 120
4.9.1 Model Development 120
4.9.2 A Failure Model Indexed by Two Scales 123
5 Parametric Failure Data Analysis 125
5.1 Introduction and Perspective 125
5.2 Assessing Predictive Distributions in the Absence of Data 127
5.2.1 The Exponential as a Chance Distribution 127
5.2.2 The Weibull (and Gamma) as a Chance Distribution 128
5.2.3 The Bernoulli as a Chance Distribution 129
5.2.4 The Poisson as a Chance Distribution 133
5.2.5 The Generalized Gamma as a Chance Distribution 135
5.2.6 The Inverse Gaussian as a Chance Distribution 136
5.3 Prior Distributions in Chance Distributions 136
5.3.1 Eliciting Prior Distributions via Expert Testimonies 137
5.3.2 Using Objective (or Default) Priors 141
5.4 Predictive Distributions Incorporating Failure Data 144
5.4.1 Design Strategies for Industrial Life-testing 145
5.4.2 Stopping Rules: Non-informative and Informative 147
5.4.3 The Total Time on Test 149
5.4.4 Exponential Life-testing Procedures 150
5.4.5 Weibull Life-testing Procedures 155
5.4.6 Life-testing Under the Generalized Gamma and the Inverse Gaussian 156
5.4.7 Bernoulli Life-testing Procedures 157
5.4.8 Life-testing and Inference Under the BVE 159
5.5 Information from Life-tests: Learning from Data 161
5.5.1 Preliminaries: Entropy and Information 161
5.5.2 Learning for Inference from Life-test Data: Testing for Confidence
164
5.5.3 Life-testing for Decision Making: Acceptance Sampling 166
5.6 Optimal Testing: Design of Life-testing Experiments 170
5.7 Adversarial Life-testing and Acceptance Sampling 173
5.8 Accelerated Life-testing and Dose-response Experiments 175
5.8.1 Formulating Accelerated Life-testing Problems 175
5.8.2 The Kalman Filter Model for Prediction and Smoothing 177
5.8.3 Inference from Accelerated Tests Using the Kalman Filter 179
5.8.4 Designing Accelerated Life-testing Experiments 183
6 Composite Reliability: Signatures 187
6.1 Introduction: Hierarchical Models 187
6.2 'Composite Reliability': Partial Exchangeability 188
6.2.1 Simulating Exchangeable and Partially Exchangeable Sequences 189
6.2.2 The Composite Reliability of Ultra-reliable Units 190
6.2.3 Assessing Reliability and Composite Reliability 192
6.3 Signature Analysis and Signatures as Covariates 193
6.3.1 Assessing the Power Spectrum via a Regression Model 195
6.3.2 Bayesian Assessment of the Power Spectrum 195
6.3.3 A Hierarchical Bayes Assessment of the Power Spectrum 198
6.3.4 The Spectrum as a Covariate Using an Accelerated Life Model 200
6.3.5 Closing Remarks on Signatures and Covariates 202
7 Survival in Dynamic Environments 205
7.1 Introduction: Why Stochastic Hazard Functions? 205
7.2 Hazard Rate Processes 206
7.2.1 Hazard Rates as Shot-noise Processes 207
7.2.2 Hazard Rates as Lévy Processes 208
7.2.3 Hazard Rates as Functions of Diffusion Processes 210
7.3 Cumulative Hazard Processes 211
7.3.1 The Cumulative Hazard as a Compound Poisson Process 213
7.3.2 The Cumulative Hazard as an Increasing Lévy Process 213
7.3.3 Cumulative Hazard as Geometric Brownian Motion 214
7.3.4 The Cumulative Hazard as a Markov Additive Process 215
7.4 Competing Risks and Competing Risk Processes 218
7.4.1 Deterministic Competing Risks 219
7.4.2 Stochastic Competing Risks and Competing Risk Processes 220
7.5 Degradation and Aging Processes 222
7.5.1 A Probabilistic Framework for Degradation Modeling 223
7.5.2 Specifying Degradation Processes 223
8 Point Processes for Event Histories 227
8.1 Introduction: What is Event History? 227
8.1.1 Parameterizing the Intensity Function 229
8.2 Other Point Processes in Reliability and Life-testing 229
8.2.1 Multiple Failure Modes and Competing Risks 229
8.2.2 Items Experiencing Degradation and Deterioration 231
8.2.3 Units Experiencing Maintenance and Repair 231
8.2.4 Life-testing Under Censorship and Withdrawals 233
8.3 Multiplicative Intensity and Multivariate Point Processes 234
8.3.1 Multivariate Counting and Intensity Processes 234
8.4 Dynamic Processes and Statistical Models: Martingales 236
8.4.1 Decomposition of Continuous Time Processes 238
8.4.2 Stochastic Integrals and a Martingale Central Limit Theorem 239
8.5 Point Processes with Multiplicative Intensities 240
9 Non-parametric Bayes Methods in Reliability 243
9.1 The What and Why of Non-parametric Bayes 243
9.2 The Dirichlet Distribution and its Variants 244
9.2.1 The Ordered Dirichlet Distribution 246
9.2.2 The Generalized Dirichlet - Concept of Neutrality 246
9.3 A Non-parametric Bayes Approach to Bioassay 247
9.3.1 A Prior for Potency 248
9.3.2 The Posterior Potency 249
9.4 Prior Distributions on the Hazard Function 250
9.4.1 Independent Beta Priors on Piecewise Constant Hazards 250
9.4.2 The Extended Gamma Process as a Prior 251
9.5 Prior Distributions for the Cumulative Hazard Function 253
9.5.1 Neutral to the Right Probabilities and Gamma Process Priors 253
9.5.2 Beta Process Priors for the Cumulative Hazard 255
9.6 Priors for the Cumulative Distribution Function 259
9.6.1 The Dirichlet Process Prior 260
9.6.2 Neutral to the Right-prior Processes 264
10 Survivability of Co-operative, Competing and Vague Systems 269
10.1 Introduction: Notion of Systems and their Components 269
10.1.1 Overview of the Chapter 269
10.2 Coherent Systems and their Qualitative Properties 270
10.2.1 The Reliability of Coherent Systems 274
10.3 The Survivability of Coherent Systems 281
10.3.1 Performance Processes and their Driving Processes 282
10.3.2 System Survivability Under Hierarchical Independence 283
10.3.3 System Survivability Under Interdependence 284
10.3.4 Prior Distributions on the Unit Hypercube 286
10.4 Machine Learning Methods in Survivability Assessment 291
10.4.1 An Overview of the Neural Net Methodology 292
10.4.2 A Two-phased Neural Net for System Survivability 293
10.5 Reliability Allocation: Optimal System Design 294
10.5.1 The Decision Theoretic Formulation 294
10.5.2 Reliability Apportionment for Series Systems 296
10.5.3 Reliability Apportionment for Parallel Redundant Systems 297
10.5.4 Apportioning Node Reliabilities in Networks 298
10.5.5 Apportioning Reliability Under Interdependence 298
10.6 The Utility of Reliability: Optimum System Selection 299
10.6.1 Decision-making for System Selection 300
10.6.2 The Utility of Reliability 301
10.7 Multi-state and Vague Stochastic Systems 303
10.7.1 Vagueness or Imprecision 304
10.7.2 Many-valued Logic: A Synopsis 305
10.7.3 Consistency Profiles and Probabilities of Vague Sets 305
10.7.4 Reliability of Components in Vague Binary States 307
10.7.5 Reliability of Systems in Vague Binary States 307
10.7.6 Concluding Comments on Vague Stochastic Systems 308
11 Reliability and Survival in Econometrics and Finance 309
11.1 Introduction and Overview 309
11.2 Relating Metrics of Reliability to those of Income Inequality 310
11.2.1 Some Metrics of Reliability and Survival 310
11.2.2 Metrics of Income Inequality 311
11.2.3 Relating the Metrics 313
11.2.4 The Entropy of Income Shares 315
11.2.5 Lorenz Curve Analysis of Failure Data 315
11.3 Invoking Reliability Theory in Financial Risk Assessment 317
11.3.1 Asset Pricing of Risk-free Bonds: An Overview 317
11.3.2 Re-interpreting the Exponentiation Formula 319
11.3.3 A Characterization of Present Value Functions 320
11.3.4 Present Value Functions Under Stochastic Interest Rates 325
11.4 Inferential Issues in Asset Pricing 328
11.4.1 Formulating the Inferential Problem 329
11.4.2 A Strategy for Pooling Present Value Functions 329
11.4.3 Illustrative Example: Pooling Present Value Functions 331
11.5 Concluding Comments 332
Appendix A Markov Chain Monté Carlo Simulation 335
A.1 The Gibbs Sampling Algorithm 335
Appendix B Fourier Series Models and the Power Spectrum 339
B.1 Preliminaries: Trigonometric Functions 339
B.2 Orthogonality of Trigonometric Functions 340
B.3 The Fourier Representation of a Finite Sequence of Numbers 341
B.4 Fourier Series Models for Time Series Data 342
B.4.1 The Spectrum and the Periodgram of f(t) 343
Appendix C Network Survivability and Borel's Paradox 345
C.1 Preamble 345
C.2 Re-assessing Testimonies of Experts Who have Vanished 345
C.3 The Paradox in Two Dimensions 346
C.4 The Paradox in Network Survivability Assessment 347
Bibliography 349
Index 365
Acknowledgements xv
1 Introduction and Overview 1
1.1 Preamble: What do 'Reliability', 'Risk' and 'Robustness' Mean? 1
1.2 Objectives and Prospective Readership 3
1.3 Reliability, Risk and Survival: State-of-the-Art 3
1.4 Risk Management: A Motivation for Risk Analysis 4
1.5 Books on Reliability, Risk and Survival Analysis 6
1.6 Overview of the Book 7
2 The Quantification of Uncertainty 9
2.1 Uncertain Quantities and Uncertain Events: Their Definition and
Codification 9
2.2 Probability: A Satisfactory Way to Quantify Uncertainty 10
2.2.1 The Rules of Probability 11
2.2.2 Justifying the Rules of Probability 12
2.3 Overview of the Different Interpretations of Probability 13
2.3.1 A Brief History of Probability 14
2.3.2 The Different Kinds of Probability 16
2.4 Extending the Rules of Probability: Law of Total Probability and Bayes'
Law 19
2.4.1 Marginalization 20
2.4.2 The Law of Total Probability 20
2.4.3 Bayes' Law: The Incorporation of Evidence and the Likelihood 20
2.5 The Bayesian Paradigm: A Prescription for Reliability, Risk and
Survival Analysis 22
2.6 Probability Models, Parameters, Inference and Prediction 23
2.6.1 The Genesis of Probability Models and Their Parameters 24
2.6.2 Statistical Inference and Probabilistic Prediction 26
2.7 Testing Hypotheses: Posterior Odds and Bayes Factors 27
2.7.1 Bayes Factors: Weight of Evidence and Change in Odds 28
2.7.2 Uses of the Bayes Factor 30
2.7.3 Alternatives to Bayes Factors 31
2.8 Utility as Probability and Maximization of Expected Utility 32
2.8.1 Utility as a Probability 32
2.8.2 Maximization of Expected Utility 33
2.8.3 Attitudes to Risk: The Utility of Money 33
2.9 Decision Trees and Influence Diagrams for Risk Analysis 34
2.9.1 The Decision Tree 34
2.9.2 The Influence Diagram 35
3 Exchangeability and Indifference 45
3.1 Introduction to Exchangeability: de Finetti's Theorem 45
3.1.1 Motivation for the Judgment of Exchangeability 46
3.1.2 Relationship between Independence and Exchangeability 46
3.1.3 de Finetti's Representation Theorem for Zero-one Exchangeable
Sequences 48
3.1.4 Exchangeable Sequences and the Law of Large Numbers 49
3.2 de Finetti-style Theorems for Infinite Sequences of Non-binary Random
Quantities 50
3.2.1 Sufficiency and Indifference in Zero-one Exchangeable Sequences 51
3.2.2 Invariance Conditions Leading to Mixtures of Other Distributions 51
3.3 Error Bounds on de Finetti-style Results for Finite Sequences of Random
Quantities 55
3.3.1 Bounds for Finitely Extendable Zero-one Random Quantities 55
3.3.2 Bounds for Finitely Extendable Non-binary Random Quantities 56
4 Stochastic Models of Failure 59
4.1 Introduction 59
4.2 Preliminaries: Univariate, Multivariate and Multi-indexed Distribution
Functions 59
4.3 The Predictive Failure Rate Function of a Univariate Probability
Distribution 62
4.3.1 The Case of Discontinuity 65
4.4 Interpretation and Uses of the Failure Rate Function - the Model
Failure Rate 66
4.4.1 The True Failure Rate: Does it Exist? 69
4.4.2 Decreasing Failure Rates, Reliability Growth, Burn-in and the Bathtub
Curve 69
4.4.3 The Retrospective (or Reversed) Failure Rate 74
4.5 Multivariate Analogues of the Failure Rate Function 76
4.5.1 The Hazard Gradient 76
4.5.2 The Multivariate Failure Rate Function 77
4.5.3 The Conditional Failure Rate Functions 78
4.6 The Hazard Potential of Items and Individuals 79
4.6.1 Hazard Potentials and Dependent Lifelengths 81
4.6.2 The Hazard Gradient and Conditional Hazard Potentials 83
4.7 Probability Models for Interdependent Lifelengths 85
4.7.1 Preliminaries: Bivariate Distributions 85
4.7.2 The Bivariate Exponential Distributions of Gumbel 89
4.7.3 Freund's Bivariate Exponential Distribution 91
4.7.4 The Bivariate Exponential of Marshall and Olkin 93
4.7.5 The Bivariate Pareto as a Failure Model 107
4.7.6 A Bivariate Exponential Induced by a Shot-noise Process 110
4.7.7 A Bivariate Exponential Induced by a Bivariate Pareto's Copula 115
4.7.8 Other Specialized Bivariate Distributions 115
4.8 Causality and Models for Cascading Failures 117
4.8.1 Probabilistic Causality and Causal Failures 117
4.8.2 Cascading and Models of Cascading Failures 118
4.9 Failure Distributions with Multiple Scales 120
4.9.1 Model Development 120
4.9.2 A Failure Model Indexed by Two Scales 123
5 Parametric Failure Data Analysis 125
5.1 Introduction and Perspective 125
5.2 Assessing Predictive Distributions in the Absence of Data 127
5.2.1 The Exponential as a Chance Distribution 127
5.2.2 The Weibull (and Gamma) as a Chance Distribution 128
5.2.3 The Bernoulli as a Chance Distribution 129
5.2.4 The Poisson as a Chance Distribution 133
5.2.5 The Generalized Gamma as a Chance Distribution 135
5.2.6 The Inverse Gaussian as a Chance Distribution 136
5.3 Prior Distributions in Chance Distributions 136
5.3.1 Eliciting Prior Distributions via Expert Testimonies 137
5.3.2 Using Objective (or Default) Priors 141
5.4 Predictive Distributions Incorporating Failure Data 144
5.4.1 Design Strategies for Industrial Life-testing 145
5.4.2 Stopping Rules: Non-informative and Informative 147
5.4.3 The Total Time on Test 149
5.4.4 Exponential Life-testing Procedures 150
5.4.5 Weibull Life-testing Procedures 155
5.4.6 Life-testing Under the Generalized Gamma and the Inverse Gaussian 156
5.4.7 Bernoulli Life-testing Procedures 157
5.4.8 Life-testing and Inference Under the BVE 159
5.5 Information from Life-tests: Learning from Data 161
5.5.1 Preliminaries: Entropy and Information 161
5.5.2 Learning for Inference from Life-test Data: Testing for Confidence
164
5.5.3 Life-testing for Decision Making: Acceptance Sampling 166
5.6 Optimal Testing: Design of Life-testing Experiments 170
5.7 Adversarial Life-testing and Acceptance Sampling 173
5.8 Accelerated Life-testing and Dose-response Experiments 175
5.8.1 Formulating Accelerated Life-testing Problems 175
5.8.2 The Kalman Filter Model for Prediction and Smoothing 177
5.8.3 Inference from Accelerated Tests Using the Kalman Filter 179
5.8.4 Designing Accelerated Life-testing Experiments 183
6 Composite Reliability: Signatures 187
6.1 Introduction: Hierarchical Models 187
6.2 'Composite Reliability': Partial Exchangeability 188
6.2.1 Simulating Exchangeable and Partially Exchangeable Sequences 189
6.2.2 The Composite Reliability of Ultra-reliable Units 190
6.2.3 Assessing Reliability and Composite Reliability 192
6.3 Signature Analysis and Signatures as Covariates 193
6.3.1 Assessing the Power Spectrum via a Regression Model 195
6.3.2 Bayesian Assessment of the Power Spectrum 195
6.3.3 A Hierarchical Bayes Assessment of the Power Spectrum 198
6.3.4 The Spectrum as a Covariate Using an Accelerated Life Model 200
6.3.5 Closing Remarks on Signatures and Covariates 202
7 Survival in Dynamic Environments 205
7.1 Introduction: Why Stochastic Hazard Functions? 205
7.2 Hazard Rate Processes 206
7.2.1 Hazard Rates as Shot-noise Processes 207
7.2.2 Hazard Rates as Lévy Processes 208
7.2.3 Hazard Rates as Functions of Diffusion Processes 210
7.3 Cumulative Hazard Processes 211
7.3.1 The Cumulative Hazard as a Compound Poisson Process 213
7.3.2 The Cumulative Hazard as an Increasing Lévy Process 213
7.3.3 Cumulative Hazard as Geometric Brownian Motion 214
7.3.4 The Cumulative Hazard as a Markov Additive Process 215
7.4 Competing Risks and Competing Risk Processes 218
7.4.1 Deterministic Competing Risks 219
7.4.2 Stochastic Competing Risks and Competing Risk Processes 220
7.5 Degradation and Aging Processes 222
7.5.1 A Probabilistic Framework for Degradation Modeling 223
7.5.2 Specifying Degradation Processes 223
8 Point Processes for Event Histories 227
8.1 Introduction: What is Event History? 227
8.1.1 Parameterizing the Intensity Function 229
8.2 Other Point Processes in Reliability and Life-testing 229
8.2.1 Multiple Failure Modes and Competing Risks 229
8.2.2 Items Experiencing Degradation and Deterioration 231
8.2.3 Units Experiencing Maintenance and Repair 231
8.2.4 Life-testing Under Censorship and Withdrawals 233
8.3 Multiplicative Intensity and Multivariate Point Processes 234
8.3.1 Multivariate Counting and Intensity Processes 234
8.4 Dynamic Processes and Statistical Models: Martingales 236
8.4.1 Decomposition of Continuous Time Processes 238
8.4.2 Stochastic Integrals and a Martingale Central Limit Theorem 239
8.5 Point Processes with Multiplicative Intensities 240
9 Non-parametric Bayes Methods in Reliability 243
9.1 The What and Why of Non-parametric Bayes 243
9.2 The Dirichlet Distribution and its Variants 244
9.2.1 The Ordered Dirichlet Distribution 246
9.2.2 The Generalized Dirichlet - Concept of Neutrality 246
9.3 A Non-parametric Bayes Approach to Bioassay 247
9.3.1 A Prior for Potency 248
9.3.2 The Posterior Potency 249
9.4 Prior Distributions on the Hazard Function 250
9.4.1 Independent Beta Priors on Piecewise Constant Hazards 250
9.4.2 The Extended Gamma Process as a Prior 251
9.5 Prior Distributions for the Cumulative Hazard Function 253
9.5.1 Neutral to the Right Probabilities and Gamma Process Priors 253
9.5.2 Beta Process Priors for the Cumulative Hazard 255
9.6 Priors for the Cumulative Distribution Function 259
9.6.1 The Dirichlet Process Prior 260
9.6.2 Neutral to the Right-prior Processes 264
10 Survivability of Co-operative, Competing and Vague Systems 269
10.1 Introduction: Notion of Systems and their Components 269
10.1.1 Overview of the Chapter 269
10.2 Coherent Systems and their Qualitative Properties 270
10.2.1 The Reliability of Coherent Systems 274
10.3 The Survivability of Coherent Systems 281
10.3.1 Performance Processes and their Driving Processes 282
10.3.2 System Survivability Under Hierarchical Independence 283
10.3.3 System Survivability Under Interdependence 284
10.3.4 Prior Distributions on the Unit Hypercube 286
10.4 Machine Learning Methods in Survivability Assessment 291
10.4.1 An Overview of the Neural Net Methodology 292
10.4.2 A Two-phased Neural Net for System Survivability 293
10.5 Reliability Allocation: Optimal System Design 294
10.5.1 The Decision Theoretic Formulation 294
10.5.2 Reliability Apportionment for Series Systems 296
10.5.3 Reliability Apportionment for Parallel Redundant Systems 297
10.5.4 Apportioning Node Reliabilities in Networks 298
10.5.5 Apportioning Reliability Under Interdependence 298
10.6 The Utility of Reliability: Optimum System Selection 299
10.6.1 Decision-making for System Selection 300
10.6.2 The Utility of Reliability 301
10.7 Multi-state and Vague Stochastic Systems 303
10.7.1 Vagueness or Imprecision 304
10.7.2 Many-valued Logic: A Synopsis 305
10.7.3 Consistency Profiles and Probabilities of Vague Sets 305
10.7.4 Reliability of Components in Vague Binary States 307
10.7.5 Reliability of Systems in Vague Binary States 307
10.7.6 Concluding Comments on Vague Stochastic Systems 308
11 Reliability and Survival in Econometrics and Finance 309
11.1 Introduction and Overview 309
11.2 Relating Metrics of Reliability to those of Income Inequality 310
11.2.1 Some Metrics of Reliability and Survival 310
11.2.2 Metrics of Income Inequality 311
11.2.3 Relating the Metrics 313
11.2.4 The Entropy of Income Shares 315
11.2.5 Lorenz Curve Analysis of Failure Data 315
11.3 Invoking Reliability Theory in Financial Risk Assessment 317
11.3.1 Asset Pricing of Risk-free Bonds: An Overview 317
11.3.2 Re-interpreting the Exponentiation Formula 319
11.3.3 A Characterization of Present Value Functions 320
11.3.4 Present Value Functions Under Stochastic Interest Rates 325
11.4 Inferential Issues in Asset Pricing 328
11.4.1 Formulating the Inferential Problem 329
11.4.2 A Strategy for Pooling Present Value Functions 329
11.4.3 Illustrative Example: Pooling Present Value Functions 331
11.5 Concluding Comments 332
Appendix A Markov Chain Monté Carlo Simulation 335
A.1 The Gibbs Sampling Algorithm 335
Appendix B Fourier Series Models and the Power Spectrum 339
B.1 Preliminaries: Trigonometric Functions 339
B.2 Orthogonality of Trigonometric Functions 340
B.3 The Fourier Representation of a Finite Sequence of Numbers 341
B.4 Fourier Series Models for Time Series Data 342
B.4.1 The Spectrum and the Periodgram of f(t) 343
Appendix C Network Survivability and Borel's Paradox 345
C.1 Preamble 345
C.2 Re-assessing Testimonies of Experts Who have Vanished 345
C.3 The Paradox in Two Dimensions 346
C.4 The Paradox in Network Survivability Assessment 347
Bibliography 349
Index 365
"As the author mentions in his preface, the book can be read inseveral different ways, as a text for a graduate level course onreliability or as a source book for "information and openproblems." This book has been a joy to read for this reviewer."(International Statistical Review, August 2008)
"Singpurwalla seems to be at his best in probabilistic modelingof reality. He has written what must be one of the first booksreliability written from a subjective, Bayesian point of view."(International Statistical Review, August 2008)
"The material of this book will be most profitable forpractitioners and researchers in reliability and survivability, whowill greatly appreciate it as a source of information and openproblems." (Mathematical Reviews, 2008h)
"This is a very interesting, provocative, and worthwhile book."(Biometrics, June 2008)
"What I liked most about this book, however, is the way itblends interesting technical material with foundational discussionabout the nature of uncertainty." (Biometrics, June2008)
"The investigation of the theoretical models under considerationin the book is first class..." (Law, Probability and RiskAdvance Access, September 2007)
"I feel that I have learned an effective plotting technique fromthese plots..." (Technometrics, February 2008)
"...a cornucopia of probability models and inference methodsfor different problems...[that] serve as a rich taxonomy thatstatisticians can use to fit models...works as both aneducational tool and as a reference." (MAA Reviews, March 6,2007)…mehr