
Broschiertes Buch
Applications to Chemical Processes
1. Mai 2025
Elsevier Science
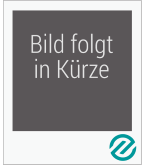
Broschiertes Buch
Elsevier Science
Ähnliche Artikel
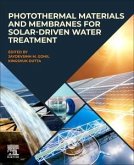
Broschiertes Buch
24. Januar 2025
Elsevier Science
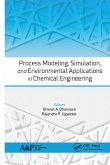
Broschiertes Buch
31. März 2021
Apple Academic Press
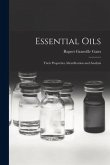
18,99 €
Versandfertig in über 4 Wochen
Broschiertes Buch
9. September 2021
Creative Media Partners, LLC
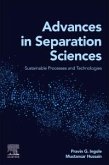
Broschiertes Buch
Sustainable Processes and Technologies
3. Dezember 2024
Elsevier Science
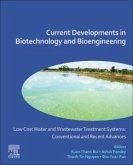
Broschiertes Buch
Current Developments in Biotechnology and Bioengineering
18. Oktober 2024
Elsevier Science
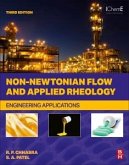
Broschiertes Buch
Engineering Applications
3rd edition
15. Februar 2025
Elsevier Science
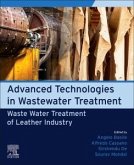
Broschiertes Buch
Waste Water Treatment of Leather Industry
28. November 2024
Elsevier Science
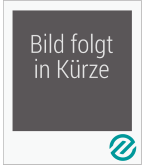
Broschiertes Buch
4. Februar 2025
Elsevier Science
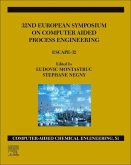
Broschiertes Buch
Escape-32 Volume 51
10. Juni 2022
Elsevier Science
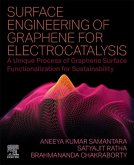
Broschiertes Buch
A Unique Process of Graphene Surface Functionalization for Sustainability
1. Februar 2025
Elsevier Science
Ähnlichkeitssuche: Fact®Finder von OMIKRON