Martin A. Tanner
Tools for Statistical Inference (eBook, PDF)
Methods for the Exploration of Posterior Distributions and Likelihood Functions
89,95 €
89,95 €
inkl. MwSt.
Sofort per Download lieferbar
45 °P sammeln
89,95 €
Als Download kaufen
89,95 €
inkl. MwSt.
Sofort per Download lieferbar
45 °P sammeln
Jetzt verschenken
Alle Infos zum eBook verschenken
89,95 €
inkl. MwSt.
Sofort per Download lieferbar
Alle Infos zum eBook verschenken
45 °P sammeln
Martin A. Tanner
Tools for Statistical Inference (eBook, PDF)
Methods for the Exploration of Posterior Distributions and Likelihood Functions
- Format: PDF
- Merkliste
- Auf die Merkliste
- Bewerten Bewerten
- Teilen
- Produkt teilen
- Produkterinnerung
- Produkterinnerung
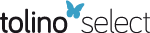
Bitte loggen Sie sich zunächst in Ihr Kundenkonto ein oder registrieren Sie sich bei
bücher.de, um das eBook-Abo tolino select nutzen zu können.
Hier können Sie sich einloggen
Hier können Sie sich einloggen
Sie sind bereits eingeloggt. Klicken Sie auf 2. tolino select Abo, um fortzufahren.
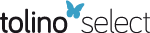
Bitte loggen Sie sich zunächst in Ihr Kundenkonto ein oder registrieren Sie sich bei bücher.de, um das eBook-Abo tolino select nutzen zu können.
A unified introduction to a variety of computational algorithms for likelihood and Bayesian inference. This third edition expands the discussion of many of the techniques presented, and includes additional examples as well as exercise sets at the end of each chapter.
- Geräte: PC
- ohne Kopierschutz
- eBook Hilfe
- Größe: 14.32MB
Andere Kunden interessierten sich auch für
- Martin A. TannerTools for Statistical Inference (eBook, PDF)62,95 €
- Empirical Bayes and Likelihood Inference (eBook, PDF)40,95 €
- Jun ShaoThe Jackknife and Bootstrap (eBook, PDF)258,95 €
- Bayesian Inference in Wavelet-Based Models (eBook, PDF)73,95 €
- Generalized Linear Models (eBook, PDF)73,95 €
- Statistical Modelling (eBook, PDF)73,95 €
- Case Studies in Bayesian Statistics (eBook, PDF)73,95 €
-
-
-
A unified introduction to a variety of computational algorithms for likelihood and Bayesian inference. This third edition expands the discussion of many of the techniques presented, and includes additional examples as well as exercise sets at the end of each chapter.
Dieser Download kann aus rechtlichen Gründen nur mit Rechnungsadresse in A, B, BG, CY, CZ, D, DK, EW, E, FIN, F, GR, HR, H, IRL, I, LT, L, LR, M, NL, PL, P, R, S, SLO, SK ausgeliefert werden.
Produktdetails
- Produktdetails
- Verlag: Springer US
- Seitenzahl: 208
- Erscheinungstermin: 6. Dezember 2012
- Englisch
- ISBN-13: 9781461240242
- Artikelnr.: 43991556
- Verlag: Springer US
- Seitenzahl: 208
- Erscheinungstermin: 6. Dezember 2012
- Englisch
- ISBN-13: 9781461240242
- Artikelnr.: 43991556
- Herstellerkennzeichnung Die Herstellerinformationen sind derzeit nicht verfügbar.
1. Introduction.- Exercises.- 2. Normal Approximations to Likelihoods and to Posteriors.- 2.1. Likelihood/Posterior Density.- 2.2. Specification of the Prior.- 2.3. Maximum Likelihood.- 2.4. Normal-Based Inference.- 2.5. The ?-Method (Propagation of Errors).- 2.6. Highest Posterior Density Regions.- Exercises.- 3. Nonnormal Approximations to Likelihoods and Posteriors.- 3.1. Numerical Integration.- 3.2. Posterior Moments and Marginalization Based on Laplace's Method.- 3.3. Monte Carlo Methods.- Exercises.- 4. The EM Algorithm.- 4.1. Introduction.- 4.2. Theory.- 4.3. EM in the Exponential Family.- 4.4. Standard Errors in the Context of EM.- 4.5. Monte Carlo Implementation of the E-Step.- 4.6. Acceleration of EM (Louis' Turbo EM).- 4.7. Facilitating the M-Step.- Exercises.- 5. The Data Augmentation Algorithm.- 5.1. Introduction and Motivation.- 5.2. Computing and Sampling from the Predictive Distribution.- 5.3. Calculating the Content and Boundary of the HPD Region.- 5.4. Remarks on the General Implementation of the Data Augmentation Algorithm.- 5.5. Overview of the Convergence Theory of Data Augmentation.- 5.6. Poor Man's Data Augmentation Algorithms.- 5.7. Sampling/Importance Resampling (SIR).- 5.8. General Imputation Methods.- 5.9. Further Importance Sampling Ideas.- 5.10. Sampling in the Context of Multinomial Data.- Exercises.- 6. Markov Chain Monte Carlo: The Gibbs Sampler and the Metropolis Algorithm.- 6.1. Introduction to the Gibbs Sampler.- 6.2. Examples.- 6.3. Assessing Convergence of the Chain.- 6.4. The Griddy Gibbs Sampler.- 6.5. The Metropolis Algorithm.- 6.6. Conditional Inference via the Gibbs Sampler.- Exercises.- References.
1. Introduction.- Exercises.- 2. Normal Approximations to Likelihoods and to Posteriors.- 2.1. Likelihood/Posterior Density.- 2.2. Specification of the Prior.- 2.3. Maximum Likelihood.- 2.4. Normal-Based Inference.- 2.5. The ?-Method (Propagation of Errors).- 2.6. Highest Posterior Density Regions.- Exercises.- 3. Nonnormal Approximations to Likelihoods and Posteriors.- 3.1. Numerical Integration.- 3.2. Posterior Moments and Marginalization Based on Laplace's Method.- 3.3. Monte Carlo Methods.- Exercises.- 4. The EM Algorithm.- 4.1. Introduction.- 4.2. Theory.- 4.3. EM in the Exponential Family.- 4.4. Standard Errors in the Context of EM.- 4.5. Monte Carlo Implementation of the E-Step.- 4.6. Acceleration of EM (Louis' Turbo EM).- 4.7. Facilitating the M-Step.- Exercises.- 5. The Data Augmentation Algorithm.- 5.1. Introduction and Motivation.- 5.2. Computing and Sampling from the Predictive Distribution.- 5.3. Calculating the Content and Boundary of the HPD Region.- 5.4. Remarks on the General Implementation of the Data Augmentation Algorithm.- 5.5. Overview of the Convergence Theory of Data Augmentation.- 5.6. Poor Man's Data Augmentation Algorithms.- 5.7. Sampling/Importance Resampling (SIR).- 5.8. General Imputation Methods.- 5.9. Further Importance Sampling Ideas.- 5.10. Sampling in the Context of Multinomial Data.- Exercises.- 6. Markov Chain Monte Carlo: The Gibbs Sampler and the Metropolis Algorithm.- 6.1. Introduction to the Gibbs Sampler.- 6.2. Examples.- 6.3. Assessing Convergence of the Chain.- 6.4. The Griddy Gibbs Sampler.- 6.5. The Metropolis Algorithm.- 6.6. Conditional Inference via the Gibbs Sampler.- Exercises.- References.