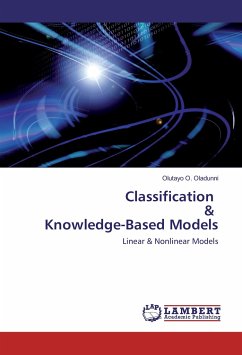
Classification & Knowledge-Based Models
Linear & Nonlinear Models
Versandkostenfrei!
Versandfertig in 6-10 Tagen
38,99 €
inkl. MwSt.
PAYBACK Punkte
19 °P sammeln!
This book presents a review and development of classification and knowledge-based methods. It focuses on linearly and nonlinearly separable binary classification problems, which are then generalized to solve multi-categorical discrimination problems. In the case of nonlinearly separable classification problems, kernel functions are used to calculate the similarity or dissimilarity of the input vectors and the functional values are used to create a positive definite kernel matrix and reduced kernel matrix. Using a regularized least squares cost function the resulting optimization problem is exp...
This book presents a review and development of classification and knowledge-based methods. It focuses on linearly and nonlinearly separable binary classification problems, which are then generalized to solve multi-categorical discrimination problems. In the case of nonlinearly separable classification problems, kernel functions are used to calculate the similarity or dissimilarity of the input vectors and the functional values are used to create a positive definite kernel matrix and reduced kernel matrix. Using a regularized least squares cost function the resulting optimization problem is expressed as a single unconstrained optimization problem which can be solved by matrix and/or iterative numerical methods. Advantages of this formulation include the explicit expressions used in the classification weights of the classifier(s); the model's ability to incorporate several classes in a single formulation and to provide quick solutions to the optimal classification weights for multi-categorical separation; and its ability to provide solutions without the use of specialized solver software.