Tiffany Timbers, Trevor Campbell, Melissa Lee, Joel Ostblom, Lindsey Heagy
Data Science (eBook, ePUB)
A First Introduction with Python
62,95 €
62,95 €
inkl. MwSt.
Sofort per Download lieferbar
31 °P sammeln
62,95 €
Als Download kaufen
62,95 €
inkl. MwSt.
Sofort per Download lieferbar
31 °P sammeln
Jetzt verschenken
Alle Infos zum eBook verschenken
62,95 €
inkl. MwSt.
Sofort per Download lieferbar
Alle Infos zum eBook verschenken
31 °P sammeln
Tiffany Timbers, Trevor Campbell, Melissa Lee, Joel Ostblom, Lindsey Heagy
Data Science (eBook, ePUB)
A First Introduction with Python
- Format: ePub
- Merkliste
- Auf die Merkliste
- Bewerten Bewerten
- Teilen
- Produkt teilen
- Produkterinnerung
- Produkterinnerung
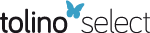
Bitte loggen Sie sich zunächst in Ihr Kundenkonto ein oder registrieren Sie sich bei
bücher.de, um das eBook-Abo tolino select nutzen zu können.
Hier können Sie sich einloggen
Hier können Sie sich einloggen
Sie sind bereits eingeloggt. Klicken Sie auf 2. tolino select Abo, um fortzufahren.
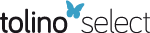
Bitte loggen Sie sich zunächst in Ihr Kundenkonto ein oder registrieren Sie sich bei bücher.de, um das eBook-Abo tolino select nutzen zu können.
Data Science: A First Introduction with Python focuses on using the Python programming language in Jupyter notebooks to perform data manipulation and cleaning, create effective visualizations, and extract insights from data using classification, regression, clustering, and inference.
- Geräte: eReader
- mit Kopierschutz
- eBook Hilfe
Andere Kunden interessierten sich auch für
- Jerry BonnellExploring Data Science with R and the Tidyverse (eBook, ePUB)81,95 €
- Tiffany TimbersData Science (eBook, PDF)62,95 €
- Taylor R. BrownAn Introduction to R and Python for Data Analysis (eBook, ePUB)52,95 €
- Jerry BonnellExploring Data Science with R and the Tidyverse (eBook, PDF)81,95 €
- David K. GosserR for Quantitative Chemistry (eBook, ePUB)59,95 €
- Ramzi W. NahhasIntroduction to Regression Methods for Public Health Using R (eBook, ePUB)52,95 €
- Enrique Garcia CejaBehavior Analysis with Machine Learning Using R (eBook, ePUB)48,95 €
-
-
-
Data Science: A First Introduction with Python focuses on using the Python programming language in Jupyter notebooks to perform data manipulation and cleaning, create effective visualizations, and extract insights from data using classification, regression, clustering, and inference.
Dieser Download kann aus rechtlichen Gründen nur mit Rechnungsadresse in A, B, BG, CY, CZ, D, DK, EW, E, FIN, F, GR, HR, H, IRL, I, LT, L, LR, M, NL, PL, P, R, S, SLO, SK ausgeliefert werden.
Produktdetails
- Produktdetails
- Verlag: Taylor & Francis eBooks
- Erscheinungstermin: 23. August 2024
- Englisch
- ISBN-13: 9781040106709
- Artikelnr.: 72286891
- Verlag: Taylor & Francis eBooks
- Erscheinungstermin: 23. August 2024
- Englisch
- ISBN-13: 9781040106709
- Artikelnr.: 72286891
- Herstellerkennzeichnung Die Herstellerinformationen sind derzeit nicht verfügbar.
Tiffany Timbers is an Associate Professor of Teaching in the Department of Statistics and Co-Director for the Master of Data Science program (Vancouver Option) at the University of British Columbia. In these roles she teaches and develops curriculum around the responsible application of Data Science to solve real-world problems. One of her favourite courses she teaches is a graduate course on collaborative software development, which focuses on teaching how to create R and Python packages using modern tools and workflows.
Trevor Campbell is an Associate Professor in the Department of Statistics at the University of British Columbia. His research focuses on automated, scalable Bayesian inference algorithms, Bayesian nonparametrics, streaming data, and Bayesian theory. He was previously a postdoctoral associate in the Computer Science and Artificial Intelligence Laboratory (CSAIL) and Institute for Data, Systems, and Society (IDSS) at MIT and a Ph.D. candidate in the Laboratory for Information and Decision Systems (LIDS) at MIT.
Melissa Lee is an Assistant Professor of Teaching in the Department of Statistics at the University of British Columbia. She teaches and develops curriculum for undergraduate statistics and data science courses. Her work focuses on student-centered approaches to teaching, developing and assessing open educational resources, and promoting equity, diversity, and inclusion initiatives.
Joel Ostblom is an Assistant Professor of Teaching in the Statistics Department at the University of British Columbia. He teaches and develops data science courses at the graduate and undergraduate level, with a focus on data visualization, data science ethics, and machine learning. Joel cares deeply about spreading data literacy and excitement over programmatic data analysis, which is reflected in his contributions to open source projects and openly accessible data science learning resources.
Lindsey Heagy is an Assistant Professor in the Department of Earth, Ocean and Atmospheric Sciences and Director of the Geophysical Inversion Facility at UBC. Her research combines computational methods in numerical simulations, inversions, and machine learning for using geophysical data to characterize the subsurface. Primary applications of interest include mineral exploration, carbon sequestration, groundwater, and environmental studies.
Trevor Campbell is an Associate Professor in the Department of Statistics at the University of British Columbia. His research focuses on automated, scalable Bayesian inference algorithms, Bayesian nonparametrics, streaming data, and Bayesian theory. He was previously a postdoctoral associate in the Computer Science and Artificial Intelligence Laboratory (CSAIL) and Institute for Data, Systems, and Society (IDSS) at MIT and a Ph.D. candidate in the Laboratory for Information and Decision Systems (LIDS) at MIT.
Melissa Lee is an Assistant Professor of Teaching in the Department of Statistics at the University of British Columbia. She teaches and develops curriculum for undergraduate statistics and data science courses. Her work focuses on student-centered approaches to teaching, developing and assessing open educational resources, and promoting equity, diversity, and inclusion initiatives.
Joel Ostblom is an Assistant Professor of Teaching in the Statistics Department at the University of British Columbia. He teaches and develops data science courses at the graduate and undergraduate level, with a focus on data visualization, data science ethics, and machine learning. Joel cares deeply about spreading data literacy and excitement over programmatic data analysis, which is reflected in his contributions to open source projects and openly accessible data science learning resources.
Lindsey Heagy is an Assistant Professor in the Department of Earth, Ocean and Atmospheric Sciences and Director of the Geophysical Inversion Facility at UBC. Her research combines computational methods in numerical simulations, inversions, and machine learning for using geophysical data to characterize the subsurface. Primary applications of interest include mineral exploration, carbon sequestration, groundwater, and environmental studies.
Preface Foreword Acknowledgments 1. Python and Pandas 2. Reading in data
locally and from the web 3. Cleaning and wrangling data 4. Effective data
visualization 5. Classification I: training & predicting 6. Classification
II: evaluation & tuning 7. Regression I: K-nearest neighbors 8. Regression
II: linear regression 9. Clustering 10. Statistical inference 11. Combining
code and text with Jupyter 12. Collaboration with version control 13.
Setting up your computer Bibliography Index
locally and from the web 3. Cleaning and wrangling data 4. Effective data
visualization 5. Classification I: training & predicting 6. Classification
II: evaluation & tuning 7. Regression I: K-nearest neighbors 8. Regression
II: linear regression 9. Clustering 10. Statistical inference 11. Combining
code and text with Jupyter 12. Collaboration with version control 13.
Setting up your computer Bibliography Index
Preface Foreword Acknowledgments 1. Python and Pandas 2. Reading in data
locally and from the web 3. Cleaning and wrangling data 4. Effective data
visualization 5. Classification I: training & predicting 6. Classification
II: evaluation & tuning 7. Regression I: K-nearest neighbors 8. Regression
II: linear regression 9. Clustering 10. Statistical inference 11. Combining
code and text with Jupyter 12. Collaboration with version control 13.
Setting up your computer Bibliography Index
locally and from the web 3. Cleaning and wrangling data 4. Effective data
visualization 5. Classification I: training & predicting 6. Classification
II: evaluation & tuning 7. Regression I: K-nearest neighbors 8. Regression
II: linear regression 9. Clustering 10. Statistical inference 11. Combining
code and text with Jupyter 12. Collaboration with version control 13.
Setting up your computer Bibliography Index