Structural Health Monitoring (eBook, PDF)
A Machine Learning Perspective
Alle Infos zum eBook verschenken
Structural Health Monitoring (eBook, PDF)
A Machine Learning Perspective
- Format: PDF
- Merkliste
- Auf die Merkliste
- Bewerten Bewerten
- Teilen
- Produkt teilen
- Produkterinnerung
- Produkterinnerung
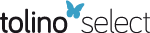
Hier können Sie sich einloggen
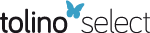
Bitte loggen Sie sich zunächst in Ihr Kundenkonto ein oder registrieren Sie sich bei bücher.de, um das eBook-Abo tolino select nutzen zu können.
Written by global leaders and pioneers in the field, this book is a must-have read for researchers, practicing engineers and university faculty working in SHM. Structural Health Monitoring: A Machine Learning Perspective is the first comprehensive book on the general problem of structural health monitoring. The authors, renowned experts in the field, consider structural health monitoring in a new manner by casting the problem in the context of a machine learning/statistical pattern recognition paradigm, first explaining the paradigm in general terms then explaining the process in detail with…mehr
- Geräte: PC
- mit Kopierschutz
- eBook Hilfe
- Größe: 44.02MB
- Charles R. FarrarStructural Health Monitoring (eBook, ePUB)117,99 €
- Robert E. MelchersStructural Reliability Analysis and Prediction (eBook, PDF)92,99 €
- Ryan L. HarneHarnessing Bistable Structural Dynamics (eBook, PDF)130,99 €
- Feng FuAdvanced Modelling Techniques in Structural Design (eBook, PDF)93,99 €
- Arbitrary Lagrangian Eulerian and Fluid-Structure Interaction (eBook, PDF)139,99 €
- Sukhvarsh JerathStructural Stability Theory and Practice (eBook, PDF)113,99 €
- Karl-Eugen KurrerThe History of the Theory of Structures (eBook, PDF)124,99 €
-
-
-
Dieser Download kann aus rechtlichen Gründen nur mit Rechnungsadresse in A, B, BG, CY, CZ, D, DK, EW, E, FIN, F, GR, HR, H, IRL, I, LT, L, LR, M, NL, PL, P, R, S, SLO, SK ausgeliefert werden.
- Produktdetails
- Verlag: John Wiley & Sons
- Seitenzahl: 656
- Erscheinungstermin: 10. Oktober 2012
- Englisch
- ISBN-13: 9781118443200
- Artikelnr.: 37354671
- Verlag: John Wiley & Sons
- Seitenzahl: 656
- Erscheinungstermin: 10. Oktober 2012
- Englisch
- ISBN-13: 9781118443200
- Artikelnr.: 37354671
- Herstellerkennzeichnung Die Herstellerinformationen sind derzeit nicht verfügbar.
Acknowledgements xix
1 Introduction 1
1.1 How Engineers and Scientists Study Damage 2
1.2 Motivation for Developing SHM Technology 3
1.3 Definition of Damage 4
1.4 A Statistical Pattern Recognition Paradigm for SHM 7
1.4.1 Operational Evaluation 10
1.4.2 Data Acquisition 10
1.4.3 Data Normalisation 10
1.4.4 Data Cleansing 11
1.4.5 Data Compression 11
1.4.6 Data Fusion 11
1.4.7 Feature Extraction 12
1.4.8 Statistical Modelling for Feature Discrimination 12
1.5 Local versus Global Damage Detection 13
1.6 Fundamental Axioms of Structural Health Monitoring 14
1.7 The Approach Taken in This Book 15
References 15
2 Historical Overview 17
2.1 Rotating Machinery Applications 17
2.1.1 Operational Evaluation for Rotating Machinery 18
2.1.2 Data Acquisition for Rotating Machinery 18
2.1.3 Feature Extraction for Rotating Machinery 19
2.1.4 Statistical Modelling for Damage Detection in Rotating Machinery 20
2.1.5 Concluding Comments about Condition Monitoring of Rotating Machinery
21
2.2 Offshore Oil Platforms 21
2.2.1 Operational Evaluation for Offshore Platforms 21
2.2.2 Data Acquisition for Offshore Platforms 24
2.2.3 Feature Extraction for Offshore Platforms 24
2.2.4 Statistical Modelling for Offshore Platforms 25
2.2.5 Lessons Learned from Offshore Oil Platform Structural Health
Monitoring Studies 25
2.3 Aerospace Structures 25
2.3.1 Operational Evaluation for Aerospace Structures 28
2.3.2 Data Acquisition for Aerospace Structures 29
2.3.3 Feature Extraction and Statistical Modelling for Aerospace Structures
31
2.3.4 Statistical Models Used for Aerospace SHM Applications 32
2.3.5 Concluding Comments about Aerospace SHM Applications 32
2.4 Civil Engineering Infrastructure 32
2.4.1 Operational Evaluation for Bridge Structures 34
2.4.2 Data Acquisition for Bridge Structures 34
2.4.3 Features Based on Modal Properties 35
2.4.4 Statistical Classification of Features for Civil Engineering
Infrastructure 36
2.4.5 Applications to Bridge Structures 36
2.5 Summary 37
References 38
3 Operational Evaluation 45
3.1 Economic and Life-Safety Justifications for Structural Health
Monitoring 45
3.2 Defining the Damage to Be Detected 46
3.3 The Operational and Environmental Conditions 47
3.4 Data Acquisition Limitations 47
3.5 Operational Evaluation Example: Bridge Monitoring 48
3.6 Operational Evaluation Example: Wind Turbines 51
3.7 Concluding Comment on Operational Evaluation 52
References 52
4 Sensing and Data Acquisition 53
4.1 Introduction 53
4.2 Sensing and Data Acquisition Strategies for SHM 53
4.2.1 Strategy I 54
4.2.2 Strategy II 54
4.3 Conceptual Challenges for Sensing and Data Acquisition Systems 55
4.4 What Types of Data Should Be Acquired? 56
4.4.1 Dynamic Input and Response Quantities 57
4.4.2 Other Damage-Sensitive Physical Quantities 59
4.4.3 Environmental Quantities 59
4.4.4 Operational Quantities 60
4.5 Current SHM Sensing Systems 60
4.5.1 Wired Systems 60
4.5.2 Wireless Systems 61
4.6 Sensor Network Paradigms 63
4.6.1 Sensor Arrays Directly Connected to Central Processing Hardware 64
4.6.2 Decentralised Processing with Hopping Connection 65
4.6.3 Decentralised Processing with Hybrid Connection 66
4.7 Future Sensing Network Paradigms 67
4.8 Defining the Sensor System Properties 68
4.8.1 Required Sensitivity and Range 70
4.8.2 Required Bandwidth and Frequency Resolution 71
4.8.3 Sensor Number and Locations 71
4.8.4 Sensor Calibration, Stability and Reliability 72
4.9 Define the Data Sampling Parameters 73
4.10 Define the Data Acquisition System 74
4.11 Active versus Passive Sensing 75
4.12 Multiscale Sensing 75
4.13 Powering the Sensing System 77
4.14 Signal Conditioning 77
4.15 Sensor and Actuator Optimisation 78
4.16 Sensor Fusion 79
4.17 Summary of Sensing and Data Acquisition Issues for Structural Health
Monitoring 82
References 83
5 Case Studies 87
5.1 The I-40 Bridge 87
5.1.1 Preliminary Testing and Data Acquisition 89
5.1.2 Undamaged Ambient Vibration Tests 90
5.1.3 Forced Vibration Tests 91
5.2 The Concrete Column 92
5.2.1 Quasi-Static Loading 95
5.2.2 Dynamic Excitation 95
5.2.3 Data Acquisition 95
5.3 The 8-DOF System 98
5.3.1 Physical Parameters 100
5.3.2 Data Acquisition 100
5.4 Simulated Building Structure 100
5.4.1 Experimental Procedure and Data Acquisition 101
5.4.2 Measured Data 102
5.5 The Alamosa Canyon Bridge 104
5.5.1 Experimental Procedures and Data Acquisition 104
5.5.2 Environmental Measurements 107
5.5.3 Vibration Tests Performed to Study Variability of Modal Properties
108
5.6 The Gnat Aircraft 108
5.6.1 Simulating Damage with a Modified Inspection Panel 109
5.6.2 Simulating Damage by Panel Removal 112
References 116
6 Introduction to Probability and Statistics 119
6.1 Introduction 119
6.2 Probability: Basic Definitions 120
6.3 Random Variables and Distributions 122
6.4 Expected Values 125
6.5 The Gaussian Distribution (and Others) 130
6.6 Multivariate Statistics 132
6.7 The Multivariate Gaussian Distribution 133
6.8 Conditional Probability and the Bayes Theorem 134
6.9 Confidence Limits and Cumulative Distribution Functions 137
6.10 Outlier Analysis 140
6.10.1 Outliers in Univariate Data 140
6.10.2 Outliers in Multivariate Data 141
6.10.3 Calculation of Critical Values of Discordancy or Thresholds 141
6.11 Density Estimation 142
6.12 Extreme Value Statistics 148
6.12.1 Introduction 148
6.12.2 Basic Theory 148
6.12.3 Determination of Limit Distributions 151
6.13 Dimension Reduction - Principal Component Analysis 155
6.13.1 Simple Projection 156
6.13.2 Principal Component Analysis (PCA) 156
6.14 Conclusions 158
References 159
7 Damage-Sensitive Features 161
7.1 Common Waveforms and Spectral Functions Used in the Feature Extraction
Process 163
7.1.1 Waveform Comparisons 164
7.1.2 Autocorrelation and Cross-Correlation Functions 165
7.1.3 The Power Spectral and Cross-Spectral Density Functions 166
7.1.4 The Impulse Response Function and the Frequency Response Function 168
7.1.5 The Coherence Function 169
7.1.6 Some Remarks Regarding Waveforms and Spectra 170
7.2 Basic Signal Statistics 171
7.3 Transient Signals: Temporal Moments 178
7.4 Transient Signals: Decay Measures 181
7.5 Acoustic Emission Features 183
7.6 Features Used with Guided-Wave Approaches to SHM 185
7.6.1 Preprocessing 186
7.6.2 Baseline Comparisons 186
7.6.3 Damage Localisation 188
7.7 Features Used with Impedance Measurements 188
7.8 Basic Modal Properties 191
7.8.1 Resonance Frequencies 192
7.8.2 Inverse versus Forward Modelling Approaches to Feature Extraction 194
7.8.3 Resonance Frequencies: The Forward Approach 195
7.8.4 Resonance Frequencies: Sensitivity Issues 195
7.8.5 Mode Shapes 197
7.8.6 Load-Dependent Ritz Vectors 203
7.9 Features Derived from Basic Modal Properties 206
7.9.1 Mode Shape Curvature 207
7.9.2 Modal Strain Energy 210
7.9.3 Modal Flexibility 215
7.10 Model Updating Approaches 218
7.10.1 Objective Functions and Constraints 220
7.10.2 Direct Solution for the Modal Force Error 221
7.10.3 Optimal Matrix Update Methods 222
7.10.4 Sensitivity-Based Update Methods 226
7.10.5 Eigenstructure Assignment Method 230
7.10.6 Hybrid Matrix Update Methods 231
7.10.7 Concluding Comment on Model Updating Approaches 231
7.11 Time Series Models 232
7.12 Feature Selection 234
7.12.1 Sensitivity Analysis 234
7.12.2 Information Content 238
7.12.3 Assessment of Robustness 239
7.12.4 Optimisation Procedures 239
7.13 Metrics 239
7.14 Concluding Comments 240
References 240
8 Features Based on Deviations from Linear Response 245
8.1 Types of Damage that Can Produce a Nonlinear System Response 245
8.2 Motivation for Exploring Nonlinear System Identification Methods for
SHM 248
8.2.1 Coherence Function 250
8.2.2 Linearity and Reciprocity Checks 251
8.2.3 Harmonic Distortion 256
8.2.4 Frequency Response Function Distortions 261
8.2.5 Probability Density Function 264
8.2.6 Correlation Tests 266
8.2.7 The Holder Exponent 266
8.2.8 Linear Time Series Prediction Errors 271
8.2.9 Nonlinear Time Series Models 273
8.2.10 Hilbert Transform 277
8.2.11 Nonlinear Acoustics Methods 279
8.3 Applications of Nonlinear Dynamical Systems Theory 280
8.3.1 Modelling a Cracked Beam as a Bilinear System 281
8.3.2 Chaotic Interrogation of a Damaged Beam 282
8.3.3 Local Attractor Variance 284
8.3.4 Detection of Damage Using the Local Attractor Variance 286
8.4 Nonlinear System Identification Approaches 288
8.4.1 Restoring Force Surface Model 288
8.5 Concluding Comments Regarding Feature Extraction Based on Nonlinear
System Response 291
References 292
9 Machine Learning and Statistical Pattern Recognition 295
9.1 Introduction 295
9.2 Intelligent Damage Detection 295
9.3 Data Processing and Fusion for Damage Identification 298
9.4 Statistical Pattern Recognition: Hypothesis Testing 300
9.5 Statistical Pattern Recognition: General Frameworks 303
9.6 Discriminant Functions and Decision Boundaries 306
9.7 Decision Trees 308
9.8 Training - Maximum Likelihood 309
9.9 Nearest Neighbour Classification 312
9.10 Case Study: An Acoustic Emission Experiment 312
9.10.1 Analysis and Classification of the AE Data 314
9.11 Summary 320
References 320
10 Unsupervised Learning - Novelty Detection 321
10.1 Introduction 321
10.2 A Gaussian-Distributed Normal Condition - Outlier Analysis 322
10.3 A Non-Gaussian Normal Condition - A Neural Network Approach 325
10.4 Nonparametric Density Estimation - A Case Study 329
10.4.1 The Experimental Structure and Data Capture 331
10.4.2 Preprocessing of Data and Features 332
10.4.3 Novelty Detection 333
10.5 Statistical Process Control 338
10.5.1 Feature Extraction Based on Autoregressive Modelling 339
10.5.2 The X-Bar Control Chart: An Experimental Case Study 340
10.6 Other Control Charts and Multivariate SPC 343
10.6.1 The S Control Chart 344
10.6.2 The CUSUM Chart 344
10.6.3 The EWMA Chart 345
10.6.4 The Hotelling or Shewhart T2 Chart 346
10.6.5 The Multivariate CUSUM Chart 347
10.6.6 The Multivariate EWMA Chart 347
10.7 Thresholds for Novelty Detection 348
10.7.1 Extreme Value Statistics 348
10.7.2 Type I and Type II Errors: The ROC Curve 354
10.8 Summary 359
References 359
11 Supervised Learning - Classification and Regression 361
11.1 Introduction 361
11.2 Artificial Neural Networks 361
11.2.1 Biological Motivation 361
11.2.2 The Parallel Processing Paradigm 364
11.2.3 The Artificial Neuron 365
11.2.4 The Perceptron 366
11.2.5 The Multilayer Perceptron 367
11.3 A Neural Network Case Study: A Classification Problem 372
11.4 Other Neural Network Structures 374
11.4.1 Feedforward Networks 374
11.4.2 Recurrent Networks 375
11.4.3 Cellular Networks 375
11.5 Statistical Learning Theory and Kernel Methods 375
11.5.1 Structural Risk Minimisation 375
11.5.2 Support Vector Machines 377
11.5.3 Kernels 381
11.6 Case Study II: Support Vector Classification 382
11.7 Support Vector Regression 384
11.8 Case Study III: Support Vector Regression 386
11.9 Feature Selection for Classification Using Genetic Algorithms 389
11.9.1 Feature Selection Using Engineering Judgement 390
11.9.2 Genetic Feature Selection 390
11.9.3 Issues of Network Generalisation 395
11.9.4 Discussion and Conclusions 397
11.10 Discussion and Conclusions 398
References 400
12 Data Normalisation 403
12.1 Introduction 403
12.2 An Example Where Data Normalisation Was Neglected 405
12.3 Sources of Environmental and Operational Variability 406
12.4 Sensor System Design 409
12.5 Modelling Operational and Environmental Variability 411
12.6 Look-Up Tables 414
12.7 Machine Learning Approaches to Data Normalisation 421
12.7.1 Auto-Associative Neural Networks 422
12.7.2 Factor Analysis 423
12.7.3 Mahalanobis Squared-Distance (MSD) 424
12.7.4 Singular Value Decomposition 424
12.7.5 Application to the Simulated Building Structure Data 425
12.8 Intelligent Feature Selection: A Projection Method 429
12.9 Cointegration 431
12.9.1 Theory 432
12.9.2 Illustration 433
12.10 Summary 436
References 436
13 Fundamental Axioms of Structural Health Monitoring 439
13.1 Introduction 439
13.2 Axiom I. All Materials Have Inherent Flaws or Defects 440
13.3 Axiom II. Damage Assessment Requires a Comparison between Two System
States 441
13.4 Axiom III. Identifying the Existence and Location of Damage Can Be
Done in an Unsupervised Learning Mode, but Identifying the Type of Damage
Present and the Damage Severity Can Generally Only Be Done in a Supervised
Learning Mode 444
13.5 Axiom IVa. Sensors Cannot Measure Damage. Feature Extraction through
Signal Processing and Statistical Classification Are Necessary to Convert
Sensor Data into Damage Information 446
13.6 Axiom IVb. Without Intelligent Feature Extraction, the More Sensitive
a Measurement is to Damage, the More Sensitive it is to Changing
Operational and Environmental Conditions 447
13.7 Axiom V. The Length and Time Scales Associated with Damage Initiation
and Evolution Dictate the Required Properties of the SHM Sensing System 448
13.8 Axiom VI. There is a Trade-off between the Sensitivity to Damage of an
Algorithm and Its Noise Rejection Capability 449
13.9 Axiom VII. The Size of Damage that Can Be Detected from Changes in
System Dynamics is Inversely Proportional to the Frequency Range of
Excitation 451
13.10 Axiom VIII. Damage Increases the Complexity of a Structure 454
13.11 Summary 458
References 459
14 Damage Prognosis 461
14.1 Introduction 461
14.2 Motivation for Damage Prognosis 462
14.3 The Current State of Damage Prognosis 463
14.4 Defining the Damage Prognosis Problem 464
14.5 The Damage Prognosis Process 465
14.6 Emerging Technologies Impacting the Damage Prognosis Process 467
14.6.1 Damage Sensing Systems 467
14.6.2 Prediction Modelling for Future Loading Estimates 467
14.6.3 Model Verification and Validation 467
14.6.4 Reliability Analysis for Damage Prognosis Decision Making 467
14.7 A Prognosis Case Study: Crack Propagation in a Titanium Plate 468
14.7.1 The Computational Model 469
14.7.2 Monte Carlo Simulation 471
14.7.3 Issues 471
14.8 Damage Prognosis of UAV Structural Components 474
14.9 Concluding Comments on Damage Prognosis 475
14.10 Cradle-to-Grave System State Awareness 476
References 476
Appendix A Signal Processing for SHM 479
A.1 Deterministic and Random Signals 479
A.1.1 Basic Definitions 479
A.1.2 Transducers, Sensors and Calibration 480
A.1.3 Classification of Deterministic Signals 481
A.1.4 Classification of Random Signals 485
A.2 Fourier Analysis and Spectra 489
A.2.1 Fourier Series 489
A.2.2 The Square Wave Revisited 493
A.2.3 A First Look at Spectra 495
A.2.4 The Exponential Form of the Fourier Series 496
A.3 The Fourier Transform 497
A.3.1 Basic Transform Theory 497
A.3.2 An Interesting Function that is not a Function 499
A.3.3 The Fourier Transform of a Periodic Function 501
A.3.4 The Fourier Transform of a Pulse/Impulse 502
A.3.5 The Convolution Theorem 504
A.3.6 Parseval's Theorem 506
A.3.7 The Effect of a Finite Time Window 506
A.3.8 The Effect of Differentiation and Integration 509
A.4 Frequency Response Functions and the Impulse Response 510
A.4.1 Basic Definitions 510
A.4.2 Harmonic Probing 511
A.5 The Discrete Fourier Transform 512
A.5.1 Basic Definitions 512
A.5.2 More About Sampling 516
A.5.3 The Fast Fourier Transform 519
A.5.4 The DFT of a Sinusoid 524
A.6 Practical Matters: Windows and Averaging 525
A.6.1 Windows 525
A.6.2 The Harris Test 527
A.6.3 Averaging and Power Spectral Density 528
A.7 Correlations and Spectra 532
A.8 FRF Estimation and Coherence 535
A.8.1 FRF Estimation I 535
A.8.2 The Coherence Function 536
A.8.3 FRF Estimators II 538
A.9 Wavelets 540
A.9.1 Introduction and Continuous Wavelets 540
A.9.2 Discrete and Orthogonal Wavelets 549
A.10 Filters 564
A.10.1 Introduction to Filters 564
A.10.2 A Digital Low-Pass Filter 566
A.10.3 A High-Pass Filter 569
A.10.4 A Simple Classification of Filters 570
A.10.5 Filter Design 571
A.10.6 The Bilinear Transformation 573
A.10.7 An Example of Digital Filter Design 576
A.10.8 Combining Filters 578
A.10.9 General Butterworth Filters 579
A.11 System Identification 583
A.11.1 Introduction 583
A.11.2 Discrete-Time Models in the Frequency Domain 586
A.11.3 Least-Squares Parameter Estimation 587
A.11.4 Parameter Uncertainty 589
A.11.5 A Case Study 590
A.12 Summary 591
References 592
Appendix B EssentialLinear StructuralDynamics 593
B.1 Continuous-Time Systems: The Time Domain 593
B.2 Continuous-Time Systems: The Frequency Domain 600
B.3 The Impulse Response 603
B.4 Discrete-Time Models: Time Domain 605
B.5 Multi-Degree-of-Freedom (MDOF) Systems 607
B.6 Modal Analysis 613
B.6.1 Free, Undamped Motion 613
B.6.2 Free, Damped Motion 617
B.6.3 Forced, Damped Motion 618
References 621
Index 623
Acknowledgements xix
1 Introduction 1
1.1 How Engineers and Scientists Study Damage 2
1.2 Motivation for Developing SHM Technology 3
1.3 Definition of Damage 4
1.4 A Statistical Pattern Recognition Paradigm for SHM 7
1.4.1 Operational Evaluation 10
1.4.2 Data Acquisition 10
1.4.3 Data Normalisation 10
1.4.4 Data Cleansing 11
1.4.5 Data Compression 11
1.4.6 Data Fusion 11
1.4.7 Feature Extraction 12
1.4.8 Statistical Modelling for Feature Discrimination 12
1.5 Local versus Global Damage Detection 13
1.6 Fundamental Axioms of Structural Health Monitoring 14
1.7 The Approach Taken in This Book 15
References 15
2 Historical Overview 17
2.1 Rotating Machinery Applications 17
2.1.1 Operational Evaluation for Rotating Machinery 18
2.1.2 Data Acquisition for Rotating Machinery 18
2.1.3 Feature Extraction for Rotating Machinery 19
2.1.4 Statistical Modelling for Damage Detection in Rotating Machinery 20
2.1.5 Concluding Comments about Condition Monitoring of Rotating Machinery
21
2.2 Offshore Oil Platforms 21
2.2.1 Operational Evaluation for Offshore Platforms 21
2.2.2 Data Acquisition for Offshore Platforms 24
2.2.3 Feature Extraction for Offshore Platforms 24
2.2.4 Statistical Modelling for Offshore Platforms 25
2.2.5 Lessons Learned from Offshore Oil Platform Structural Health
Monitoring Studies 25
2.3 Aerospace Structures 25
2.3.1 Operational Evaluation for Aerospace Structures 28
2.3.2 Data Acquisition for Aerospace Structures 29
2.3.3 Feature Extraction and Statistical Modelling for Aerospace Structures
31
2.3.4 Statistical Models Used for Aerospace SHM Applications 32
2.3.5 Concluding Comments about Aerospace SHM Applications 32
2.4 Civil Engineering Infrastructure 32
2.4.1 Operational Evaluation for Bridge Structures 34
2.4.2 Data Acquisition for Bridge Structures 34
2.4.3 Features Based on Modal Properties 35
2.4.4 Statistical Classification of Features for Civil Engineering
Infrastructure 36
2.4.5 Applications to Bridge Structures 36
2.5 Summary 37
References 38
3 Operational Evaluation 45
3.1 Economic and Life-Safety Justifications for Structural Health
Monitoring 45
3.2 Defining the Damage to Be Detected 46
3.3 The Operational and Environmental Conditions 47
3.4 Data Acquisition Limitations 47
3.5 Operational Evaluation Example: Bridge Monitoring 48
3.6 Operational Evaluation Example: Wind Turbines 51
3.7 Concluding Comment on Operational Evaluation 52
References 52
4 Sensing and Data Acquisition 53
4.1 Introduction 53
4.2 Sensing and Data Acquisition Strategies for SHM 53
4.2.1 Strategy I 54
4.2.2 Strategy II 54
4.3 Conceptual Challenges for Sensing and Data Acquisition Systems 55
4.4 What Types of Data Should Be Acquired? 56
4.4.1 Dynamic Input and Response Quantities 57
4.4.2 Other Damage-Sensitive Physical Quantities 59
4.4.3 Environmental Quantities 59
4.4.4 Operational Quantities 60
4.5 Current SHM Sensing Systems 60
4.5.1 Wired Systems 60
4.5.2 Wireless Systems 61
4.6 Sensor Network Paradigms 63
4.6.1 Sensor Arrays Directly Connected to Central Processing Hardware 64
4.6.2 Decentralised Processing with Hopping Connection 65
4.6.3 Decentralised Processing with Hybrid Connection 66
4.7 Future Sensing Network Paradigms 67
4.8 Defining the Sensor System Properties 68
4.8.1 Required Sensitivity and Range 70
4.8.2 Required Bandwidth and Frequency Resolution 71
4.8.3 Sensor Number and Locations 71
4.8.4 Sensor Calibration, Stability and Reliability 72
4.9 Define the Data Sampling Parameters 73
4.10 Define the Data Acquisition System 74
4.11 Active versus Passive Sensing 75
4.12 Multiscale Sensing 75
4.13 Powering the Sensing System 77
4.14 Signal Conditioning 77
4.15 Sensor and Actuator Optimisation 78
4.16 Sensor Fusion 79
4.17 Summary of Sensing and Data Acquisition Issues for Structural Health
Monitoring 82
References 83
5 Case Studies 87
5.1 The I-40 Bridge 87
5.1.1 Preliminary Testing and Data Acquisition 89
5.1.2 Undamaged Ambient Vibration Tests 90
5.1.3 Forced Vibration Tests 91
5.2 The Concrete Column 92
5.2.1 Quasi-Static Loading 95
5.2.2 Dynamic Excitation 95
5.2.3 Data Acquisition 95
5.3 The 8-DOF System 98
5.3.1 Physical Parameters 100
5.3.2 Data Acquisition 100
5.4 Simulated Building Structure 100
5.4.1 Experimental Procedure and Data Acquisition 101
5.4.2 Measured Data 102
5.5 The Alamosa Canyon Bridge 104
5.5.1 Experimental Procedures and Data Acquisition 104
5.5.2 Environmental Measurements 107
5.5.3 Vibration Tests Performed to Study Variability of Modal Properties
108
5.6 The Gnat Aircraft 108
5.6.1 Simulating Damage with a Modified Inspection Panel 109
5.6.2 Simulating Damage by Panel Removal 112
References 116
6 Introduction to Probability and Statistics 119
6.1 Introduction 119
6.2 Probability: Basic Definitions 120
6.3 Random Variables and Distributions 122
6.4 Expected Values 125
6.5 The Gaussian Distribution (and Others) 130
6.6 Multivariate Statistics 132
6.7 The Multivariate Gaussian Distribution 133
6.8 Conditional Probability and the Bayes Theorem 134
6.9 Confidence Limits and Cumulative Distribution Functions 137
6.10 Outlier Analysis 140
6.10.1 Outliers in Univariate Data 140
6.10.2 Outliers in Multivariate Data 141
6.10.3 Calculation of Critical Values of Discordancy or Thresholds 141
6.11 Density Estimation 142
6.12 Extreme Value Statistics 148
6.12.1 Introduction 148
6.12.2 Basic Theory 148
6.12.3 Determination of Limit Distributions 151
6.13 Dimension Reduction - Principal Component Analysis 155
6.13.1 Simple Projection 156
6.13.2 Principal Component Analysis (PCA) 156
6.14 Conclusions 158
References 159
7 Damage-Sensitive Features 161
7.1 Common Waveforms and Spectral Functions Used in the Feature Extraction
Process 163
7.1.1 Waveform Comparisons 164
7.1.2 Autocorrelation and Cross-Correlation Functions 165
7.1.3 The Power Spectral and Cross-Spectral Density Functions 166
7.1.4 The Impulse Response Function and the Frequency Response Function 168
7.1.5 The Coherence Function 169
7.1.6 Some Remarks Regarding Waveforms and Spectra 170
7.2 Basic Signal Statistics 171
7.3 Transient Signals: Temporal Moments 178
7.4 Transient Signals: Decay Measures 181
7.5 Acoustic Emission Features 183
7.6 Features Used with Guided-Wave Approaches to SHM 185
7.6.1 Preprocessing 186
7.6.2 Baseline Comparisons 186
7.6.3 Damage Localisation 188
7.7 Features Used with Impedance Measurements 188
7.8 Basic Modal Properties 191
7.8.1 Resonance Frequencies 192
7.8.2 Inverse versus Forward Modelling Approaches to Feature Extraction 194
7.8.3 Resonance Frequencies: The Forward Approach 195
7.8.4 Resonance Frequencies: Sensitivity Issues 195
7.8.5 Mode Shapes 197
7.8.6 Load-Dependent Ritz Vectors 203
7.9 Features Derived from Basic Modal Properties 206
7.9.1 Mode Shape Curvature 207
7.9.2 Modal Strain Energy 210
7.9.3 Modal Flexibility 215
7.10 Model Updating Approaches 218
7.10.1 Objective Functions and Constraints 220
7.10.2 Direct Solution for the Modal Force Error 221
7.10.3 Optimal Matrix Update Methods 222
7.10.4 Sensitivity-Based Update Methods 226
7.10.5 Eigenstructure Assignment Method 230
7.10.6 Hybrid Matrix Update Methods 231
7.10.7 Concluding Comment on Model Updating Approaches 231
7.11 Time Series Models 232
7.12 Feature Selection 234
7.12.1 Sensitivity Analysis 234
7.12.2 Information Content 238
7.12.3 Assessment of Robustness 239
7.12.4 Optimisation Procedures 239
7.13 Metrics 239
7.14 Concluding Comments 240
References 240
8 Features Based on Deviations from Linear Response 245
8.1 Types of Damage that Can Produce a Nonlinear System Response 245
8.2 Motivation for Exploring Nonlinear System Identification Methods for
SHM 248
8.2.1 Coherence Function 250
8.2.2 Linearity and Reciprocity Checks 251
8.2.3 Harmonic Distortion 256
8.2.4 Frequency Response Function Distortions 261
8.2.5 Probability Density Function 264
8.2.6 Correlation Tests 266
8.2.7 The Holder Exponent 266
8.2.8 Linear Time Series Prediction Errors 271
8.2.9 Nonlinear Time Series Models 273
8.2.10 Hilbert Transform 277
8.2.11 Nonlinear Acoustics Methods 279
8.3 Applications of Nonlinear Dynamical Systems Theory 280
8.3.1 Modelling a Cracked Beam as a Bilinear System 281
8.3.2 Chaotic Interrogation of a Damaged Beam 282
8.3.3 Local Attractor Variance 284
8.3.4 Detection of Damage Using the Local Attractor Variance 286
8.4 Nonlinear System Identification Approaches 288
8.4.1 Restoring Force Surface Model 288
8.5 Concluding Comments Regarding Feature Extraction Based on Nonlinear
System Response 291
References 292
9 Machine Learning and Statistical Pattern Recognition 295
9.1 Introduction 295
9.2 Intelligent Damage Detection 295
9.3 Data Processing and Fusion for Damage Identification 298
9.4 Statistical Pattern Recognition: Hypothesis Testing 300
9.5 Statistical Pattern Recognition: General Frameworks 303
9.6 Discriminant Functions and Decision Boundaries 306
9.7 Decision Trees 308
9.8 Training - Maximum Likelihood 309
9.9 Nearest Neighbour Classification 312
9.10 Case Study: An Acoustic Emission Experiment 312
9.10.1 Analysis and Classification of the AE Data 314
9.11 Summary 320
References 320
10 Unsupervised Learning - Novelty Detection 321
10.1 Introduction 321
10.2 A Gaussian-Distributed Normal Condition - Outlier Analysis 322
10.3 A Non-Gaussian Normal Condition - A Neural Network Approach 325
10.4 Nonparametric Density Estimation - A Case Study 329
10.4.1 The Experimental Structure and Data Capture 331
10.4.2 Preprocessing of Data and Features 332
10.4.3 Novelty Detection 333
10.5 Statistical Process Control 338
10.5.1 Feature Extraction Based on Autoregressive Modelling 339
10.5.2 The X-Bar Control Chart: An Experimental Case Study 340
10.6 Other Control Charts and Multivariate SPC 343
10.6.1 The S Control Chart 344
10.6.2 The CUSUM Chart 344
10.6.3 The EWMA Chart 345
10.6.4 The Hotelling or Shewhart T2 Chart 346
10.6.5 The Multivariate CUSUM Chart 347
10.6.6 The Multivariate EWMA Chart 347
10.7 Thresholds for Novelty Detection 348
10.7.1 Extreme Value Statistics 348
10.7.2 Type I and Type II Errors: The ROC Curve 354
10.8 Summary 359
References 359
11 Supervised Learning - Classification and Regression 361
11.1 Introduction 361
11.2 Artificial Neural Networks 361
11.2.1 Biological Motivation 361
11.2.2 The Parallel Processing Paradigm 364
11.2.3 The Artificial Neuron 365
11.2.4 The Perceptron 366
11.2.5 The Multilayer Perceptron 367
11.3 A Neural Network Case Study: A Classification Problem 372
11.4 Other Neural Network Structures 374
11.4.1 Feedforward Networks 374
11.4.2 Recurrent Networks 375
11.4.3 Cellular Networks 375
11.5 Statistical Learning Theory and Kernel Methods 375
11.5.1 Structural Risk Minimisation 375
11.5.2 Support Vector Machines 377
11.5.3 Kernels 381
11.6 Case Study II: Support Vector Classification 382
11.7 Support Vector Regression 384
11.8 Case Study III: Support Vector Regression 386
11.9 Feature Selection for Classification Using Genetic Algorithms 389
11.9.1 Feature Selection Using Engineering Judgement 390
11.9.2 Genetic Feature Selection 390
11.9.3 Issues of Network Generalisation 395
11.9.4 Discussion and Conclusions 397
11.10 Discussion and Conclusions 398
References 400
12 Data Normalisation 403
12.1 Introduction 403
12.2 An Example Where Data Normalisation Was Neglected 405
12.3 Sources of Environmental and Operational Variability 406
12.4 Sensor System Design 409
12.5 Modelling Operational and Environmental Variability 411
12.6 Look-Up Tables 414
12.7 Machine Learning Approaches to Data Normalisation 421
12.7.1 Auto-Associative Neural Networks 422
12.7.2 Factor Analysis 423
12.7.3 Mahalanobis Squared-Distance (MSD) 424
12.7.4 Singular Value Decomposition 424
12.7.5 Application to the Simulated Building Structure Data 425
12.8 Intelligent Feature Selection: A Projection Method 429
12.9 Cointegration 431
12.9.1 Theory 432
12.9.2 Illustration 433
12.10 Summary 436
References 436
13 Fundamental Axioms of Structural Health Monitoring 439
13.1 Introduction 439
13.2 Axiom I. All Materials Have Inherent Flaws or Defects 440
13.3 Axiom II. Damage Assessment Requires a Comparison between Two System
States 441
13.4 Axiom III. Identifying the Existence and Location of Damage Can Be
Done in an Unsupervised Learning Mode, but Identifying the Type of Damage
Present and the Damage Severity Can Generally Only Be Done in a Supervised
Learning Mode 444
13.5 Axiom IVa. Sensors Cannot Measure Damage. Feature Extraction through
Signal Processing and Statistical Classification Are Necessary to Convert
Sensor Data into Damage Information 446
13.6 Axiom IVb. Without Intelligent Feature Extraction, the More Sensitive
a Measurement is to Damage, the More Sensitive it is to Changing
Operational and Environmental Conditions 447
13.7 Axiom V. The Length and Time Scales Associated with Damage Initiation
and Evolution Dictate the Required Properties of the SHM Sensing System 448
13.8 Axiom VI. There is a Trade-off between the Sensitivity to Damage of an
Algorithm and Its Noise Rejection Capability 449
13.9 Axiom VII. The Size of Damage that Can Be Detected from Changes in
System Dynamics is Inversely Proportional to the Frequency Range of
Excitation 451
13.10 Axiom VIII. Damage Increases the Complexity of a Structure 454
13.11 Summary 458
References 459
14 Damage Prognosis 461
14.1 Introduction 461
14.2 Motivation for Damage Prognosis 462
14.3 The Current State of Damage Prognosis 463
14.4 Defining the Damage Prognosis Problem 464
14.5 The Damage Prognosis Process 465
14.6 Emerging Technologies Impacting the Damage Prognosis Process 467
14.6.1 Damage Sensing Systems 467
14.6.2 Prediction Modelling for Future Loading Estimates 467
14.6.3 Model Verification and Validation 467
14.6.4 Reliability Analysis for Damage Prognosis Decision Making 467
14.7 A Prognosis Case Study: Crack Propagation in a Titanium Plate 468
14.7.1 The Computational Model 469
14.7.2 Monte Carlo Simulation 471
14.7.3 Issues 471
14.8 Damage Prognosis of UAV Structural Components 474
14.9 Concluding Comments on Damage Prognosis 475
14.10 Cradle-to-Grave System State Awareness 476
References 476
Appendix A Signal Processing for SHM 479
A.1 Deterministic and Random Signals 479
A.1.1 Basic Definitions 479
A.1.2 Transducers, Sensors and Calibration 480
A.1.3 Classification of Deterministic Signals 481
A.1.4 Classification of Random Signals 485
A.2 Fourier Analysis and Spectra 489
A.2.1 Fourier Series 489
A.2.2 The Square Wave Revisited 493
A.2.3 A First Look at Spectra 495
A.2.4 The Exponential Form of the Fourier Series 496
A.3 The Fourier Transform 497
A.3.1 Basic Transform Theory 497
A.3.2 An Interesting Function that is not a Function 499
A.3.3 The Fourier Transform of a Periodic Function 501
A.3.4 The Fourier Transform of a Pulse/Impulse 502
A.3.5 The Convolution Theorem 504
A.3.6 Parseval's Theorem 506
A.3.7 The Effect of a Finite Time Window 506
A.3.8 The Effect of Differentiation and Integration 509
A.4 Frequency Response Functions and the Impulse Response 510
A.4.1 Basic Definitions 510
A.4.2 Harmonic Probing 511
A.5 The Discrete Fourier Transform 512
A.5.1 Basic Definitions 512
A.5.2 More About Sampling 516
A.5.3 The Fast Fourier Transform 519
A.5.4 The DFT of a Sinusoid 524
A.6 Practical Matters: Windows and Averaging 525
A.6.1 Windows 525
A.6.2 The Harris Test 527
A.6.3 Averaging and Power Spectral Density 528
A.7 Correlations and Spectra 532
A.8 FRF Estimation and Coherence 535
A.8.1 FRF Estimation I 535
A.8.2 The Coherence Function 536
A.8.3 FRF Estimators II 538
A.9 Wavelets 540
A.9.1 Introduction and Continuous Wavelets 540
A.9.2 Discrete and Orthogonal Wavelets 549
A.10 Filters 564
A.10.1 Introduction to Filters 564
A.10.2 A Digital Low-Pass Filter 566
A.10.3 A High-Pass Filter 569
A.10.4 A Simple Classification of Filters 570
A.10.5 Filter Design 571
A.10.6 The Bilinear Transformation 573
A.10.7 An Example of Digital Filter Design 576
A.10.8 Combining Filters 578
A.10.9 General Butterworth Filters 579
A.11 System Identification 583
A.11.1 Introduction 583
A.11.2 Discrete-Time Models in the Frequency Domain 586
A.11.3 Least-Squares Parameter Estimation 587
A.11.4 Parameter Uncertainty 589
A.11.5 A Case Study 590
A.12 Summary 591
References 592
Appendix B EssentialLinear StructuralDynamics 593
B.1 Continuous-Time Systems: The Time Domain 593
B.2 Continuous-Time Systems: The Frequency Domain 600
B.3 The Impulse Response 603
B.4 Discrete-Time Models: Time Domain 605
B.5 Multi-Degree-of-Freedom (MDOF) Systems 607
B.6 Modal Analysis 613
B.6.1 Free, Undamped Motion 613
B.6.2 Free, Damped Motion 617
B.6.3 Forced, Damped Motion 618
References 621
Index 623