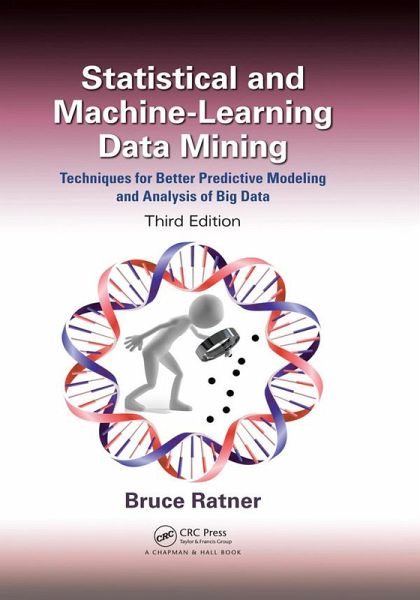
Statistical and Machine-Learning Data Mining: (eBook, ePUB)
Techniques for Better Predictive Modeling and Analysis of Big Data, Third Edition
Versandkostenfrei!
Sofort per Download lieferbar
48,95 €
inkl. MwSt.
Weitere Ausgaben:
PAYBACK Punkte
24 °P sammeln!
The third edition of a bestseller, Statistical and Machine-Learning Data Mining: Techniques for Better Predictive Modeling and Analysis of Big Data is still the only book, to date, to distinguish between statistical data mining and machine-learning data mining.
Dieser Download kann aus rechtlichen Gründen nur mit Rechnungsadresse in A, B, BG, CY, CZ, D, DK, EW, E, FIN, F, GR, HR, H, IRL, I, LT, L, LR, M, NL, PL, P, R, S, SLO, SK ausgeliefert werden.