William Q. Meeker, Gerald J. Hahn, Luis A. Escobar
Statistical Intervals (eBook, ePUB)
A Guide for Practitioners and Researchers
98,99 €
98,99 €
inkl. MwSt.
Sofort per Download lieferbar
0 °P sammeln
98,99 €
Als Download kaufen
98,99 €
inkl. MwSt.
Sofort per Download lieferbar
0 °P sammeln
Jetzt verschenken
Alle Infos zum eBook verschenken
98,99 €
inkl. MwSt.
Sofort per Download lieferbar
Alle Infos zum eBook verschenken
0 °P sammeln
William Q. Meeker, Gerald J. Hahn, Luis A. Escobar
Statistical Intervals (eBook, ePUB)
A Guide for Practitioners and Researchers
- Format: ePub
- Merkliste
- Auf die Merkliste
- Bewerten Bewerten
- Teilen
- Produkt teilen
- Produkterinnerung
- Produkterinnerung
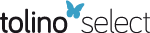
Bitte loggen Sie sich zunächst in Ihr Kundenkonto ein oder registrieren Sie sich bei
bücher.de, um das eBook-Abo tolino select nutzen zu können.
Hier können Sie sich einloggen
Hier können Sie sich einloggen
Sie sind bereits eingeloggt. Klicken Sie auf 2. tolino select Abo, um fortzufahren.
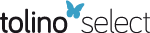
Bitte loggen Sie sich zunächst in Ihr Kundenkonto ein oder registrieren Sie sich bei bücher.de, um das eBook-Abo tolino select nutzen zu können.
Describes statistical intervals to quantify sampling uncertainty,focusing on key application needs and recently developed methodology in an easy-to-apply format
Statistical intervals provide invaluable tools for quantifying sampling uncertainty. The widely hailed first edition, published in 1991, described the use and construction of the most important statistical intervals. Particular emphasis was given to intervals-such as prediction intervals, tolerance intervals and confidence intervals on distribution quantiles-frequently needed in practice, but often neglected in introductory…mehr
- Geräte: eReader
- ohne Kopierschutz
- eBook Hilfe
Andere Kunden interessierten sich auch für
- Esa UusipaikkaConfidence Intervals in Generalized Regression Models (eBook, ePUB)65,95 €
- Nathalie PeyrardStatistical Approaches for Hidden Variables in Ecology (eBook, ePUB)139,99 €
- B. L. S. Prakasa RaoStatistical Inference for Fractional Diffusion Processes (eBook, ePUB)92,99 €
- Gerald Van BelleStatistical Rules of Thumb (eBook, ePUB)79,99 €
- Ian L. DrydenStatistical Shape Analysis (eBook, ePUB)77,99 €
- Dmitri KorolioukDynamics of Statistical Experiments (eBook, ePUB)139,99 €
- Anco HundepoolStatistical Disclosure Control (eBook, ePUB)79,99 €
-
-
-
Describes statistical intervals to quantify sampling uncertainty,focusing on key application needs and recently developed methodology in an easy-to-apply format
Statistical intervals provide invaluable tools for quantifying sampling uncertainty. The widely hailed first edition, published in 1991, described the use and construction of the most important statistical intervals. Particular emphasis was given to intervals-such as prediction intervals, tolerance intervals and confidence intervals on distribution quantiles-frequently needed in practice, but often neglected in introductory courses.
Vastly improved computer capabilities over the past 25 years have resulted in an explosion of the tools readily available to analysts. This second edition-more than double the size of the first-adds these new methods in an easy-to-apply format. In addition to extensive updating of the original chapters, the second edition includes new chapters on:
New technical appendices provide justification of the methods and pathways to extensions and further applications. A webpage directs readers to current readily accessible computer software and other useful information.
Statistical Intervals: A Guide for Practitioners and Researchers, Second Edition is an up-to-date working guide and reference for all who analyze data, allowing them to quantify the uncertainty in their results using statistical intervals.
Statistical intervals provide invaluable tools for quantifying sampling uncertainty. The widely hailed first edition, published in 1991, described the use and construction of the most important statistical intervals. Particular emphasis was given to intervals-such as prediction intervals, tolerance intervals and confidence intervals on distribution quantiles-frequently needed in practice, but often neglected in introductory courses.
Vastly improved computer capabilities over the past 25 years have resulted in an explosion of the tools readily available to analysts. This second edition-more than double the size of the first-adds these new methods in an easy-to-apply format. In addition to extensive updating of the original chapters, the second edition includes new chapters on:
- Likelihood-based statistical intervals
- Nonparametric bootstrap intervals
- Parametric bootstrap and other simulation-based intervals
- An introduction to Bayesian intervals
- Bayesian intervals for the popular binomial, Poisson and normal distributions
- Statistical intervals for Bayesian hierarchical models
- Advanced case studies, further illustrating the use of the newly described methods
New technical appendices provide justification of the methods and pathways to extensions and further applications. A webpage directs readers to current readily accessible computer software and other useful information.
Statistical Intervals: A Guide for Practitioners and Researchers, Second Edition is an up-to-date working guide and reference for all who analyze data, allowing them to quantify the uncertainty in their results using statistical intervals.
Dieser Download kann aus rechtlichen Gründen nur mit Rechnungsadresse in D ausgeliefert werden.
Produktdetails
- Produktdetails
- Verlag: John Wiley & Sons
- Erscheinungstermin: 22. August 2017
- Englisch
- ISBN-13: 9781118595169
- Artikelnr.: 52552893
- Verlag: John Wiley & Sons
- Erscheinungstermin: 22. August 2017
- Englisch
- ISBN-13: 9781118595169
- Artikelnr.: 52552893
- Herstellerkennzeichnung Die Herstellerinformationen sind derzeit nicht verfügbar.
William Q. Meeker is Professor of Statistics and Distinguished Professor of Liberal Arts and Sciences at Iowa State University. He is the co-author of Statistical Methods for Reliability Data, 2nd Edition (Wiley, 2021) and of numerous publications in the engineering and statistical literature and has won many awards for his research.
Gerald J. Hahn served for 46 years as applied statistician and manager of an 18-person statistics group supporting General Electric and has co-authored four books. His accomplishments have been recognized by GE's prestigious Coolidge Fellowship and 19 professional society awards.
Luis A. Escobar is Professor of Statistics at Louisiana State University. He is the co-author of Statistical Methods for Reliability Data, 2nd Edition (Wiley, 2021) and several book chapters. His publications have appeared in the engineering and statistical literature and he has won several research and teaching awards.
Gerald J. Hahn served for 46 years as applied statistician and manager of an 18-person statistics group supporting General Electric and has co-authored four books. His accomplishments have been recognized by GE's prestigious Coolidge Fellowship and 19 professional society awards.
Luis A. Escobar is Professor of Statistics at Louisiana State University. He is the co-author of Statistical Methods for Reliability Data, 2nd Edition (Wiley, 2021) and several book chapters. His publications have appeared in the engineering and statistical literature and he has won several research and teaching awards.
Preface to Second Edition iii
Preface to First Edition vii
Acknowledgments x
1 Introduction, Basic Concepts, and Assumptions 1
1.1 Statistical Inference 2
1.2 Different Types of Statistical Intervals: An Overview 2
1.3 The Assumption of Sample Data 3
1.4 The Central Role of Practical Assumptions Concerning Representative
Data 4
1.5 Enumerative Versus Analytic Studies 5
1.6 Basic Assumptions for Enumerative Studies 7
1.7 Considerations in the Conduct of Analytic Studies 10
1.8 Convenience and Judgment Samples 11
1.9 Sampling People 12
1.10 Infinite Population Assumptions 13
1.11 Practical Assumptions: Overview 14
1.12 Practical Assumptions: Further Example 14
1.13 Planning the Study 17
1.14 The Role of Statistical Distributions 17
1.15 The Interpretation of Statistical Intervals 18
1.16 Statistical Intervals and Big Data 19
1.17 Comment Concerning Subsequent Discussion 19
2 Overview of Different Types of Statistical Intervals 21
2.1 Choice of a Statistical Interval 21
2.2 Confidence Intervals 23
2.3 Prediction Intervals 24
2.4 Statistical Tolerance Intervals 26
2.5 Which Statistical Interval Do I Use? 27
2.6 Choosing a Confidence Level 28
2.7 Two-Sided Statistical Intervals Versus One-Sided Statistical Bounds 29
2.8 The Advantage of Using Confidence Intervals Instead of Significance
Tests 30
2.9 Simultaneous Statistical Intervals 31
3 Constructing Statistical Intervals Assuming a Normal Distribution Using
Simple Tabulations 33
3.1 Introduction 34
3.2 Circuit Pack Voltage Output Example 35
3.3 Two-Sided Statistical Intervals 36
3.4 One-Sided Statistical Bounds 38
4 Methods for Calculating Statistical Intervals for a Normal Distribution
43
4.1 Notation 44
4.2 Confidence Interval for the Mean of a Normal Distribution 45
4.3 Confidence Interval for the Standard Deviation of a Normal Distribution
45
4.4 Confidence Interval for a Normal Distribution Quantile 46
4.5 Confidence Interval for the Distribution Proportion Less (Greater) Than
a Specified Value 47
4.6 Statistical Tolerance Intervals 48
4.7 Prediction Interval to Contain a Single Future Observation or the Mean
of m Future Observations 50
4.8 Prediction Interval to Contain at least k of m Future Observations 51
4.9 Prediction Interval to Contain the Standard Deviation of m Future
Observations 52
4.10 The Assumption of a Normal Distribution 53
4.11 Assessing Distribution Normality and Dealing with Nonnormality 54
4.12 Data Transformations and Inferences from Transformed Data 57
4.13 Statistical Intervals for Linear Regression Analysis 60
4.14 Statistical Intervals for Comparing Populations and Processes 62
5 Distribution-Free Statistical Intervals 65
5.1 Introduction 66
5.2 Distribution-Free Confidence Intervals and One-Sided Confidence Bounds
for a Quantile 68
5.3 Distribution-Free Tolerance Intervals and Bounds to Contain a Specified
Proportion of a Distribution 78
5.4 Prediction Intervals to Contain a Specified Ordered Observation in a
Future Sample 81
5.5 Distribution-Free Prediction Intervals and Bounds to Contain at Least k
of m Future Observations 84
6 Statistical Intervals for a Binomial Distribution 89
6.1 Introduction to Binomial Distribution Statistical Intervals 90
6.2 Confidence Intervals for the Actual Proportion Nonconforming in the
Sampled Distribution 92
6.3 Confidence Interval for the Proportion of Nonconforming Units in a
Finite Population 102
6.4 Confidence Intervals for the Probability that the Number of
Nonconforming Units in a Sample is Less than or Equal to (or Greater than)
a Specified Number 104
6.5 Confidence Intervals for the Quantile of the Distribution of the Number
of Nonconforming Units 105
6.6 Tolerance Intervals and One-Sided Tolerance Bounds for the Distribution
of the Number of Nonconforming Units 107
6.7 Prediction Intervals for the Number Nonconforming in a Future Sample
108
7 Statistical Intervals for a Poisson Distribution 115
7.1 Introduction 116
7.2 Confidence Intervals for the Event-Occurrence Rate of a Poisson
Distribution 117
7.3 Confidence Intervals for the Probability that the Number of Events in a
Specified Amount of Exposure is Less than or Equal to (or Greater than) a
Specified Number 124
7.4 Confidence Intervals for the Quantile of the Distribution of the Number
of Events in a Specified Amount of Exposure 125
7.5 Tolerance Intervals and One-Sided Tolerance Bounds for the Distribution
of the Number of Events in a Specified Amount of Exposure 127
7.6 Prediction Intervals for the Number of Events in a Future Amount of
Exposure 128
8 Sample Size Requirements for Confidence Intervals on Distribution
Parameters 135
8.1 Basic Requirements for Sample Size Determination 136
8.2 Sample Size for a Confidence Interval for a Normal Distribution Mean
137
8.3 Sample Size to Estimate a Normal Distribution Standard Deviation 141
8.4 Sample Size to Estimate a Normal Distribution Quantile 143
8.5 Sample Size to Estimate a Binomial Proportion 143
8.6 Sample Size to Estimate a Poisson Occurrence Rate 144
9 Sample Size Requirements for Tolerance Intervals, Tolerance Bounds, and
Related Demonstration Tests 148
9.1 Sample Size for Normal Distribution Tolerance Intervals and One-Sided
Tolerance Bounds148
9.2 Sample Size to Pass a One-Sided Demonstration Test Based on Normally
Distributed Measurements 150
9.3 Minimum Sample Size For Distribution-Free Two-Sided Tolerance Intervals
and One-Sided Tolerance Bounds 152
9.4 Sample Size for Controlling the Precision of Two-Sided
Distribution-Free Tolerance In-tervals and One-Sided Distribution-Free
Tolerance Bounds 153
9.5 Sample Size to Demonstrate that a Binomial Proportion Exceeds (is
Exceeded by) a Specified Value 154
10 Sample Size Requirements for Prediction Intervals 164
10.1 Prediction Interval Width: The Basic Idea 164
10.2 Sample Size for a Normal Distribution Prediction Interval 165
10.3 Sample Size for Distribution-Free Prediction Intervals for k of m
Future Observations 170
11 Basic Case Studies 172
11.1 Demonstration that the Operating Temperature of Most Manufactured
Devices will not Exceed a Specified Value 173
11.2 Forecasting Future Demand for Spare Parts 177
11.3 Estimating the Probability of Passing an Environmental Emissions Test
180
11.4 Planning a Demonstration Test to Verify that a Radar System has a
Satisfactory Prob-ability of Detection 182
11.5 Estimating the Probability of Exceeding a Regulatory Limit 184
11.6 Estimating the Reliability of a Circuit Board 189
11.7 Using Sample Results to Estimate the Probability that a Demonstration
Test will be Successful 191
11.8 Estimating the Proportion within Specifications for a Two-Variable
Problem 194
11.9 Determining the Minimum Sample Size for a Demonstration Test 195
12 Likelihood-Based Statistical Intervals 197
12.1 Introduction to Likelihood-Based Inference 198
12.2 Likelihood Function and Maximum Likelihood Estimation 200
12.3 Likelihood-Based Confidence Intervals for Single-Parameter
Distributions 203
12.4 Likelihood-Based Estimation Methods for Location-Scale and
Log-Location-Scale Distri-butions 206
12.5 Likelihood-Based Confidence Intervals for Parameters and Scalar
Functions of Parameters212
12.6 Wald-Approximation Confidence Intervals 216
12.7 Some Other Likelihood-Based Statistical Intervals 224
13 Nonparametric Bootstrap Statistical Intervals 226
13.1 Introduction 227
13.2 Nonparametric Methods for Generating Bootstrap Samples and Obtaining
Bootstrap Estimates 227
13.3 Bootstrap Operational Considerations 231
13.4 Nonparametric Bootstrap Confidence Interval Methods 233
14 Parametric Bootstrap and Other Simulation-Based Statistical Intervals
245
14.1 Introduction 246
14.2 Parametric Bootstrap Samples and Bootstrap Estimates 247
14.3 Bootstrap Confidence Intervals Based on Pivotal Quantities 250
14.4 Generalized Pivotal Quantities 253
14.5 Simulation-Based Tolerance Intervals for Location-Scale or
Log-Location-Scale Distribu-tions 258
14.6 Simulation-Based Prediction Intervals and One-Sided Prediction Bounds
for k of m Fu-ture Observations from Location-Scale or Log-Location-Scale
Distributions 260
14.7 Other Simulation and Bootstrap Methods and Application to Other
Distributions and Models 263
15 Introduction to Bayesian Statistical Intervals 270
15.1 Bayesian Inference: Overview 271
15.2 Bayesian Inference: an Illustrative Example 274
15.3 More About Specification of a Prior Distribution 283
15.4 Implementing Bayesian Analyses Using Markov Chain Monte Carlo
Simulation 286
15.5 Bayesian Tolerance and Prediction Intervals 291
16 Bayesian Statistical Intervals for the Binomial, Poisson and Normal
Distributions 297
16.1 Bayesian Intervals for the Binomial Distribution 298
16.2 Bayesian Intervals for the Poisson Distribution 306
16.3 Bayesian Intervals for the Normal Distribution 311
17 Statistical Intervals for Bayesian Hierarchical Models 321
17.1 Bayesian Hierarchical Models and Random Effects 322
17.2 Normal Distribution Hierarchical Models 323
17.3 Binomial Distribution Hierarchical Models 325
17.4 Poisson Distribution Hierarchical Models 328
17.5 Longitudinal Repeated Measures Models 329
18 Advanced Case Studies 335
18.1 Confidence Interval for the Proportion of Defective Integrated
Circuits 336
18.2 Confidence Intervals for Components of Variance in a Measurement
Process 339
18.3 Tolerance Interval to Characterize the Distribution of Process Output
in the Presence of Measurement Error 344
18.4 Confidence Interval for the Proportion of Product Conforming to a
Two-Sided Specification345
18.5 Confidence Interval for the Treatment Effect in a Marketing Campaign
348
18.6 Confidence Interval for the Probability of Detection with Limited
Hit-Miss Data 349
18.7 Using Prior Information to Estimate the Service-Life Distribution of a
Rocket Motor 353
Epilogue 357
A Notation and Acronyms 360
B Generic Definition of Statistical Intervals and Formulas for Computing
Coverage Probabilities 367
B.1 Introduction 367
B.2 Two-sided Confidence Intervals and One-sided Confidence Bounds for
Distribution Pa-rameters or a Function of Parameters 368
B.3 Two Sided Control-the-Center Tolerance Intervals to Contain at Least a
Specified Pro-portion of a Distribution 371
B.4 Two Sided Tolerance Intervals to Control Both Tails of a Distribution
374
B.5 One-Sided Tolerance Bounds 377
B.6 Two-sided Prediction Intervals and One-Sided Prediction Bounds for
Future Observations378
B.7 Two-Sided Simultaneous Prediction Intervals and One-Sided Simultaneous
Prediction Bounds 381
B.8 Calibration of Statistical Intervals 383
C Useful Probability Distributions 384
C.1 Probability Distribution and R Computations 384
C.2 Important Characteristics of Random Variables 385
C.3 Continuous Distributions 388
C.4 Discrete Distributions 398
D General Results from Statistical Theory and Some Methods Used to
Construct Sta-tistical Intervals 404
D.1 cdfs and pdfs of Functions of Random Variables 405
D.2 Statistical Error Propagation-The Delta Method 409
D.3 Likelihood and Fisher Information Matrices 410
D.4 Convergence in Distribution 413
D.5 Outline of General ML Theory 415
D.6 The CDF pivotal method for constructing confidence intervals 419
D.7 Bonferroni approximate statistical intervals 424
E Pivotal Methods for Constructing Parametric Statistical Intervals 427
E.1 General definition and examples of pivotal quantities 428
E.2 Pivotal Quantities for the Normal Distribution 428
E.3 Confidence intervals for a Normal Distribution Based on Pivotal
Quantities 429
E.4 Confidence Intervals for Two Normal Distributions Based on Pivotal
Quantities 432
E.5 Tolerance Intervals for a Normal Distribution Based on Pivotal
Quantities 432
E.6 Normal Distribution Prediction Intervals Based on Pivotal Quantities
434
E.7 Pivotal Quantities for Log-Location-Scale Distributions 436
F Generalized Pivotal Quantities 440
F.1 Definition of Generalized Pivotal Quantities 440
F.2 A Substitution Method to Obtain GPQs 441
F.3 Examples of GPQs for Functions of Location-Scale Distribution
Parameters 441
F.4 Conditions for Exact Intervals Derived from GPQs 443
G Distribution-Free Intervals Based on Order Statistics 446
G.1 Basic Statistical Results Used in this Appendix 446
G.2 Distribution-Free Confidence Intervals and Bounds for a Distribution
Quantile 447
G.3 Distribution-Free Tolerance Intervals to Contain a Given Proportion of
a Distribution 448
G.4 Distribution-Free Prediction Interval to Contain a Specified Ordered
Observation From a Future Sample 449
G.5 Distribution-Free Prediction Intervals and Bounds to Contain at Least k
of m Future Observations From a Future Sample 451
H Basic Results from Bayesian Inference Models 455
H.1 Basic Statistical Results Used in this Appendix 455
H.2 Bayes' Theorem 456
H.3 Conjugate Prior Distributions 456
H.4 Jeffreys Prior Distributions 459
H.5 Posterior Predictive Distributions 463
H.6 Posterior Predictive Distributions Based on Jeffreys Prior
Distributions 465
I Probability of Successful Demonstration 468
I.1 Demonstration Tests Based on a Normal Distribution Assumption 468
I.2 Distribution-Free Demonstration Tests 469
J Tables 471
References 508
Subject Index 525
Preface to First Edition vii
Acknowledgments x
1 Introduction, Basic Concepts, and Assumptions 1
1.1 Statistical Inference 2
1.2 Different Types of Statistical Intervals: An Overview 2
1.3 The Assumption of Sample Data 3
1.4 The Central Role of Practical Assumptions Concerning Representative
Data 4
1.5 Enumerative Versus Analytic Studies 5
1.6 Basic Assumptions for Enumerative Studies 7
1.7 Considerations in the Conduct of Analytic Studies 10
1.8 Convenience and Judgment Samples 11
1.9 Sampling People 12
1.10 Infinite Population Assumptions 13
1.11 Practical Assumptions: Overview 14
1.12 Practical Assumptions: Further Example 14
1.13 Planning the Study 17
1.14 The Role of Statistical Distributions 17
1.15 The Interpretation of Statistical Intervals 18
1.16 Statistical Intervals and Big Data 19
1.17 Comment Concerning Subsequent Discussion 19
2 Overview of Different Types of Statistical Intervals 21
2.1 Choice of a Statistical Interval 21
2.2 Confidence Intervals 23
2.3 Prediction Intervals 24
2.4 Statistical Tolerance Intervals 26
2.5 Which Statistical Interval Do I Use? 27
2.6 Choosing a Confidence Level 28
2.7 Two-Sided Statistical Intervals Versus One-Sided Statistical Bounds 29
2.8 The Advantage of Using Confidence Intervals Instead of Significance
Tests 30
2.9 Simultaneous Statistical Intervals 31
3 Constructing Statistical Intervals Assuming a Normal Distribution Using
Simple Tabulations 33
3.1 Introduction 34
3.2 Circuit Pack Voltage Output Example 35
3.3 Two-Sided Statistical Intervals 36
3.4 One-Sided Statistical Bounds 38
4 Methods for Calculating Statistical Intervals for a Normal Distribution
43
4.1 Notation 44
4.2 Confidence Interval for the Mean of a Normal Distribution 45
4.3 Confidence Interval for the Standard Deviation of a Normal Distribution
45
4.4 Confidence Interval for a Normal Distribution Quantile 46
4.5 Confidence Interval for the Distribution Proportion Less (Greater) Than
a Specified Value 47
4.6 Statistical Tolerance Intervals 48
4.7 Prediction Interval to Contain a Single Future Observation or the Mean
of m Future Observations 50
4.8 Prediction Interval to Contain at least k of m Future Observations 51
4.9 Prediction Interval to Contain the Standard Deviation of m Future
Observations 52
4.10 The Assumption of a Normal Distribution 53
4.11 Assessing Distribution Normality and Dealing with Nonnormality 54
4.12 Data Transformations and Inferences from Transformed Data 57
4.13 Statistical Intervals for Linear Regression Analysis 60
4.14 Statistical Intervals for Comparing Populations and Processes 62
5 Distribution-Free Statistical Intervals 65
5.1 Introduction 66
5.2 Distribution-Free Confidence Intervals and One-Sided Confidence Bounds
for a Quantile 68
5.3 Distribution-Free Tolerance Intervals and Bounds to Contain a Specified
Proportion of a Distribution 78
5.4 Prediction Intervals to Contain a Specified Ordered Observation in a
Future Sample 81
5.5 Distribution-Free Prediction Intervals and Bounds to Contain at Least k
of m Future Observations 84
6 Statistical Intervals for a Binomial Distribution 89
6.1 Introduction to Binomial Distribution Statistical Intervals 90
6.2 Confidence Intervals for the Actual Proportion Nonconforming in the
Sampled Distribution 92
6.3 Confidence Interval for the Proportion of Nonconforming Units in a
Finite Population 102
6.4 Confidence Intervals for the Probability that the Number of
Nonconforming Units in a Sample is Less than or Equal to (or Greater than)
a Specified Number 104
6.5 Confidence Intervals for the Quantile of the Distribution of the Number
of Nonconforming Units 105
6.6 Tolerance Intervals and One-Sided Tolerance Bounds for the Distribution
of the Number of Nonconforming Units 107
6.7 Prediction Intervals for the Number Nonconforming in a Future Sample
108
7 Statistical Intervals for a Poisson Distribution 115
7.1 Introduction 116
7.2 Confidence Intervals for the Event-Occurrence Rate of a Poisson
Distribution 117
7.3 Confidence Intervals for the Probability that the Number of Events in a
Specified Amount of Exposure is Less than or Equal to (or Greater than) a
Specified Number 124
7.4 Confidence Intervals for the Quantile of the Distribution of the Number
of Events in a Specified Amount of Exposure 125
7.5 Tolerance Intervals and One-Sided Tolerance Bounds for the Distribution
of the Number of Events in a Specified Amount of Exposure 127
7.6 Prediction Intervals for the Number of Events in a Future Amount of
Exposure 128
8 Sample Size Requirements for Confidence Intervals on Distribution
Parameters 135
8.1 Basic Requirements for Sample Size Determination 136
8.2 Sample Size for a Confidence Interval for a Normal Distribution Mean
137
8.3 Sample Size to Estimate a Normal Distribution Standard Deviation 141
8.4 Sample Size to Estimate a Normal Distribution Quantile 143
8.5 Sample Size to Estimate a Binomial Proportion 143
8.6 Sample Size to Estimate a Poisson Occurrence Rate 144
9 Sample Size Requirements for Tolerance Intervals, Tolerance Bounds, and
Related Demonstration Tests 148
9.1 Sample Size for Normal Distribution Tolerance Intervals and One-Sided
Tolerance Bounds148
9.2 Sample Size to Pass a One-Sided Demonstration Test Based on Normally
Distributed Measurements 150
9.3 Minimum Sample Size For Distribution-Free Two-Sided Tolerance Intervals
and One-Sided Tolerance Bounds 152
9.4 Sample Size for Controlling the Precision of Two-Sided
Distribution-Free Tolerance In-tervals and One-Sided Distribution-Free
Tolerance Bounds 153
9.5 Sample Size to Demonstrate that a Binomial Proportion Exceeds (is
Exceeded by) a Specified Value 154
10 Sample Size Requirements for Prediction Intervals 164
10.1 Prediction Interval Width: The Basic Idea 164
10.2 Sample Size for a Normal Distribution Prediction Interval 165
10.3 Sample Size for Distribution-Free Prediction Intervals for k of m
Future Observations 170
11 Basic Case Studies 172
11.1 Demonstration that the Operating Temperature of Most Manufactured
Devices will not Exceed a Specified Value 173
11.2 Forecasting Future Demand for Spare Parts 177
11.3 Estimating the Probability of Passing an Environmental Emissions Test
180
11.4 Planning a Demonstration Test to Verify that a Radar System has a
Satisfactory Prob-ability of Detection 182
11.5 Estimating the Probability of Exceeding a Regulatory Limit 184
11.6 Estimating the Reliability of a Circuit Board 189
11.7 Using Sample Results to Estimate the Probability that a Demonstration
Test will be Successful 191
11.8 Estimating the Proportion within Specifications for a Two-Variable
Problem 194
11.9 Determining the Minimum Sample Size for a Demonstration Test 195
12 Likelihood-Based Statistical Intervals 197
12.1 Introduction to Likelihood-Based Inference 198
12.2 Likelihood Function and Maximum Likelihood Estimation 200
12.3 Likelihood-Based Confidence Intervals for Single-Parameter
Distributions 203
12.4 Likelihood-Based Estimation Methods for Location-Scale and
Log-Location-Scale Distri-butions 206
12.5 Likelihood-Based Confidence Intervals for Parameters and Scalar
Functions of Parameters212
12.6 Wald-Approximation Confidence Intervals 216
12.7 Some Other Likelihood-Based Statistical Intervals 224
13 Nonparametric Bootstrap Statistical Intervals 226
13.1 Introduction 227
13.2 Nonparametric Methods for Generating Bootstrap Samples and Obtaining
Bootstrap Estimates 227
13.3 Bootstrap Operational Considerations 231
13.4 Nonparametric Bootstrap Confidence Interval Methods 233
14 Parametric Bootstrap and Other Simulation-Based Statistical Intervals
245
14.1 Introduction 246
14.2 Parametric Bootstrap Samples and Bootstrap Estimates 247
14.3 Bootstrap Confidence Intervals Based on Pivotal Quantities 250
14.4 Generalized Pivotal Quantities 253
14.5 Simulation-Based Tolerance Intervals for Location-Scale or
Log-Location-Scale Distribu-tions 258
14.6 Simulation-Based Prediction Intervals and One-Sided Prediction Bounds
for k of m Fu-ture Observations from Location-Scale or Log-Location-Scale
Distributions 260
14.7 Other Simulation and Bootstrap Methods and Application to Other
Distributions and Models 263
15 Introduction to Bayesian Statistical Intervals 270
15.1 Bayesian Inference: Overview 271
15.2 Bayesian Inference: an Illustrative Example 274
15.3 More About Specification of a Prior Distribution 283
15.4 Implementing Bayesian Analyses Using Markov Chain Monte Carlo
Simulation 286
15.5 Bayesian Tolerance and Prediction Intervals 291
16 Bayesian Statistical Intervals for the Binomial, Poisson and Normal
Distributions 297
16.1 Bayesian Intervals for the Binomial Distribution 298
16.2 Bayesian Intervals for the Poisson Distribution 306
16.3 Bayesian Intervals for the Normal Distribution 311
17 Statistical Intervals for Bayesian Hierarchical Models 321
17.1 Bayesian Hierarchical Models and Random Effects 322
17.2 Normal Distribution Hierarchical Models 323
17.3 Binomial Distribution Hierarchical Models 325
17.4 Poisson Distribution Hierarchical Models 328
17.5 Longitudinal Repeated Measures Models 329
18 Advanced Case Studies 335
18.1 Confidence Interval for the Proportion of Defective Integrated
Circuits 336
18.2 Confidence Intervals for Components of Variance in a Measurement
Process 339
18.3 Tolerance Interval to Characterize the Distribution of Process Output
in the Presence of Measurement Error 344
18.4 Confidence Interval for the Proportion of Product Conforming to a
Two-Sided Specification345
18.5 Confidence Interval for the Treatment Effect in a Marketing Campaign
348
18.6 Confidence Interval for the Probability of Detection with Limited
Hit-Miss Data 349
18.7 Using Prior Information to Estimate the Service-Life Distribution of a
Rocket Motor 353
Epilogue 357
A Notation and Acronyms 360
B Generic Definition of Statistical Intervals and Formulas for Computing
Coverage Probabilities 367
B.1 Introduction 367
B.2 Two-sided Confidence Intervals and One-sided Confidence Bounds for
Distribution Pa-rameters or a Function of Parameters 368
B.3 Two Sided Control-the-Center Tolerance Intervals to Contain at Least a
Specified Pro-portion of a Distribution 371
B.4 Two Sided Tolerance Intervals to Control Both Tails of a Distribution
374
B.5 One-Sided Tolerance Bounds 377
B.6 Two-sided Prediction Intervals and One-Sided Prediction Bounds for
Future Observations378
B.7 Two-Sided Simultaneous Prediction Intervals and One-Sided Simultaneous
Prediction Bounds 381
B.8 Calibration of Statistical Intervals 383
C Useful Probability Distributions 384
C.1 Probability Distribution and R Computations 384
C.2 Important Characteristics of Random Variables 385
C.3 Continuous Distributions 388
C.4 Discrete Distributions 398
D General Results from Statistical Theory and Some Methods Used to
Construct Sta-tistical Intervals 404
D.1 cdfs and pdfs of Functions of Random Variables 405
D.2 Statistical Error Propagation-The Delta Method 409
D.3 Likelihood and Fisher Information Matrices 410
D.4 Convergence in Distribution 413
D.5 Outline of General ML Theory 415
D.6 The CDF pivotal method for constructing confidence intervals 419
D.7 Bonferroni approximate statistical intervals 424
E Pivotal Methods for Constructing Parametric Statistical Intervals 427
E.1 General definition and examples of pivotal quantities 428
E.2 Pivotal Quantities for the Normal Distribution 428
E.3 Confidence intervals for a Normal Distribution Based on Pivotal
Quantities 429
E.4 Confidence Intervals for Two Normal Distributions Based on Pivotal
Quantities 432
E.5 Tolerance Intervals for a Normal Distribution Based on Pivotal
Quantities 432
E.6 Normal Distribution Prediction Intervals Based on Pivotal Quantities
434
E.7 Pivotal Quantities for Log-Location-Scale Distributions 436
F Generalized Pivotal Quantities 440
F.1 Definition of Generalized Pivotal Quantities 440
F.2 A Substitution Method to Obtain GPQs 441
F.3 Examples of GPQs for Functions of Location-Scale Distribution
Parameters 441
F.4 Conditions for Exact Intervals Derived from GPQs 443
G Distribution-Free Intervals Based on Order Statistics 446
G.1 Basic Statistical Results Used in this Appendix 446
G.2 Distribution-Free Confidence Intervals and Bounds for a Distribution
Quantile 447
G.3 Distribution-Free Tolerance Intervals to Contain a Given Proportion of
a Distribution 448
G.4 Distribution-Free Prediction Interval to Contain a Specified Ordered
Observation From a Future Sample 449
G.5 Distribution-Free Prediction Intervals and Bounds to Contain at Least k
of m Future Observations From a Future Sample 451
H Basic Results from Bayesian Inference Models 455
H.1 Basic Statistical Results Used in this Appendix 455
H.2 Bayes' Theorem 456
H.3 Conjugate Prior Distributions 456
H.4 Jeffreys Prior Distributions 459
H.5 Posterior Predictive Distributions 463
H.6 Posterior Predictive Distributions Based on Jeffreys Prior
Distributions 465
I Probability of Successful Demonstration 468
I.1 Demonstration Tests Based on a Normal Distribution Assumption 468
I.2 Distribution-Free Demonstration Tests 469
J Tables 471
References 508
Subject Index 525
Preface to Second Edition iii
Preface to First Edition vii
Acknowledgments x
1 Introduction, Basic Concepts, and Assumptions 1
1.1 Statistical Inference 2
1.2 Different Types of Statistical Intervals: An Overview 2
1.3 The Assumption of Sample Data 3
1.4 The Central Role of Practical Assumptions Concerning Representative
Data 4
1.5 Enumerative Versus Analytic Studies 5
1.6 Basic Assumptions for Enumerative Studies 7
1.7 Considerations in the Conduct of Analytic Studies 10
1.8 Convenience and Judgment Samples 11
1.9 Sampling People 12
1.10 Infinite Population Assumptions 13
1.11 Practical Assumptions: Overview 14
1.12 Practical Assumptions: Further Example 14
1.13 Planning the Study 17
1.14 The Role of Statistical Distributions 17
1.15 The Interpretation of Statistical Intervals 18
1.16 Statistical Intervals and Big Data 19
1.17 Comment Concerning Subsequent Discussion 19
2 Overview of Different Types of Statistical Intervals 21
2.1 Choice of a Statistical Interval 21
2.2 Confidence Intervals 23
2.3 Prediction Intervals 24
2.4 Statistical Tolerance Intervals 26
2.5 Which Statistical Interval Do I Use? 27
2.6 Choosing a Confidence Level 28
2.7 Two-Sided Statistical Intervals Versus One-Sided Statistical Bounds 29
2.8 The Advantage of Using Confidence Intervals Instead of Significance
Tests 30
2.9 Simultaneous Statistical Intervals 31
3 Constructing Statistical Intervals Assuming a Normal Distribution Using
Simple Tabulations 33
3.1 Introduction 34
3.2 Circuit Pack Voltage Output Example 35
3.3 Two-Sided Statistical Intervals 36
3.4 One-Sided Statistical Bounds 38
4 Methods for Calculating Statistical Intervals for a Normal Distribution
43
4.1 Notation 44
4.2 Confidence Interval for the Mean of a Normal Distribution 45
4.3 Confidence Interval for the Standard Deviation of a Normal Distribution
45
4.4 Confidence Interval for a Normal Distribution Quantile 46
4.5 Confidence Interval for the Distribution Proportion Less (Greater) Than
a Specified Value 47
4.6 Statistical Tolerance Intervals 48
4.7 Prediction Interval to Contain a Single Future Observation or the Mean
of m Future Observations 50
4.8 Prediction Interval to Contain at least k of m Future Observations 51
4.9 Prediction Interval to Contain the Standard Deviation of m Future
Observations 52
4.10 The Assumption of a Normal Distribution 53
4.11 Assessing Distribution Normality and Dealing with Nonnormality 54
4.12 Data Transformations and Inferences from Transformed Data 57
4.13 Statistical Intervals for Linear Regression Analysis 60
4.14 Statistical Intervals for Comparing Populations and Processes 62
5 Distribution-Free Statistical Intervals 65
5.1 Introduction 66
5.2 Distribution-Free Confidence Intervals and One-Sided Confidence Bounds
for a Quantile 68
5.3 Distribution-Free Tolerance Intervals and Bounds to Contain a Specified
Proportion of a Distribution 78
5.4 Prediction Intervals to Contain a Specified Ordered Observation in a
Future Sample 81
5.5 Distribution-Free Prediction Intervals and Bounds to Contain at Least k
of m Future Observations 84
6 Statistical Intervals for a Binomial Distribution 89
6.1 Introduction to Binomial Distribution Statistical Intervals 90
6.2 Confidence Intervals for the Actual Proportion Nonconforming in the
Sampled Distribution 92
6.3 Confidence Interval for the Proportion of Nonconforming Units in a
Finite Population 102
6.4 Confidence Intervals for the Probability that the Number of
Nonconforming Units in a Sample is Less than or Equal to (or Greater than)
a Specified Number 104
6.5 Confidence Intervals for the Quantile of the Distribution of the Number
of Nonconforming Units 105
6.6 Tolerance Intervals and One-Sided Tolerance Bounds for the Distribution
of the Number of Nonconforming Units 107
6.7 Prediction Intervals for the Number Nonconforming in a Future Sample
108
7 Statistical Intervals for a Poisson Distribution 115
7.1 Introduction 116
7.2 Confidence Intervals for the Event-Occurrence Rate of a Poisson
Distribution 117
7.3 Confidence Intervals for the Probability that the Number of Events in a
Specified Amount of Exposure is Less than or Equal to (or Greater than) a
Specified Number 124
7.4 Confidence Intervals for the Quantile of the Distribution of the Number
of Events in a Specified Amount of Exposure 125
7.5 Tolerance Intervals and One-Sided Tolerance Bounds for the Distribution
of the Number of Events in a Specified Amount of Exposure 127
7.6 Prediction Intervals for the Number of Events in a Future Amount of
Exposure 128
8 Sample Size Requirements for Confidence Intervals on Distribution
Parameters 135
8.1 Basic Requirements for Sample Size Determination 136
8.2 Sample Size for a Confidence Interval for a Normal Distribution Mean
137
8.3 Sample Size to Estimate a Normal Distribution Standard Deviation 141
8.4 Sample Size to Estimate a Normal Distribution Quantile 143
8.5 Sample Size to Estimate a Binomial Proportion 143
8.6 Sample Size to Estimate a Poisson Occurrence Rate 144
9 Sample Size Requirements for Tolerance Intervals, Tolerance Bounds, and
Related Demonstration Tests 148
9.1 Sample Size for Normal Distribution Tolerance Intervals and One-Sided
Tolerance Bounds148
9.2 Sample Size to Pass a One-Sided Demonstration Test Based on Normally
Distributed Measurements 150
9.3 Minimum Sample Size For Distribution-Free Two-Sided Tolerance Intervals
and One-Sided Tolerance Bounds 152
9.4 Sample Size for Controlling the Precision of Two-Sided
Distribution-Free Tolerance In-tervals and One-Sided Distribution-Free
Tolerance Bounds 153
9.5 Sample Size to Demonstrate that a Binomial Proportion Exceeds (is
Exceeded by) a Specified Value 154
10 Sample Size Requirements for Prediction Intervals 164
10.1 Prediction Interval Width: The Basic Idea 164
10.2 Sample Size for a Normal Distribution Prediction Interval 165
10.3 Sample Size for Distribution-Free Prediction Intervals for k of m
Future Observations 170
11 Basic Case Studies 172
11.1 Demonstration that the Operating Temperature of Most Manufactured
Devices will not Exceed a Specified Value 173
11.2 Forecasting Future Demand for Spare Parts 177
11.3 Estimating the Probability of Passing an Environmental Emissions Test
180
11.4 Planning a Demonstration Test to Verify that a Radar System has a
Satisfactory Prob-ability of Detection 182
11.5 Estimating the Probability of Exceeding a Regulatory Limit 184
11.6 Estimating the Reliability of a Circuit Board 189
11.7 Using Sample Results to Estimate the Probability that a Demonstration
Test will be Successful 191
11.8 Estimating the Proportion within Specifications for a Two-Variable
Problem 194
11.9 Determining the Minimum Sample Size for a Demonstration Test 195
12 Likelihood-Based Statistical Intervals 197
12.1 Introduction to Likelihood-Based Inference 198
12.2 Likelihood Function and Maximum Likelihood Estimation 200
12.3 Likelihood-Based Confidence Intervals for Single-Parameter
Distributions 203
12.4 Likelihood-Based Estimation Methods for Location-Scale and
Log-Location-Scale Distri-butions 206
12.5 Likelihood-Based Confidence Intervals for Parameters and Scalar
Functions of Parameters212
12.6 Wald-Approximation Confidence Intervals 216
12.7 Some Other Likelihood-Based Statistical Intervals 224
13 Nonparametric Bootstrap Statistical Intervals 226
13.1 Introduction 227
13.2 Nonparametric Methods for Generating Bootstrap Samples and Obtaining
Bootstrap Estimates 227
13.3 Bootstrap Operational Considerations 231
13.4 Nonparametric Bootstrap Confidence Interval Methods 233
14 Parametric Bootstrap and Other Simulation-Based Statistical Intervals
245
14.1 Introduction 246
14.2 Parametric Bootstrap Samples and Bootstrap Estimates 247
14.3 Bootstrap Confidence Intervals Based on Pivotal Quantities 250
14.4 Generalized Pivotal Quantities 253
14.5 Simulation-Based Tolerance Intervals for Location-Scale or
Log-Location-Scale Distribu-tions 258
14.6 Simulation-Based Prediction Intervals and One-Sided Prediction Bounds
for k of m Fu-ture Observations from Location-Scale or Log-Location-Scale
Distributions 260
14.7 Other Simulation and Bootstrap Methods and Application to Other
Distributions and Models 263
15 Introduction to Bayesian Statistical Intervals 270
15.1 Bayesian Inference: Overview 271
15.2 Bayesian Inference: an Illustrative Example 274
15.3 More About Specification of a Prior Distribution 283
15.4 Implementing Bayesian Analyses Using Markov Chain Monte Carlo
Simulation 286
15.5 Bayesian Tolerance and Prediction Intervals 291
16 Bayesian Statistical Intervals for the Binomial, Poisson and Normal
Distributions 297
16.1 Bayesian Intervals for the Binomial Distribution 298
16.2 Bayesian Intervals for the Poisson Distribution 306
16.3 Bayesian Intervals for the Normal Distribution 311
17 Statistical Intervals for Bayesian Hierarchical Models 321
17.1 Bayesian Hierarchical Models and Random Effects 322
17.2 Normal Distribution Hierarchical Models 323
17.3 Binomial Distribution Hierarchical Models 325
17.4 Poisson Distribution Hierarchical Models 328
17.5 Longitudinal Repeated Measures Models 329
18 Advanced Case Studies 335
18.1 Confidence Interval for the Proportion of Defective Integrated
Circuits 336
18.2 Confidence Intervals for Components of Variance in a Measurement
Process 339
18.3 Tolerance Interval to Characterize the Distribution of Process Output
in the Presence of Measurement Error 344
18.4 Confidence Interval for the Proportion of Product Conforming to a
Two-Sided Specification345
18.5 Confidence Interval for the Treatment Effect in a Marketing Campaign
348
18.6 Confidence Interval for the Probability of Detection with Limited
Hit-Miss Data 349
18.7 Using Prior Information to Estimate the Service-Life Distribution of a
Rocket Motor 353
Epilogue 357
A Notation and Acronyms 360
B Generic Definition of Statistical Intervals and Formulas for Computing
Coverage Probabilities 367
B.1 Introduction 367
B.2 Two-sided Confidence Intervals and One-sided Confidence Bounds for
Distribution Pa-rameters or a Function of Parameters 368
B.3 Two Sided Control-the-Center Tolerance Intervals to Contain at Least a
Specified Pro-portion of a Distribution 371
B.4 Two Sided Tolerance Intervals to Control Both Tails of a Distribution
374
B.5 One-Sided Tolerance Bounds 377
B.6 Two-sided Prediction Intervals and One-Sided Prediction Bounds for
Future Observations378
B.7 Two-Sided Simultaneous Prediction Intervals and One-Sided Simultaneous
Prediction Bounds 381
B.8 Calibration of Statistical Intervals 383
C Useful Probability Distributions 384
C.1 Probability Distribution and R Computations 384
C.2 Important Characteristics of Random Variables 385
C.3 Continuous Distributions 388
C.4 Discrete Distributions 398
D General Results from Statistical Theory and Some Methods Used to
Construct Sta-tistical Intervals 404
D.1 cdfs and pdfs of Functions of Random Variables 405
D.2 Statistical Error Propagation-The Delta Method 409
D.3 Likelihood and Fisher Information Matrices 410
D.4 Convergence in Distribution 413
D.5 Outline of General ML Theory 415
D.6 The CDF pivotal method for constructing confidence intervals 419
D.7 Bonferroni approximate statistical intervals 424
E Pivotal Methods for Constructing Parametric Statistical Intervals 427
E.1 General definition and examples of pivotal quantities 428
E.2 Pivotal Quantities for the Normal Distribution 428
E.3 Confidence intervals for a Normal Distribution Based on Pivotal
Quantities 429
E.4 Confidence Intervals for Two Normal Distributions Based on Pivotal
Quantities 432
E.5 Tolerance Intervals for a Normal Distribution Based on Pivotal
Quantities 432
E.6 Normal Distribution Prediction Intervals Based on Pivotal Quantities
434
E.7 Pivotal Quantities for Log-Location-Scale Distributions 436
F Generalized Pivotal Quantities 440
F.1 Definition of Generalized Pivotal Quantities 440
F.2 A Substitution Method to Obtain GPQs 441
F.3 Examples of GPQs for Functions of Location-Scale Distribution
Parameters 441
F.4 Conditions for Exact Intervals Derived from GPQs 443
G Distribution-Free Intervals Based on Order Statistics 446
G.1 Basic Statistical Results Used in this Appendix 446
G.2 Distribution-Free Confidence Intervals and Bounds for a Distribution
Quantile 447
G.3 Distribution-Free Tolerance Intervals to Contain a Given Proportion of
a Distribution 448
G.4 Distribution-Free Prediction Interval to Contain a Specified Ordered
Observation From a Future Sample 449
G.5 Distribution-Free Prediction Intervals and Bounds to Contain at Least k
of m Future Observations From a Future Sample 451
H Basic Results from Bayesian Inference Models 455
H.1 Basic Statistical Results Used in this Appendix 455
H.2 Bayes' Theorem 456
H.3 Conjugate Prior Distributions 456
H.4 Jeffreys Prior Distributions 459
H.5 Posterior Predictive Distributions 463
H.6 Posterior Predictive Distributions Based on Jeffreys Prior
Distributions 465
I Probability of Successful Demonstration 468
I.1 Demonstration Tests Based on a Normal Distribution Assumption 468
I.2 Distribution-Free Demonstration Tests 469
J Tables 471
References 508
Subject Index 525
Preface to First Edition vii
Acknowledgments x
1 Introduction, Basic Concepts, and Assumptions 1
1.1 Statistical Inference 2
1.2 Different Types of Statistical Intervals: An Overview 2
1.3 The Assumption of Sample Data 3
1.4 The Central Role of Practical Assumptions Concerning Representative
Data 4
1.5 Enumerative Versus Analytic Studies 5
1.6 Basic Assumptions for Enumerative Studies 7
1.7 Considerations in the Conduct of Analytic Studies 10
1.8 Convenience and Judgment Samples 11
1.9 Sampling People 12
1.10 Infinite Population Assumptions 13
1.11 Practical Assumptions: Overview 14
1.12 Practical Assumptions: Further Example 14
1.13 Planning the Study 17
1.14 The Role of Statistical Distributions 17
1.15 The Interpretation of Statistical Intervals 18
1.16 Statistical Intervals and Big Data 19
1.17 Comment Concerning Subsequent Discussion 19
2 Overview of Different Types of Statistical Intervals 21
2.1 Choice of a Statistical Interval 21
2.2 Confidence Intervals 23
2.3 Prediction Intervals 24
2.4 Statistical Tolerance Intervals 26
2.5 Which Statistical Interval Do I Use? 27
2.6 Choosing a Confidence Level 28
2.7 Two-Sided Statistical Intervals Versus One-Sided Statistical Bounds 29
2.8 The Advantage of Using Confidence Intervals Instead of Significance
Tests 30
2.9 Simultaneous Statistical Intervals 31
3 Constructing Statistical Intervals Assuming a Normal Distribution Using
Simple Tabulations 33
3.1 Introduction 34
3.2 Circuit Pack Voltage Output Example 35
3.3 Two-Sided Statistical Intervals 36
3.4 One-Sided Statistical Bounds 38
4 Methods for Calculating Statistical Intervals for a Normal Distribution
43
4.1 Notation 44
4.2 Confidence Interval for the Mean of a Normal Distribution 45
4.3 Confidence Interval for the Standard Deviation of a Normal Distribution
45
4.4 Confidence Interval for a Normal Distribution Quantile 46
4.5 Confidence Interval for the Distribution Proportion Less (Greater) Than
a Specified Value 47
4.6 Statistical Tolerance Intervals 48
4.7 Prediction Interval to Contain a Single Future Observation or the Mean
of m Future Observations 50
4.8 Prediction Interval to Contain at least k of m Future Observations 51
4.9 Prediction Interval to Contain the Standard Deviation of m Future
Observations 52
4.10 The Assumption of a Normal Distribution 53
4.11 Assessing Distribution Normality and Dealing with Nonnormality 54
4.12 Data Transformations and Inferences from Transformed Data 57
4.13 Statistical Intervals for Linear Regression Analysis 60
4.14 Statistical Intervals for Comparing Populations and Processes 62
5 Distribution-Free Statistical Intervals 65
5.1 Introduction 66
5.2 Distribution-Free Confidence Intervals and One-Sided Confidence Bounds
for a Quantile 68
5.3 Distribution-Free Tolerance Intervals and Bounds to Contain a Specified
Proportion of a Distribution 78
5.4 Prediction Intervals to Contain a Specified Ordered Observation in a
Future Sample 81
5.5 Distribution-Free Prediction Intervals and Bounds to Contain at Least k
of m Future Observations 84
6 Statistical Intervals for a Binomial Distribution 89
6.1 Introduction to Binomial Distribution Statistical Intervals 90
6.2 Confidence Intervals for the Actual Proportion Nonconforming in the
Sampled Distribution 92
6.3 Confidence Interval for the Proportion of Nonconforming Units in a
Finite Population 102
6.4 Confidence Intervals for the Probability that the Number of
Nonconforming Units in a Sample is Less than or Equal to (or Greater than)
a Specified Number 104
6.5 Confidence Intervals for the Quantile of the Distribution of the Number
of Nonconforming Units 105
6.6 Tolerance Intervals and One-Sided Tolerance Bounds for the Distribution
of the Number of Nonconforming Units 107
6.7 Prediction Intervals for the Number Nonconforming in a Future Sample
108
7 Statistical Intervals for a Poisson Distribution 115
7.1 Introduction 116
7.2 Confidence Intervals for the Event-Occurrence Rate of a Poisson
Distribution 117
7.3 Confidence Intervals for the Probability that the Number of Events in a
Specified Amount of Exposure is Less than or Equal to (or Greater than) a
Specified Number 124
7.4 Confidence Intervals for the Quantile of the Distribution of the Number
of Events in a Specified Amount of Exposure 125
7.5 Tolerance Intervals and One-Sided Tolerance Bounds for the Distribution
of the Number of Events in a Specified Amount of Exposure 127
7.6 Prediction Intervals for the Number of Events in a Future Amount of
Exposure 128
8 Sample Size Requirements for Confidence Intervals on Distribution
Parameters 135
8.1 Basic Requirements for Sample Size Determination 136
8.2 Sample Size for a Confidence Interval for a Normal Distribution Mean
137
8.3 Sample Size to Estimate a Normal Distribution Standard Deviation 141
8.4 Sample Size to Estimate a Normal Distribution Quantile 143
8.5 Sample Size to Estimate a Binomial Proportion 143
8.6 Sample Size to Estimate a Poisson Occurrence Rate 144
9 Sample Size Requirements for Tolerance Intervals, Tolerance Bounds, and
Related Demonstration Tests 148
9.1 Sample Size for Normal Distribution Tolerance Intervals and One-Sided
Tolerance Bounds148
9.2 Sample Size to Pass a One-Sided Demonstration Test Based on Normally
Distributed Measurements 150
9.3 Minimum Sample Size For Distribution-Free Two-Sided Tolerance Intervals
and One-Sided Tolerance Bounds 152
9.4 Sample Size for Controlling the Precision of Two-Sided
Distribution-Free Tolerance In-tervals and One-Sided Distribution-Free
Tolerance Bounds 153
9.5 Sample Size to Demonstrate that a Binomial Proportion Exceeds (is
Exceeded by) a Specified Value 154
10 Sample Size Requirements for Prediction Intervals 164
10.1 Prediction Interval Width: The Basic Idea 164
10.2 Sample Size for a Normal Distribution Prediction Interval 165
10.3 Sample Size for Distribution-Free Prediction Intervals for k of m
Future Observations 170
11 Basic Case Studies 172
11.1 Demonstration that the Operating Temperature of Most Manufactured
Devices will not Exceed a Specified Value 173
11.2 Forecasting Future Demand for Spare Parts 177
11.3 Estimating the Probability of Passing an Environmental Emissions Test
180
11.4 Planning a Demonstration Test to Verify that a Radar System has a
Satisfactory Prob-ability of Detection 182
11.5 Estimating the Probability of Exceeding a Regulatory Limit 184
11.6 Estimating the Reliability of a Circuit Board 189
11.7 Using Sample Results to Estimate the Probability that a Demonstration
Test will be Successful 191
11.8 Estimating the Proportion within Specifications for a Two-Variable
Problem 194
11.9 Determining the Minimum Sample Size for a Demonstration Test 195
12 Likelihood-Based Statistical Intervals 197
12.1 Introduction to Likelihood-Based Inference 198
12.2 Likelihood Function and Maximum Likelihood Estimation 200
12.3 Likelihood-Based Confidence Intervals for Single-Parameter
Distributions 203
12.4 Likelihood-Based Estimation Methods for Location-Scale and
Log-Location-Scale Distri-butions 206
12.5 Likelihood-Based Confidence Intervals for Parameters and Scalar
Functions of Parameters212
12.6 Wald-Approximation Confidence Intervals 216
12.7 Some Other Likelihood-Based Statistical Intervals 224
13 Nonparametric Bootstrap Statistical Intervals 226
13.1 Introduction 227
13.2 Nonparametric Methods for Generating Bootstrap Samples and Obtaining
Bootstrap Estimates 227
13.3 Bootstrap Operational Considerations 231
13.4 Nonparametric Bootstrap Confidence Interval Methods 233
14 Parametric Bootstrap and Other Simulation-Based Statistical Intervals
245
14.1 Introduction 246
14.2 Parametric Bootstrap Samples and Bootstrap Estimates 247
14.3 Bootstrap Confidence Intervals Based on Pivotal Quantities 250
14.4 Generalized Pivotal Quantities 253
14.5 Simulation-Based Tolerance Intervals for Location-Scale or
Log-Location-Scale Distribu-tions 258
14.6 Simulation-Based Prediction Intervals and One-Sided Prediction Bounds
for k of m Fu-ture Observations from Location-Scale or Log-Location-Scale
Distributions 260
14.7 Other Simulation and Bootstrap Methods and Application to Other
Distributions and Models 263
15 Introduction to Bayesian Statistical Intervals 270
15.1 Bayesian Inference: Overview 271
15.2 Bayesian Inference: an Illustrative Example 274
15.3 More About Specification of a Prior Distribution 283
15.4 Implementing Bayesian Analyses Using Markov Chain Monte Carlo
Simulation 286
15.5 Bayesian Tolerance and Prediction Intervals 291
16 Bayesian Statistical Intervals for the Binomial, Poisson and Normal
Distributions 297
16.1 Bayesian Intervals for the Binomial Distribution 298
16.2 Bayesian Intervals for the Poisson Distribution 306
16.3 Bayesian Intervals for the Normal Distribution 311
17 Statistical Intervals for Bayesian Hierarchical Models 321
17.1 Bayesian Hierarchical Models and Random Effects 322
17.2 Normal Distribution Hierarchical Models 323
17.3 Binomial Distribution Hierarchical Models 325
17.4 Poisson Distribution Hierarchical Models 328
17.5 Longitudinal Repeated Measures Models 329
18 Advanced Case Studies 335
18.1 Confidence Interval for the Proportion of Defective Integrated
Circuits 336
18.2 Confidence Intervals for Components of Variance in a Measurement
Process 339
18.3 Tolerance Interval to Characterize the Distribution of Process Output
in the Presence of Measurement Error 344
18.4 Confidence Interval for the Proportion of Product Conforming to a
Two-Sided Specification345
18.5 Confidence Interval for the Treatment Effect in a Marketing Campaign
348
18.6 Confidence Interval for the Probability of Detection with Limited
Hit-Miss Data 349
18.7 Using Prior Information to Estimate the Service-Life Distribution of a
Rocket Motor 353
Epilogue 357
A Notation and Acronyms 360
B Generic Definition of Statistical Intervals and Formulas for Computing
Coverage Probabilities 367
B.1 Introduction 367
B.2 Two-sided Confidence Intervals and One-sided Confidence Bounds for
Distribution Pa-rameters or a Function of Parameters 368
B.3 Two Sided Control-the-Center Tolerance Intervals to Contain at Least a
Specified Pro-portion of a Distribution 371
B.4 Two Sided Tolerance Intervals to Control Both Tails of a Distribution
374
B.5 One-Sided Tolerance Bounds 377
B.6 Two-sided Prediction Intervals and One-Sided Prediction Bounds for
Future Observations378
B.7 Two-Sided Simultaneous Prediction Intervals and One-Sided Simultaneous
Prediction Bounds 381
B.8 Calibration of Statistical Intervals 383
C Useful Probability Distributions 384
C.1 Probability Distribution and R Computations 384
C.2 Important Characteristics of Random Variables 385
C.3 Continuous Distributions 388
C.4 Discrete Distributions 398
D General Results from Statistical Theory and Some Methods Used to
Construct Sta-tistical Intervals 404
D.1 cdfs and pdfs of Functions of Random Variables 405
D.2 Statistical Error Propagation-The Delta Method 409
D.3 Likelihood and Fisher Information Matrices 410
D.4 Convergence in Distribution 413
D.5 Outline of General ML Theory 415
D.6 The CDF pivotal method for constructing confidence intervals 419
D.7 Bonferroni approximate statistical intervals 424
E Pivotal Methods for Constructing Parametric Statistical Intervals 427
E.1 General definition and examples of pivotal quantities 428
E.2 Pivotal Quantities for the Normal Distribution 428
E.3 Confidence intervals for a Normal Distribution Based on Pivotal
Quantities 429
E.4 Confidence Intervals for Two Normal Distributions Based on Pivotal
Quantities 432
E.5 Tolerance Intervals for a Normal Distribution Based on Pivotal
Quantities 432
E.6 Normal Distribution Prediction Intervals Based on Pivotal Quantities
434
E.7 Pivotal Quantities for Log-Location-Scale Distributions 436
F Generalized Pivotal Quantities 440
F.1 Definition of Generalized Pivotal Quantities 440
F.2 A Substitution Method to Obtain GPQs 441
F.3 Examples of GPQs for Functions of Location-Scale Distribution
Parameters 441
F.4 Conditions for Exact Intervals Derived from GPQs 443
G Distribution-Free Intervals Based on Order Statistics 446
G.1 Basic Statistical Results Used in this Appendix 446
G.2 Distribution-Free Confidence Intervals and Bounds for a Distribution
Quantile 447
G.3 Distribution-Free Tolerance Intervals to Contain a Given Proportion of
a Distribution 448
G.4 Distribution-Free Prediction Interval to Contain a Specified Ordered
Observation From a Future Sample 449
G.5 Distribution-Free Prediction Intervals and Bounds to Contain at Least k
of m Future Observations From a Future Sample 451
H Basic Results from Bayesian Inference Models 455
H.1 Basic Statistical Results Used in this Appendix 455
H.2 Bayes' Theorem 456
H.3 Conjugate Prior Distributions 456
H.4 Jeffreys Prior Distributions 459
H.5 Posterior Predictive Distributions 463
H.6 Posterior Predictive Distributions Based on Jeffreys Prior
Distributions 465
I Probability of Successful Demonstration 468
I.1 Demonstration Tests Based on a Normal Distribution Assumption 468
I.2 Distribution-Free Demonstration Tests 469
J Tables 471
References 508
Subject Index 525