Natalie Heisler, Maura R. Grossman
Standards for the Control of Algorithmic Bias (eBook, ePUB)
The Canadian Administrative Context
20,95 €
20,95 €
inkl. MwSt.
Sofort per Download lieferbar
10 °P sammeln
20,95 €
Als Download kaufen
20,95 €
inkl. MwSt.
Sofort per Download lieferbar
10 °P sammeln
Jetzt verschenken
Alle Infos zum eBook verschenken
20,95 €
inkl. MwSt.
Sofort per Download lieferbar
Alle Infos zum eBook verschenken
10 °P sammeln
Natalie Heisler, Maura R. Grossman
Standards for the Control of Algorithmic Bias (eBook, ePUB)
The Canadian Administrative Context
- Format: ePub
- Merkliste
- Auf die Merkliste
- Bewerten Bewerten
- Teilen
- Produkt teilen
- Produkterinnerung
- Produkterinnerung
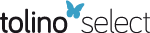
Bitte loggen Sie sich zunächst in Ihr Kundenkonto ein oder registrieren Sie sich bei
bücher.de, um das eBook-Abo tolino select nutzen zu können.
Hier können Sie sich einloggen
Hier können Sie sich einloggen
Sie sind bereits eingeloggt. Klicken Sie auf 2. tolino select Abo, um fortzufahren.
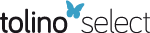
Bitte loggen Sie sich zunächst in Ihr Kundenkonto ein oder registrieren Sie sich bei bücher.de, um das eBook-Abo tolino select nutzen zu können.
Governments around the world use machine learning in automated decision-making systems for a broad range of functions. This book seeks to answer the question: what standards should be applied to machine learning to mitigate disparate impact in automated decision-making?
- Geräte: eReader
- mit Kopierschutz
- eBook Hilfe
Andere Kunden interessierten sich auch für
- Natalie HeislerStandards for the Control of Algorithmic Bias (eBook, PDF)20,95 €
- Roger BrownswordLaw 3.0 (eBook, ePUB)21,95 €
- AI, Machine Learning and Deep Learning (eBook, ePUB)46,95 €
- Tine MunkThe Rise of Politically Motivated Cyber Attacks (eBook, ePUB)43,95 €
- The GDPR Challenge (eBook, ePUB)85,95 €
- Victoria BainesRhetoric of InSecurity (eBook, ePUB)39,95 €
- Roger BrownswordLaw 3.0 (eBook, PDF)20,95 €
-
-
-
Governments around the world use machine learning in automated decision-making systems for a broad range of functions. This book seeks to answer the question: what standards should be applied to machine learning to mitigate disparate impact in automated decision-making?
Dieser Download kann aus rechtlichen Gründen nur mit Rechnungsadresse in A, B, BG, CY, CZ, D, DK, EW, E, FIN, F, GR, HR, H, IRL, I, LT, L, LR, M, NL, PL, P, R, S, SLO, SK ausgeliefert werden.
Produktdetails
- Produktdetails
- Verlag: Taylor & Francis
- Seitenzahl: 108
- Erscheinungstermin: 4. Juli 2023
- Englisch
- ISBN-13: 9781000927580
- Artikelnr.: 68308372
- Verlag: Taylor & Francis
- Seitenzahl: 108
- Erscheinungstermin: 4. Juli 2023
- Englisch
- ISBN-13: 9781000927580
- Artikelnr.: 68308372
- Herstellerkennzeichnung Die Herstellerinformationen sind derzeit nicht verfügbar.
Natalie Heisler has advised public- and private-sector organizations around the world in the strategy and deployment of data, analytics, and artificial intelligence for more than twenty years. Natalie brings a unique, multidisciplinary perspective to her work, spanning social, regulatory, policy, and technical dimensions. Natalie has a BA in Psychology, an MSc in Mathematics, and an MA in Political Science and lives in Toronto, Canada.
Maura R. Grossman, JD, PhD, is a research professor in the David R. Cheriton School of Computer Science at the University of Waterloo and an affiliate faculty member at the Vector Institute of Artificial Intelligence, both in Ontario, Canada. She also is principal at Maura Grossman Law, in Buffalo, New York, USA. Professor Grossman's multidisciplinary work falls at the intersection of law, health, technology, ethics, and policy.
Maura R. Grossman, JD, PhD, is a research professor in the David R. Cheriton School of Computer Science at the University of Waterloo and an affiliate faculty member at the Vector Institute of Artificial Intelligence, both in Ontario, Canada. She also is principal at Maura Grossman Law, in Buffalo, New York, USA. Professor Grossman's multidisciplinary work falls at the intersection of law, health, technology, ethics, and policy.
Acknowledgements *
List of Tables *
List of Abbreviations *
Chapter One: Introduction *
1.1 Regulation of Artificial Intelligence: The European Context *
1.2 Regulation of Artificial Intelligence: The Canadian Administrative
Context *
1.3 Equality Rights: Disparate Impact in ADM *
1.3.1 Case Study: Disparate Impact in the COMPAS ADM *
1.4 Situating Disparate Impact in the Charter *
1.5 The Role of Standards in Protecting Human Rights *
1.5.1 Narrowing the Scope of Administrative Law *
1.5.2 Soft Law and Its Status in Judicial Review *
1.6 Methodology *
Chapter Two: Administrative Law and Standards for the Control of
Algorithmic Bias *
2.1 Foundational Principles: Transparency, Deference and Proportionality *
2.1.1 Transparency *
2.1.2 Deference *
2.1.3 Proportionality *
2.2 Reasonableness Review *
2.2.1 Illustrative Scenario *
2.3 Standards to Mitigate the Creation of Biased Predictions *
2.3.1 Construct Validity *
2.3.2 Representativeness of Input Data *
2.3.3 Knowledge Limits *
2.3.4 Measurement Validity in Model Inputs *
2.3.5 Measurement Validity in Output Variables *
2.3.6 Accuracy of Input Data *
2.4 Standards for the Evaluation of Predictions *
2.4.1 Accuracy of Predictions and Inferences: Uncertainty *
2.4.2 Individual Fairness *
2.5 Chapter Summary: Proposed Standards for the Control of Algorithmic Bias
*
Chapter Three: Substantive Equality and Standards for the Measurement of
Disparity *
3.1 The Measure of Disparity in the Prima Facie Test of Discrimination *
3.2 Legislative and Policy Approaches to the Measurement of Disparity *
3.3 The Supreme Court of Canada on Measures of Disparity in Fraser *
3.4 Disaggregated Data *
3.5 Chapter Summary: Standards for the Measurement of Disparity *
Chapter Four: Implementation Recommendations *
4.1 Overview of the Standards Framework *
4.2 Implementing the Standards Framework *
Chapter Five: Conclusions and Further Research *
References *
List of Tables *
List of Abbreviations *
Chapter One: Introduction *
1.1 Regulation of Artificial Intelligence: The European Context *
1.2 Regulation of Artificial Intelligence: The Canadian Administrative
Context *
1.3 Equality Rights: Disparate Impact in ADM *
1.3.1 Case Study: Disparate Impact in the COMPAS ADM *
1.4 Situating Disparate Impact in the Charter *
1.5 The Role of Standards in Protecting Human Rights *
1.5.1 Narrowing the Scope of Administrative Law *
1.5.2 Soft Law and Its Status in Judicial Review *
1.6 Methodology *
Chapter Two: Administrative Law and Standards for the Control of
Algorithmic Bias *
2.1 Foundational Principles: Transparency, Deference and Proportionality *
2.1.1 Transparency *
2.1.2 Deference *
2.1.3 Proportionality *
2.2 Reasonableness Review *
2.2.1 Illustrative Scenario *
2.3 Standards to Mitigate the Creation of Biased Predictions *
2.3.1 Construct Validity *
2.3.2 Representativeness of Input Data *
2.3.3 Knowledge Limits *
2.3.4 Measurement Validity in Model Inputs *
2.3.5 Measurement Validity in Output Variables *
2.3.6 Accuracy of Input Data *
2.4 Standards for the Evaluation of Predictions *
2.4.1 Accuracy of Predictions and Inferences: Uncertainty *
2.4.2 Individual Fairness *
2.5 Chapter Summary: Proposed Standards for the Control of Algorithmic Bias
*
Chapter Three: Substantive Equality and Standards for the Measurement of
Disparity *
3.1 The Measure of Disparity in the Prima Facie Test of Discrimination *
3.2 Legislative and Policy Approaches to the Measurement of Disparity *
3.3 The Supreme Court of Canada on Measures of Disparity in Fraser *
3.4 Disaggregated Data *
3.5 Chapter Summary: Standards for the Measurement of Disparity *
Chapter Four: Implementation Recommendations *
4.1 Overview of the Standards Framework *
4.2 Implementing the Standards Framework *
Chapter Five: Conclusions and Further Research *
References *
Acknowledgements *
List of Tables *
List of Abbreviations *
Chapter One: Introduction *
1.1 Regulation of Artificial Intelligence: The European Context *
1.2 Regulation of Artificial Intelligence: The Canadian Administrative
Context *
1.3 Equality Rights: Disparate Impact in ADM *
1.3.1 Case Study: Disparate Impact in the COMPAS ADM *
1.4 Situating Disparate Impact in the Charter *
1.5 The Role of Standards in Protecting Human Rights *
1.5.1 Narrowing the Scope of Administrative Law *
1.5.2 Soft Law and Its Status in Judicial Review *
1.6 Methodology *
Chapter Two: Administrative Law and Standards for the Control of
Algorithmic Bias *
2.1 Foundational Principles: Transparency, Deference and Proportionality *
2.1.1 Transparency *
2.1.2 Deference *
2.1.3 Proportionality *
2.2 Reasonableness Review *
2.2.1 Illustrative Scenario *
2.3 Standards to Mitigate the Creation of Biased Predictions *
2.3.1 Construct Validity *
2.3.2 Representativeness of Input Data *
2.3.3 Knowledge Limits *
2.3.4 Measurement Validity in Model Inputs *
2.3.5 Measurement Validity in Output Variables *
2.3.6 Accuracy of Input Data *
2.4 Standards for the Evaluation of Predictions *
2.4.1 Accuracy of Predictions and Inferences: Uncertainty *
2.4.2 Individual Fairness *
2.5 Chapter Summary: Proposed Standards for the Control of Algorithmic Bias
*
Chapter Three: Substantive Equality and Standards for the Measurement of
Disparity *
3.1 The Measure of Disparity in the Prima Facie Test of Discrimination *
3.2 Legislative and Policy Approaches to the Measurement of Disparity *
3.3 The Supreme Court of Canada on Measures of Disparity in Fraser *
3.4 Disaggregated Data *
3.5 Chapter Summary: Standards for the Measurement of Disparity *
Chapter Four: Implementation Recommendations *
4.1 Overview of the Standards Framework *
4.2 Implementing the Standards Framework *
Chapter Five: Conclusions and Further Research *
References *
List of Tables *
List of Abbreviations *
Chapter One: Introduction *
1.1 Regulation of Artificial Intelligence: The European Context *
1.2 Regulation of Artificial Intelligence: The Canadian Administrative
Context *
1.3 Equality Rights: Disparate Impact in ADM *
1.3.1 Case Study: Disparate Impact in the COMPAS ADM *
1.4 Situating Disparate Impact in the Charter *
1.5 The Role of Standards in Protecting Human Rights *
1.5.1 Narrowing the Scope of Administrative Law *
1.5.2 Soft Law and Its Status in Judicial Review *
1.6 Methodology *
Chapter Two: Administrative Law and Standards for the Control of
Algorithmic Bias *
2.1 Foundational Principles: Transparency, Deference and Proportionality *
2.1.1 Transparency *
2.1.2 Deference *
2.1.3 Proportionality *
2.2 Reasonableness Review *
2.2.1 Illustrative Scenario *
2.3 Standards to Mitigate the Creation of Biased Predictions *
2.3.1 Construct Validity *
2.3.2 Representativeness of Input Data *
2.3.3 Knowledge Limits *
2.3.4 Measurement Validity in Model Inputs *
2.3.5 Measurement Validity in Output Variables *
2.3.6 Accuracy of Input Data *
2.4 Standards for the Evaluation of Predictions *
2.4.1 Accuracy of Predictions and Inferences: Uncertainty *
2.4.2 Individual Fairness *
2.5 Chapter Summary: Proposed Standards for the Control of Algorithmic Bias
*
Chapter Three: Substantive Equality and Standards for the Measurement of
Disparity *
3.1 The Measure of Disparity in the Prima Facie Test of Discrimination *
3.2 Legislative and Policy Approaches to the Measurement of Disparity *
3.3 The Supreme Court of Canada on Measures of Disparity in Fraser *
3.4 Disaggregated Data *
3.5 Chapter Summary: Standards for the Measurement of Disparity *
Chapter Four: Implementation Recommendations *
4.1 Overview of the Standards Framework *
4.2 Implementing the Standards Framework *
Chapter Five: Conclusions and Further Research *
References *