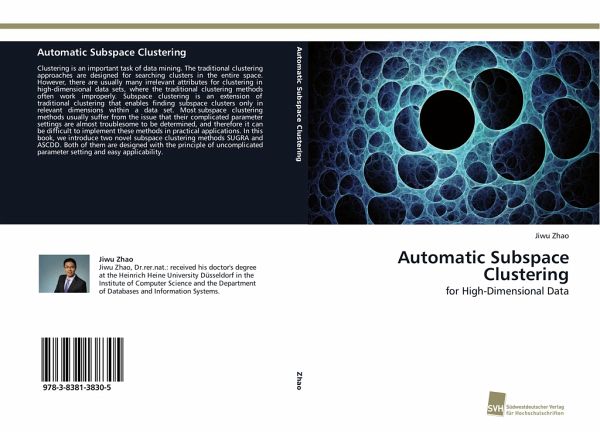
Automatic Subspace Clustering
for High-Dimensional Data
Versandkostenfrei!
Versandfertig in 6-10 Tagen
39,99 €
inkl. MwSt.
PAYBACK Punkte
20 °P sammeln!
Clustering is an important task of data mining. The traditional clustering approaches are designed for searching clusters in the entire space. However, there are usually many irrelevant attributes for clustering in high-dimensional data sets, where the traditional clustering methods often work improperly. Subspace clustering is an extension of traditional clustering that enables finding subspace clusters only in relevant dimensions within a data set. Most subspace clustering methods usually suffer from the issue that their complicated parameter settings are almost troublesome to be determined,...
Clustering is an important task of data mining. The traditional clustering approaches are designed for searching clusters in the entire space. However, there are usually many irrelevant attributes for clustering in high-dimensional data sets, where the traditional clustering methods often work improperly. Subspace clustering is an extension of traditional clustering that enables finding subspace clusters only in relevant dimensions within a data set. Most subspace clustering methods usually suffer from the issue that their complicated parameter settings are almost troublesome to be determined, and therefore it can be difficult to implement these methods in practical applications. In this book, we introduce two novel subspace clustering methods SUGRA and ASCDD. Both of them are designed with the principle of uncomplicated parameter setting and easy applicability.