The Visual Organization (eBook, PDF)
Data Visualization, Big Data, and the Quest for Better Decisions
Alle Infos zum eBook verschenken
The Visual Organization (eBook, PDF)
Data Visualization, Big Data, and the Quest for Better Decisions
- Format: PDF
- Merkliste
- Auf die Merkliste
- Bewerten Bewerten
- Teilen
- Produkt teilen
- Produkterinnerung
- Produkterinnerung
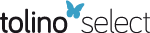
Hier können Sie sich einloggen
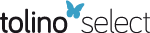
Bitte loggen Sie sich zunächst in Ihr Kundenkonto ein oder registrieren Sie sich bei bücher.de, um das eBook-Abo tolino select nutzen zu können.
The era of Big Data as arrived, and most organizations are woefully unprepared. Slowly, many are discovering that stalwarts like Excel spreadsheets, KPIs, standard reports, and even traditional business intelligence tools aren't sufficient. These old standbys can't begin to handle today's increasing streams, volumes, and types of data. Amidst all of the chaos, though, a new type of organization is emerging. In The Visual Organization, award-winning author and technology expert Phil Simon looks at how an increasingly number of organizations are embracing new dataviz tools and, more important, a…mehr
- Geräte: PC
- mit Kopierschutz
- eBook Hilfe
- Größe: 34.8MB
- Phil SimonThe Visual Organization (eBook, ePUB)38,99 €
- Alan AndersonBusiness Statistics For Dummies (eBook, PDF)16,99 €
- Phil SimonMessage Not Received (eBook, PDF)22,99 €
- Douglas W. HubbardHow to Measure Anything Workbook (eBook, PDF)20,99 €
- Jack M. KeenMaking Technology Investments Profitable (eBook, PDF)32,99 €
- Mark J. NigriniBenford's Law (eBook, PDF)54,99 €
- Randall BoltenPainting with Numbers (eBook, PDF)25,99 €
-
-
-
Dieser Download kann aus rechtlichen Gründen nur mit Rechnungsadresse in A, B, BG, CY, CZ, D, DK, EW, E, FIN, F, GR, HR, H, IRL, I, LT, L, LR, M, NL, PL, P, R, S, SLO, SK ausgeliefert werden.
Hinweis: Dieser Artikel kann nur an eine deutsche Lieferadresse ausgeliefert werden.
- Produktdetails
- Verlag: John Wiley & Sons
- Seitenzahl: 240
- Erscheinungstermin: 19. Februar 2014
- Englisch
- ISBN-13: 9781118858417
- Artikelnr.: 40515054
- Verlag: John Wiley & Sons
- Seitenzahl: 240
- Erscheinungstermin: 19. Februar 2014
- Englisch
- ISBN-13: 9781118858417
- Artikelnr.: 40515054
- Herstellerkennzeichnung Die Herstellerinformationen sind derzeit nicht verfügbar.
Preface xix
Acknowledgments xxv
How to Help This Book xxvii
Part I Book Overview and Background 1
Introduction 3
Adventures in Twitter Data Discovery 4
Contemporary Dataviz 101 9
Primary Objective 9
Benefits 11
More Important Than Ever 13
Revenge of the Laggards: The Current State of Dataviz 15
Book Overview 18
Defining the Visual Organization 19
Central Thesis of Book 19
Cui Bono? 20
Methodology: Story Matters Here 21
The Quest for Knowledge and Case Studies 24
Differentiation: A Note on Other Dataviz Texts 25
Plan of Attack 26
Next 27
Notes 27
Chapter 1 The Ascent of the Visual Organization 29
The Rise of Big Data 30
Open Data 30
The Burgeoning Data Ecosystem 33
The New Web: Visual, Semantic, and API-Driven 34
The Arrival of the Visual Web 34
Linked Data and a More Semantic Web 35
The Relative Ease of Accessing Data 36
Greater Efficiency via Clouds and Data Centers 37
Better Data Tools 38
Greater Organizational Transparency 40
The Copycat Economy: Monkey See, Monkey Do 41
Data Journalism and the Nate Silver Effect 41
Digital Man 44
The Arrival of the Visual Citizen 44
Mobility 47
The Visual Employee: A More Tech- and Data-Savvy Workforce 47
Navigating Our Data-Driven World 48
Next 49
Notes 49
Chapter 2 Transforming Data into Insights: The Tools 51
Dataviz: Part of an Intelligent and Holistic Strategy 52
The Tyranny of Terminology: Dataviz, BI, Reporting, Analytics, and KPIs 53
Do Visual Organizations Eschew All Tried-and-True Reporting Tools? 55
Drawing Some Distinctions 56
The Dataviz Fab Five 57
Applications from Large Enterprise Software Vendors 57
LESVs: The Case For 58
LESVs: The Case Against 59
Best-of-Breed Applications 61
Cost 62
Ease of Use and Employee Training 62
Integration and the Big Data World 63
Popular Open-Source Tools 64
D3.js 64
R 65
Others 66
Design Firms 66
Startups, Web Services, and Additional Resources 70
The Final Word: One Size Doesn't Fit All 72
Next 73
Notes 73
Part II Introducing the Visual Organization 75
Chapter 3 The Quintessential Visual Organization 77
Netflix 1.0: Upsetting the Applecart 77
Netflix 2.0: Self-Cannibalization 78
Dataviz: Part of a Holistic Big Data Strategy 80
Dataviz: Imbued in the Netflix Culture 81
Customer Insights 82
Better Technical and Network Diagnostics 84
Embracing the Community 88
Lessons 89
Next 90
Notes 90
Chapter 4 Dataviz in the DNA 93
The Beginnings 94
UX Is Paramount 95
The Plumbing 97
Embracing Free and Open-Source Tools 98
Extensive Use of APIs 101
Lessons 101
Next 102
Note 102
Chapter 5 Transparency in Texas 103
Background 104
Early Dataviz Efforts 105
Embracing Traditional BI 106
Data Discovery 107
Better Visibility into Student Life 108
Expansion: Spreading Dataviz Throughout the System 110
Results 111
Lessons 113
Next 113
Notes 114
Part III Getting Started: Becoming a Visual Organization 115
Chapter 6 The Four-Level Visual Organization Framework 117
Big Disclaimers 118
A Simple Model 119
Limits and Clarifications 120
Progression 122
Is Progression Always Linear? 123
Can a Small Organization Best Position Itself to Reach Levels 3 and 4? If
So, How? 123
Can an Organization Start at Level 3 or 4 and Build from the Top Down? 123
Is Intralevel Progression Possible? 123
Are Intralevel and Interlevel Progression Inevitable? 123
Can Different Parts of the Organization Exist on Different Levels? 124
Should an Organization Struggling with Levels 1 and 2 Attempt to Move to
Level 3 or 4? 124
Regression: Reversion to Lower Levels 124
Complements, Not Substitutes 125
Accumulated Advantage 125
The Limits of Lower Levels 125
Relativity and Sublevels 125
Should Every Organization Aspire to Level 4? 126
Next 126
Chapter 7 WWVOD? 127
Visualizing the Impact of a Reorg 128
Visualizing Employee Movement 129
Starting Down the Dataviz Path 129
Results and Lessons 133
Future 135
A Marketing Example 136
Next 137
Notes 137
Chapter 8 Building the Visual Organization 139
Data Tips and Best Practices 139
Data: The Primordial Soup 139
Walk Before You Run . . . At Least for Now 140
A Dataviz Is Often Just the Starting Point 140
Visualize Both Small and Big Data 141
Don't Forget the Metadata 141
Look Outside of the Enterprise 143
The Beginnings: All Data Is Not Required 143
Visualize Good and Bad Data 144
Enable Drill-Down 144
Design Tips and Best Practices 148
Begin with the End in Mind (Sort of) 148
Subtract When Possible 150
UX: Participation and Experimentation Are Paramount 150
Encourage Interactivity 151
Use Motion and Animation Carefully 151
Use Relative-Not Absolute-Figures 151
Technology Tips and Best Practices 152
Where Possible, Consider Using APIs 152
Embrace New Tools 152
Know the Limitations of Dataviz Tools 153
Be Open 153
Management Tips and Best Practices 154
Encourage Self-Service, Exploration, and Data Democracy 154
Exhibit a Healthy Skepticism 154
Trust the Process, Not the Result 155
Avoid the Perils of Silos and Specialization 156
If Possible, Visualize 156
Seek Hybrids When Hiring 157
Think Direction First, Precision Later 157
Next 158
Notes 158
Chapter 9 The Inhibitors: Mistakes, Myths, and Challenges 159
Mistakes 160
Falling into the Traditional ROI Trap 160
Always-and Blindly-Trusting a Dataviz 161
Ignoring the Audience 162
Developing in a Cathedral 162
Set It and Forget It 162
Bad Dataviz 163
TMI 163
Using Tiny Graphics 163
Myths 165
Data-visualizations Guarantee Certainty and Success 165
Data Visualization Is Easy 165
Data Visualizations Are Projects 166
There Is One "Right" Visualization 166
Excel Is Sufficient 167
Challenges 167
The Quarterly Visualization Mentality 167
Data Defiance 168
Unlearning History: Overcoming the Disappointments of Prior Tools 168
Next 169
Notes 169
Part IV Conclusion and the Future of Dataviz 171
Coda: We're Just Getting Started 173
Four Critical Data-Centric Trends 175
Wearable Technology and the Quantified Self 175
Machine Learning and the Internet of Things 176
Multidimensional Data 177
The Forthcoming Battle Over Data Portability and Ownership 179
Final Thoughts: Nothing Stops This Train 181
Notes 182
Afterword: My Life in Data 183
Appendix: Supplemental Dataviz Resources 187
Selected Bibliography 191
About the Author 193
Index 195
Preface xix
Acknowledgments xxv
How to Help This Book xxvii
Part I Book Overview and Background 1
Introduction 3
Adventures in Twitter Data Discovery 4
Contemporary Dataviz 101 9
Primary Objective 9
Benefits 11
More Important Than Ever 13
Revenge of the Laggards: The Current State of Dataviz 15
Book Overview 18
Defining the Visual Organization 19
Central Thesis of Book 19
Cui Bono? 20
Methodology: Story Matters Here 21
The Quest for Knowledge and Case Studies 24
Differentiation: A Note on Other Dataviz Texts 25
Plan of Attack 26
Next 27
Notes 27
Chapter 1 The Ascent of the Visual Organization 29
The Rise of Big Data 30
Open Data 30
The Burgeoning Data Ecosystem 33
The New Web: Visual, Semantic, and API-Driven 34
The Arrival of the Visual Web 34
Linked Data and a More Semantic Web 35
The Relative Ease of Accessing Data 36
Greater Efficiency via Clouds and Data Centers 37
Better Data Tools 38
Greater Organizational Transparency 40
The Copycat Economy: Monkey See, Monkey Do 41
Data Journalism and the Nate Silver Effect 41
Digital Man 44
The Arrival of the Visual Citizen 44
Mobility 47
The Visual Employee: A More Tech- and Data-Savvy Workforce 47
Navigating Our Data-Driven World 48
Next 49
Notes 49
Chapter 2 Transforming Data into Insights: The Tools 51
Dataviz: Part of an Intelligent and Holistic Strategy 52
The Tyranny of Terminology: Dataviz, BI, Reporting, Analytics, and KPIs 53
Do Visual Organizations Eschew All Tried-and-True Reporting Tools? 55
Drawing Some Distinctions 56
The Dataviz Fab Five 57
Applications from Large Enterprise Software Vendors 57
LESVs: The Case For 58
LESVs: The Case Against 59
Best-of-Breed Applications 61
Cost 62
Ease of Use and Employee Training 62
Integration and the Big Data World 63
Popular Open-Source Tools 64
D3.js 64
R 65
Others 66
Design Firms 66
Startups, Web Services, and Additional Resources 70
The Final Word: One Size Doesn't Fit All 72
Next 73
Notes 73
Part II Introducing the Visual Organization 75
Chapter 3 The Quintessential Visual Organization 77
Netflix 1.0: Upsetting the Applecart 77
Netflix 2.0: Self-Cannibalization 78
Dataviz: Part of a Holistic Big Data Strategy 80
Dataviz: Imbued in the Netflix Culture 81
Customer Insights 82
Better Technical and Network Diagnostics 84
Embracing the Community 88
Lessons 89
Next 90
Notes 90
Chapter 4 Dataviz in the DNA 93
The Beginnings 94
UX Is Paramount 95
The Plumbing 97
Embracing Free and Open-Source Tools 98
Extensive Use of APIs 101
Lessons 101
Next 102
Note 102
Chapter 5 Transparency in Texas 103
Background 104
Early Dataviz Efforts 105
Embracing Traditional BI 106
Data Discovery 107
Better Visibility into Student Life 108
Expansion: Spreading Dataviz Throughout the System 110
Results 111
Lessons 113
Next 113
Notes 114
Part III Getting Started: Becoming a Visual Organization 115
Chapter 6 The Four-Level Visual Organization Framework 117
Big Disclaimers 118
A Simple Model 119
Limits and Clarifications 120
Progression 122
Is Progression Always Linear? 123
Can a Small Organization Best Position Itself to Reach Levels 3 and 4? If
So, How? 123
Can an Organization Start at Level 3 or 4 and Build from the Top Down? 123
Is Intralevel Progression Possible? 123
Are Intralevel and Interlevel Progression Inevitable? 123
Can Different Parts of the Organization Exist on Different Levels? 124
Should an Organization Struggling with Levels 1 and 2 Attempt to Move to
Level 3 or 4? 124
Regression: Reversion to Lower Levels 124
Complements, Not Substitutes 125
Accumulated Advantage 125
The Limits of Lower Levels 125
Relativity and Sublevels 125
Should Every Organization Aspire to Level 4? 126
Next 126
Chapter 7 WWVOD? 127
Visualizing the Impact of a Reorg 128
Visualizing Employee Movement 129
Starting Down the Dataviz Path 129
Results and Lessons 133
Future 135
A Marketing Example 136
Next 137
Notes 137
Chapter 8 Building the Visual Organization 139
Data Tips and Best Practices 139
Data: The Primordial Soup 139
Walk Before You Run . . . At Least for Now 140
A Dataviz Is Often Just the Starting Point 140
Visualize Both Small and Big Data 141
Don't Forget the Metadata 141
Look Outside of the Enterprise 143
The Beginnings: All Data Is Not Required 143
Visualize Good and Bad Data 144
Enable Drill-Down 144
Design Tips and Best Practices 148
Begin with the End in Mind (Sort of) 148
Subtract When Possible 150
UX: Participation and Experimentation Are Paramount 150
Encourage Interactivity 151
Use Motion and Animation Carefully 151
Use Relative-Not Absolute-Figures 151
Technology Tips and Best Practices 152
Where Possible, Consider Using APIs 152
Embrace New Tools 152
Know the Limitations of Dataviz Tools 153
Be Open 153
Management Tips and Best Practices 154
Encourage Self-Service, Exploration, and Data Democracy 154
Exhibit a Healthy Skepticism 154
Trust the Process, Not the Result 155
Avoid the Perils of Silos and Specialization 156
If Possible, Visualize 156
Seek Hybrids When Hiring 157
Think Direction First, Precision Later 157
Next 158
Notes 158
Chapter 9 The Inhibitors: Mistakes, Myths, and Challenges 159
Mistakes 160
Falling into the Traditional ROI Trap 160
Always-and Blindly-Trusting a Dataviz 161
Ignoring the Audience 162
Developing in a Cathedral 162
Set It and Forget It 162
Bad Dataviz 163
TMI 163
Using Tiny Graphics 163
Myths 165
Data-visualizations Guarantee Certainty and Success 165
Data Visualization Is Easy 165
Data Visualizations Are Projects 166
There Is One "Right" Visualization 166
Excel Is Sufficient 167
Challenges 167
The Quarterly Visualization Mentality 167
Data Defiance 168
Unlearning History: Overcoming the Disappointments of Prior Tools 168
Next 169
Notes 169
Part IV Conclusion and the Future of Dataviz 171
Coda: We're Just Getting Started 173
Four Critical Data-Centric Trends 175
Wearable Technology and the Quantified Self 175
Machine Learning and the Internet of Things 176
Multidimensional Data 177
The Forthcoming Battle Over Data Portability and Ownership 179
Final Thoughts: Nothing Stops This Train 181
Notes 182
Afterword: My Life in Data 183
Appendix: Supplemental Dataviz Resources 187
Selected Bibliography 191
About the Author 193
Index 195