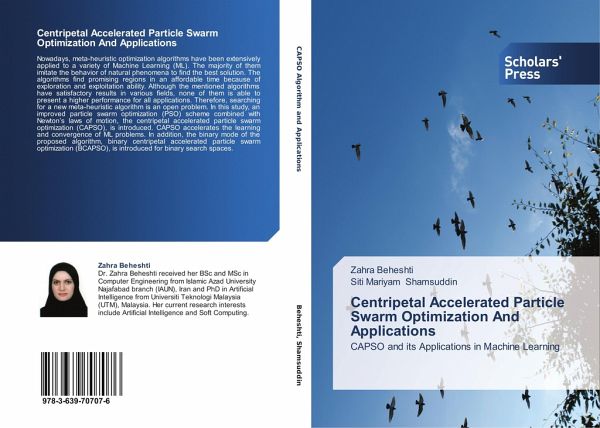
Centripetal Accelerated Particle Swarm Optimization And Applications
CAPSO and its Applications in Machine Learning
Versandkostenfrei!
Versandfertig in 6-10 Tagen
52,99 €
inkl. MwSt.
PAYBACK Punkte
26 °P sammeln!
Nowadays, meta-heuristic optimization algorithms have been extensively applied to a variety of Machine Learning (ML). The majority of them imitate the behavior of natural phenomena to find the best solution. The algorithms find promising regions in an affordable time because of exploration and exploitation ability. Although the mentioned algorithms have satisfactory results in various fields, none of them is able to present a higher performance for all applications. Therefore, searching for a new meta-heuristic algorithm is an open problem. In this study, an improved particle swarm optimizatio...
Nowadays, meta-heuristic optimization algorithms have been extensively applied to a variety of Machine Learning (ML). The majority of them imitate the behavior of natural phenomena to find the best solution. The algorithms find promising regions in an affordable time because of exploration and exploitation ability. Although the mentioned algorithms have satisfactory results in various fields, none of them is able to present a higher performance for all applications. Therefore, searching for a new meta-heuristic algorithm is an open problem. In this study, an improved particle swarm optimization (PSO) scheme combined with Newton s laws of motion, the centripetal accelerated particle swarm optimization (CAPSO), is introduced. CAPSO accelerates the learning and convergence of ML problems. In addition, the binary mode of the proposed algorithm, binary centripetal accelerated particle swarm optimization (BCAPSO), is introduced for binary search spaces.