Spatio-temporal Design (eBook, ePUB)
Advances in Efficient Data Acquisition
Redaktion: Mateu, Jorge; Müller, Werner G.
Alle Infos zum eBook verschenken
Spatio-temporal Design (eBook, ePUB)
Advances in Efficient Data Acquisition
Redaktion: Mateu, Jorge; Müller, Werner G.
- Format: ePub
- Merkliste
- Auf die Merkliste
- Bewerten Bewerten
- Teilen
- Produkt teilen
- Produkterinnerung
- Produkterinnerung
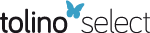
Hier können Sie sich einloggen
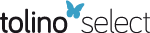
Bitte loggen Sie sich zunächst in Ihr Kundenkonto ein oder registrieren Sie sich bei bücher.de, um das eBook-Abo tolino select nutzen zu können.
A state-of-the-art presentation of optimum spatio-temporal sampling design - bridging classic ideas with modern statistical modeling concepts and the latest computational methods.
Spatio-temporal Design presents a comprehensive state-of-the-art presentation combining both classical and modern treatments of network design and planning for spatial and spatio-temporal data acquisition. A common problem set is interwoven throughout the chapters, providing various perspectives to illustrate a complete insight to the problem at hand.
Motivated by the high demand for statistical analysis of…mehr
- Geräte: eReader
- mit Kopierschutz
- eBook Hilfe
- Größe: 16.79MB
- Timothy C. HaasIntroduction to Probability and Statistics for Ecosystem Managers (eBook, ePUB)76,99 €
- Spatio-temporal Design (eBook, PDF)83,99 €
- N. W. GalweyIntroduction to Mixed Modelling (eBook, ePUB)78,99 €
- Noel CressieStatistics for Spatio-Temporal Data (eBook, ePUB)80,99 €
- Nikolaos LimniosStatistical Methods and Modeling of Seismogenesis (eBook, ePUB)139,99 €
- Michael ShermanSpatial Statistics and Spatio-Temporal Data (eBook, ePUB)84,99 €
- Jean-Paul ChilèsGeostatistics (eBook, ePUB)143,99 €
-
-
-
Spatio-temporal Design presents a comprehensive state-of-the-art presentation combining both classical and modern treatments of network design and planning for spatial and spatio-temporal data acquisition. A common problem set is interwoven throughout the chapters, providing various perspectives to illustrate a complete insight to the problem at hand.
Motivated by the high demand for statistical analysis of data that takes spatial and spatio-temporal information into account, this book incorporates ideas from the areas of time series, spatial statistics and stochastic processes, and combines them to discuss optimum spatio-temporal sampling design.
Spatio-temporal Design: Advances in Efficient Data Acquisition:
- Provides an up-to-date account of how to collect space-time data for monitoring, with a focus on statistical aspects and the latest computational methods
- Discusses basic methods and distinguishes between design and model-based approaches to collecting space-time data.
- Features model-based frequentist design for univariate and multivariate geostatistics, and second-phase spatial sampling.
- Integrates common data examples and case studies throughout the book in order to demonstrate the different approaches and their integration.
- Includes real data sets, data generating mechanisms and simulation scenarios.
- Accompanied by a supporting website featuring R code.
Spatio-temporal Design presents an excellent book for graduate level students as well as a valuable reference for researchers and practitioners in the fields of applied mathematics, engineering, and the environmental and health sciences.
Dieser Download kann aus rechtlichen Gründen nur mit Rechnungsadresse in D ausgeliefert werden.
- Produktdetails
- Verlag: Wiley-IEEE Press
- Erscheinungstermin: 5. November 2012
- Englisch
- ISBN-13: 9781118441886
- Artikelnr.: 37362464
- Verlag: Wiley-IEEE Press
- Erscheinungstermin: 5. November 2012
- Englisch
- ISBN-13: 9781118441886
- Artikelnr.: 37362464
- Herstellerkennzeichnung Die Herstellerinformationen sind derzeit nicht verfügbar.
Foreword xix
1 Collecting spatio-temporal data 1
Jorge Mateu and Werner G. Müller
1.1 Introduction 1
1.2 Paradigms in spatio-temporal design 2
1.3 Paradigms in spatio-temporal modeling 3
1.4 Geostatistics and spatio-temporal random functions 4
1.4.1 Relevant spatio-temporal concepts 4
1.4.2 Properties of the spatio-temporal covariance and variogram functions
6
1.4.3 Spatio-temporal kriging 8
1.4.4 Spatio-temporal covariance models 10
1.4.5 Parametric estimation of spatio-temporal covariograms 11
1.5 Types of design criteria and numerical optimization 13
1.6 The problem set: Upper Austria 17
1.6.1 Climatic data 17
1.6.2 Grassland usage 18
1.7 The chapters 23
Acknowledgments 28
References 28
2 Model-based frequentist design for univariate and multivariate
geostatistics 37
Dale L. Zimmerman and Jie li
2.1 Introduction 37
2.2 Design for univariate geostatistics 38
2.2.1 Data-model framework 38
2.2.2 Design criteria 38
2.2.3 Algorithms 42
2.2.4 Toy example 42
2.3 Design for multivariate geostatistics 45
2.3.1 Data-model framework 45
2.3.2 Design criteria 47
2.3.3 Toy example 48
2.4 Application: Austrian precipitation data network 50
2.5 Conclusions 52
References 53
3 Model-based criteria heuristics for second-phase spatial sampling 54
Eric M. Delmelle
3.1 Introduction 54
3.2 Geometric and geostatistical designs 56
3.2.1 Efficiency of spatial sampling designs 56
3.2.2 Sampling spatial variables in a geostatistical context 57
3.2.3 Sampling designs minimizing the kriging variance 58
3.3 Augmented designs: Second-phase sampling 59
3.3.1 Additional sampling schemes to maximize change in the kriging
variance 59
3.3.2 A weighted kriging variance approach 60
3.4 A simulated annealing approach 63
3.5 Illustration 65
3.5.1 Initial sampling designs 66
3.5.2 Augmented designs 68
3.6 Discussion 68
References 69
4 Spatial sampling design by means of spectral approximations to the error
process 72
Gunter Spöck and Jürgen Pilz
4.1 Introduction 72
4.2 A brief review on spatial sampling design 75
4.3 The spatial mixed linear model 76
4.4 Classical Bayesian experimental design problem 77
4.5 The Smith and Zhu design criterion 79
4.6 Spatial sampling design for trans-Gaussian kriging 81
4.7 The spatDesign toolbox 82
4.7.1 Covariance estimation and variography software 83
4.7.2 Spatial interpolation and kriging software 84
4.7.3 Spatial sampling design software 85
4.8 An example session 89
4.8.1 Preparatory calculations 89
4.8.2 Optimal design for the BSLM 93
4.8.3 Design for the trans-Gaussian kriging 94
4.9 Conclusions 98
References 99
5 Entropy-based network design using hierarchical Bayesian kriging 103
Baisuo Jin, Yuehua Wu and Baiqi Miao
5.1 Introduction 103
5.2 Entropy-based network design using hierarchical Bayesian kriging 105
5.3 The data 107
5.4 Spatio-temporal modeling 107
5.5 Obtaining a staircase data structure 111
5.6 Estimating the hyperparameters H g and the spatial correlations between
gauge stations 113
5.7 Spatial predictive distribution over the 445 areas located in the 18
districts of Upper Austria 117
5.8 Adding gauge stations over the 445 areas located in the 18 districts of
Upper Austria 120
5.9 Closing down an existing gauge station 122
5.10 Model evaluation 124
Appendix 5.1: Hierarchical Bayesian spatio-temporal modeling (or kriging)
124
Appendix 5.2: Some estimated parameters 128
Acknowledgments 129
References 129
6 Accounting for design in the analysis of spatial data 131
Brian J. Reich and Montserrat Fuentes
6.1 Introduction 131
6.2 Modeling approaches 134
6.2.1 Informative missingness 134
6.2.2 Informative sampling 135
6.2.3 A two-stage approach for informative sampling 136
6.3 Analysis of the Austrian precipitation data 137
6.4 Discussion 139
References 141
7 Spatial design for knot selection in knot-based dimension reduction
models 142
Alan E. Gelfand, Sudipto Banerjee and Andrew O. Finley
7.1 Introduction 142
7.2 Handling large spatial datasets 145
7.3 Dimension reduction approaches 146
7.3.1 Basic properties of low rank models 146
7.3.2 Predictive process models: A brief review 148
7.4 Some basic knot design ideas 149
7.4.1 A brief review of spatial design 149
7.4.2 A strategy for selecting knots 151
7.5 Illustrations 153
7.5.1 A simulation example 153
7.5.2 A simulation example using the two-step analysis 159
7.5.3 Tree height and diameter analysis 160
7.5.4 Austria precipitation analysis 162
7.6 Discussion and future work 165
References 166
8 Exploratory designs for assessing spatial dependence 170
Agnes Fussl, Werner G. Müller and Juan Rodríguez-Díaz
8.1 Introduction 170
8.1.1 The dataset and its visualization 172
8.2 Spatial links 174
8.2.1 Spatial neighbors 175
8.2.2 Spatial weights 176
8.3 Measures of spatial dependence 178
8.4 Models for areal data 180
8.4.1 H0 : A spaceless regression model 181
8.4.2 H0 : Spatial regression models 185
8.5 Design considerations 190
8.5.1 A design criterion 192
8.5.2 Example 194
8.6 Discussion 195
Appendix 8.1: R code 198
Acknowledgments 202
References 203
9 Sampling design optimization for space-time kriging 207
Gerard B.M. Heuvelink, Daniel A. Griffith, Tomislav Hengl and Stephanie J.
Melles
9.1 Introduction 207
9.2 Methodology 209
9.2.1 Space-time universal kriging 209
9.2.2 Sampling design optimization with spatial simulated annealing 211
9.3 Upper Austria case study 212
9.3.1 Descriptive statistics 212
9.3.2 Estimation of the space-time model and universal kriging 215
9.3.3 Optimal design scenario 1 218
9.3.4 Optimal design scenario 2 219
9.3.5 Optimal design scenario 3 219
9.4 Discussion and conclusions 221
Appendix 9.1: R code 222
Acknowledgment 227
References 228
10 Space-time adaptive sampling and data transformations 231
José M. Angulo, María C. Bueso and Francisco J. Alonso
10.1 Introduction 231
10.2 Adaptive sampling network design 233
10.2.1 A simulated illustration 235
10.3 Predictive information based on data transformations 238
10.4 Application to Upper Austria temperature data 242
10.5 Summary 246
Acknowledgments 247
References 247
11 Adaptive sampling design for spatio-temporal prediction 249
Thomas R. Fanshawe and Peter J. Diggle
11.1 Introduction 249
11.2 Review of spatial and spatio-temporal adaptive designs 251
11.3 The stationary Gaussian model 253
11.3.1 Model specification 253
11.3.2 Theoretically optimal designs 254
11.3.3 A comparison of design strategies 254
11.4 The dynamic process convolution model 257
11.4.1 Model specification 257
11.4.2 A comparison of design strategies 258
11.5 Upper Austria rainfall data example 262
11.6 Discussion 264
Appendix 11.1 266
References 267
12 Semiparametric dynamic design of monitoring networks for non-Gaussian
spatio-temporal data 269
Scott H. Holan and Christopher K. Wikle
12.1 Introduction 269
12.2 Semiparametric non-Gaussian space-time dynamic design 271
12.2.1 Semiparametric spatio-temporal dynamic Gamma model 271
12.2.2 Simulation-based dynamic design 274
12.2.3 Extended Kalman filter for dynamic gamma models 275
12.2.4 Extended Kalman filter design algorithm 277
12.3 Application: Upper Austria precipitation 278
12.4 Discussion 282
Acknowledgments 282
References 283
13 Active learning for monitoring network optimization 285
Devis Tuia, Alexei Pozdnoukhov, Loris Foresti and Mikhail Kanevski
13.1 Introduction 285
13.2 Statistical learning from data 287
13.2.1 Algorithmic approaches to learning 288
13.2.2 Over-fitting and model selection 288
13.3 Support vector machines and kernel methods 289
13.3.1 Classification: SVMs 290
13.3.2 Density estimation: One-class SVM 292
13.3.3 Regression: Kernel ridge regression 293
13.3.4 Regression: SVR 294
13.4 Active learning 294
13.4.1 A general framework 295
13.4.2 First steps in active learning: Reducing output variance 296
13.4.3 Exploration-exploitation strategies: Towards mixed approaches 297
13.5 Active learning with SVMs 297
13.5.1 Margin sampling 297
13.5.2 Diversity of batches of samples 299
13.5.3 Committees of models 299
13.6 Case studies 300
13.6.1 Austrian climatological data 300
13.6.2 Cesium-137 concentration after Chernobyl 304
13.6.3 Wind power plants sites evaluation 307
13.7 Conclusions 312
Acknowledgments 314
References 314
14 Stationary sampling designs based on plume simulations 319
Kristina B. Helle and Edzer Pebesma
14.1 Introduction 319
14.2 Plumes: From random fields to simulations 320
14.3 Cost functions 324
14.3.1 Detecting plumes 324
14.3.2 Mapping and characterising plumes 325
14.3.3 Combined cost functions 325
14.4 Optimisation 326
14.4.1 Greedy search 326
14.4.2 Spatial simulated annealing 328
14.4.3 Genetic algorithms 329
14.4.4 Other methods 331
14.4.5 Evaluation and sensitivity 331
14.4.6 Use case: Combination and comparison of optimisation algorithms 332
14.5 Results 334
14.5.1 Simulations 334
14.5.2 Greedy search 335
14.5.3 Sensitivity of greedy search to the plume simulations 336
14.5.4 Comparison of optimisation algorithms 337
14.6 Discussion 340
Acknowledgments 341
References 341
Index 345
Foreword xix
1 Collecting spatio-temporal data 1
Jorge Mateu and Werner G. Müller
1.1 Introduction 1
1.2 Paradigms in spatio-temporal design 2
1.3 Paradigms in spatio-temporal modeling 3
1.4 Geostatistics and spatio-temporal random functions 4
1.4.1 Relevant spatio-temporal concepts 4
1.4.2 Properties of the spatio-temporal covariance and variogram functions
6
1.4.3 Spatio-temporal kriging 8
1.4.4 Spatio-temporal covariance models 10
1.4.5 Parametric estimation of spatio-temporal covariograms 11
1.5 Types of design criteria and numerical optimization 13
1.6 The problem set: Upper Austria 17
1.6.1 Climatic data 17
1.6.2 Grassland usage 18
1.7 The chapters 23
Acknowledgments 28
References 28
2 Model-based frequentist design for univariate and multivariate
geostatistics 37
Dale L. Zimmerman and Jie li
2.1 Introduction 37
2.2 Design for univariate geostatistics 38
2.2.1 Data-model framework 38
2.2.2 Design criteria 38
2.2.3 Algorithms 42
2.2.4 Toy example 42
2.3 Design for multivariate geostatistics 45
2.3.1 Data-model framework 45
2.3.2 Design criteria 47
2.3.3 Toy example 48
2.4 Application: Austrian precipitation data network 50
2.5 Conclusions 52
References 53
3 Model-based criteria heuristics for second-phase spatial sampling 54
Eric M. Delmelle
3.1 Introduction 54
3.2 Geometric and geostatistical designs 56
3.2.1 Efficiency of spatial sampling designs 56
3.2.2 Sampling spatial variables in a geostatistical context 57
3.2.3 Sampling designs minimizing the kriging variance 58
3.3 Augmented designs: Second-phase sampling 59
3.3.1 Additional sampling schemes to maximize change in the kriging
variance 59
3.3.2 A weighted kriging variance approach 60
3.4 A simulated annealing approach 63
3.5 Illustration 65
3.5.1 Initial sampling designs 66
3.5.2 Augmented designs 68
3.6 Discussion 68
References 69
4 Spatial sampling design by means of spectral approximations to the error
process 72
Gunter Spöck and Jürgen Pilz
4.1 Introduction 72
4.2 A brief review on spatial sampling design 75
4.3 The spatial mixed linear model 76
4.4 Classical Bayesian experimental design problem 77
4.5 The Smith and Zhu design criterion 79
4.6 Spatial sampling design for trans-Gaussian kriging 81
4.7 The spatDesign toolbox 82
4.7.1 Covariance estimation and variography software 83
4.7.2 Spatial interpolation and kriging software 84
4.7.3 Spatial sampling design software 85
4.8 An example session 89
4.8.1 Preparatory calculations 89
4.8.2 Optimal design for the BSLM 93
4.8.3 Design for the trans-Gaussian kriging 94
4.9 Conclusions 98
References 99
5 Entropy-based network design using hierarchical Bayesian kriging 103
Baisuo Jin, Yuehua Wu and Baiqi Miao
5.1 Introduction 103
5.2 Entropy-based network design using hierarchical Bayesian kriging 105
5.3 The data 107
5.4 Spatio-temporal modeling 107
5.5 Obtaining a staircase data structure 111
5.6 Estimating the hyperparameters H g and the spatial correlations between
gauge stations 113
5.7 Spatial predictive distribution over the 445 areas located in the 18
districts of Upper Austria 117
5.8 Adding gauge stations over the 445 areas located in the 18 districts of
Upper Austria 120
5.9 Closing down an existing gauge station 122
5.10 Model evaluation 124
Appendix 5.1: Hierarchical Bayesian spatio-temporal modeling (or kriging)
124
Appendix 5.2: Some estimated parameters 128
Acknowledgments 129
References 129
6 Accounting for design in the analysis of spatial data 131
Brian J. Reich and Montserrat Fuentes
6.1 Introduction 131
6.2 Modeling approaches 134
6.2.1 Informative missingness 134
6.2.2 Informative sampling 135
6.2.3 A two-stage approach for informative sampling 136
6.3 Analysis of the Austrian precipitation data 137
6.4 Discussion 139
References 141
7 Spatial design for knot selection in knot-based dimension reduction
models 142
Alan E. Gelfand, Sudipto Banerjee and Andrew O. Finley
7.1 Introduction 142
7.2 Handling large spatial datasets 145
7.3 Dimension reduction approaches 146
7.3.1 Basic properties of low rank models 146
7.3.2 Predictive process models: A brief review 148
7.4 Some basic knot design ideas 149
7.4.1 A brief review of spatial design 149
7.4.2 A strategy for selecting knots 151
7.5 Illustrations 153
7.5.1 A simulation example 153
7.5.2 A simulation example using the two-step analysis 159
7.5.3 Tree height and diameter analysis 160
7.5.4 Austria precipitation analysis 162
7.6 Discussion and future work 165
References 166
8 Exploratory designs for assessing spatial dependence 170
Agnes Fussl, Werner G. Müller and Juan Rodríguez-Díaz
8.1 Introduction 170
8.1.1 The dataset and its visualization 172
8.2 Spatial links 174
8.2.1 Spatial neighbors 175
8.2.2 Spatial weights 176
8.3 Measures of spatial dependence 178
8.4 Models for areal data 180
8.4.1 H0 : A spaceless regression model 181
8.4.2 H0 : Spatial regression models 185
8.5 Design considerations 190
8.5.1 A design criterion 192
8.5.2 Example 194
8.6 Discussion 195
Appendix 8.1: R code 198
Acknowledgments 202
References 203
9 Sampling design optimization for space-time kriging 207
Gerard B.M. Heuvelink, Daniel A. Griffith, Tomislav Hengl and Stephanie J.
Melles
9.1 Introduction 207
9.2 Methodology 209
9.2.1 Space-time universal kriging 209
9.2.2 Sampling design optimization with spatial simulated annealing 211
9.3 Upper Austria case study 212
9.3.1 Descriptive statistics 212
9.3.2 Estimation of the space-time model and universal kriging 215
9.3.3 Optimal design scenario 1 218
9.3.4 Optimal design scenario 2 219
9.3.5 Optimal design scenario 3 219
9.4 Discussion and conclusions 221
Appendix 9.1: R code 222
Acknowledgment 227
References 228
10 Space-time adaptive sampling and data transformations 231
José M. Angulo, María C. Bueso and Francisco J. Alonso
10.1 Introduction 231
10.2 Adaptive sampling network design 233
10.2.1 A simulated illustration 235
10.3 Predictive information based on data transformations 238
10.4 Application to Upper Austria temperature data 242
10.5 Summary 246
Acknowledgments 247
References 247
11 Adaptive sampling design for spatio-temporal prediction 249
Thomas R. Fanshawe and Peter J. Diggle
11.1 Introduction 249
11.2 Review of spatial and spatio-temporal adaptive designs 251
11.3 The stationary Gaussian model 253
11.3.1 Model specification 253
11.3.2 Theoretically optimal designs 254
11.3.3 A comparison of design strategies 254
11.4 The dynamic process convolution model 257
11.4.1 Model specification 257
11.4.2 A comparison of design strategies 258
11.5 Upper Austria rainfall data example 262
11.6 Discussion 264
Appendix 11.1 266
References 267
12 Semiparametric dynamic design of monitoring networks for non-Gaussian
spatio-temporal data 269
Scott H. Holan and Christopher K. Wikle
12.1 Introduction 269
12.2 Semiparametric non-Gaussian space-time dynamic design 271
12.2.1 Semiparametric spatio-temporal dynamic Gamma model 271
12.2.2 Simulation-based dynamic design 274
12.2.3 Extended Kalman filter for dynamic gamma models 275
12.2.4 Extended Kalman filter design algorithm 277
12.3 Application: Upper Austria precipitation 278
12.4 Discussion 282
Acknowledgments 282
References 283
13 Active learning for monitoring network optimization 285
Devis Tuia, Alexei Pozdnoukhov, Loris Foresti and Mikhail Kanevski
13.1 Introduction 285
13.2 Statistical learning from data 287
13.2.1 Algorithmic approaches to learning 288
13.2.2 Over-fitting and model selection 288
13.3 Support vector machines and kernel methods 289
13.3.1 Classification: SVMs 290
13.3.2 Density estimation: One-class SVM 292
13.3.3 Regression: Kernel ridge regression 293
13.3.4 Regression: SVR 294
13.4 Active learning 294
13.4.1 A general framework 295
13.4.2 First steps in active learning: Reducing output variance 296
13.4.3 Exploration-exploitation strategies: Towards mixed approaches 297
13.5 Active learning with SVMs 297
13.5.1 Margin sampling 297
13.5.2 Diversity of batches of samples 299
13.5.3 Committees of models 299
13.6 Case studies 300
13.6.1 Austrian climatological data 300
13.6.2 Cesium-137 concentration after Chernobyl 304
13.6.3 Wind power plants sites evaluation 307
13.7 Conclusions 312
Acknowledgments 314
References 314
14 Stationary sampling designs based on plume simulations 319
Kristina B. Helle and Edzer Pebesma
14.1 Introduction 319
14.2 Plumes: From random fields to simulations 320
14.3 Cost functions 324
14.3.1 Detecting plumes 324
14.3.2 Mapping and characterising plumes 325
14.3.3 Combined cost functions 325
14.4 Optimisation 326
14.4.1 Greedy search 326
14.4.2 Spatial simulated annealing 328
14.4.3 Genetic algorithms 329
14.4.4 Other methods 331
14.4.5 Evaluation and sensitivity 331
14.4.6 Use case: Combination and comparison of optimisation algorithms 332
14.5 Results 334
14.5.1 Simulations 334
14.5.2 Greedy search 335
14.5.3 Sensitivity of greedy search to the plume simulations 336
14.5.4 Comparison of optimisation algorithms 337
14.6 Discussion 340
Acknowledgments 341
References 341
Index 345