Statistical Advances in the Biomedical Sciences (eBook, PDF)
Clinical Trials, Epidemiology, Survival Analysis, and Bioinformatics
Redaktion: Biswas, Atanu; Segal, Mark R.; Fine, Jason P.; Datta, Sujay
Alle Infos zum eBook verschenken
Statistical Advances in the Biomedical Sciences (eBook, PDF)
Clinical Trials, Epidemiology, Survival Analysis, and Bioinformatics
Redaktion: Biswas, Atanu; Segal, Mark R.; Fine, Jason P.; Datta, Sujay
- Format: PDF
- Merkliste
- Auf die Merkliste
- Bewerten Bewerten
- Teilen
- Produkt teilen
- Produkterinnerung
- Produkterinnerung
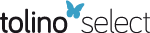
Hier können Sie sich einloggen
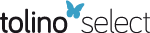
Bitte loggen Sie sich zunächst in Ihr Kundenkonto ein oder registrieren Sie sich bei bücher.de, um das eBook-Abo tolino select nutzen zu können.
The Most Comprehensive and Cutting-Edge Guide to Statistical Applications in Biomedical Research With the increasing use of biotechnology in medical research and the sophisticated advances in computing, it has become essential for practitioners in the biomedical sciences to be fully educated on the role statistics plays in ensuring the accurate analysis of research findings. Statistical Advances in the Biomedical Sciences explores the growing value of statistical knowledge in the management and comprehension of medical research and, more specifically, provides an accessible introduction to the…mehr
- Geräte: PC
- mit Kopierschutz
- eBook Hilfe
- Größe: 7.98MB
- Robert F. WoolsonStatistical Methods for the Analysis of Biomedical Data (eBook, PDF)173,99 €
- Gilberto LevyThe Biostatistics of Aging (eBook, PDF)99,99 €
- Encyclopaedic Companion to Medical Statistics (eBook, PDF)60,99 €
- Sik-Yum LeeBasic and Advanced Bayesian Structural Equation Modeling (eBook, PDF)83,99 €
- Methods and Applications of Statistics in Clinical Trials, Volume 1 (eBook, PDF)181,99 €
- Michael O'KellyClinical Trials with Missing Data (eBook, PDF)71,99 €
- Methods and Applications of Statistics in Clinical Trials, Volume 2 (eBook, PDF)177,99 €
-
-
-
Dieser Download kann aus rechtlichen Gründen nur mit Rechnungsadresse in A, B, BG, CY, CZ, D, DK, EW, E, FIN, F, GR, HR, H, IRL, I, LT, L, LR, M, NL, PL, P, R, S, SLO, SK ausgeliefert werden.
- Produktdetails
- Verlag: John Wiley & Sons
- Seitenzahl: 616
- Erscheinungstermin: 28. Juni 2008
- Englisch
- ISBN-13: 9780470181195
- Artikelnr.: 37291566
- Verlag: John Wiley & Sons
- Seitenzahl: 616
- Erscheinungstermin: 28. Juni 2008
- Englisch
- ISBN-13: 9780470181195
- Artikelnr.: 37291566
- Herstellerkennzeichnung Die Herstellerinformationen sind derzeit nicht verfügbar.
1. Phase I Clinical Trials in Oncology (Anastasia Ivanova and Nancy
Flournoy).
1.1 Introduction.
1.2 Phase I Trials in Healthy Volunteers.
1.3 Phase I Trials With Toxic Outcomes Enrolling Patients.
1.4 Other Design Problems in Dose Finding.
1.5 Concluding Remarks.
References.
2. Phase II Clinical Trials (Nigel Stallard).
2.1 Introduction.
2.2 Frequentist methods in phase II clinical trials.
2.3 Bayesian methods in phase II clinical trials.
2.4 Decision theoretic methods in phase II clinical trials.
2.5 Clinical trials combining phases II and III.
2.6 Outstanding issues in phase II clinical trials.
References.
3. Response Adaptive Designs in Phase III Clinical Trials (Atanu Biswas,
Uttam Bandyopadhyay and Rahul Bhattacharya).
3.1 Introduction
3.3 Adaptive Designs for Binary Treatment Responses Incorporating
Covariates.
3.4 Adaptive Designs for Categorical Responses.
3.5 Adaptive Designs for Continuous Responses.
3.6 Optimal Adaptive Designs.
3.7 Delayed Responses in Adaptive Designs.
3.8 Biased Coin Designs.
3.9 Real Adaptive Clinical Trials.
3.10 Data Study for Different Adaptive Scheme.
3.11 Concluding Remarks.
References.
4. Inverse Sampling for Clinical Trials: A Brief Review of Theory and
Practice (Atanu Biswas and Uttam Bandyopadhyay).
4.1 Introduction.
4.2 Two-Sample Randomized Inverse Sampling for Clinical Trials.
4.3 An Example of Inverse Sampling: Boston ECMO.
4.4 Inverse Sampling in Adaptive Designs.
4.5 Concluding.
5. The Design and Analysis Aspects of Cluster Randomized Trials (Hrishikesh
Chakraborty).
5.1 Introduction: Cluster Randomized Trials.
5.2 Intra-Cluster Correlation Coefficient and Confidence Interval.
5.3 Sample Size Calculation for Cluster Randomized Trials.
5.4 Analysis of Cluster Randomized Trial Data.
5.5 Concluding Remarks.
References.
SECTION II. EPIDEMIOLOGY.
6. HIV Dynamics Modeling and Prediction of Clinical Outcomes in AIDS
Clinical Research (Yangxin Huang and Hulin Wu).
6.1 Introduction.
6.2 HIV Dynamic Model and Treatment Effects Models.
6.3 Statistical Methods for Predictions of Clinical Outcomes.
6.4 Simulation Study.
6.5 Clinical Data Analysis.
6.6 Concluding Remarks.
References.
7. Spatial Epidemiology (Lance A. Waller).
7.1 Space and Disease.
7.2 Basic Spatial Questions and Related Data.
7.3 Quantifying Pattern in Point Data.
7.4 Predicting Spatial Observations.
7.5 Concluding Remarks.
References.
8. Modeling Disease Dynamics: Cholera as a Case Study (Edward L. Ionides,
Carles Breto and Aaron A. King).
8.1 Introduction.
8.2 Data Analysis via Population Models.
8.3 Sequential Monte Carlo.
8.4 Modeling Cholera.
8.5 Concluding Remarks.
References.
9. Misclassification and Measurement Error Models in Epidemiological
Studies (Surupa Roy and Tathagata Banerjee).
9.1 Introduction.
9.2 A Few Examples.
9.3 Binary Regression Models with Two Types of Errors.
9.4 Bivariate Binary Regression Models with Two Types of Errors.
9.5 Models for Analyzing Mixed Misclassified Binary and Continuous
Responses.
9.6 Atom Bomb Data Analysis.
9.7 Concluding Remarks.
References.
SECTION III. SURVIVAL ANALYSIS.
10. Semiparametric Maximum Likelihood Inference in Survival Analysis
(Michael R. Kosorok).
10.1 Introduction
10.2 Examples of Survival Models.
10.3 Basic Estimation and Limit Theory.
10.4 The Bootstrap.
10.5 The Profile Sampler.
10.6 The Piggyback Bootstrap.
10.7 Other Approaches.
10.8 Concluding Remarks.
References.
11. An Overview of the Semi-Competing Risks Problem (Limin Peng, Hongyu
Jiang, Richard J. Chappell and Jason P. Fine).
11.1 Introduction.
11.2 Nonparametric Inferences.
11.3 Semiparmetric One-Sample Inference.
11.4 Semiparametric Regression Method.
11.5 Concluding Remarks.
References.
12. Tests for Time-Varying Covariate Effects within Aalen's Additive
Hazards Model (Thomas H. Scheike and Torben Martinussen).
12.1 Introduction.
12.2 Model Specification and Inferential Procedures.
12.3 Numerical Results.
12.4 Concluding Remarks.
12.5 Summary.
References.
13. Analysis of Outcomes Subject to Induced Dependent Censoring: A Marked
Point Process Perspective (Eugene Huang).
13.1 Introduction.
13.2 Induced Dependent Censoring and Associated Identifiability Issues.
13.3 Marked Point Process.
13.4 Modeling Strategy for Testing and Regression.
13.5 Concluding Remarks.
References.
14. Analysis of Dependence in Multivariate Failure-Time Data (Zoe Moodie
and Li Hsu).
14.1 Introduction.
14.2 Nonparametric Bivariate Survivor Function Estimation.
14.3 Non- and Semi-Parametric Estimation of Dependence Measures.
14.4 Concluding Remarks.
References.
15. Robust Estimation for Analyzing Recurrent Events Data in the Presence
of Terminal Events (Rajeshwari Sundaram).
15.1 Introduction.
15.2 Inference Procedures.
15.3 Large Sample Properties.
15.4 Numerical Results.
15.5 Concluding Remarks.
References.
16. Tree-Based Methods for Survival Data (Mousumi Banerjee and
Anne-Michelle Noone).
16.1 Introduction.
16.2 Review of CART.
16.3 Trees for Survival Data.
16.4 Simulations to Compare Different Splitting Methods.
16.5 Example: Breast Cancer Prognostic Study.
16.6 Random forest for Survival Data.
16.7 Concluding Remarks.
References.
17. Bayesian Estimation of the Hazard Function with Randomly Right-Censored
Data (Jean-Francois Angers and Brenda MacGibbon).
17.1 Introduction.
17.2 Bayesian Functional Model Using Monotone Wavelet Approximation.
17.3 Estimation of the Sub-Density F*.
17.4 Simulations.
17.5 Example.
17.6 Concluding Remarks.
References.
SECTION IV. GENOMICS AND PROTEOMICS.
18. The Effects of Inter-Gene Associations on Statistical Inferences From
Microarray Data (Kerby Shedden).
18.1 Introduction.
18.2 Inter-Gene Correlation.
18.3 Differential Expression.
18.4 Time Course Experiments.
18.5 Meta-Analysis.
18.6 Concluding Remarks.
References.
19. A Comparison of Methods for Meta-Analysis of Gene Expression Data
(Hyungwon Choi and Debashis Ghosh).
19.1 Introduction.
19.2 Background.
19.3 Example.
19.4 Cross Comparison of Gene Signatures.
19.5 Best Common Mean Difference Method.
19.6 Effect Size Method.
19.7 Probability of Expression (POE) Assimilation Method.
19.8 Comparison of Three Methods.
19.9 Conclusions.
References.
20. Statistical Methods for Identifying Differentially Expressed Genes in
Replicated Microarray Experiments: A Review (Lynn Kuo, Fang Yu and Yifang
Zhao).
20.1 Introduction.
20.2 Normalization.
20.3 Methods for Selecting Differentially Expressed Genes.
20.4 Simulation Study.
20.5 Concluding Remarks.
References.
21. Clustering of Microarray Data via Mixture Models (Geoffrey McLachlan,
Richard W. Bean and Angus Ng).
21.1 Introduction.
21.2 Clustering of Microarray Data.
21.3 Notation.
21.4 Clustering of Tissue Samples.
21.5 The EMMIX-GENE Clustering Procedure.
21.6 Clustering of gene profile.
21.7 EMMIX-WIRE.
21.8 ML Estimation via the EM Algorithm.
21.9 Model Selection.
21.10 Example: Clustering of Time-Course Data.
21.11 Concluding Remarks.
References.
22. Censored Data Regression in High-Dimension and Low-Sample-Size Settings
for Genomic Applications (Hongzhe Li).
22.1 Introduction.
22.2 Censored Data Regression Models.
22.3 Regularized Estimation for Censored Data Regression Models.
22.4 Survival Ensemble Methods.
22.5 Nonparametric Pathway-Based Regression Models.
22.6 Dimension-Reduction-Based Methods and Bayesian Variable Selection
Methods.
22.7 Criteria for Evaluating Different Procedures.
22.8 Application to a Real Data Set and Comparisons.
22.9 Discussion and Future Research Topics.
22.10 Concluding Remarks.
References.
23. Analysis of Case-Control Studies in Genetic Epidemiology (Nilanjan
Chatterjee).
23.1 Introduction.
23.2 Maximum Likelihood Analysis of Case-Control Data with Complete
Information.
23.3 Haplotype-Based Genetic Analysis with Missing Phase Information
23.4 Concluding Remarks.
References.
24. Assessing Network Structure in the Presence of Measurement Error
(Denise Scholtens, Raji Balasubramanian and Robert Gentleman).
24.1 Introduction
24.2 Graphs of Biological Data.
24.3 Statistics on Graphs.
24.4 Graph Theoretic Models.
24.5 Types of Measurement Error.
24.6 Exploratory Data Analysis.
24.7 Influence of Measurement Error on Graph Statistics.
24.8 Biological Implications.
24.9 Conclusions.
References.
25. Prediction of RNA Splicing Signals (Mark Segal).
25.1 Introduction.
25.2 Existing Approaches to Splice Site Identification.
25.3 Splice Site Recognition Contemporary Classifiers.
25.4 Results.
25.5 Concluding Remarks.
References.
26. Statistical Methods for Biomarker Discovery Using Mass Spectrometry
(Bradley M. Broom and Kim-Anh Do).
26.1 Introduction.
26.2 Biomarker Discovery.
26.3 Statistical Methods for Pre-Processing.
26.4 Statistical Methods for Multiple Testing, Classification and
Applications spectra.
26.5 Potential Statistical Developments.
26.6 Concluding Remarks.
References.
27. Genetic Mapping of Quantitative Traits: Model-Free Sib-Pair Linkage
Approaches (Saurabh Ghosh and Parthe P. Majumder).
27.1 Introduction.
27.2 The Basic QTL Framework for Sib-Pairs.
27.3 The Haseman-Elston Regression Framework.
27.4 Nonparametric Alternatives.
27.5 The Modified Nonparametric Regression.
27.6 Comparison with Linear Regression Methods.
27.7 Significance Levels and Empirical Power.
27.8 An Application to Real Data.
27.9 Concluding Remarks.
References.
SECTION V. MISCELLANEOUS TOPICS.
28. Robustness Issues in Biomedical Studies (Ayanendranath Basu).
28.1 Introduction: The Need for Robust Procedures.
28.2 Standard Tools for Robustness.
28.3 The Robustness Question in Biomedical Studies.
28.4 Robust Estimation in the Logistic Regression Model.
28.5 Robust Estimation for Censored Survival Data.
28.6 Adaptive Robust Methods in Clinical Trials.
28.7 Concluding Remarks.
References.
29. Recent Advances in the Analysis of Episodic Hormone Data (Timothy D.
Johnson and Yuedong Wang).
29.1 Introduction.
29.2 A General Biophysical Model.
29.3 Bayesian Deconvolution Model (BDM).
29.4 Nonlinear Mixed Effects Partial Splines Models.
29.5 Concluding Remarks.
References.
30. Models for Carcinogenesis (Anup Dewanji).
30.1 Introduction.
30.2 Statistical Models.
30.3 Multistage Models.
30.4 Two-Stage Clonal Expansion Model.
30.5 Physiologically Based Pharmacokinetic Models.
30.6 Statistical Methods.
30.7 Concluding Remarks.
References.
Author Index.
Subject Index.
1. Phase I Clinical Trials in Oncology (Anastasia Ivanova and Nancy
Flournoy).
1.1 Introduction.
1.2 Phase I Trials in Healthy Volunteers.
1.3 Phase I Trials With Toxic Outcomes Enrolling Patients.
1.4 Other Design Problems in Dose Finding.
1.5 Concluding Remarks.
References.
2. Phase II Clinical Trials (Nigel Stallard).
2.1 Introduction.
2.2 Frequentist methods in phase II clinical trials.
2.3 Bayesian methods in phase II clinical trials.
2.4 Decision theoretic methods in phase II clinical trials.
2.5 Clinical trials combining phases II and III.
2.6 Outstanding issues in phase II clinical trials.
References.
3. Response Adaptive Designs in Phase III Clinical Trials (Atanu Biswas,
Uttam Bandyopadhyay and Rahul Bhattacharya).
3.1 Introduction
3.3 Adaptive Designs for Binary Treatment Responses Incorporating
Covariates.
3.4 Adaptive Designs for Categorical Responses.
3.5 Adaptive Designs for Continuous Responses.
3.6 Optimal Adaptive Designs.
3.7 Delayed Responses in Adaptive Designs.
3.8 Biased Coin Designs.
3.9 Real Adaptive Clinical Trials.
3.10 Data Study for Different Adaptive Scheme.
3.11 Concluding Remarks.
References.
4. Inverse Sampling for Clinical Trials: A Brief Review of Theory and
Practice (Atanu Biswas and Uttam Bandyopadhyay).
4.1 Introduction.
4.2 Two-Sample Randomized Inverse Sampling for Clinical Trials.
4.3 An Example of Inverse Sampling: Boston ECMO.
4.4 Inverse Sampling in Adaptive Designs.
4.5 Concluding.
5. The Design and Analysis Aspects of Cluster Randomized Trials (Hrishikesh
Chakraborty).
5.1 Introduction: Cluster Randomized Trials.
5.2 Intra-Cluster Correlation Coefficient and Confidence Interval.
5.3 Sample Size Calculation for Cluster Randomized Trials.
5.4 Analysis of Cluster Randomized Trial Data.
5.5 Concluding Remarks.
References.
SECTION II. EPIDEMIOLOGY.
6. HIV Dynamics Modeling and Prediction of Clinical Outcomes in AIDS
Clinical Research (Yangxin Huang and Hulin Wu).
6.1 Introduction.
6.2 HIV Dynamic Model and Treatment Effects Models.
6.3 Statistical Methods for Predictions of Clinical Outcomes.
6.4 Simulation Study.
6.5 Clinical Data Analysis.
6.6 Concluding Remarks.
References.
7. Spatial Epidemiology (Lance A. Waller).
7.1 Space and Disease.
7.2 Basic Spatial Questions and Related Data.
7.3 Quantifying Pattern in Point Data.
7.4 Predicting Spatial Observations.
7.5 Concluding Remarks.
References.
8. Modeling Disease Dynamics: Cholera as a Case Study (Edward L. Ionides,
Carles Breto and Aaron A. King).
8.1 Introduction.
8.2 Data Analysis via Population Models.
8.3 Sequential Monte Carlo.
8.4 Modeling Cholera.
8.5 Concluding Remarks.
References.
9. Misclassification and Measurement Error Models in Epidemiological
Studies (Surupa Roy and Tathagata Banerjee).
9.1 Introduction.
9.2 A Few Examples.
9.3 Binary Regression Models with Two Types of Errors.
9.4 Bivariate Binary Regression Models with Two Types of Errors.
9.5 Models for Analyzing Mixed Misclassified Binary and Continuous
Responses.
9.6 Atom Bomb Data Analysis.
9.7 Concluding Remarks.
References.
SECTION III. SURVIVAL ANALYSIS.
10. Semiparametric Maximum Likelihood Inference in Survival Analysis
(Michael R. Kosorok).
10.1 Introduction
10.2 Examples of Survival Models.
10.3 Basic Estimation and Limit Theory.
10.4 The Bootstrap.
10.5 The Profile Sampler.
10.6 The Piggyback Bootstrap.
10.7 Other Approaches.
10.8 Concluding Remarks.
References.
11. An Overview of the Semi-Competing Risks Problem (Limin Peng, Hongyu
Jiang, Richard J. Chappell and Jason P. Fine).
11.1 Introduction.
11.2 Nonparametric Inferences.
11.3 Semiparmetric One-Sample Inference.
11.4 Semiparametric Regression Method.
11.5 Concluding Remarks.
References.
12. Tests for Time-Varying Covariate Effects within Aalen's Additive
Hazards Model (Thomas H. Scheike and Torben Martinussen).
12.1 Introduction.
12.2 Model Specification and Inferential Procedures.
12.3 Numerical Results.
12.4 Concluding Remarks.
12.5 Summary.
References.
13. Analysis of Outcomes Subject to Induced Dependent Censoring: A Marked
Point Process Perspective (Eugene Huang).
13.1 Introduction.
13.2 Induced Dependent Censoring and Associated Identifiability Issues.
13.3 Marked Point Process.
13.4 Modeling Strategy for Testing and Regression.
13.5 Concluding Remarks.
References.
14. Analysis of Dependence in Multivariate Failure-Time Data (Zoe Moodie
and Li Hsu).
14.1 Introduction.
14.2 Nonparametric Bivariate Survivor Function Estimation.
14.3 Non- and Semi-Parametric Estimation of Dependence Measures.
14.4 Concluding Remarks.
References.
15. Robust Estimation for Analyzing Recurrent Events Data in the Presence
of Terminal Events (Rajeshwari Sundaram).
15.1 Introduction.
15.2 Inference Procedures.
15.3 Large Sample Properties.
15.4 Numerical Results.
15.5 Concluding Remarks.
References.
16. Tree-Based Methods for Survival Data (Mousumi Banerjee and
Anne-Michelle Noone).
16.1 Introduction.
16.2 Review of CART.
16.3 Trees for Survival Data.
16.4 Simulations to Compare Different Splitting Methods.
16.5 Example: Breast Cancer Prognostic Study.
16.6 Random forest for Survival Data.
16.7 Concluding Remarks.
References.
17. Bayesian Estimation of the Hazard Function with Randomly Right-Censored
Data (Jean-Francois Angers and Brenda MacGibbon).
17.1 Introduction.
17.2 Bayesian Functional Model Using Monotone Wavelet Approximation.
17.3 Estimation of the Sub-Density F*.
17.4 Simulations.
17.5 Example.
17.6 Concluding Remarks.
References.
SECTION IV. GENOMICS AND PROTEOMICS.
18. The Effects of Inter-Gene Associations on Statistical Inferences From
Microarray Data (Kerby Shedden).
18.1 Introduction.
18.2 Inter-Gene Correlation.
18.3 Differential Expression.
18.4 Time Course Experiments.
18.5 Meta-Analysis.
18.6 Concluding Remarks.
References.
19. A Comparison of Methods for Meta-Analysis of Gene Expression Data
(Hyungwon Choi and Debashis Ghosh).
19.1 Introduction.
19.2 Background.
19.3 Example.
19.4 Cross Comparison of Gene Signatures.
19.5 Best Common Mean Difference Method.
19.6 Effect Size Method.
19.7 Probability of Expression (POE) Assimilation Method.
19.8 Comparison of Three Methods.
19.9 Conclusions.
References.
20. Statistical Methods for Identifying Differentially Expressed Genes in
Replicated Microarray Experiments: A Review (Lynn Kuo, Fang Yu and Yifang
Zhao).
20.1 Introduction.
20.2 Normalization.
20.3 Methods for Selecting Differentially Expressed Genes.
20.4 Simulation Study.
20.5 Concluding Remarks.
References.
21. Clustering of Microarray Data via Mixture Models (Geoffrey McLachlan,
Richard W. Bean and Angus Ng).
21.1 Introduction.
21.2 Clustering of Microarray Data.
21.3 Notation.
21.4 Clustering of Tissue Samples.
21.5 The EMMIX-GENE Clustering Procedure.
21.6 Clustering of gene profile.
21.7 EMMIX-WIRE.
21.8 ML Estimation via the EM Algorithm.
21.9 Model Selection.
21.10 Example: Clustering of Time-Course Data.
21.11 Concluding Remarks.
References.
22. Censored Data Regression in High-Dimension and Low-Sample-Size Settings
for Genomic Applications (Hongzhe Li).
22.1 Introduction.
22.2 Censored Data Regression Models.
22.3 Regularized Estimation for Censored Data Regression Models.
22.4 Survival Ensemble Methods.
22.5 Nonparametric Pathway-Based Regression Models.
22.6 Dimension-Reduction-Based Methods and Bayesian Variable Selection
Methods.
22.7 Criteria for Evaluating Different Procedures.
22.8 Application to a Real Data Set and Comparisons.
22.9 Discussion and Future Research Topics.
22.10 Concluding Remarks.
References.
23. Analysis of Case-Control Studies in Genetic Epidemiology (Nilanjan
Chatterjee).
23.1 Introduction.
23.2 Maximum Likelihood Analysis of Case-Control Data with Complete
Information.
23.3 Haplotype-Based Genetic Analysis with Missing Phase Information
23.4 Concluding Remarks.
References.
24. Assessing Network Structure in the Presence of Measurement Error
(Denise Scholtens, Raji Balasubramanian and Robert Gentleman).
24.1 Introduction
24.2 Graphs of Biological Data.
24.3 Statistics on Graphs.
24.4 Graph Theoretic Models.
24.5 Types of Measurement Error.
24.6 Exploratory Data Analysis.
24.7 Influence of Measurement Error on Graph Statistics.
24.8 Biological Implications.
24.9 Conclusions.
References.
25. Prediction of RNA Splicing Signals (Mark Segal).
25.1 Introduction.
25.2 Existing Approaches to Splice Site Identification.
25.3 Splice Site Recognition Contemporary Classifiers.
25.4 Results.
25.5 Concluding Remarks.
References.
26. Statistical Methods for Biomarker Discovery Using Mass Spectrometry
(Bradley M. Broom and Kim-Anh Do).
26.1 Introduction.
26.2 Biomarker Discovery.
26.3 Statistical Methods for Pre-Processing.
26.4 Statistical Methods for Multiple Testing, Classification and
Applications spectra.
26.5 Potential Statistical Developments.
26.6 Concluding Remarks.
References.
27. Genetic Mapping of Quantitative Traits: Model-Free Sib-Pair Linkage
Approaches (Saurabh Ghosh and Parthe P. Majumder).
27.1 Introduction.
27.2 The Basic QTL Framework for Sib-Pairs.
27.3 The Haseman-Elston Regression Framework.
27.4 Nonparametric Alternatives.
27.5 The Modified Nonparametric Regression.
27.6 Comparison with Linear Regression Methods.
27.7 Significance Levels and Empirical Power.
27.8 An Application to Real Data.
27.9 Concluding Remarks.
References.
SECTION V. MISCELLANEOUS TOPICS.
28. Robustness Issues in Biomedical Studies (Ayanendranath Basu).
28.1 Introduction: The Need for Robust Procedures.
28.2 Standard Tools for Robustness.
28.3 The Robustness Question in Biomedical Studies.
28.4 Robust Estimation in the Logistic Regression Model.
28.5 Robust Estimation for Censored Survival Data.
28.6 Adaptive Robust Methods in Clinical Trials.
28.7 Concluding Remarks.
References.
29. Recent Advances in the Analysis of Episodic Hormone Data (Timothy D.
Johnson and Yuedong Wang).
29.1 Introduction.
29.2 A General Biophysical Model.
29.3 Bayesian Deconvolution Model (BDM).
29.4 Nonlinear Mixed Effects Partial Splines Models.
29.5 Concluding Remarks.
References.
30. Models for Carcinogenesis (Anup Dewanji).
30.1 Introduction.
30.2 Statistical Models.
30.3 Multistage Models.
30.4 Two-Stage Clonal Expansion Model.
30.5 Physiologically Based Pharmacokinetic Models.
30.6 Statistical Methods.
30.7 Concluding Remarks.
References.
Author Index.
Subject Index.
"The authors have done an excellent job of meeting the objective they put forward in the preface. They have produced an authoritative volume of readable chapters ... The chapters are written well and will be understandable to graduate students in biostatistics and statistics. The book will have an important place as a reference book on the shelf of many professional biostatisticians working in a biomedical research environment. Additionally, it should be useful as a special topics text for graduate students in biostatistics and statistics graduate programs." ( Biometrics, Dec 2008)