Multiscale Analysis of Complex Time Series (eBook, PDF)
Integration of Chaos and Random Fractal Theory, and Beyond
Alle Infos zum eBook verschenken
Multiscale Analysis of Complex Time Series (eBook, PDF)
Integration of Chaos and Random Fractal Theory, and Beyond
- Format: PDF
- Merkliste
- Auf die Merkliste
- Bewerten Bewerten
- Teilen
- Produkt teilen
- Produkterinnerung
- Produkterinnerung
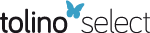
Hier können Sie sich einloggen
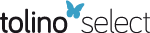
Bitte loggen Sie sich zunächst in Ihr Kundenkonto ein oder registrieren Sie sich bei bücher.de, um das eBook-Abo tolino select nutzen zu können.
The only integrative approach to chaos and random fractal theory Chaos and random fractal theory are two of the most important theories developed for data analysis. Until now, there has been no single book that encompasses all of the basic concepts necessary for researchers to fully understand the ever-expanding literature and apply novel methods to effectively solve their signal processing problems. Multiscale Analysis of Complex Time Series fills this pressing need by presenting chaos and random fractal theory in a unified manner. Adopting a data-driven approach, the book covers: * DNA…mehr
- Geräte: PC
- mit Kopierschutz
- eBook Hilfe
- Größe: 15.55MB
- Guanrong ChenFundamentals of Complex Networks (eBook, PDF)100,99 €
- Guanrong ChenFundamentals of Complex Networks (eBook, ePUB)100,99 €
- Hiroshi YabunoLinear and Nonlinear Instabilities in Mechanical Systems (eBook, PDF)93,99 €
- Radomir S. StankovicFourier Analysis on Finite Groups with Applications in Signal Processing and System Design (eBook, PDF)113,99 €
- Christopher J. ZarowskiAn Introduction to Numerical Analysis for Electrical and Computer Engineers (eBook, PDF)195,99 €
- Change Detection and Image Time-Series Analysis 2 (eBook, PDF)139,99 €
- Ulrich NehmzowScientific Methods in Mobile Robotics (eBook, PDF)113,95 €
-
-
-
Dieser Download kann aus rechtlichen Gründen nur mit Rechnungsadresse in A, B, BG, CY, CZ, D, DK, EW, E, FIN, F, GR, HR, H, IRL, I, LT, L, LR, M, NL, PL, P, R, S, SLO, SK ausgeliefert werden.
- Produktdetails
- Verlag: John Wiley & Sons
- Seitenzahl: 368
- Erscheinungstermin: 12. Juli 2008
- Englisch
- ISBN-13: 9780470191644
- Artikelnr.: 37291512
- Verlag: John Wiley & Sons
- Seitenzahl: 368
- Erscheinungstermin: 12. Juli 2008
- Englisch
- ISBN-13: 9780470191644
- Artikelnr.: 37291512
- Herstellerkennzeichnung Die Herstellerinformationen sind derzeit nicht verfügbar.
ß processes. 10.3 Application: Modeling heterogeneous Internet traffic. 10.3.1 General considerations. 10.3.2 An example. 10.4 Bibliographic notes. 10.5 Exercises. 11. Models of power-law-type behavior. 11.1 Models for heavy-tailed distribution. 11.1.1 Power-law through queuing. 11.1.2 Power-law through approximation by log-normal distribution. 11.1.3 Power-law through transformation of exponential distribution. 11.1.4 Power-law through maximization of Tsallis nonextensive entropy. 11.1.5 Power-law through optimization. 11.2 Models for 1/
ß processes. 11.2.1 1/
ß processes from superposition of relaxation processes. 11.2.2 1/
ß processes modeled by ON/OFF trains. 11.2.3 1/
ß processes modeled by self-organized criticality. 11.3 Applications. 11.3.1 Mechanism for long-range-dependent network traffic. 11.3.2 Distributional analysis of sea clutter. 11.4 Bibliographic notes. 11.5 Exercises. 12. Bifurcation theory. 12.1 Bifurcations from a steady solution in continuous time systems. 12.1.1 General considerations. 12.1.2 Saddle-node bifurcation. 12.1.3 Transcritical bifurcation. 12.1.4 Pitchfork bifurcation. 12.2 Bifurcations from a steady solution in discrete maps. 12.3 Bifurcations in high-dimensional space. 12.4 Bifurcations and fault-tolerant computations. 12.4.1 Error threshold values for arbitrary K-input NAND gates. 12.4.2 Noisy majority gate. 12.4.3 Analysis of von Neumann's multiplexing system. 12.5 Bibliographic notes. 12.6 Exercises. 13. Chaotic time series analysis. 13.1 Phase space reconstruction by the time delay embedding. 13.1.1 General considerations. 13.1.2 Defending against network intrusions and worms. 13.1.3 Optimal embedding. 13.2 Characterization of chaotic attractors. 13.2.1 Dimension. 13.2.2 Lyapunov exponents. 13.2.3 Entropy. 13.3 Test for low-dimensional chaos. 13.4 The importance of the concept of scale. 13.5 Bibliographic notes. 13.6 Exercises. 14. Power-law sensitivity to initial conditions (PSIC). 14.1 Extending exponential sensitivity to initial conditions to PSIC. 14.2 Characterizing random fractals by PSIC. 14.2.1 Characterizing 1/
ß processes by PSIC. 14.2.2 Characterizing Levy processes by PSIC. 14.3 Characterizing edge of chaos by PSIC. 14.4 Bibliographic notes. 15. Multiscale analysis by the scale-dependent Lyapunov exponent (SDLE). 15.1 Basic theory. 15.2 Classification of complex motions. 15.2.1 Chaos, noisy chaos, and noise-induced chaos. 15.2.2 1/
ß processes. 15.2.3 Levy flights. 15.2.4 SDLE for processes defined by PSIC. 15.2.5 Stochastic oscillations. 15.2.6 Complex motions with multiple scaling behaviors. 15.3 Distinguishing chaos from noise. 15.3.1 General considerations. 15.3.2 Distinguishing chaos from noise. 15.4 Characterizing hidden frequencies. 15.5 Coping with nonstationarity. 15.6 Relation between SDLE and other complexity measures. 15.7 Broad applications. 15.7.1 EEG analysis. 15.7.2 HRV analysis. 15.7.3 Economic time series analysis. 15.7.4 Sea clutter modeling. 15.8 Bibliographic notes. Appendix A: Description of data. A.1 Network traffic data. A.2 Sea clutter data. A.3 Neuronal firing data. A.4 Other data and program listings. Appendix B: Principal Component Analysis (PCA), Singular Value. Decomposition (SVD), and Karhunen-Loève (KL) expansion. Appendix C: Complexity measures. C.1 Finite size Lyapunov exponent (FSLE). C.2 Lempel-Ziv complexity. C.3 Permutation entropy. References. Index.
ß processes. 10.3 Application: Modeling heterogeneous Internet traffic. 10.3.1 General considerations. 10.3.2 An example. 10.4 Bibliographic notes. 10.5 Exercises. 11. Models of power-law-type behavior. 11.1 Models for heavy-tailed distribution. 11.1.1 Power-law through queuing. 11.1.2 Power-law through approximation by log-normal distribution. 11.1.3 Power-law through transformation of exponential distribution. 11.1.4 Power-law through maximization of Tsallis nonextensive entropy. 11.1.5 Power-law through optimization. 11.2 Models for 1/
ß processes. 11.2.1 1/
ß processes from superposition of relaxation processes. 11.2.2 1/
ß processes modeled by ON/OFF trains. 11.2.3 1/
ß processes modeled by self-organized criticality. 11.3 Applications. 11.3.1 Mechanism for long-range-dependent network traffic. 11.3.2 Distributional analysis of sea clutter. 11.4 Bibliographic notes. 11.5 Exercises. 12. Bifurcation theory. 12.1 Bifurcations from a steady solution in continuous time systems. 12.1.1 General considerations. 12.1.2 Saddle-node bifurcation. 12.1.3 Transcritical bifurcation. 12.1.4 Pitchfork bifurcation. 12.2 Bifurcations from a steady solution in discrete maps. 12.3 Bifurcations in high-dimensional space. 12.4 Bifurcations and fault-tolerant computations. 12.4.1 Error threshold values for arbitrary K-input NAND gates. 12.4.2 Noisy majority gate. 12.4.3 Analysis of von Neumann's multiplexing system. 12.5 Bibliographic notes. 12.6 Exercises. 13. Chaotic time series analysis. 13.1 Phase space reconstruction by the time delay embedding. 13.1.1 General considerations. 13.1.2 Defending against network intrusions and worms. 13.1.3 Optimal embedding. 13.2 Characterization of chaotic attractors. 13.2.1 Dimension. 13.2.2 Lyapunov exponents. 13.2.3 Entropy. 13.3 Test for low-dimensional chaos. 13.4 The importance of the concept of scale. 13.5 Bibliographic notes. 13.6 Exercises. 14. Power-law sensitivity to initial conditions (PSIC). 14.1 Extending exponential sensitivity to initial conditions to PSIC. 14.2 Characterizing random fractals by PSIC. 14.2.1 Characterizing 1/
ß processes by PSIC. 14.2.2 Characterizing Levy processes by PSIC. 14.3 Characterizing edge of chaos by PSIC. 14.4 Bibliographic notes. 15. Multiscale analysis by the scale-dependent Lyapunov exponent (SDLE). 15.1 Basic theory. 15.2 Classification of complex motions. 15.2.1 Chaos, noisy chaos, and noise-induced chaos. 15.2.2 1/
ß processes. 15.2.3 Levy flights. 15.2.4 SDLE for processes defined by PSIC. 15.2.5 Stochastic oscillations. 15.2.6 Complex motions with multiple scaling behaviors. 15.3 Distinguishing chaos from noise. 15.3.1 General considerations. 15.3.2 Distinguishing chaos from noise. 15.4 Characterizing hidden frequencies. 15.5 Coping with nonstationarity. 15.6 Relation between SDLE and other complexity measures. 15.7 Broad applications. 15.7.1 EEG analysis. 15.7.2 HRV analysis. 15.7.3 Economic time series analysis. 15.7.4 Sea clutter modeling. 15.8 Bibliographic notes. Appendix A: Description of data. A.1 Network traffic data. A.2 Sea clutter data. A.3 Neuronal firing data. A.4 Other data and program listings. Appendix B: Principal Component Analysis (PCA), Singular Value. Decomposition (SVD), and Karhunen-Loève (KL) expansion. Appendix C: Complexity measures. C.1 Finite size Lyapunov exponent (FSLE). C.2 Lempel-Ziv complexity. C.3 Permutation entropy. References. Index.